- 1Charité – Universitätsmedizin Berlin, Klinik für Psychiatrie und Psychotherapie, Berlin, Germany
- 2Charité – Universitätsmedizin Berlin, Medizinische Klinik m.S. Psychosomatik, Berlin, Germany
- 3Charité – Universitätsmedizin Berlin, Experimental and Clinical Research Center, Berlin, Germany
- 4Charité – Universitätsmedizin Berlin, Neuroscience Clinical Research Center, Berlin, Germany
Magnetic resonance imaging (MRI) of the brain is commonly used to detect where chronic and active lesions are in multiple sclerosis (MS). MRI is also extensively used as a tool to calculate and extrapolate brain health by way of volumetric analysis or advanced imaging techniques. In MS patients, psychiatric symptoms are common comorbidities, with depression being the main one. Even though these symptoms are a major determinant of quality of life in MS, they are often overlooked and undertreated. There has been evidence of bidirectional interactions between the course of MS and comorbid psychiatric symptoms. In order to mitigate disability progression in MS, treating psychiatric comorbidities should be investigated and optimized. New research for the prediction of disease states or phenotypes of disability have advanced, primarily due to new technologies and a better understanding of the aging brain.
1. Introduction
Multiple sclerosis (MS) is a neuroinflammatory and chronic disease that affects the brain, spinal cord, and optic nerves (Thompson et al., 2018). With no permanent cure for this neurological disorder, it is a lifelong affection. Patients and clinicians must decide on optimal treatment regimens, which are often changed based on already observable increases in disability (e.g., motor, cognitive, bowel, and bladder) (Gajofatto and Benedetti, 2015; Dalmau, 2018). However, it has long been hypothesized and recently shown in large population-based cohorts, that the earlier this disease is treated, the better the prognostic outcome will be of these patients; even without evidence of disease activity (Stangel et al., 2015; Prosperini et al., 2018; Wandall-Holm et al., 2022). Thus, it would be of interest to all those researching brain health, neurological aging, and predictive modeling in neuroscience to be able to diagnose and treat patients prior to any manifestations of disease-related disability. Moreover, for a better prognostic outcome in MS patients, treatment regimens may also require addressing mental health comorbidities, such as depression. Psychiatric symptoms are common comorbidities in MS and have been shown to interact with the disease course as well as influence patients’ adherence to the therapy (Tarrants et al., 2011; Moore et al., 2012; Marrie et al., 2015; McKay et al., 2018; Binzer et al., 2019). Despite its importance, mental health remains overlooked in this patient group (Marrie et al., 2009). Treating psychiatric symptoms is crucial for developing new treatments for MS, as improving mental health will contribute to the quality of life of MS patients. This mini-review of brain health in MS will aim to give a comprehensive overview of state-of-the-art magnetic resonance imaging (MRI) research that has advanced our understanding of personalized diagnosis and treatment strategies. To further shine a light on this complex neurological condition in relation to brain and mental health, this review will include current research on comorbidities related to MS, such as depression and anxiety (Figure 1).
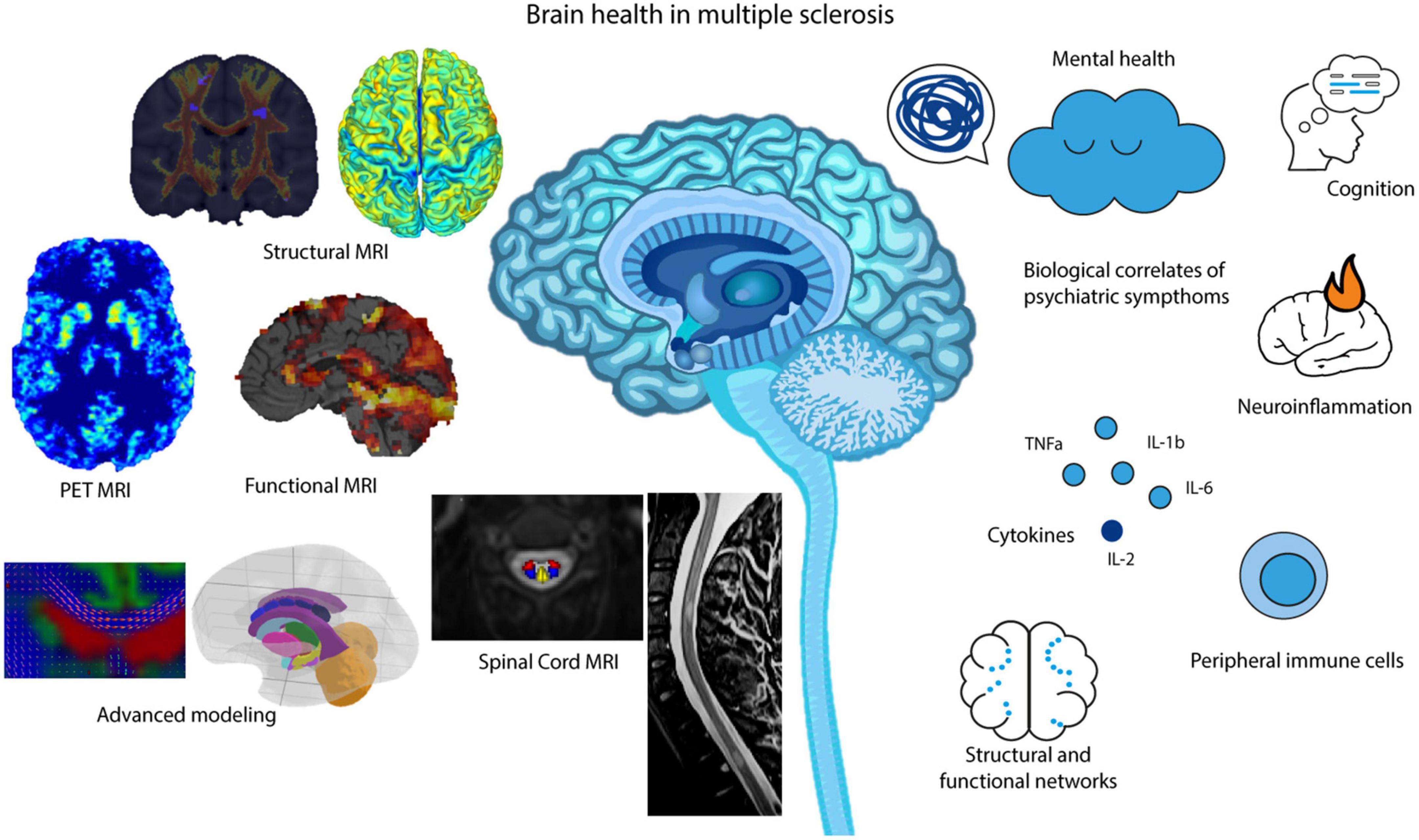
Figure 1. MR imaging, advanced analysis and modeling methods that aid in our understanding of brain health in MS, including cognition, neuroinflammation, structural and functional networks and overall mental health.
2. Magnetic resonance imaging characteristics
2.1. Brain and spinal cord lesions
Magnetic resonance imaging has been used to evaluate lesions in the brain of suspected MS patients for over 2 decades and focused on brain and spinal cord (SC) lesions (Barkhof et al., 1997). In the 2017 revised McDonald Diagnostic Criteria for MS (Thompson et al., 2018), MRI lesion identification in the entire central nervous system (CNS) is a cornerstone for correctly diagnosing patients with a dissemination in time and space. However, due to the complex nature of MS and its unpredictable disease course, lesions in the brain and SC only account for a minor amount of the symptoms observed in patients, termed the “clinico-radiological paradox” (Chard and Trip, 2017). Long-term motor disability is better reflected by location and length of SC lesions (Eden et al., 2019), but lesions can be asymptomatic and longitudinal monitoring with MRI is required (Brownlee et al., 2017; Ciccarelli et al., 2019). Thus, it would seem that lesions, which indicate demyelination in the WM and degeneration of axons in the GM, cannot be the sole imaging marker evaluated when assessing MS patient prognosis (Absinta et al., 2021). Recent advances in MRI technology and analysis methods, disease subtypes, brain and SC gray matter (GM) and white matter (WM) atrophy, WM/myelin analysis, functional MRI networks, along with lesion counts and volumes are able to be evaluated (Sastre-Garriga et al., 2020; Granziera et al., 2021; Romanello et al., 2022).
2.2. Brain and spinal cord atrophy
With increased computational research, more accurate parcellations of whole brain/SC GM and WM from MRI can be achieved in MS research (Gros et al., 2019; Guo et al., 2019; Chien et al., 2020). Atrophy in the brain and SC have been shown to relate to normal aging, but also associated with clinical disability in patients with MS when taken together with lesion load (Oh et al., 2014; Opfer et al., 2018). Since the term “atrophy” refers to a decrease in measures of particular regions, it can only be evaluated when comparing two groups or time points. Especially in the last decade, much has been elucidated about which regions of the brain are important to investigate for different MS-related disabilities. Therefore, many recent studies have focused on longitudinal analysis of MRI data to answer questions regarding changes in the brain and SC of MS patients during their disease course.
2.2.1. Brain regions of interest
Previously, MS researchers have focused on using brain atlases to parcellate regions of interest and have found that especially cortical GM and deep GM substructures are shown to be atrophied (Rocca et al., 2010; Narayana et al., 2012; Cooper et al., 2021) leading to more clinical disability accrual. Since whole brain atrophy is also found in normal aging and confounded by sex and head size (Malone et al., 2015; Opfer et al., 2018; Azevedo et al., 2019), it has been proposed to compare MS patients’ predicted “brain age” with that predicted in healthy age- and sex-matched individuals (Raffel et al., 2017). Brain age is commonly calculated by training a machine learning algorithm to create an age regression model using 3D structural MRI (raw or extracted data) as independent values with chronological age as dependent values. Then a multivariable model of healthy brain aging/maturation is constructed which can be applied to new MRI data for “brain age” prediction (Franke and Gaser, 2019). Several studies have found relevance in the brain age gap/difference between the predicted MS brain age and chronological age of the patients, where larger differences in predicted brain age are associated with WM lesion load and more rapid disability progression (Høgestøl et al., 2019; Cole et al., 2020). Recently, it was found that predicted brain age differences also contribute significantly to cognitive performance scores, with larger brain age differences associating with cognitive dysfunction in MS patients (Denissen et al., 2022).
Other studies found that including ventricular cerebrospinal fluid (CSF) volume as an MRI-extracted metric in advanced statistical models increased the prediction of confirmed disability progression (Zivadinov et al., 2016). Also, higher change in lateral ventricular volume over time is associated with disease activity (Millward et al., 2020; Barnett et al., 2021). Recently, the choroid plexus (CP) within the lateral ventricles showed enlarged volumes in MS patients (Gauthier, 2023) and were found to occur in conjunction with chronic lesions and brain atrophy (Klistorner et al., 2022). Enlargement of the CP have also been indicated as markers of inflammatory/acute disease activity (Fleischer et al., 2021; Ricigliano et al., 2021; Margoni et al., 2023); where increased gadolinium enhancement in T1-weighted MRIs (Kim et al., 2020) and T2-weighted intensity have predicted higher disease activity in MS patients (Chien et al., 2022).
2.2.2. Spinal cord regions of interest
It was thought that chronic lesions in the SC lead to atrophy at those specific levels in the cord, since large post-mortem findings of WM affection in the cervical cord was in-line with MS pathology (Gilmore et al., 2005). Although SC lesions and atrophy occur in the cervical cord at the same time (Valsasina et al., 2018), it has recently been shown that there is no such relationship and lesions and whole cord atrophy occur independently from each other in MS (Bussas et al., 2022). Thus, many MRI researchers have begun to evaluate more closely, the location of where atrophy in the cervical cord occurs and found that GM cross-sectional area and the posterior/lateral regions of the WM are most associated with disability as measured by the Expanded Disability Status Scale and different MS subtypes (Schlaeger et al., 2014; Bonacchi et al., 2020; Valsasina et al., 2022). This indicates that perhaps MRI can detect GM and WM regional affection in patients earlier in the disease course than expected, where decreased areas and volumes posteriorly and laterally lead to sensory deficits and motor disability (Mercadante and Tadi, 2022; Natali et al., 2022).
2.3. White matter affection
The WM contains the majority of myelin in the CNS and it is well researched that MS is primarily a demyelinating disease (Rosenthal et al., 2020). Axonal loss is evident in smaller fibers of the corticospinal tracts (from the cortex to the lumbar SC regions), and the sensory tracts from the cervical to lumbar SC levels, in post-mortem brain and SC samples (DeLuca et al., 2004). It has been found in every MS stage, many of the WM tracts in the brain are damaged and can be detected using diffusion tensor imaging (DTI) (Preziosa et al., 2011). Fractional anisotropy (FA) can be calculated from DTI-modeled MRI. DTI models the diffusion of water through space, where an FA value closer to 1 indicates restricted water movement (i.e., in axons that are myelinated) and an FA value closer to 0 indicates non-restricted water movement (i.e., demyelinated axons) (Basser and Pierpaoli, 1996). Reduced FA is widespread even in early relapsing-remitting MS and can be observed to decrease over time mostly in the corpus callosum, cerebral peduncle, corticospinal tract, and the posterior thalamic/optic radiation (Asaf et al., 2015). Since WM tracts are connected to GM regions in the brain, it has been of interest to look at how detected WM tract damage can affect the regional GM. One relatively large longitudinal MS study found that over a short period of time (mean follow-up time of 13.7 years), thalamic atrophy could be detected and interestingly, the mean FA in the thalamocortical tracts at baseline could predict the annual thalamic atrophy rate (Weeda et al., 2020). Recently, a longitudinal study in healthy participants observed age-related decreases in WM FA values that are associated with cognitive decline in the dimensions of memory, executive function and general cognition (Coelho et al., 2021). With MS being a demyelinating disease, it could be that the extra WM degradation occurs prior to GM atrophy, which then leads to increased “brain age” estimates and cognitive decline over time.
Diffusion-weighted imaging (DWI) can also be used to model WM tracts using probabilistic tractography, which calculates a connectivity index per voxel in the brain that can be used to evaluate the most likely path of a WM tract with the average number of streamlines that traverse it (Reid et al., 2022). WM lesions, especially those often found in MS, have been thought to impede tractography modeling in MS, thus researchers often use atlas-based versus individual-MRI-based methods (Kuchling et al., 2018). However, it is becoming more common, especially in MS studies, to identify and evaluate which WM tracts are affected by brain lesions using normative high-resolution atlases. These affected WM tracts can be seen as disconnections or as disconnectomes in the brain (Thiebaut de Schotten et al., 2020). These calculated disconnectomes based on individual brain lesion masks have recently been shown to associate well with serum neurofilament light chain levels, a known biomarker of axonal brain damage (Rise et al., 2022).
Another method for evaluating DWI WM tracts is using connectivity matrices calculated between regions of interest (or nodes) in the brain that can indicate less connected nodes using streamline weights and accounting for regional volumes (Kuceyeski et al., 2013). This method includes brain lesion masks that account for structural network modifications (an estimated structural connectivity). One study using this method showed that decreased connectivity in networks structurally related to visual, somatosensory, and attention functions were found in MS patients with higher Expanded Disability Status Scale (EDSS) score (Tozlu et al., 2021). In another study, the modularity, clustering, global and local efficiencies of the WM and GM structural networks were found to differ in a temporal fashion, based on disease duration, indicating that network reorganization occurs along with clinical disability in MS (Fleischer et al., 2017). Probabilistic tractography streamlines have also used to calculate structural connectivity matrices that were used as input features into ensemble machine learning algorithms to predict EDSS score by Barile et al. (2021) Feature importance in predicting disability based on EDSS was also evaluated that highlighted microstructural changes in WM tracts between different cortical regions were related to low, medium, and high clinical disability.
2.4. Functional MRI networks
Using resting-state functional MRI (rs-fMRI) and task-based fMRI, researchers have identified “Neural Networks” that are related to different brain functions. By measuring the blood oxygen level dependent (BOLD) signal in the brain using echo planar imaging (EPI) sequences (Kirilina et al., 2016), it is possible to extract information regarding the Amplitude of Low Frequency Fluctuations (ALFF) and regional homogeneity (ReHo) (Lv et al., 2018). These metrics can be used to reconstruct localized regional brain function, where the BOLD signal detected during tasks given to participants indicate specific neuronal activity (Christie et al., 2017). Using this type of functional-connectivity MRI has led to defining a default-mode network, where there is baseline activity detected in rs-fMRI from just wakefulness (Greicius et al., 2004). Recently, it has been found that altered functional brain states and connectivity dynamics, or analysis of the temporal, non-static rs-fMRI networks are related to cognitive decline and clinical disability in MS patients, even at an early stage of the disease (Broeders et al., 2022; Romanello et al., 2022). Task-based fMRI investigations in MS patients have often revealed increased activations of higher-order brain functional areas, such as in classical motor, frontal, and parietal regions, which is thought of as functional networks acting in a compensatory fashion to maintain normal good performance (Rocca et al., 2022). This was recently shown using visual attention paradigms, where higher visual and attention-related, as well as the default-mode network connectivity, was associated with better Brief Visuospatial Memory Test–Revised scores. However, reduced connectivity was found between visual cortical regions with eye-fields (Veréb et al., 2021). Another study hypothesized that task-based fMRI networks may show increased or decreased activation in a disease-related temporal fashion. Indeed, using a visually guided force-matching task, it was found that fMRI activation was lower in the cerebellar, occipital and superior parietal cortical regions, which also correlated with higher EDSS in minimally impaired early MS patients (Strik et al., 2021).
Interestingly, SC lesions have also been found to interrupt the functional connectivity in the cervical cord and may be the pathophysiological reason for disability based on the ventral motor and dorsal sensory networks in later MS (Conrad et al., 2018). These findings suggest there will become a larger role for rs- and task-based fMRI in evaluating brain and CNS health of MS patients in the future (Nejad-Davarani et al., 2016).
3. Mental health and MS
In MS patients, psychiatric symptoms are common comorbidities, with depression being the main one (Marrie et al., 2015; Gold et al., 2020). In addition to withholding pharmacological treatment and rehabilitation programs, depressive symptoms result in worsening functional outcomes (Binzer et al., 2019). Even though these symptoms are a major determinant of quality of life in MS, they are often overlooked and undertreated (Marrie et al., 2009). MS patients have a 30.5% prevalence for developing depression and 22.1% for anxiety, with clinically significant depressive or anxiety-related symptoms found in 35% and 34% of patients (Boeschoten et al., 2017). Prevalence for depression raises up to 44.5% during relapses and lifetime prevalence increases to 50% (Feinstein, 2011). Clinically isolated syndrome (CIS) and early MS patients from a meta-analysis of 51 studies were reported to exhibit depressive and anxiety-based symptoms in 17% and 35% of patients, respectively (Rintala et al., 2019).
There has been evidence of bidirectional interactions between the course of MS and comorbid psychiatric symptoms. Two large longitudinal cohort studies have suggested that the presence of psychiatric comorbidities increased the risk of MS disability progression (McKay et al., 2018; Binzer et al., 2019). In the retrospective cohort study from the Canadian provinces of British Columbia and Nova Scotia, McKay et al. (2018) followed 2,312 incident cases of adult-onset MS for around 10 years and found 38.5% of participants met the criteria for mood and anxiety disorders. They were associated with subsequent neurologic disability progression as measured by the EDSS score and the effect was statistically significant among women but not men. Interestingly, more than 40% of these MS patients met the criteria for comorbid psychiatric disorder prior to the MS onset. With 37%, the most prevalent psychiatric comorbidity was depression, while anxiety was present in 22.1% and bipolar disorder in 5.1% of MS patients. A further separate analysis of the individual effect of each psychiatric comorbidity showed that only depression was significantly associated with higher EDSS scores. The results of a Swedish cohort study by Binzer et al. (2019) showed that MS patients with depression, defined as being diagnosed with depression or requiring treatment with antidepressants, have faster disease progression than non-depressed MS patients. Moreover, they reported significant results for both men and women, indicating that the lack of significant results for men in the Canadian study could have been because of the small number of male participants. A nested case-control study with 10,204 incident MS cases investigated the occurrence of different symptoms in MS versus healthy participants from the first record of the disease and up to 10 years before it (Disanto et al., 2018). MS patients showed a significantly higher risk of being diagnosed with depression up to 10 and with anxiety up to 5 years before the disease onset. Disanto et al. (2018) found that psychiatric symptoms were reported years before the first record of the disease, which suggests mood disturbances are not a consequence of the pathology, but rather an integral part of it (Feinstein et al., 2014). On the other hand, clinical relapses and neurological disability in MS have been linked with higher rates of depressive symptoms (Moore et al., 2012). In a study by Moore et al. (2012) that looked at clinically significant depression symptoms during and post-MS relapse the point prevalence of depression during a confirmed MS relapse was 44.5%. It significantly decreased but stayed high in the follow-up, 29.2% at two and 34.4% at 6 months follow-up, suggesting that although the improvement in disability leads to improvement of depressive symptoms, they still stay persistent and high.
3.1. Biological correlates of psychiatric symptoms in MS
In order to mitigate disability progression in MS, treating psychiatric comorbidities should be investigated and optimized. Studies investigating biomarkers of psychiatric symptoms in MS may be relevant for understanding the biology that underlies the comorbidity of inflammatory and mood disorders with implications for prevention and treatment. Neuroinflammation seems to be one of the major correlates as studies show that hippocampal neuroinflammation, measured as microglial activation in the hippocampus (Colasanti et al., 2016) was related to depression in MS patients. Moreover, the data show how subclinical intrathecal inflammation, even without detrimental symptoms, can induce mood alterations (Rossi et al., 2017), which can further predict inflammatory reactivation in MS relapses. Relapsing MS patients showed greater values for state anxiety and depression [measured as State-Trait Anxiety Inventory (STAI)-state and Beck’s Depression Inventory II (BDI II)] in comparison to the remitting MS patients, but similar trait anxiety scores. Along with the reduction of neuroinflammation, Rossi et al. (2017) found that there was a reduction in state anxiety and depression scores suggesting that (subclinical) inflammation affects anxiety and depression in MS. Taken together, this suggests that inflammation may be a critical biological event involved in mood disorders and in MS. When it comes to studies investigating proinflammatory cytokines, known to be associated with depression severity, there is evidence for the correlation of cytokines in cerebrospinal fluid (CSF) and mood changes. The levels of tumor necrosis factor-alpha (TNF-a) interleukin-1 beta (IL-1b) (Rossi et al., 2017), and interleukin 6 (IL-6) (Brenner et al., 2018) were found to be associated with depression severity as measured by BDI II, while levels of Interleukin-2 (IL-2) were found to be correlated with anxiety measured by STAI-state. A study investigating cellular frequencies in the peripheral immune system identified CD4+ T central memory cells expressing low levels of CCR7+ as a robust biological correlate of MS-associated depression. These cell frequencies were correlated with depression severity, but not MS disease severity, and associated with neuroinflammation measured as lesion load on MRI (Brasanac et al., 2022). Thus, indicating inflammation and immune system changes as biological pathways implicated in the shared pathobiology of MS and depression (mood and immune disorders). Among other biological correlates of MS-associated depression, studies have pointed to hypothalamic-pituitary-adrenal axis hyperactivity (Gold et al., 2011), hippocampal atrophy (Gold et al., 2014), and larger cerebral T2 lesion load (Feinstein et al., 2004). Furthermore, a study testing the association of depression and lesions in amygdala-prefrontal fiber tracts found that depressed MS patients were less able to regulate negative emotions, indicating that emotional regulation in MS-associated depression was affected by lesion load (Meyer-Arndt et al., 2022). In addition, disruption in frontal–parietal white matter tract over the course of 5 years could predict a diagnosis of depression in multiple sclerosis (Ashton et al., 2021).
3.2. Cognition and age in MS-related depression
Studies have shown that severely depressed MS patients have difficulties with working memory (Arnett et al., 1999a,b), information processing speed (Lubrini et al., 2012), and executive functioning (Arnett et al., 2001; Feinstein et al., 2014). The biology underlying this comorbidity could be the atrophy of the hippocampus as one study has shown that enlarged temporal horns are linked to depression and consolidation deficits in memory tasks (Kiy et al., 2011). In a large cohort of MS patients with 13,821 individuals, depressive symptoms were found to be correlated with worse cognitive performance measured as information processing speed, manual dexterity and walking speed (Chan et al., 2021). This association was related to the age of participants, younger MS patients experiencing moderate to severe MS showed slower processing speed while older MS patients had slower walking speed. In the animal model of MS, the experimental autoimmune encephalomyelitis (EAE), data show that changes in cognition and behavior appear even before demyelination starts and correlates with cytokines TNFa and IL1b in the hypothalamus, as well as with corticosteroid hormone levels (Acharjee et al., 2013).
When it comes to the link between psychiatric symptoms and age in MS, results are ambiguous. Several studies are showing a trend toward a decreasing prevalence of depressive symptoms with increase in age of MS patients (Patten et al., 2003; Garcia and Finlayson, 2005). Furthermore, data show that younger age at onset is correlated with the occurrence of depression in MS patients (Beiske et al., 2008) and that the shorter the duration of MS (relapses) the higher the risk for depression (Williams et al., 2005). A possible explanation for the change in prevalence of depression could be the underlying biology of MS. While depression has been associated with inflammation in the early phases of the disease characterized by relapses, with age inflammatory processes are replaced with neurodegeneration characterized by motor and cognitive disability (Gold and Irwin, 2009; Musella et al., 2018). In contrast with these results are studies reporting a positive association between depression and age of MS patients (da Silva et al., 2011; Mattioli et al., 2011), while several other studies did not find any relationship between age and depression in MS. More studies are needed to further investigate and elucidate this aspect of MS.
4. Discussion and future directions
Increasingly more research is going into the neuroinflammatory and metabolic substrates involved in MS, using positron emission tomography (PET) imaging. PET imaging gives information about metabolism of in vivo organs, such as the brain, since it uses radiotracers that are actively taken up into tissues in active catabolism or can bind to receptors on cell surfaces (Politis and Piccini, 2012). PET tracer detection was initially conducted using computed tomography (CT) machines due to its function in detecting X-ray photons (radiation); however, hybrid PET/MRI machines are becoming more prominent in the research field due to the superior contrast that MRI can image between soft-tissues (Antoch and Bockisch, 2009). Since PET allows for identification of active metabolism, researchers have begun to evaluate its capability in visualizing task-based and resting-state networks (Rischka et al., 2018; Fang et al., 2021). Very recently, an amyloid radiotracer (11C-PiB) (Rabinovici et al., 2007), which also has a high capacity to bind to myelin, was used to longitudinally evaluate remyelination in MS patient brains (Tonietto et al., 2023). Tonietto et al. (2023) found that enlargement of the CP was associated with failure to remyelinate periventricular WM leading to possible regional cortical atrophy in relapsing-remitting MS patients.
With new technological advancements, machine learning (ML) and deep learning in CNS disorders have become more popular, especially using MRI data due to its complex extraction of metrics and 3D or 4D nature (4th dimension is related to the temporal aspect as in fMRI). There have been several studies in the last few years that have given rise to interesting information related to brain health and MS. Recently, it has been found that ML can be used to predict MS future disease activity in patients using unprocessed MRIs, where within the periventricular region and CP there seems to be higher T2-weighted intensities in people with higher future disease activity (Chien et al., 2022). Along the same line, unsupervised ML in combination with advanced statistical methods have been used to identify different MS-related MRI-extracted subtypes that lead to different confirmed disability progression and relapse rates (Eshaghi et al., 2021). Also, using a deep learning algorithm trained on unprocessed Alzheimer’s brain MRIs, one study found a way to transfer this learned algorithm (transfer learning) to identify MS brains versus healthy participant brains (Eitel et al., 2019). Thus, it can be seen that the assessment of brain health and patient prognosis in MS is moving toward the use of more advanced imaging related to networks and use of ML and deep learning technologies.
Even though the majority of MS patients have comorbid mental health disorders there are not so many studies exploring their long-term effects (McKay et al., 2018). Having a non-treated comorbid psychiatric disorder may hamper adherence to disease-modifying therapies (Tarrants et al., 2011) or promote unhealthy coping strategies (McKay et al., 2016) in MS patients. It is of paramount importance to treat comorbid mood disorders in MS as mental health represents one of the major determinants of the quality of life (Marrie et al., 2009) and underlying mood disorder is associated with suicidal ideation in this population at a rate higher than the general population (Feinstein and Pavisian, 2017). For developing new treatments for MS patients and for successful therapy, it is crucial to understand and treat psychiatric symptoms as well. Therefore, the way forward could be to combine therapies targeted for a specific disease phenotype, such as MS-associated depression.
Although depression has been identified as one of the main mental health disorders found in MS, high comorbidity with mental health disorders is not specific to MS or limited to inflammatory diseases. Besides MS, other chronic conditions such as cardiovascular, neurological, and metabolic disorders also share depression as one of the most frequent accompanying comorbidities (Gold et al., 2020). Contributing factors to this comorbidity range from shared genetics to converging underlying biology, as well as various psychological and lifestyle factors. Numerous studies in recent decades have compounded evidence that points to the contributions of the immune system and inflammation in developing mood disorders (Beurel et al., 2020). Recently, more emphasis on identifying subtypes of depression has been made in mental health research. For example, the subtype of inflammatory depression has been identified (Lynall et al., 2020), which also involves depressed patients with underlying inflammatory disorders such as MS. Further investigation of these disease subtypes can help identify patient populations that can benefit from targeted therapeutic strategies.
In the last decades, imaging studies in the field of depression research have significantly grown in number (Zhuo et al., 2019). However, real progress has been hampered by underpowered studies and a lack of reproducibility. To address these issues ENIMGA MDD consortium was formed a decade ago and they have been able to identify subtle structural brain changes related to distinct clinical and demographic characteristics of depression (Schmaal et al., 2020). Despite new technological advancements in MRI and mental health research in MS, there are still limitations particularly in answering questions about psychiatric disorders. In almost all MRI studies, there are confounding variables that are required to be considered, such as age (Opfer et al., 2018), sex (Voskuhl et al., 2020; Chyzhyk et al., 2022), and often brain lesion load (Sinnecker et al., 2012). However, with more and more confounders, the importance of variables of interest in predicting outcomes may be lost or become biased, especially in machine learning applications (Snoek et al., 2019). Larger and standardized benchmark MRI datasets may help reduce bias and increase prediction accuracies (Eitel et al., 2021). There is also speculation that our general lack of understanding of the mechanisms of psychiatric illnesses is a potential reason as to why we have no concrete neurobiological insights from over 30 years of functional neuroimaging (Nour et al., 2022). Thus, it will be important to move toward a more holistic understanding of neurobiology and mental health by conscientiously designing prediction models that inherently reduce bias and allow for comprehensive real-world data usage (Hooker, 2021).
Author contributions
JB and CC contributed to the writing of the original draft, read, and approved the submitted version.
Funding
We acknowledged financial support from the Open Access Publication Fund of Charité – Universitätsmedizin Berlin and the German Research Foundation (DFG).
Conflict of interest
CC has received honoraria for speaking from Bayer and research funding from Novartis and Alexion, unrelated to this study. CC is a member of the Standing Committee on Science for the Canadian Institutes of Health Research (CIHR).
The remaining author declares that the research was conducted in the absence of any commercial or financial relationships that could be construed as a potential conflict of interest.
Publisher’s note
All claims expressed in this article are solely those of the authors and do not necessarily represent those of their affiliated organizations, or those of the publisher, the editors and the reviewers. Any product that may be evaluated in this article, or claim that may be made by its manufacturer, is not guaranteed or endorsed by the publisher.
References
Absinta, M., Maric, D., Gharagozloo, M., Garton, T., Smith, M. D., Jin, J., et al. (2021). A lymphocyte-microglia-astrocyte axis in chronic active multiple sclerosis. Nature 597, 709–714. doi: 10.1038/s41586-021-03892-7
Acharjee, S., Nayani, N., Tsutsui, M., Hill, M. N., Ousman, S. S., and Pittman, Q. J. (2013). Altered cognitive-emotional behavior in early experimental autoimmune encephalitis–Cytokine and hormonal correlates. Brain Behav. Immunity 33, 164–172. doi: 10.1016/j.bbi.2013.07.003
Antoch, G., and Bockisch, A. (2009). Combined PET/MRI: A new dimension in whole-body oncology imaging? Eur. J. Nucl. Med. Mol. Imaging 36, 113–120. doi: 10.1007/s00259-008-0951-6
Arnett, P. A., Higginson, C. I., and Randolph, J. J. (2001). Depression in multiple sclerosis: Relationship to planning ability. J. Int. Neuropsychol. Soc. 7, 665–674. doi: 10.1017/s1355617701766027
Arnett, P. A., Higginson, C. I., Voss, W. D., Bender, W. I., Wurst, J. M., and Tippin, J. M. (1999a). Depression in multiple sclerosis: Relationship to working memory capacity. Neuropsychology 13, 546–556. doi: 10.1037//0894-4105.13.4.546
Arnett, P. A., Higginson, C. I., Voss, W. D., Wright, B., Bender, W. I., Wurst, J. M., et al. (1999b). Depressed mood in multiple sclerosis: Relationship to capacity-demanding memory and attentional functioning. Neuropsychology 13, 434–446. doi: 10.1037//0894-4105.13.3.434
Asaf, A., Evan, S., and Anat, A. (2015). Injury to white matter tracts in relapsing–remitting multiple sclerosis: A possible therapeutic window within the first 5 years from onset using diffusion-tensor imaging tract-based spatial statistics. Neuroimage 8, 261–266. doi: 10.1016/j.nicl.2015.04.020
Ashton, K., Fuchs, T. A., Oship, D., Zivadinov, R., Jakimovski, D., Bergsland, N., et al. (2021). Diagnosis of depression in multiple sclerosis is predicted by frontal-parietal white matter tract disruption. J. Neurol. 268, 169–177. doi: 10.1007/s00415-020-10110-3
Azevedo, C. J., Cen, S. Y., Jaberzadeh, A., Zheng, L., Hauser, S. L., and Pelletier, D. (2019). Contribution of normal aging to brain atrophy in MS. Neurol. Neuroimmunol. Neuroinflamm. 6:e616. doi: 10.1212/NXI.0000000000000616
Barile, B., Marzullo, A., Stamile, C., Durand-Dubief, F., and Sappey-Marinier, D. (2021). Ensemble learning for multiple sclerosis disability estimation using brain structural connectivity. Brain Connect. 12, 476–488. doi: 10.1089/brain.2020.1003
Barkhof, F., Filippi, M., Miller, D. H., Scheltens, P., Campi, A., Polman, C. H., et al. (1997). Comparison of MRI criteria at first presentation to predict conversion to clinically definite multiple sclerosis. Brain 120(Pt 11), 2059–2069. doi: 10.1093/brain/120.11.2059
Barnett, M., Bergsland, N., Weinstock-Guttman, B., Butzkueven, H., Kalincik, T., Desmond, P., et al. (2021). Brain atrophy and lesion burden are associated with disability progression in a multiple sclerosis real-world dataset using only T2-FLAIR: The NeuroSTREAM MSBase study. Neuroimage 32:102802. doi: 10.1016/j.nicl.2021.102802
Basser, P. J., and Pierpaoli, C. (1996). Microstructural and physiological features of tissues elucidated by quantitative-diffusion-tensor MRI. J. Magn. Reson. B 111, 209–219. doi: 10.1006/jmrb.1996.0086
Beiske, A. G., Svensson, E., Sandanger, I., Czujko, B., Pedersen, E. D., Aarseth, J. H., et al. (2008). Depression and anxiety amongst multiple sclerosis patients. Eur. J. Neurol. 15, 239–245. doi: 10.1111/j.1468-1331.2007.02041.x
Beurel, E., Toups, M., and Nemeroff, C. B. (2020). The bidirectional relationship of depression and inflammation: Double trouble. Neuron 107, 234–256. doi: 10.1016/j.neuron.2020.06.002
Binzer, S., McKay, K. A., Brenner, P., Hillert, J., and Manouchehrinia, A. (2019). Disability worsening among persons with multiple sclerosis and depression: A Swedish cohort study. Neurology 93, e2216–e2223. doi: 10.1212/WNL.0000000000008617
Boeschoten, R. E., Braamse, A. M. J., Beekman, A. T. F., Cuijpers, P., van Oppen, P., Dekker, J., et al. (2017). Prevalence of depression and anxiety in multiple sclerosis: A systematic review and meta-analysis. J. Neurol. Sci. 372, 331–341. doi: 10.1016/j.jns.2016.11.067
Bonacchi, R., Pagani, E., Meani, A., Cacciaguerra, L., Preziosa, P., De Meo, E., et al. (2020). Clinical relevance of multiparametric MRI assessment of cervical cord damage in multiple sclerosis. Radiology 296, 605–615. doi: 10.1148/radiol.2020200430
Brasanac, J., Ramien, C., Gamradt, S., Taenzer, A., Glau, L., Ritter, K., et al. (2022). Immune signature of multiple sclerosis-associated depression. Brain Behav. Immunity 100, 174–182. doi: 10.1016/j.bbi.2021.11.022
Brenner, P., Granqvist, M., Königsson, J., Al Nimer, F., Piehl, F., and Jokinen, J. (2018). Depression and fatigue in multiple sclerosis: Relation to exposure to violence and cerebrospinal fluid immunomarkers. Psychoneuroendocrinology 89, 53–58. doi: 10.1016/j.psyneuen.2018.01.002
Broeders, T. A. A., Douw, L., Eijlers, A. J. C., Dekker, I., Uitdehaag, B. M. J., Barkhof, F., et al. (2022). A more unstable resting-state functional network in cognitively declining multiple sclerosis. Brain Commun. 4:fcac095. doi: 10.1093/braincomms/fcac095
Brownlee, W. J., Altmann, D. R., Alves Da Mota, P., Swanton, J. K., Miszkiel, K. A., Wheeler-Kingshott, C. G., et al. (2017). Association of asymptomatic spinal cord lesions and atrophy with disability 5 years after a clinically isolated syndrome. Mult. Scler. 23, 665–674. doi: 10.1177/1352458516663034
Bussas, M., El Husseini, M., Harabacz, L., Pineker, V., Grahl, S., Pongratz, V., et al. (2022). Multiple sclerosis lesions and atrophy in the spinal cord: Distribution across vertebral levels and correlation with disability. Neuroimage 34:103006. doi: 10.1016/j.nicl.2022.103006
Chan, C. K., Tian, F., Pimentel Maldonado, D., Mowry, E. M., and Fitzgerald, K. C. (2021). Depression in multiple sclerosis across the adult lifespan. Mult. Scler. 27, 1771–1780. doi: 10.1177/1352458520979304
Chard, D., and Trip, S. A. (2017). Resolving the clinico-radiological paradox in multiple sclerosis. F1000Res 6:1828. doi: 10.12688/f1000research.11932.1
Chien, C., Juenger, V., Scheel, M., Brandt, A. U., and Paul, F. (2020). Considerations for mean upper cervical cord area implementation in a longitudinal MRI setting: Methods, interrater reliability, and MRI quality control. AJNR Am. J. Neuroradiol. 41, 343–350. doi: 10.3174/ajnr.A6394
Chien, C., Seiler, M., Eitel, F., Schmitz-Hübsch, T., Paul, F., and Ritter, K. (2022). Prediction of high and low disease activity in early MS patients using multiple kernel learning identifies importance of lateral ventricle intensity. Mult. Scler. J. 8:20552173221109770. doi: 10.1177/20552173221109770
Christie, I. N., Wells, J. A., Kasparov, S., Gourine, A. V., and Lythgoe, M. F. (2017). Volumetric spatial correlations of neurovascular coupling studied using single pulse opto-fMRI. Sci. Rep. 7:41583. doi: 10.1038/srep41583
Chyzhyk, D., Varoquaux, G., Milham, M., and Thirion, B. (2022). How to remove or control confounds in predictive models, with applications to brain biomarkers. Gigascience 11:giac014. doi: 10.1093/gigascience/giac014
Ciccarelli, O., Cohen, J. A., Reingold, S. C., Weinshenker, B. G., and International Conference on Spinal Cord Involvement and Imaging in Multiple Sclerosis and Neuromyelitis Optica Spectrum Disorders (2019). Spinal cord involvement in multiple sclerosis and neuromyelitis optica spectrum disorders. Lancet Neurol. 18, 185–197. doi: 10.1016/S1474-4422(18)30460-5
Coelho, A., Fernandes, H. M., Magalhães, R., Moreira, P. S., Marques, P., Soares, J. M., et al. (2021). Signatures of white-matter microstructure degradation during aging and its association with cognitive status. Sci. Rep. 11:4517. doi: 10.1038/s41598-021-83983-7
Colasanti, A., Guo, Q., Giannetti, P., Wall, M. B., Newbould, R. D., Bishop, C., et al. (2016). Hippocampal neuroinflammation, functional connectivity, and depressive symptoms in multiple sclerosis. Biol. Psychiatry 80, 62–72. doi: 10.1016/j.biopsych.2015.11.022
Cole, J. H., Raffel, J., Friede, T., Eshaghi, A., Brownlee, W. J., Chard, D., et al. (2020). Longitudinal assessment of multiple sclerosis with the brain-age paradigm. Ann. Neurol. 88, 93–105. doi: 10.1002/ana.25746
Conrad, B. N., Barry, R. L., Rogers, B. P., Maki, S., Mishra, A., Thukral, S., et al. (2018). Multiple sclerosis lesions affect intrinsic functional connectivity of the spinal cord. Brain 141, 1650–1664. doi: 10.1093/brain/awy083
Cooper, G., Chien, C., Zimmermann, H., Bellmann-Strobl, J., Ruprecht, K., Kuchling, J., et al. (2021). Longitudinal analysis of T1w/T2w ratio in patients with multiple sclerosis from first clinical presentation. Mult. Scler. 27, 2180–2190. doi: 10.1177/13524585211003479
da Silva, A. M., Vilhena, E., Lopes, A., Santos, E., Gonçalves, M. A., Pinto, C., et al. (2011). Depression and anxiety in a Portuguese MS population: Associations with physical disability and severity of disease. J. Neurol. Sci. 306, 66–70. doi: 10.1016/j.jns.2011.03.042
Dalmau, J. (2018). Diagnostic and treatment challenges, a new section in N2. Neurol. Neuroimmunol. Neuroinflamm. 5:e511. doi: 10.1212/NXI.0000000000000511
DeLuca, G. C., Ebers, G. C., and Esiri, M. M. (2004). Axonal loss in multiple sclerosis: A pathological survey of the corticospinal and sensory tracts. Brain 127, 1009–1018. doi: 10.1093/brain/awh118
Denissen, S., Engemann, D. A., De Cock, A., Costers, L., Baijot, J., Laton, J., et al. (2022). Brain age as a surrogate marker for cognitive performance in multiple sclerosis. Eur. J. Neurol. 29, 3039–3049. doi: 10.1111/ene.15473
Disanto, G., Zecca, C., MacLachlan, S., Sacco, R., Handunnetthi, L., Meier, U. C., et al. (2018). Prodromal symptoms of multiple sclerosis in primary care. Ann. Neurol. 83, 1162–1173. doi: 10.1002/ana.25247
Eden, D., Gros, C., Badji, A., Dupont, S. M., De Leener, B., Maranzano, J., et al. (2019). Spatial distribution of multiple sclerosis lesions in the cervical spinal cord. Brain 142, 633–646. doi: 10.1093/brain/awy352
Eitel, F., Schulz, M.-A., Seiler, M., Walter, H., and Ritter, K. (2021). Promises and pitfalls of deep neural networks in neuroimaging-based psychiatric research. Exp. Neurol. 339:113608. doi: 10.1016/j.expneurol.2021.113608
Eitel, F., Soehler, E., Bellmann-Strobl, J., Brandt, A. U., Ruprecht, K., Giess, R. M., et al. (2019). Uncovering convolutional neural network decisions for diagnosing multiple sclerosis on conventional MRI using layer-wise relevance propagation. Neuroimage Clin. 24:102003. doi: 10.1016/j.nicl.2019.102003
Eshaghi, A., Young, A. L., Wijeratne, P. A., Prados, F., Arnold, D. L., Narayanan, S., et al. (2021). Identifying multiple sclerosis subtypes using unsupervised machine learning and MRI data. Nat. Commun. 12:2078. doi: 10.1038/s41467-021-22265-2
Fang, X. T., Toyonaga, T., Hillmer, A. T., Matuskey, D., Holmes, S. E., Radhakrishnan, R., et al. (2021). Identifying brain networks in synaptic density PET (11C-UCB-J) with independent component analysis. Neuroimage 237:118167. doi: 10.1016/j.neuroimage.2021.118167
Feinstein, A. (2011). Multiple sclerosis and depression. Mult. Scler. 17, 1276–1281. doi: 10.1177/1352458511417835
Feinstein, A., and Pavisian, B. (2017). Multiple sclerosis and suicide. Mult. Scler. 23, 923–927. doi: 10.1177/1352458517702553
Feinstein, A., Magalhaes, S., Richard, J.-F., Audet, B., and Moore, C. (2014). The link between multiple sclerosis and depression. Nat. Rev. Neurol. 10, 507–517. doi: 10.1038/nrneurol.2014.139
Feinstein, A., Roy, P., Lobaugh, N., Feinstein, K., O’Connor, P., and Black, S. (2004). Structural brain abnormalities in multiple sclerosis patients with major depression. Neurology 62, 586–590. doi: 10.1212/01.WNL.0000110316.12086.0C
Fleischer, V., Gonzalez-Escamilla, G., Ciolac, D., Albrecht, P., Küry, P., Gruchot, J., et al. (2021). Translational value of choroid plexus imaging for tracking neuroinflammation in mice and humans. Proc. Natl. Acad. Sci. U.S.A. 118:e2025000118. doi: 10.1073/pnas.2025000118
Fleischer, V., Gröger, A., Koirala, N., Droby, A., Muthuraman, M., Kolber, P., et al. (2017). Increased structural white and grey matter network connectivity compensates for functional decline in early multiple sclerosis. Mult. Scler. 23, 432–441. doi: 10.1177/1352458516651503
Franke, K., and Gaser, C. (2019). Ten years of BrainAGE as a neuroimaging biomarker of brain aging: What insights have we gained? Front. Neurol. 10:789. doi: 10.3389/fneur.2019.00789
Gajofatto, A., and Benedetti, M. D. (2015). Treatment strategies for multiple sclerosis: When to start, when to change, when to stop? World J. Clin. Cases 3, 545–555. doi: 10.12998/wjcc.v3.i7.545
Garcia, J., and Finlayson, M. (2005). Mental health and mental health service use among people aged 45+ with multiple sclerosis. Can. J. Commun. Ment. Health 24, 9–22. doi: 10.7870/cjcmh-2005-0011
Gauthier, S. A. (2023). Location, location, location: Myelin repair and proximity to ventricular CSF in multiple sclerosis. Brain 146, 10–12. doi: 10.1093/brain/awac448
Gilmore, C. P., DeLuca, G. C., Bö, L., Owens, T., Lowe, J., Esiri, M. M., et al. (2005). Spinal cord atrophy in multiple sclerosis caused by white matter volume loss. Arch. Neurol. 62, 1859–1862. doi: 10.1001/archneur.62.12.1859
Gold, S. M., and Irwin, M. R. (2009). Depression and immunity: Inflammation and depressive symptoms in multiple sclerosis. Immunol. Allergy Clin. North Am. 29, 309–320. doi: 10.1016/j.iac.2009.02.008
Gold, S. M., Köhler-Forsberg, O., Moss-Morris, R., Mehnert, A., Miranda, J. J., Bullinger, M., et al. (2020). Comorbid depression in medical diseases. Nat. Rev. Dis. Primers 6, 1–22. doi: 10.1038/s41572-020-0200-2
Gold, S. M., Krüger, S., Ziegler, K. J., Krieger, T., Schulz, K.-H., Otte, C., et al. (2011). Endocrine and immune substrates of depressive symptoms and fatigue in multiple sclerosis patients with comorbid major depression. J. Neurol. Neurosurg. Psychiatry 82, 814–818. doi: 10.1136/jnnp.2010.230029
Gold, S. M., O’Connor, M.-F., Gill, R., Kern, K. C., Shi, Y., Henry, R. G., et al. (2014). Detection of altered hippocampal morphology in multiple sclerosis-associated depression using automated surface mesh modeling. Hum. Brain Mapp. 35, 30–37. doi: 10.1002/hbm.22154
Granziera, C., Wuerfel, J., Barkhof, F., Calabrese, M., De Stefano, N., Enzinger, C., et al. (2021). Quantitative magnetic resonance imaging towards clinical application in multiple sclerosis. Brain 144, 1296–1311. doi: 10.1093/brain/awab029
Greicius, M. D., Srivastava, G., Reiss, A. L., and Menon, V. (2004). Default-mode network activity distinguishes Alzheimer’s disease from healthy aging: Evidence from functional MRI. Proc. Natl. Acad. Sci. U.S.A. 101, 4637–4642. doi: 10.1073/pnas.0308627101
Gros, C., De Leener, B., Badji, A., Maranzano, J., Eden, D., Dupont, S. M., et al. (2019). Automatic segmentation of the spinal cord and intramedullary multiple sclerosis lesions with convolutional neural networks. Neuroimage 184, 901–915. doi: 10.1016/j.neuroimage.2018.09.081
Guo, C., Ferreira, D., Fink, K., Westman, E., and Granberg, T. (2019). Repeatability and reproducibility of FreeSurfer, FSL-SIENAX and SPM brain volumetric measurements and the effect of lesion filling in multiple sclerosis. Eur. Radiol. 29, 1355–1364. doi: 10.1007/s00330-018-5710-x
Høgestøl, E. A., Kaufmann, T., Nygaard, G. O., Beyer, M. K., Sowa, P., Nordvik, J. E., et al. (2019). Cross-sectional and longitudinal MRI brain scans reveal accelerated brain aging in multiple sclerosis. Front. Neurol. 10:450. doi: 10.3389/fneur.2019.00450
Hooker, S. (2021). Moving beyond “algorithmic bias is a data problem.” Patterns 2:100241. doi: 10.1016/j.patter.2021.100241
Kim, H., Lim, Y.-M., Kim, G., Lee, E.-J., Lee, J. H., Kim, H. W., et al. (2020). Choroid plexus changes on magnetic resonance imaging in multiple sclerosis and neuromyelitis optica spectrum disorder. J. Neurol. Sci. 415:116904. doi: 10.1016/j.jns.2020.116904
Kirilina, E., Lutti, A., Poser, B. A., Blankenburg, F., and Weiskopf, N. (2016). The quest for the best: The impact of different EPI sequences on the sensitivity of random effect fMRI group analyses. Neuroimage 126, 49–59. doi: 10.1016/j.neuroimage.2015.10.071
Kiy, G., Lehmann, P., Hahn, H. K., Eling, P., Kastrup, A., and Hildebrandt, H. (2011). Decreased hippocampal volume, indirectly measured, is associated with depressive symptoms and consolidation deficits in multiple sclerosis. Mult. Scler. 17, 1088–1097. doi: 10.1177/1352458511403530
Klistorner, S., Barnett, M. H., Parratt, J., Yiannikas, C., Graham, S. L., and Klistorner, A. (2022). Choroid plexus volume in multiple sclerosis predicts expansion of chronic lesions and brain atrophy. Ann. Clin. Transl. Neurol. 9, 1528–1537. doi: 10.1002/acn3.51644
Kuceyeski, A., Maruta, J., Relkin, N., and Raj, A. (2013). The network modification (NeMo) tool: Elucidating the effect of white matter integrity changes on cortical and subcortical structural connectivity. Brain Connect. 3, 451–463. doi: 10.1089/brain.2013.0147
Kuchling, J., Backner, Y., Oertel, F. C., Raz, N., Bellmann-Strobl, J., Ruprecht, K., et al. (2018). Comparison of probabilistic tractography and tract-based spatial statistics for assessing optic radiation damage in patients with autoimmune inflammatory disorders of the central nervous system. Neuroimage Clin. 19, 538–550. doi: 10.1016/j.nicl.2018.05.004
Lubrini, G., Perianez, J. A., Rios-Lago, M., and Frank, A. (2012). [Processing speed in relapsing-remitting multiple sclerosis: The role played by the depressive symptoms]. Rev. Neurol. 55, 585–592.
Lv, H., Wang, Z., Tong, E., Williams, L. M., Zaharchuk, G., Zeineh, M., et al. (2018). Resting-state functional MRI: Everything that nonexperts have always wanted to know. AJNR Am. J. Neuroradiol. 39, 1390–1399. doi: 10.3174/ajnr.A5527
Lynall, M.-E., Turner, L., Bhatti, J., Cavanagh, J., de Boer, P., Mondelli, V., et al. (2020). Peripheral blood cell–stratified subgroups of inflamed depression. Biol. Psychiatry 88, 185–196. doi: 10.1016/j.biopsych.2019.11.017
Malone, I. B., Leung, K. K., Clegg, S., Barnes, J., Whitwell, J. L., Ashburner, J., et al. (2015). Accurate automatic estimation of total intracranial volume: A nuisance variable with less nuisance. Neuroimage 104, 366–372. doi: 10.1016/j.neuroimage.2014.09.034
Margoni, M., Gueye, M., Meani, A., Pagani, E., Moiola, L., Preziosa, P., et al. (2023). Choroid plexus enlargement in paediatric multiple sclerosis: Clinical relevance and effect of sex. J. Neurol. Neurosurg. Psychiatry 94:181–188. doi: 10.1136/jnnp-2022-330343
Marrie, R. A., Fisk, J. D., Tremlett, H., Wolfson, C., Warren, S., Tennakoon, A., et al. (2015). Differences in the burden of psychiatric comorbidity in MS vs. the general population. Neurology 85, 1972–1979. doi: 10.1212/WNL.0000000000002174
Marrie, R., Horwitz, R., and Vollmer, T. (2009). The burden of mental comorbidity in multiple sclerosis: Frequent, underdiagnosed, and undertreated. Available online at: https://journals.sagepub.com/doi/10.1177/1352458508099477?url_ver=Z39.88-2003&rfr_id=ori:rid:crossref.org&rfr_dat=cr_pub%20%200pubmed (accessed Dec 16, 2022).
Mattioli, F., Bellomi, F., Stampatori, C., Parrinello, G., and Capra, R. (2011). Depression, disability and cognitive impairment in multiple sclerosis: A cross sectional Italian study. Neurol. Sci. 32, 825–832. doi: 10.1007/s10072-011-0624-2
McKay, K. A., Tremlett, H., Fisk, J. D., Patten, S. B., Fiest, K., Berrigan, L., et al. (2016). Adverse health behaviours are associated with depression and anxiety in multiple sclerosis: A prospective multisite study. Mult. Scler. 22, 685–693. doi: 10.1177/1352458515599073
McKay, K. A., Tremlett, H., Fisk, J. D., Zhang, T., Patten, S. B., Kastrukoff, L., et al. (2018). Psychiatric comorbidity is associated with disability progression in multiple sclerosis. Neurology 90, e1316–e1323. doi: 10.1212/WNL.0000000000005302
Mercadante, A. A., and Tadi, P. (2022). “Neuroanatomy, gray matter,” in StatPearls. Treasure Island, FL: StatPearls Publishing.
Meyer-Arndt, L., Kuchling, J., Brasanac, J., Hermann, A., Asseyer, S., Bellmann-Strobl, J., et al. (2022). Prefrontal-amygdala emotion regulation and depression in multiple sclerosis. Brain Commun. 4:fcac152. doi: 10.1093/braincomms/fcac152
Millward, J. M., Delgado, P. R., Smorodchenko, A., Boehmert, L., Periquito, J., Reimann, H. M., et al. (2020). Transient enlargement of brain ventricles during relapsing-remitting multiple sclerosis and experimental autoimmune encephalomyelitis. JCI Insight 5:e140040. doi: 10.1172/jci.insight.140040
Moore, P., Hirst, C., Harding, K. E., Clarkson, H., Pickersgill, T. P., and Robertson, N. P. (2012). Multiple sclerosis relapses and depression. J. Psychosom. Res. 73, 272–276. doi: 10.1016/j.jpsychores.2012.08.004
Musella, A., Gentile, A., Rizzo, F. R., De Vito, F., Fresegna, D., Bullitta, S., et al. (2018). Interplay between age and neuroinflammation in multiple sclerosis: Effects on motor and cognitive functions. Front. Aging Neurosci. 10:238. doi: 10.3389/fnagi.2018.00238
Narayana, P. A., Govindarajan, K. A., Goel, P., Datta, S., Lincoln, J. A., Cofield, S. S., et al. (2012). Regional cortical thickness in relapsing remitting multiple sclerosis: A multi-center study. Neuroimage Clin. 2, 120–131. doi: 10.1016/j.nicl.2012.11.009
Natali, A. L., Reddy, V., and Bordoni, B. (2022). Neuroanatomy, corticospinal cord tract,” in StatPearls. Treasure Island, FL: StatPearls Publishing.
Nejad-Davarani, S. P., Chopp, M., Peltier, S., Li, L., Davoodi-Bojd, E., Lu, M., et al. (2016). Resting state fMRI connectivity analysis as a tool for detection of abnormalities in five different cognitive networks of the brain in multiple sclerosis patients. Clin. Case Rep. Rev. 2, 464–471. doi: 10.15761/CCRR.1000S1001
Nour, M. M., Liu, Y., and Dolan, R. J. (2022). Functional neuroimaging in psychiatry and the case for failing better. Neuron 110, 2524–2544. doi: 10.1016/j.neuron.2022.07.005
Oh, J., Seigo, M., Saidha, S., Sotirchos, E., Zackowski, K., Chen, M., et al. (2014). Spinal cord normalization in multiple sclerosis. J. Neuroimaging 24, 577–584. doi: 10.1111/jon.12097
Opfer, R., Ostwaldt, A.-C., Sormani, M. P., Gocke, C., Walker-Egger, C., Manogaran, P., et al. (2018). Estimates of age-dependent cutoffs for pathological brain volume loss using SIENA/FSL-a longitudinal brain volumetry study in healthy adults. Neurobiol. Aging 65, 1–6. doi: 10.1016/j.neurobiolaging.2017.12.024
Patten, S. B., Beck, C. A., Williams, J. V. A., Barbui, C., and Metz, L. M. (2003). Major depression in multiple sclerosis: A population-based perspective. Neurology 61, 1524–1527. doi: 10.1212/01.wnl.0000095964.34294.b4
Politis, M., and Piccini, P. (2012). Positron emission tomography imaging in neurological disorders. J. Neurol. 259, 1769–1780. doi: 10.1007/s00415-012-6428-3
Preziosa, P., Rocca, M. A., Mesaros, S., Pagani, E., Stosic-Opincal, T., Kacar, K., et al. (2011). Intrinsic damage to the major white matter tracts in patients with different clinical phenotypes of multiple sclerosis: A voxelwise diffusion-tensor MR study. Radiology 260, 541–550. doi: 10.1148/radiol.11110315
Prosperini, L., Annovazzi, P., Boffa, L., Buscarinu, M. C., Gallo, A., Matta, M., et al. (2018). No evidence of disease activity (NEDA-3) and disability improvement after alemtuzumab treatment for multiple sclerosis: A 36-month real-world study. J. Neurol. 265, 2851–2860. doi: 10.1007/s00415-018-9070-x
Rabinovici, G. D., Furst, A. J., O’Neil, J. P., Racine, C. A., Mormino, E. C., Baker, S. L., et al. (2007). 11C-PIB PET imaging in Alzheimer disease and frontotemporal lobar degeneration. Neurology 68, 1205–1212. doi: 10.1212/01.wnl.0000259035.98480.ed
Raffel, J., Cole, J., Record, C., Sridharan, S., Sharp, D., and Nicholas, R. (2017). Brain age: A novel approach to quantify the impact of multiple sclerosis on the brain (P1.371). Neurology 88, P1.371.
Reid, A. T., Camilleri, J. A., Hoffstaedter, F., and Eickhoff, S. B. (2022). Tract-specific statistics based on diffusion-weighted probabilistic tractography. Commun. Biol. 5, 1–12. doi: 10.1038/s42003-022-03073-w
Ricigliano, V. A. G., Morena, E., Colombi, A., Tonietto, M., Hamzaoui, M., Poirion, E., et al. (2021). Choroid plexus enlargement in inflammatory multiple sclerosis: 3.0-T MRI and translocator protein PET evaluation. Radiology 301, 166–177. doi: 10.1148/radiol.2021204426
Rintala, A., Matcham, F., Radaelli, M., Locafaro, G., Simblett, S., Barattieri di San, et al. (2019). Emotional outcomes in clinically isolated syndrome and early phase multiple sclerosis: A systematic review and meta-analysis. J. Psychosom. Res. 124:109761. doi: 10.1016/j.jpsychores.2019.109761
Rischka, L., Gryglewski, G., Pfaff, S., Vanicek, T., Hienert, M., Klöbl, M., et al. (2018). Reduced task durations in functional PET imaging with [18F]FDG approaching that of functional MRI. Neuroimage 181, 323–330. doi: 10.1016/j.neuroimage.2018.06.079
Rise, H. H., Brune, S., Chien, C., Berge, T., Bos, S. D., Andorrà, M., et al. (2022). Brain disconnectome mapping derived from white matter lesions and serum neurofilament light levels in multiple sclerosis: A longitudinal multicenter study. Neuroimage 35:103099. doi: 10.1016/j.nicl.2022.103099
Rocca, M. A., Mesaros, S., Pagani, E., Sormani, M. P., Comi, G., and Filippi, M. (2010). Thalamic damage and long-term progression of disability in multiple sclerosis. Radiology 257, 463–469. doi: 10.1148/radiol.10100326
Rocca, M. A., Schoonheim, M. M., Valsasina, P., Geurts, J. J. G., and Filippi, M. (2022). Task- and resting-state fMRI studies in multiple sclerosis: From regions to systems and time-varying analysis. Current status and future perspective. Neuroimage Clin. 35:103076. doi: 10.1016/j.nicl.2022.103076
Romanello, A., Krohn, S., von Schwanenflug, N., Chien, C., Bellmann-Strobl, J., Ruprecht, K., et al. (2022). Functional connectivity dynamics reflect disability and multi-domain clinical impairment in patients with relapsing-remitting multiple sclerosis. Neuroimage Clin. 36:103203. doi: 10.1016/j.nicl.2022.103203
Rosenthal, J. F., Hoffman, B. M., and Tyor, W. R. (2020). CNS inflammatory demyelinating disorders: MS, NMOSD and MOG antibody associated disease. J. Investig. Med. 68, 321–330. doi: 10.1136/jim-2019-001126
Rossi, S., Studer, V., Motta, C., Polidoro, S., Perugini, J., Macchiarulo, G., et al. (2017). Neuroinflammation drives anxiety and depression in relapsing-remitting multiple sclerosis. Neurology 89, 1338–1347. doi: 10.1212/WNL.0000000000004411
Sastre-Garriga, J., Pareto, D., Battaglini, M., Rocca, M. A., Ciccarelli, O., Enzinger, C., et al. (2020). MAGNIMS consensus recommendations on the use of brain and spinal cord atrophy measures in clinical practice. Nat. Rev. Neurol. 16, 171–182. doi: 10.1038/s41582-020-0314-x
Schlaeger, R., Papinutto, N., Panara, V., Bevan, C., Lobach, I. V., Bucci, M., et al. (2014). Spinal cord gray matter atrophy correlates with multiple sclerosis disability. Ann. Neurol. 76, 568–580. doi: 10.1002/ana.24241
Schmaal, L., Pozzi, E., Ho, C., van Velzen, L. S., Veer, I. M., Opel, N., et al. (2020). ENIGMA MDD: Seven years of global neuroimaging studies of major depression through worldwide data sharing. Transl. Psychiatry 10, 1–19. doi: 10.1038/s41398-020-0842-6
Sinnecker, T., Mittelstaedt, P., Dörr, J., Pfueller, C. F., Harms, L., Niendorf, T., et al. (2012). Multiple sclerosis lesions and irreversible brain tissue damage: A comparative ultrahigh-field strength magnetic resonance imaging study. Arch. Neurol. 69, 739–745. doi: 10.1001/archneurol.2011.2450
Snoek, L., Miletić, S., and Scholte, H. S. (2019). How to control for confounds in decoding analyses of neuroimaging data. Neuroimage 184, 741–760. doi: 10.1016/j.neuroimage.2018.09.074
Stangel, M., Penner, I. K., Kallmann, B. A., Lukas, C., and Kieseier, B. C. (2015). Towards the implementation of ‘no evidence of disease activity’ in multiple sclerosis treatment: The multiple sclerosis decision model. Ther. Adv. Neurol. Disord. 8, 3–13. doi: 10.1177/1756285614560733
Strik, M., Shanahan, C. J., van der Walt, A., Boonstra, F. M. C., Glarin, R., Galea, M. P., et al. (2021). Functional correlates of motor control impairments in multiple sclerosis: A 7 Tesla task functional MRI study. Hum. Brain Mapp. 42, 2569–2582. doi: 10.1002/hbm.25389
Tarrants, M., Oleen-Burkey, M., Castelli-Haley, J., and Lage, M. J. (2011). The impact of comorbid depression on adherence to therapy for multiple sclerosis. Mult. Scler. Int. 2011:271321. doi: 10.1155/2011/271321
Thiebaut de Schotten, M., Foulon, C., and Nachev, P. (2020). Brain disconnections link structural connectivity with function and behaviour. Nat. Commun. 11:5094. doi: 10.1038/s41467-020-18920-9
Thompson, A. J., Banwell, B. L., Barkhof, F., Carroll, W. M., Coetzee, T., Comi, G., et al. (2018). Diagnosis of multiple sclerosis: 2017 revisions of the McDonald criteria. Lancet Neurol. 17, 162–173. doi: 10.1016/S1474-4422(17)30470-2
Tonietto, M., Poirion, E., Lazzarotto, A., Ricigliano, V., Papeix, C., Bottlaender, M., et al. (2023). Periventricular remyelination failure in multiple sclerosis: A substrate for neurodegeneration. Brain 146, 182–194. doi: 10.1093/brain/awac334
Tozlu, C., Jamison, K., Gu, Z., Gauthier, S. A., and Kuceyeski, A. (2021). Estimated connectivity networks outperform observed connectivity networks when classifying people with multiple sclerosis into disability groups. Neuroimage 32:102827. doi: 10.1016/j.nicl.2021.102827
Valsasina, P., Aboulwafa, M., Preziosa, P., Messina, R., Falini, A., Comi, G., et al. (2018). Cervical cord T1-weighted hypointense lesions at MR imaging in multiple sclerosis: Relationship to cord atrophy and disability. Radiology 288, 234–244. doi: 10.1148/radiol.2018172311
Valsasina, P., Gobbi, C., Zecca, C., Rovira, A., Sastre-Garriga, J., Kearney, H., et al. (2022). Characterizing 1-year development of cervical cord atrophy across different MS phenotypes: A voxel-wise, multicentre analysis. Mult. Scler. 28, 885–899. doi: 10.1177/13524585211045545
Veréb, D., Tóth, E., Bozsik, B., Király, A., Szabó, N., Kincses, B., et al. (2021). Altered brain network function during attention-modulated visual processing in multiple sclerosis. Mult. Scler. 27, 1341–1349. doi: 10.1177/1352458520958360
Voskuhl, R. R., Patel, K., Paul, F., Gold, S. M., Scheel, M., Kuchling, J., et al. (2020). Sex differences in brain atrophy in multiple sclerosis. Biol. Sex Differ. 11:49. doi: 10.1186/s13293-020-00326-3
Wandall-Holm, M. F., Buron, M. D., Kopp, T. I., Thielen, K., Sellebjerg, F., and Magyari, M. (2022). Time to first treatment and risk of disability pension in relapsing-remitting multiple sclerosis. J. Neurol. Neurosurg. Psychiatry 93, 858–864. doi: 10.1136/jnnp-2022-329058
Weeda, M. M., Pruis, I. J., Westerveld, A. S. R., Brouwer, I., Bellenberg, B., Barkhof, F., et al. (2020). Damage in the thalamocortical tracts is associated with subsequent thalamus atrophy in early multiple sclerosis. Front. Neurol. 11:575611. doi: 10.3389/fneur.2020.575611
Williams, R. M., Turner, A. P., Hatzakis, M., Bowen, J. D., Rodriquez, A. A., and Haselkorn, J. K. (2005). Prevalence and correlates of depression among veterans with multiple sclerosis. Neurology 64, 75–80. doi: 10.1212/01.WNL.0000148480.31424.2A
Zhuo, C., Li, G., Lin, X., Jiang, D., Xu, Y., Tian, H., et al. (2019). The rise and fall of MRI studies in major depressive disorder. Transl. Psychiatry 9, 1–14. doi: 10.1038/s41398-019-0680-6
Keywords: multiple sclerosis, magnetic resonance imaging (MRI), mental health, depression, neuroinflammation
Citation: Brasanac J and Chien C (2023) A review on multiple sclerosis prognostic findings from imaging, inflammation, and mental health studies. Front. Hum. Neurosci. 17:1151531. doi: 10.3389/fnhum.2023.1151531
Received: 26 January 2023; Accepted: 21 April 2023;
Published: 11 May 2023.
Edited by:
Marcello Moccia, University of Naples Federico II, ItalyReviewed by:
Szilvia Anett Nagy, University of Pécs, HungaryCopyright © 2023 Brasanac and Chien. This is an open-access article distributed under the terms of the Creative Commons Attribution License (CC BY). The use, distribution or reproduction in other forums is permitted, provided the original author(s) and the copyright owner(s) are credited and that the original publication in this journal is cited, in accordance with accepted academic practice. No use, distribution or reproduction is permitted which does not comply with these terms.
*Correspondence: Claudia Chien, Y2xhdWRpYS5jaGllbkBjaGFyaXRlLmRl