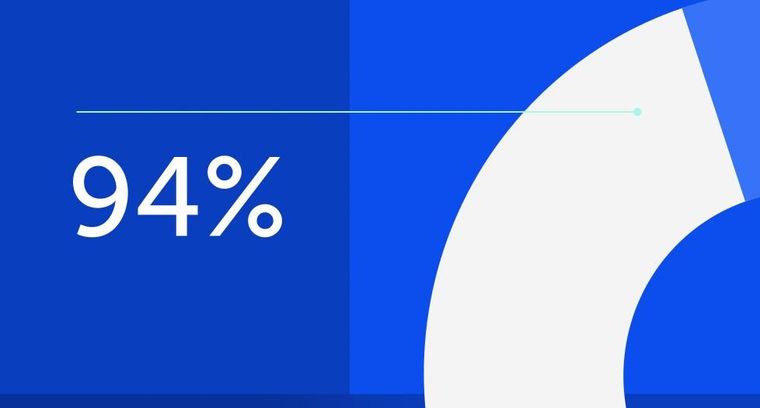
94% of researchers rate our articles as excellent or good
Learn more about the work of our research integrity team to safeguard the quality of each article we publish.
Find out more
STUDY PROTOCOL article
Front. Hum. Neurosci., 31 August 2022
Sec. Brain Imaging and Stimulation
Volume 16 - 2022 | https://doi.org/10.3389/fnhum.2022.971062
Background: Temporal lobe epilepsy (TLE) is the most prevalent refractory focal epilepsy and is more likely accompanied by cognitive impairment. The fully understanding of the neuronal activity underlying TLE is of great significance.
Objective: This study aimed to comprehensively explore the potential brain activity abnormalities affected by TLE and detect whether the changes were associated with cognition.
Methods: Six static intrinsic brain activity (IBA) indicators [amplitude of low-frequency fluctuation (ALFF), fractional ALFF (fALFF), regional homogeneity (ReHo), degree centrality (DC), global signal correlation (GSCorr), and voxel-mirrored homotopic connectivity (VMHC)] and their corresponding dynamic indicators, such as dynamic ALFF (dALFF), dynamic fALFF (dfALFF), dynamic ReHo (dReHo), dynamic DC (dDC), dynamic VMHC (dVMHC), and dynamic GSCorr (dGSCorr), in 57 patients with unilateral TLE and 42 healthy volunteers were compared. Correlation analyses were also performed between these indicators in areas displaying group differences and cognitive function, epilepsy duration, and severity.
Results: Marked overlap was present among the abnormal brain regions detected using various static and dynamic indicators, primarily including increased ALFF/dALFF/fALFF in the bilateral medial temporal lobe and thalamus, decreased ALFF/dALFF/fALFF in the frontal lobe contralateral to the epileptogenic side, decreased fALFF, ReHo, dReHo, DC, dDC, GSCorr, dGSCorr, and VMHC in the temporal neocortex ipsilateral to the epileptogenic foci, decreased dReHo, dDC, dGSCorr, and dVMHC in the occipital lobe, and increased ALFF, fALFF, dfALFF, ReHo, and DC in the supplementary motor area ipsilateral to the epileptogenic foci. Furthermore, most IBA indicators in the abnormal brain region significantly correlated with the duration of epilepsy and several cognitive scale scores (P < 0.05).
Conclusion: The combined application of static and dynamic IBA indicators could comprehensively reveal more real abnormal neuronal activity and the impairment and compensatory mechanisms of cognitive function in TLE. Moreover, it might help in the lateralization of epileptogenic foci and exploration of the transmission and inhibition pathways of epileptic activity.
As one of the most common cerebral diseases, epilepsy affects more than 70 million people worldwide (Thijs et al., 2019). Temporal lobe epilepsy (TLE) is the most prevalent refractory focal epilepsy (Engel, 2001), and is more likely accompanied by cognitive impairment, particularly in memory and language (Allone et al., 2017). It also impairs advanced social cognition, such as perception and personality (Schacher et al., 2006), accompanied by depression, anxiety, or other psychiatric symptoms (Keezer et al., 2016). Considering the high morbidity, severe cognitive problems, and poor quality of life, the neuropathologic mechanisms underlying TLE should be fully understood to facilitate the development of a more precise diagnosis and effective treatment (Wang et al., 2021).
Temporal lobe epilepsy is a disorder of large neural networks affecting brain activity (Spencer, 2002; Cataldi et al., 2013). Spencer (2002) first proposed the concept of “medial temporal/limbic network” in 2002, believing that it was bilateral, cortical, and subcortical, and included hippocampi, amygdala, entorhinal cortices, lateral temporal neocortices, and extratemporal components of the medial thalamus and inferior frontal lobes. In addition, Blumenfeld et al. (2004), (Norden and Blumenfeld, 2002; Blumenfeld and Taylor, 2003) proposed a “network inhibition hypothesis” in which complex partial seizures arising in the temporal lobe propagated to the medial thalamus and upper brainstem reticular formation, disrupting their normal activating functions. This, in turn, might lead secondarily to functional inactivation of widespread regions of the frontal and parietal association cortices, causing the loss of consciousness. Thus, anatomical structures that are abnormally activated or inhibited could contribute to epileptic symptoms, impaired cognitive function (Holmes et al., 2014; Burianová et al., 2017), or psychiatric complications (Jiang et al., 2018). The existence and importance of the temporal epilepsy network have been confirmed using various modalities (Spencer, 2002), such as clinical observations, EEG (Bettus et al., 2008), SPECT (Blumenfeld et al., 2004), PET (Concha et al., 2005), volumetric (Bernasconi et al., 2003; Araújo et al., 2006), and functional MRI (Zhang et al., 2010; Maneshi et al., 2014). The study of the TLE network might contribute to our in-depth understanding of the TLE pathophysiologic mechanism and is expected to be an auxiliary tool for the clinical diagnosis or provide a new direction for the treatment.
Blood oxygenation level-dependent (BOLD) resting-state functional magnetic resonance imaging (rs-fMRI) focuses on spontaneous/intrinsic fluctuations of BOLD signals in a low-frequency band (<0.1 Hz). Its functional significance was first proposed by Biswal et al. (1995). Intrinsic brain activity (IBA) plays a vital role in brain function (Fox and Raichle, 2007; Raichle, 2010, 2015). A growing number of rs-fMRI-based IBA measurements have been proposed, such as functional connectivity (FC) (Biswal et al., 1995), amplitude of low-frequency fluctuation (ALFF) (Zang et al., 2007), fractional ALFF (fALFF) (Zou et al., 2008), regional homogeneity (ReHo) (Zang et al., 2004), degree centrality (DC) (Zuo et al., 2012), voxel-mirrored homotopic connectivity (VMHC) (Zuo et al., 2010), global signal correlation (GSCorr) (Yan et al., 2017), and independent component analysis (ICA) (Beckmann et al., 2005). Each method aims to reveal a distinct aspect of intrinsic brain function (Margulies et al., 2010). The distinctions and commonalities among these measures are yet to be fully explored (Yan et al., 2017).
Temporal lobe epilepsy can affect brain activity. Zhang et al. (2010) and Singh et al. (2020) demonstrated that patients with TLE had increasing ALFF in the mesial temporal epilepsy network regions and decreasing ALFF in the default-mode network (DMN) regions. Zhang et al. (2010) also demonstrated that the increases in ALFF and ReHo might be associated with the interictal epileptiform discharges, and ALFF might be applied for the lateralization. Previous studies have focused mainly on ALFF, fALFF, or ReHo (Zeng et al., 2013; Zhao et al., 2020), and the results were partially inconsistent. Studies on VMHC (Xu et al., 2014; Shi et al., 2021), DC (Liang et al., 2020; Nakai et al., 2021), and GSCorr are rare or even missing.
Moreover, the aforementioned traditional IBA measurements are static, assuming that the BOLD signal is stationary during the scanning period (Liao et al., 2014). However, the activity of the human brain is environmentally sensitive and activity-dependent, implying that the IBA is highly dynamic and fluctuates over time, which underlies the integration of brain function (Park et al., 2018). Thus, static IBA metrics are insufficient to describe the transient changes in IBA. Fortunately, recent investigations of brain activity have taken fluctuation over time into account, which can be quantified by measuring the temporal variance through the sliding window approach (Chen et al., 2017). By calculating the standard deviation (SD) or variability of the aforementioned metrics in all the windows, we can acquire dynamic IBA metrics, such as dynamic FC (dFC), dynamic ALFF (dALFF), dynamic fALFF (dfALFF), dynamic ReHo (dReHo), dynamic DC (dDC), dynamic VMHC (dVMHC), and dynamic GSCorr (dGSCorr). These metrics, extended from static to dynamic, reflect the dynamic change degree of each index in the time dimension. They not only further reflect rich dynamic information of IBA, but also proved to have high stability (Yan et al., 2017).
In a literature search of the aforementioned IBA dynamic indicators, most research on TLE was found to focus on dynamic FC studies (Jiang et al., 2020; Li et al., 2022; Pang et al., 2022). However, the relevant studies which applied the other dynamic indicators, such as dALFF, dfALFF, dReHo, dDC, dVMHC and dGSCorr, in TLE patients could not be retrieved. Only one piece of literature has been retrieved to investigate the dALFF alteration in benign childhood epilepsy (BECTS) (Morgan et al., 2020).
Consequently, this study was performed to comprehensively understand the abnormalities of IBA in patients with TLE and analyze the consistency and complementarity of various indicators. We studied the static and temporal dynamic characteristics of IBA in patients with unilateral TLE from the perspectives of local activity (ALFF and fALFF), local synchronization or connectivity (ReHo and VMHC), and the whole brain connectivity (DC and GSCorr). Moreover, the correlations between abnormal IBA and cognition, epilepsy duration, or severity were explored. These might enhance the understanding of the relationship between TLE and cognition deficits.
The participants were divided into two groups. The TLE group was comprised of 64 patients with unilateral TLE who had consulted the Neurology Clinic and Inpatient Department of The First Affiliated Hospital of Zhengzhou University. The healthy control (HC) group was comprised of 45 healthy volunteers from the local communities matched for age and sex.
The diagnosis was made based on a detailed history, neurologic examination, electroencephalography (EEG) recordings, and imaging findings. The inclusion criteria for patients were as follows: (1) all right-handed, (2) >14 years old, and (3) met any 2 or more of the following criteria: ① clinical semiology consistent with seizures of the unilateral left/right temporal lobe origin, ② interictal or ictal epileptic discharges of the unilateral left/right temporal lobe origin according to scalp EEG or intracranial electrode EEG, ③ MRI results that suggested the left/right hippocampal sclerosis of the patients or positron emission tomography (PET) results that suggested the left/right hypometabolic temporal lobe. The exclusion criteria for patients were as follows: (1) patients with brain structural abnormalities (except for hippocampal sclerosis), other systemic diseases, psychiatric disorders, history of alcohol abuse, and so forth, (2) unidentified lateralization of TLE, and (3) patients who have taken antiepileptic drugs regularly.
The inclusion criteria for controls were as follows: (1) normal head MRI results, (2) >14 years old, and (3) all right-handed. The exclusion criteria for controls were as follows: patients with any prior history of neurologic or psychiatric diseases, history of alcohol or cigarette abuse, and so forth. Handedness was rated according to the Edinburgh Handedness Inventory.
This study was approved by the Research Ethics Committee at The First Affiliated Hospital of Zhengzhou University (No. 2019-KY-232), and informed consent was obtained from all participants or their legal guardian/next of kin.
Resting-state fMRI data were acquired using a 3-T Magnetom Prisma MRI scanner (Siemens Healthcare, Erlangen, Germany) with a 64-channel head coil. All participants were advised to lie down flat, not think, close their eyes, and breathe quietly. 3D T1-weighted structural images were acquired using a magnetization prepared rapid acquisition gradient echo sequence with the following parameters: TR/TE = 2,300/2.32 ms; FOV = 240 mm × 240 mm; slice number = 176; and voxel size = 0.9 × 0.9 × 0.9 mm3. Functional images were acquired using a simultaneous multi-slice (SMS) echo-planar imaging sequence with the following parameters and a total scan time of 400 s: TR/TE = 1,000/30 ms; FOV = 220 × 220 mm2; slice thickness = 2.2 mm; flip angle = 70; voxel size = 2.0 × 2.0 × 2.2 mm3; slice number = 52; volumes = 400; SMS = 4.
For all the participants in the TLE and HC groups, the clinical data such as sex, age, education years, and epilepsy duration years were recorded. All participants underwent national hospital seizure severity scale (NHS3) testing and neuropsychologic testing prior to scanning to measure their seizure severity and cognitive function. The neuropsychologic test included Mini-Mental State Examination (MMSE), memory and executive screening (MES), Montreal cognitive assessment-basic (MOCA-B), auditory verbal learning test (AVLT), and shape trail making test-A/B (STT-A/B). The AVLT test included 6 scores: immediate recall scores (N1, N2, and N3), 5-min short delayed recall score (N4), 20-min long-delayed recall score (N5), and reconfiguration. The scales used in this study were all in Mandarin Chinese versions. The aforementioned scale was evaluated by the same physician who had passed the systematic training and examination.
The rs-fMRI data were preprocessed using the Data Processing Assistant for Resting-State fMRI Analysis Toolkit (DPARSF, V5.2) (Chao-Gan and Yu-Feng, 2010). The first 10 volumes were deleted, and then slice-timing and realignment were performed. Participants with head motion of >2.5 mm in maximum displacement or >2.5 rotation in angular motion were excluded. Seven patients with TLE and 3 healthy controls were excluded because of excessive head motion. Next, the functional images were spatially normalized to the standard EPI template and resampled to 3 × 3 × 3 mm3. Several spurious variances, including 24 head motion parameters, cerebrospinal fluid signals, and white matter signals, were regressed by multiple linear regression analysis. Moreover, the time points whose mean frame-wise displacement (FD) (Power et al., 2012) value exceeded 0.2 mm and the linear regression factor were also regressed in this step. Subsequently, functional images were temporal bandpass filtered between 0.01 and 0.1 Hz.
The Data Processing and Analysis of Brain Imaging (DPABI, V6.0_210501) toolbox (Yan et al., 2016) was used to calculate ALFF, fALFF, ReHo, DC, GSCorr, and VMHC. The calculation of ALFF was based on the low-frequency range from 0.01 to 0.1 Hz. Then, we calculated fALFF by obtaining the ratio of the power spectrum of low frequency (0.01–0.1 Hz) to that of the entire frequency range (0–0.25 Hz). For ReHo, Kendall’s coefficient of concordance of the time course of every 27 nearest neighboring voxels was calculated (Zang et al., 2004). DC (Zuo et al., 2012) was defined as the weighted sum of voxels whose correlation coefficient with all the other voxels in the whole brain was above the threshold (r > 0.25 in this study). GSCorr (Power et al., 2017) was calculated as the Pearson correlation between the global signal and all the other voxels in the whole brain with Fisher Z-transformation. Before calculating VMHC, T1 image segmentation and smooth (with a 6-mm full width at half-maximum (FWHM)] Gaussian kernel were processed. Considering the differences in geometric properties between the bilateral cerebral hemispheres, we re-normalized each T1 structure image to the Montreal Neurological Institute (MNI) template with symmetrical left and right brains using non-linear registration. The foregoing MNI templates were applied to the processed fMRI data. The functional homotopy of each pair of mirrored voxels was calculated using Pearson’s correlation coefficient. Subsequently, the Fisher Z-transformation was used to convert the coefficient values into VMHC values.
The dynamic parameters (dALFF, dfALFF, dReHo, dDC, dGSCorr, and VMHC) were analyzed using temporal dynamic analysis toolkits (Yan et al., 2017) based on DPABI. An approach based on a sliding window, which was sensitive in detecting time-dependent variations, was performed to characterize the temporal dynamic patterns (Hindriks et al., 2016). Ideally, the length of a window should be small enough to monitor potentially transient signals and large enough to analyze the lowest fluctuations of interest in the signals (Sakoğlu et al., 2010). Previous studies on sliding window connectivity have applied a sliding window length as small as 10 s (Thompson et al., 2013) and as long as 180 s (Gonzalez-Castillo et al., 2015). In this study, a moderate-length sliding rectwin window of 60 TRs (60 s) and a shifting step size of 30 TRs (30 s) were applied. We also examined the effect with other window lengths and step sizes, and they were included in validation analyses.
To track the dALFF (similar to other indicators), we used a sliding-window approach to separate the full-length BOLD fMRI time series into a series of small data segments (60 TRs in this study). After applying the rectwin windows to BOLD signals, we obtained a windowed time series of 12 windows in total. Then, within each window, we calculated the ALFF for every voxel in the brain. The sliding window was systematically shifted by 30 TRs and the corresponding ALFF was computed. This process was performed until the entire data length was covered. The temporal variability of dALFF was defined as the standard deviation (SD) of dALFF maps across time windows.
The maps of all the static and dynamic indicators were Z-standardized (Yan et al., 2016) within a gray matter mask across all the voxels and then spatially smoothed (except VMHC) with a Gaussian kernel of 6-mm FWHM to reduce the global effects of variability across participants and improve the signal-to-noise ratio.
For expanding the study sample size and explaining the group changes as either ipsilateral or contralateral to left temporal lobe epilepsy (LTLE) (Blumenfeld et al., 2009; Zeng et al., 2013), we right/left flipped the maps of the 27 patients with right temporal lobe epilepsy (RTLE) to obtain the mirror copies as LTLE and then finally obtained the data of 57 LTLE for the subsequent statistical analysis.
The demographic and cognitive scale scores were evaluated between the groups of TLE and HC using SPSS software (version 17.0; IBM, IL, United States). Differences in sex were analyzed with a chi-square test (P < 0.05). Differences in age and cognitive scale scores were analyzed with a 2-sample t-test or a non-parametric test (P < 0.05).
To examine the between-group differences in the 12 measurements of static and dynamic indicators, we employed a 2-sample t-test using DPABI, with age, sex, education, and mean FD as covariates. Multiple comparison correction was performed based on the Gaussian random field theory (GRF, voxelwise P < 0.005, cluster-wise P < 0.05). A similar group comparison was also applied between LTLE and RTLE groups. The figures were drawn using both DPABI and BrainNet Viewer (Xia et al., 2013).
With the peak voxels of abnormal regions as spherical centers, spherical regions of intrest (ROIs) were constructed around these abnormal regions (with a 6-mm radius). We used Spearman correlation analysis with SPSS software (version 17.0; IBM, IL, United States) to assess the relationship between metrics in these abnormal regions and the epilepsy duration, NHS3, and cognitive scores. Statistical significance was defined as P < 0.05.
We carried out additional analyses to validate our findings of the dynamic IBA indicators. We examined the difference with other window sizes/step sizes (60/10 TRs and 80/10 TRs) to validate our results.
The demographic, clinical, and neuropsychologic characteristics of 57 patients with TLE and 42 HCs are listed in Table 1. No remarkable differences were noted in age and sex between the TLE and HC groups (P > 0.05). Cognitive decline was observed in patients with TLE. MMSE, MES, MOCA-B, and AVLT (N1, N2, N3, N4, N5, and recognition) all decreased in the TLE group compared with the HC group (P < 0.05). However, STT-A and STT-B increased in the TLE group (P < 0.05).
Increased ALFF values were observed in the left median cingulate gyrus (MCG), posterior cingulate gyrus (PCG), cerebellum 4_5/6/8/9, hippocampus, thalamus, supplementary motor area (SMA), precuneus, cuneus, right pallidum, lenticula, amygdala, and parahippocampal gyrus (PHG) in the TLE group compared with the HC group. However, TLE displayed decreasing ALFF values in the right cerebellum Crus1, precentral gyrus (PreCG), postcentral gyrus (PostCG), triangular inferior frontal gyrus (TIFG), middle frontal gyrus (MFG), and orbital frontal gyrus (OFG) (GRF corrected, Pvoxel < 0.005, Pcluster < 0.05; Figure 1A and Table 2).
Figure 1. Brain regions showing considerably altered static ALFF (A), fALFF (B), ReHo (C), DC (D), GSCorr (E), and VMHC (F) between TLE and HCs groups. GRF corrected; voxel-wise P < 0.005, cluster-wise P < 0.05. The color bar indicates the t-value. Warm colors indicated increased indicator values, while cold colors indicated decreased indicator values. ALFF, amplitude of low-frequency fluctuation; DC, degree centrality; fALFF, fractional ALFF; GRF, Gaussian random field theory; GSCorr, global signal correlation; ReHo, regional homogeneity; TLE, temporal lobe epilepsy; VMHC, voxel-mirrored homotopic connectivity.
Increased dALFF variability was observed in the bilateral MCG, left PCG, left precuneus, left cerebellum 4_5/6/8/10, bilateral hippocampus, thalamus, right basal ganglia, right amygdala, and bilateral olfactory cortex in the TLE group compared with the HC group. However, TLE displayed decreasing dALFF variability in the right OFG, superior frontal gyrus (SFG), and rectus gyrus (GRF corrected, Pvoxel < 0.005, Pcluster < 0.05; Figure 2A and Table 2).
Figure 2. Brain regions showing considerably altered dALFF (A), dfALFF (B), dReHo (C), dDC (D), dGSCorr (E), and dVMHC (F) between patients with TLE and HCs. GRF corrected; voxel-wise P < 0.005, cluster-wise P < 0.05. The color bar indicates the t-value. Warm colors indicated increased indicator values, while cold colors indicated decreased indicator values. dALFF, dynamic amplitude of low-frequency fluctuation; dfALFF, dynamic fractional ALFF; dDC, dynamic degree centrality; dGSCorr, dynamic global signal correlation; GRF, Gaussian random field theory; HCs, healthy controls; dReHo, dynamic regional homogeneity; TLE, temporal lobe epilepsy; dVMHC, dynamic voxel-mirrored homotopic connectivity.
Increased fALFF values were detected in the bilateral hippocampus, right amygdala, right temporal pole (TP), left middle temporal gyrus (MTG), inferior temporal gyrus (ITG), superior marginal gyrus (SMG), SMA, SFG, PHG, and thalamus in the TLE group compared with the HC group. However, the fALFF values decreased in the left SMG, MTG, superior temporal gyrus (STG), angular gyrus (AG), and right PreCG, PostCG, MFG, and IFG (GRF corrected, Pvoxel < 0.005, Pcluster < 0.05; Figure 1B and Table 2).
Increased dfALFF variability was detected in the bilateral SMA, left medial superior frontal gyrus (MSFG), and right dorsolateral superior frontal gyrus (DSFG) in the TLE group compared with the HC group (GRF corrected, Pvoxel < 0.005, Pcluster < 0.05; Figure 2B and Table 2).
Increased ReHo values in the left SMA and MSFG but decreased ReHo values in the left MTG, STG, ITG, TP, and SMG were detected in the TLE group compared with the HC group increased ReHo values were found in the left SMA, MSFG, but decreased ReHo values were found in the left MTG, STG, ITG, TP, SMG (GRF corrected, Pvoxel < 0.005, Pcluster < 0.05; Figure 1C and Table 2).
For dReHo, TLE decreased in left STG, Heschl’s gyrus, SMG, MTG, and right calcarine cortex (CAL), and cuneus (GRF corrected, Pvoxel < 0.005, Pcluster < 0.05; Figure 2C and Table 2).
Increased DC values in the left precuneus, cuneus, CAL, and SMA but decreased DC values in the left MTG, STG, ITG, SMG, and Heschl’s gyrus were noted in the TLE group compared with HC group (GRF corrected, Pvoxel < 0.005, Pcluster < 0.05; Figure 1D and Table 2).
Increased dDC variability in the left MTG but decreased dDC variability in bilateral CAL and cuneus, left superior occipital gyrus (SOG), left lingual gyrus (LG), left STG, and right precuneus were detected in the TLE group compared with the HC group (GRF corrected, Pvoxel < 0.005, Pcluster < 0.05; Figure 2D and Table 2).
Increased GSCorr values in the left MTG and ITG but decreased GSCorr values in the left TP, MTG, STG, ITG, and Heschl’s gyrus were observed in the TLE group compared with the HC group (GRF corrected, Pvoxel < 0.005, Pcluster < 0.05; Figure 1E and Table 2).
Decreased dGSCorr variability was detected in the bilateral CAL, cuneus, left SOG, left middle occipital gyrus (MOG), left inferior occipital gyrus (IOG), right LG, left STG, and Heschl’s gyrus in the TLE group compared with the HC group (GRF corrected, Pvoxel < 0.005, Pcluster < 0.05; Figure 2E and Table 2).
Decreased VMHC values were noted in the bilateral MTG, ITG, STG, TP, cerebellum Crus1/Crus2/6, and precuneus in the TLE group compared with the HC group (GRF corrected, Pvoxel < 0.005, Pcluster < 0.05; Figure 1F and Table 2).
For dVMHC, TLE decreased in bilateral CAL, cuneus, and paracentral lobule (PCL) (GRF corrected, Pvoxel < 0.005, Pcluster < 0.05; Figure 2F and Table 2).
We have performed a statistical analysis of the aforementioned static and dynamic IBA indicators between the LTLE (30 patients) and RTLE groups (27 patients). However, no significant cluster was found between LTLE and RTLE groups after multiple comparison correction (GRF corrected, Pvoxel < 0.005, Pcluster < 0.05).
As depicted in Table 3, significant correlations were detected between the values of ALFF, fALFF, ReHo, DC, GSCorr, VMHC, dALFF, dfALFF, dReHo, dDC, and dGSCorr in abnormal brain regions and several cognitive scales scores (P < 0.05). Moreover, most of them significantly correlated with the duration of epilepsy (P < 0.05). However, no significant correlation was observed between dVMHC and duration of epilepsy, NHS3, or cognitive scales scores (P > 0.05).
Table 3. Correlations between the values of IBA indicators in abnormal brain regions and epilepsy duration, NHS3, or cognitive scales scores.
When other window sizes/step sizes (60/10 TRs and 80/10 TRs) were used to validate the findings of the dynamic IBA indicators, the results were basically consistent with our main results.
We identified alteration of IBA in patients with TLE using static and dynamic ALFF, fALFF, ReHo, DC, VMHC, and GSCorr in TLE and HC groups. A large amount of overlap existed among the abnormal brain regions detected using various indicators. The convergence of the findings might suggest the existence of a more fundamental mechanism capable of revealing more real abnormal neuronal activity in TLE other than specific effects caused by particular imaging algorithms.
In our study, brain regions displaying considerable differences overlapped in ALFF, dALFF, and fALFF, which increased in the bilateral medial temporal lobe (MTL) and thalamus. Epileptic seizures could initiate a neural circuit and lead to aberrant neural communication with brain areas outside the epileptogenic region. MTL and thalamus were reported to compose a temporal epilepsy network proposed previously, which were involved in the initiation, propagation, and facilitation of epileptic activity (Spencer, 2002). An increase in ALFF in these regions has been reported in the study by Zhang et al. (2010). Moreover, the widespread increases in ALFF/dALFF or fALFF in various cortical and subcortical structures (cerebellum, CG, precuneus, SMA, and pons ipsilateral to the epileptogenic foci) besides the MTL and thalamus might also implicate the pathologic substrates of an epileptic network in TLE (Schacher et al., 2006).
The ALFF changes in the BOLD signal have been suggested to be associated with local neuronal activity (Logothetis et al., 2001; Yang et al., 2007) and interictal epileptic discharges (IEDs) (Zhang et al., 2010). fALFF represents the ratio of the low-frequency power spectrum to that of the entire frequency range, which could effectively suppress the physiologic noise (Zou et al., 2008). Liao et al. (2019) demonstrated that the electrophysiologic relevance of ALFF variability depended on EEG power fluctuations, suggesting a neurophysiologic basis. Thus, dALFF was supposed to represent the temporal variability of IBA fluctuation, and higher dALFF represented greater volatility and instability of the neuronal activity. The increase in ALFF, fALFF, and dALFF indicated that epileptic activity might not only induce hyperactivity of neuronal activity in the epilepsy network but also cause an abnormal unstable state of neuronal activity, further leading to a chaotic state of brain function.
Medial temporal lobe structures, such as the hippocampus, PHG, and amygdala, are involved in memory, learning, and emotion regulation (Burwell, 2000; Viard et al., 2007). Excessive but unstable brain activity in the hippocampus and PHG may lead to the failure to accomplish learning and memory processes efficiently. The hyperactivity of the amygdala may contribute to the fear aura, emotional lability, and temperament change. Thalamus was reported to be a relay center in a complex reciprocal network linking cortices and other subcortical structures (Behrens et al., 2003; Blumenfeld et al., 2004) and responsible for information integration and executive functioning (Tuchscherer et al., 2010). Our correlation analysis results confirmed that increased ALFF, fALFF, or dALFF in MTL and thalamus was related to longer epilepsy duration and more serious cognition decline. These results suggested that TLE, as a persisting disease, revealed a gradually aggravated effect on the brain activity and cognition of patients. Considering the thalamus as an example, the increased fALFF and dALFF in the thalamus negatively correlated with MMSE and MES/MOCA, respectively. In addition, increased fALFF and dALFF both negatively correlated with the time consumption of STT-A/B. These indicated that excessive but unstable activity in the thalamus was related to instability of the information transmission and further execution or cognition impairment.
In our study, regions displaying decreased ALFF, fALFF, or dALFF, mainly located in the frontal lobe contralateral to the epileptogenic side, overlapped with the regions of PreCG, PostCG, IFG, MFG, and OFG. The sensorimotor network (SMN) was mainly composed of PreCG, PostCG, and SMA. The decrease in ALFF and fALFF in SMN might represent the decline or inhibition of brain activity and lead to a series of motor symptoms or disorders caused by epilepsy (Laufs et al., 2014). Moreover, as an advanced cognitive center, the frontal lobe was also essential for the language, execution, and control functions (Rosch and Mostofsky, 2019) and was a component of the cognitive-attention network (Bush, 2011). OFG could adaptively shape decision-making and affective behavior (Rudebeck and Rich, 2018). As a structure related to epileptic activity propagation, the frontal lobe could be highly affected. Its dysfunction in TLE has been affirmed by behavioral tests (Hermann et al., 1991), fALFF based on rs-fMRI (Reyes et al., 2016), as well as PET (Guedj et al., 2015) and SPECT (Blumenfeld et al., 2004) imaging.
Similar to these studies, our results indicated that the decreasing ALFF and fALFF values in the contralateral PostCG negatively correlated with epilepsy duration and STT-B, suggesting that the activity decline in PostCG was associated with the impairment of execution-control functions and cognitive flexibility, which was reflected by STT, and worsened with prolonged epilepsy duration. According to the “network inhibition hypothesis” of TLE proposed by Blumenfeld et al. (2004), (Norden and Blumenfeld, 2002; Blumenfeld and Taylor, 2003), we cautiously speculated that the negative activation changes in ALFF, fALFF, and dALFF in the frontal lobe further confirmed the existence of the inhibition network. On the one hand, the negative activation represented functional inactivation, which would lead to cognition decline and loss of consciousness. On the other hand, it might represent an inhibitory effect of the brain on epileptic activity.
Our study demonstrated that various static and dynamic IBA indicators, including fALFF, ReHo, dReHo, DC, dDC, GSCorr, dGSCorr, and VMHC, decreased in the temporal neocortex ipsilateral to the epileptogenic foci. A previous VMHC study verified the decisive role of the temporal lobe in distinguishing TLE from HC (Gao et al., 2021). These highly consistent results suggested a clinical potential for the disease classification and lateralization of TLE using IBA indicators.
The temporal neocortex included the structure of STG, MTG, ITG, TP, and Heschl’s gyrus, and was related to auditory function, language, memory, cognitive function, and emotion (Olson et al., 2007). Primary and association auditory cortices (Brodmann 41 and 42) and partial Wernicke’s area were located at STG; they were responsible for auditory and language information processing and also memory and cognitive function (Fletcher et al., 1995). The dysfunction of the auditory cortex might induce acousma and impairment of auditory-related cognition. MTG (Kiehl et al., 2004) and ITG (Dien et al., 2013) were also thought to be the key regions for language processing and formulation. Accessory visual areas (Brodmann 20 and 21), which were related to visual perception, were located at MTG and ITG, respectively. Moreover, the ITG was a key node in the broad network of frontal, temporal, parietal, occipital, and subcortical structures. Numerous studies demonstrated that fMRI revealed abnormality of the temporal neocortex and its correlation with cognitive function (Vaughan et al., 2016; Trimmel et al., 2018; Gao et al., 2021).
Similarly, our correlation analysis results further demonstrated that the abnormal IBA of the temporal neocortex was associated with multiple cognitive scores and duration of epilepsy. The decreasing ReHo and VMHC in MTG negatively correlated with epilepsy duration but positively correlated with MMSE or MOCA. The GSCorr in ITG positively correlated with AVLT. These findings indicated that the weakness of local and global function in the temporal neocortex was a crucial feature in patients with unilateral TLE, and might be responsible for the impairment of memory and cognitive function, which would deteriorate with time.
However, during the correlation assessment of dynamic indicators, we demonstrated that the decreasing dReHo, dDC, and dGSCorr were negatively correlated with AVLT. This might seem contrary to the aforementioned speculation, but in fact, the lower temporal variability represented a relatively more stable functional status, which we considered to reflect the existence of a “decompensatory mechanism” (Yang et al., 2018; Li et al., 2020; Singh et al., 2020). The existence of functional compensation has been reported in previous fMRI research (Yang et al., 2018; Li et al., 2020; Singh et al., 2020). It might improve the patients’ memory to a certain extent but could not replace the original functions effectively and might cause other functional disorders such as the decline in cognitive flexibility due to the low temporal variability in our study.
According to the implication represented by each indicator, we speculated that the dysfunction in the temporal neocortex was reflected not only in a decline of IBA intensity but also in reduced functional connectivity among neighboring regions, interhemispheric homotopic regions, and the remaining brain regions. Thus, large clusters of neurons were involved in inducing great interference in global brain function networks. The highly consistent decreased activation of static and dynamic IBA indicators might indicate a more fundamental regulatory mechanism in TLE, which we prudently speculated to be the “surround inhibition hypothesis” (Schwartz and Bonhoeffer, 2001; Van Paesschen et al., 2003; Blumenfeld et al., 2004). It was hypothesized that the regions surrounding the epileptogenic focus might serve an adaptive function to cut down the possibility of seizure spread to other regions. Actually, TLE was mostly confined to the limbic cortex and rarely generalized. Combined with the aforementioned decreased activation of the frontal lobe, we further speculated that the temporal neocortex was also the component of the TLE inhibition network.
In our study, the most impressive result of dynamic IBA indicators was that dReho, dDC, dGSCorr, and dVMHC decreased in the bilateral occipital lobe. However, no abnormal changes in the occipital lobe were detected in the static indicators, indicating the complementarity of dynamic and static indicators. The temporal dynamic indicators were expected to become a novel and more powerful and sensitive imaging-related neurobiologic indicator.
Visual network (VIN) was part of the sensor cortex systems and mainly located in the occipital lobe, including CAL, LG, cuneus, and so forth. As the center of the visual cortex, the occipital lobe exhibited a range of visual functions, such as vision processing and visual memory encoding (Mechelli et al., 2000), and was related to executive function and attention (Ishai et al., 2002) because the acquisition of visual information was a prerequisite for attention. Memory and alertness function depend on sensory-perceptual information processing, such as vision and audition (Conway, 2001). Thus, the occipital lobe’s dysfunction was not merely related to a primary visual abnormality, such as visual hallucinations or auras, but also to high-order visual function and cognition impairment (Alessio et al., 2013).
Previous studies detected VIN abnormalities in patients with TLE (Zhang et al., 2009; Cataldi et al., 2013). Lu et al. (2018) reported a consistent decline in dynamic ReHo, DC, and ALFF in the occipital lobe of patients with autism. According to the implication represented by each static indicator, we speculated that the decreases in dReho, dVMHC, dDC, and dGSCorr suggested a lack of essential flexibility and plasticity in functional connectivity among neighboring regions, interhemispheric homotopic regions, and the remaining brain regions. We speculated that inflexible IBA in the visual processing brain areas might lead to difficulty in instant and efficient management of visual information, short-term memory, and alertness. The STT-B mainly evaluated the flexibility of cognitive function based on the sensitivity and working speed of visual perception. Our results indicated that the decreasing dReHo values in CAL negatively correlated with STT-B, which confirmed our speculation. Besides, the epilepsy duration negatively correlated with dReHo, dDC, and dGSCorr, indicating that the IBA flexibility worsened with prolonged epilepsy duration.
Nevertheless, we demonstrated that the decreasing dDC and dGSCorr negatively correlated with AVLT, which seemed to contradict our speculation. However, as AVLT emphasized auditory and verbal memory, we speculated that this phenomenon might be related to the “decompensation mechanism” (Yang et al., 2018; Li et al., 2020; Singh et al., 2020) occurring in VIN after the severe dysfunction of the temporal neocortex in patients with TLE.
Our study demonstrated that ALFF, fALFF, dfALFF, ReHo, and DC all increased in SMA ipsilateral to the epileptogenic foci. SMA was a vital motor cortex belonging to the sensorimotor network and could evoke spasticity and cortical motor aphasia (Rostomily et al., 1991; Trimmel et al., 2018). SMA plays an indispensable role in the “intention-action” process (Kalaska, 2009), and participates in the preparation, initiation, and monitoring of complex movements (Picard and Strick, 1996; Michelon et al., 2006). Several fMRI studies have reported the functional changes in the SMA elicited by epilepsy (O’Muircheartaigh et al., 2012; Laufs et al., 2014; Morgan et al., 2020). It was suggested that SMA was responsible for motor dysfunction in patients with epilepsy and positively responded to drug treatment (Morgan et al., 2020).
The increases in ALFF, fALFF, and dfALFF represent a high amplitude and excessive unstable state of IBA due to epileptic activity. Based on this, we speculated that SMA ipsilateral to the epileptogenic foci was a crucial constituent of the TLE network and was related to the propagation and facilitation of epileptic activity. The increased ReHo and DC in SMA further verified this speculation. Similarly, the increased activation of ALFF and ReHo in SMA has been reported earlier (Zhang et al., 2010).
The correlation results revealed that the dfALFF and MMSE negatively correlated, indicating that the over-high temporal variability in SMA might affect the precise and stable execution of higher-order motor and cognitive processes, further inducing cognition decline.
The present study had several limitations. Firstly, the sample size was small, and all participants came from a single dataset. Studies involving larger samples or another independent dataset are required in the future to confirm our findings. Secondly, the present study compared only the differences between patients with right/left flipped LTLE and HCs, but the differences among patients with LTLE and RTLE and HCs were not analyzed directly due to the small sample size. Previous studies have shown several brain activity measurements’ differences between LTLE and RTLE. Zhang et al. (2010) and Amiri et al. (2020) have separately demonstrated that the utility of ALFF analysis and graph theory application to brain network analysis might be a potential biomarker to assist in the determination of TLE laterality. Pang et al. (Li et al., 2022) found that RTLE patients exhibited more pronounced aberrant connectivity patterns and topological properties revealed by static or dynamic functional connectivity. Consequently, a subgroup analysis is necessary in the future study. Thirdly, further studies on the other IBA indicators, such as FC and ICA, etc., are needed to validate the results, especially the detailed functional connectivity between abnormally activated brain regions and other regions. Fourthly, we did not monitor the numbers or frequency of the epileptic spike during the fMRI scan period simultaneously. And cause some of the IBA indicators might be affected by the epileptic spikes, so the application of simultaneous EEG/fMRI in the future study was important. Fifthly, about the sliding window technique that we have performed, the question about the choice of window width has been constantly discussed, tried and verified. So the application of other dynamic assessment methods (Chen et al., 2017) to verify our results are necessary in the future.
We comprehensively estimated the differences in IBA between patients with TLE and HC using multiple novel static/dynamic indicators, as well as their correlation with cognition. IBA abnormalities in patients with TLE were detected in multiple brain network–related regions, including the temporal lobe epilepsy network (MTL structure and thalamus), sensorimotor network (SMA, PreCG, and PostCG), auditory network (temporal neocortex), and visual network (occipital lobe). The combined application of static and dynamic IBA indicators could comprehensively reveal more real abnormal neuronal activity and the impairment and compensatory mechanisms of cognitive function in TLE. Moreover, it might help in the lateralization of epileptogenic foci and exploration of the transmission and inhibition pathways of epileptic activity.
The studies involving human participants were reviewed and approved by the Research Ethics Committee at The First Affiliated Hospital of Zhengzhou University. Written informed consent to participate in this study was provided by the participants’ legal guardian/next of kin.
CS wrote the first draft of the manuscript. CS and XNZ performed the data and statistical analysis. SH provided the methodological advice. YL provided the clinical diagnosis of the participants. CS, XNZ, KM, KW, and XM collected the data. XCZ and JZ provided the technical advice and proofread the manuscript. JC and YZ contributed to the design of the study and proofread the manuscript. All authors have read and agreed to the published version of the manuscript.
This research study was supported by The First Affiliated Hospital of Zhengzhou University.
We thank all participants who participate in this study.
XCZ and JZ were employed by Siemens Healthcare Ltd.
The remaining authors declare that the research was conducted in the absence of any commercial or financial relationships that could be construed as a potential conflict of interest.
All claims expressed in this article are solely those of the authors and do not necessarily represent those of their affiliated organizations, or those of the publisher, the editors and the reviewers. Any product that may be evaluated in this article, or claim that may be made by its manufacturer, is not guaranteed or endorsed by the publisher.
Alessio, A., Pereira, F. R., Sercheli, M. S., Rondina, J. M., Ozelo, H. B., Bilevicius, E., et al. (2013). Brain plasticity for verbal and visual memories in patients with mesial temporal lobe epilepsy and hippocampal sclerosis: An fMRI study. Hum. Brain Mapp. 34, 186–199. doi: 10.1002/hbm.21432
Allone, C., Lo Buono, V., Corallo, F., Pisani, L. R., Pollicino, P., Bramanti, P., et al. (2017). Neuroimaging and cognitive functions in temporal lobe epilepsy: A review of the literature. J. Neurol. Sci. 381, 7–15. doi: 10.1016/j.jns.2017.08.007
Amiri, S., Mehvari-Habibabadi, J., Mohammadi-Mobarakeh, N., Hashemi-Fesharaki, S. S., Mirbagheri, M. M., Elisevich, K., et al. (2020). Graph theory application with functional connectivity to distinguish left from right temporal lobe epilepsy. Epilepsy Res. 167:106449. doi: 10.1016/j.eplepsyres.2020.106449
Araújo, D., Santos, A. C., Velasco, T. R., Wichert-Ana, L., Terra-Bustamante, V. C., and Alexandre, V. Jr., et al. (2006). Volumetric evidence of bilateral damage in unilateral mesial temporal lobe epilepsy. Epilepsia. 47, 1354–1359. doi: 10.1111/j.1528-1167.2006.00605.x
Beckmann, C. F., DeLuca, M., Devlin, J. T., and Smith, S. M. (2005). Investigations into resting-state connectivity using independent component analysis. Philos. Trans. R. Soc. Lond. B Biol. Sci. 360, 1001–1013. doi: 10.1098/rstb.2005.1634
Behrens, T. E., Johansen-Berg, H., Woolrich, M. W., Smith, S. M., Wheeler-Kingshott, C. A., Boulby, P. A., et al. (2003). Non-invasive mapping of connections between human thalamus and cortex using diffusion imaging. Nat. Neurosci. 6, 750–757. doi: 10.1038/nn1075
Bernasconi, N., Bernasconi, A., Caramanos, Z., Antel, S. B., Andermann, F., and Arnold, D. L. (2003). Mesial temporal damage in temporal lobe epilepsy: A volumetric MRI study of the hippocampus, amygdala and parahippocampal region. Brain 126(Pt 2), 462–469. doi: 10.1093/brain/awg034
Bettus, G., Wendling, F., Guye, M., Valton, L., Régis, J., Chauvel, P., et al. (2008). Enhanced EEG functional connectivity in mesial temporal lobe epilepsy. Epilepsy Res. 81, 58–68. doi: 10.1016/j.eplepsyres.2008.04.020
Biswal, B., Yetkin, F. Z., Haughton, V. M., and Hyde, J. S. (1995). Functional connectivity in the motor cortex of resting human brain using echo-planar MRI. Magn. Reson. Med. 34, 537–541. doi: 10.1002/mrm.1910340409
Blumenfeld, H., McNally, K. A., Vanderhill, S. D., Paige, A. L., Chung, R., Davis, K., et al. (2004). Positive and negative network correlations in temporal lobe epilepsy. Cereb. Cortex 14, 892–902. doi: 10.1093/cercor/bhh048
Blumenfeld, H., and Taylor, J. (2003). Why do seizures cause loss of consciousness? Neuroscientist 9, 301–310. doi: 10.1177/1073858403255624
Blumenfeld, H., Varghese, G. I., Purcaro, M. J., Motelow, J. E., Enev, M., McNally, K. A., et al. (2009). Cortical and subcortical networks in human secondarily generalized tonic-clonic seizures. Brain 132(Pt 4), 999–1012. doi: 10.1093/brain/awp028
Burianová, H., Faizo, N. L., Gray, M., Hocking, J., Galloway, G., and Reutens, D. (2017). Altered functional connectivity in mesial temporal lobe epilepsy. Epilepsy Res. 137, 45–52. doi: 10.1016/j.eplepsyres.2017.09.001
Burwell, R. D. (2000). The parahippocampal region: Corticocortical connectivity. Ann. N. Y. Acad. Sci. 911, 25–42. doi: 10.1111/j.1749-6632.2000.tb06717.x
Bush, G. (2011). Cingulate, frontal, and parietal cortical dysfunction in attention-deficit/hyperactivity disorder. Biol. Psychiatry 69, 1160–1167. doi: 10.1016/j.biopsych.2011.01.022
Cataldi, M., Avoli, M., and de Villers-Sidani, E. (2013). Resting state networks in temporal lobe epilepsy. Epilepsia 54, 2048–2059. doi: 10.1111/epi.12400
Chao-Gan, Y., and Yu-Feng, Z. (2010). DPARSF: A MATLAB toolbox for “Pipeline” data analysis of resting-state fMRI. Front. Syst. Neurosci. 4:13. doi: 10.3389/fnsys.2010.00013
Chen, J. E., Rubinov, M., and Chang, C. (2017). Methods and considerations for dynamic analysis of functional MR imaging data. Neuroimaging Clin. N. Am. 27, 547–560. doi: 10.1016/j.nic.2017.06.009
Concha, L., Beaulieu, C., and Gross, D. W. (2005). Bilateral limbic diffusion abnormalities in unilateral temporal lobe epilepsy. Ann. Neurol. 57, 188–196. doi: 10.1002/ana.20334
Conway, M. A. (2001). Sensory-perceptual episodic memory and its context: Autobiographical memory. Philos. Trans. R. Soc. Lond. B Biol. Sci. 356, 1375–1384. doi: 10.1098/rstb.2001.0940
Dien, J., Brian, E. S., Molfese, D. L., and Gold, B. T. (2013). Combined ERP/fMRI evidence for early word recognition effects in the posterior inferior temporal gyrus. Cortex 49, 2307–2321. doi: 10.1016/j.cortex.2013.03.008
Engel, J. Jr. (2001). International League Against Epilepsy (ILAE). A proposed diagnostic scheme for people with epileptic seizures and with epilepsy: Report of the ILAE Task Force on Classification and Terminology. Epilepsia 42, 796–803. doi: 10.1046/j.1528-1157.2001.10401.x
Fletcher, P. C., Frith, C. D., Grasby, P. M., Shallice, T., Frackowiak, R. S., and Dolan, R. J. (1995). Brain systems for encoding and retrieval of auditory-verbal memory. An in vivo study in humans. Brain 118(Pt 2), 401–416. doi: 10.1093/brain/118.2.401
Fox, M. D., and Raichle, M. E. (2007). Spontaneous fluctuations in brain activity observed with functional magnetic resonance imaging. Nat. Rev. Neurosci. 8, 700–711. doi: 10.1038/nrn2201
Gao, Y. J., Wang, X., Xiong, P. G., Ren, H. W., Zhou, S. Y., Yan, Y. G., et al. (2021). Abnormalities of the default-mode network homogeneity and executive dysfunction in people with first-episode, treatment-naive left temporal lobe epilepsy. Eur. Rev. Med. Pharmacol. Sci. 25, 2039–2049. doi: 10.26355/eurrev_202102_25108
Gonzalez-Castillo, J., Hoy, C. W., Handwerker, D. A., Robinson, M. E., Buchanan, L. C., Saad, Z. S., et al. (2015). Tracking ongoing cognition in individuals using brief, whole-brain functional connectivity patterns. Proc. Natl. Acad. Sci. U.S.A. 112, 8762–8767. doi: 10.1073/pnas.1501242112
Guedj, E., Bonini, F., Gavaret, M., Trébuchon, A., Aubert, S., Boucekine, M., et al. (2015). 18FDG-PET in different subtypes of temporal lobe epilepsy: SEEG validation and predictive value. Epilepsia 56, 414–421. doi: 10.1111/epi.12917
Hermann, B. P., Seidenberg, M., Haltiner, A., and Wyler, A. R. (1991). Mood state in unilateral temporal lobe epilepsy. Biol. Psychiatry 30, 1205–1218. doi: 10.1016/0006-3223(91)90157-h
Hindriks, R., Adhikari, M. H., Murayama, Y., Ganzetti, M., Mantini, D., Logothetis, N. K., et al. (2016). Corrigendum to “Can sliding-window correlations reveal dynamic functional connectivity in resting-state fMRI?” [NeuroImage 127 (2016) 242-256]. Neuroimage 132:115. doi: 10.1016/j.neuroimage.2016.02.007
Holmes, M., Folley, B. S., Sonmezturk, H. H., Gore, J. C., Kang, H., Abou-Khalil, B., et al. (2014). Resting state functional connectivity of the hippocampus associated with neurocognitive function in left temporal lobe epilepsy. Hum. Brain Mapp. 35, 735–744. doi: 10.1002/hbm.22210
Ishai, A., Haxby, J. V., and Ungerleider, L. G. (2002). Visual imagery of famous faces: Effects of memory and attention revealed by fMRI. Neuroimage 17, 1729–1741. doi: 10.1006/nimg.2002.1330
Jiang, L. W., Qian, R. B., Fu, X. M., Zhang, D., Peng, N., Niu, C. S., et al. (2018). Altered attention networks and DMN in refractory epilepsy: A resting-state functional and causal connectivity study. Epilepsy Behav. 88, 81–86. doi: 10.1016/j.yebeh.2018.06.045
Jiang, S., Luo, C., Huang, Y., Li, Z., Chen, Y., Li, X., et al. (2020). Altered static and dynamic spontaneous neural activity in drug-naïve and drug-receiving benign childhood epilepsy with centrotemporal spikes. Front. Hum. Neurosci. 14:361. doi: 10.3389/fnhum.2020.00361
Kalaska, J. F. (2009). From intention to action: Motor cortex and the control of reaching movements. Adv. Exp. Med. Biol. 629, 139–178. doi: 10.1007/978-0-387-77064-2_8
Keezer, M. R., Sisodiya, S. M., and Sander, J. W. (2016). Comorbidities of epilepsy: Current concepts and future perspectives [published correction appears in Lancet Neurol. 2016 Jan;15(1):28]. Lancet Neurol. 15, 106–115. doi: 10.1016/S1474-4422(15)00225-2
Kiehl, K. A., Smith, A. M., Mendrek, A., Forster, B. B., Hare, R. D., and Liddle, P. F. (2004). Temporal lobe abnormalities in semantic processing by criminal psychopaths as revealed by functional magnetic resonance imaging. Psychiatry Res. 130, 297–312. doi: 10.1016/j.pscychresns.2004.02.002
Laufs, H., Rodionov, R., Thornton, R., Duncan, J. S., Lemieux, L., and Tagliazucchi, E. (2014). Altered FMRI connectivity dynamics in temporal lobe epilepsy might explain seizure semiology. Front. Neurol. 5:175. doi: 10.3389/fneur.2014.00175
Li, H., Ding, F., Chen, C., Huang, P., Xu, J., Chen, Z., et al. (2022). Dynamic functional connectivity in modular organization of the hippocampal network marks memory phenotypes in temporal lobe epilepsy. Hum. Brain Mapp. 43, 1917–1929. doi: 10.1002/hbm.25763
Li, R., Hu, C., Wang, L., Liu, D., Liu, D., Liao, W., et al. (2020). Disruption of functional connectivity among subcortical arousal system and cortical networks in temporal lobe epilepsy. Brain Imaging Behav. 14, 762–771. doi: 10.1007/s11682-018-0014-y
Liang, X., Pang, X., Liu, J., Zhao, J., Yu, L., and Zheng, J. (2020). Comparison of topological properties of functional brain networks with graph theory in temporal lobe epilepsy with different duration of disease. Ann. Transl. Med. 8:1503. doi: 10.21037/atm-20-6823
Liao, W., Li, J., Ji, G. J., Wu, G. R., Long, Z., Xu, Q., et al. (2019). Endless fluctuations: Temporal dynamics of the amplitude of low frequency fluctuations. IEEE Trans. Med. Imaging 38, 2523–2532. doi: 10.1109/TMI.2019.2904555
Liao, W., Wu, G. R., Xu, Q., Ji, G. J., Zhang, Z., Zang, Y. F., et al. (2014). DynamicBC: A MATLAB toolbox for dynamic brain connectome analysis. Brain Connect. 4, 780–790. doi: 10.1089/brain.2014.0253
Logothetis, N. K., Pauls, J., Augath, M., Trinath, T., and Oeltermann, A. (2001). Neurophysiological investigation of the basis of the fMRI signal. Nature 412, 150–157. doi: 10.1038/35084005
Lu, B., Chen, X., Li, L., Shen, Y. Q., Chen, N. X., Mei, T., et al. (2018). Aberrant dynamics of spontaneous brain activity and its integration in patients with autism spectrum disorder. Chin. Sci. Bull. 63, 1452–1463.
Maneshi, M., Vahdat, S., Fahoum, F., Grova, C., and Gotman, J. (2014). Specific resting-state brain networks in mesial temporal lobe epilepsy. Front. Neurol. 5:127. doi: 10.3389/fneur.2014.00127
Margulies, D. S., Böttger, J., Long, X., Lv, Y., Kelly, C., Schäfer, A., et al. (2010). Resting developments: A review of fMRI post-processing methodologies for spontaneous brain activity. MAGMA 23, 289–307. doi: 10.1007/s10334-010-0228-5
Mechelli, A., Humphreys, G. W., Mayall, K., Olson, A., and Price, C. J. (2000). Differential effects of word length and visual contrast in the fusiform and lingual gyri during reading. Proc. Biol. Sci. 267, 1909–1913. doi: 10.1098/rspb.2000.1229
Michelon, P., Vettel, J. M., and Zacks, J. M. (2006). Lateral somatotopic organization during imagined and prepared movements. J. Neurophysiol. 95, 811–822. doi: 10.1152/jn.00488.2005
Morgan, V. L., Chang, C., Englot, D. J., and Rogers, B. P. (2020). Temporal lobe epilepsy alters spatio-temporal dynamics of the hippocampal functional network. Neuroimage Clin. 26:102254. doi: 10.1016/j.nicl.2020.102254
Nakai, Y., Nishibayashi, H., Donishi, T., Terada, M., Nakao, N., and Kaneoke, Y. (2021). Regional abnormality of functional connectivity is associated with clinical manifestations in individuals with intractable focal epilepsy. Sci. Rep. 11:1545. doi: 10.1038/s41598-021-81207-6
Norden, A. D., and Blumenfeld, H. (2002). The role of subcortical structures in human epilepsy. Epilepsy Behav. 3, 219–231. doi: 10.1016/s1525-5050(02)00029-x
Olson, I. R., Plotzker, A., and Ezzyat, Y. (2007). The Enigmatic temporal pole: A review of findings on social and emotional processing. Brain 130(Pt 7), 1718–1731. doi: 10.1093/brain/awm052
O’Muircheartaigh, J., Vollmar, C., Barker, G. J., Kumari, V., Symms, M. R., Thompson, P., et al. (2012). Abnormal thalamocortical structural and functional connectivity in juvenile myoclonic epilepsy. Brain 135(Pt 12), 3635–3644. doi: 10.1093/brain/aws296
Pang, X., Liang, X., Zhao, J., Wu, P., Li, X., Wei, W., et al. (2022). Abnormal static and dynamic functional connectivity in left and right temporal lobe epilepsy. Front. Neurosci. 15:820641. doi: 10.3389/fnins.2021.820641
Park, H. J., Friston, K. J., Pae, C., Park, B., and Razi, A. (2018). Dynamic effective connectivity in resting state fMRI. Neuroimage 180(Pt B), 594–608. doi: 10.1016/j.neuroimage.2017.11.033
Picard, N., and Strick, P. L. (1996). Motor areas of the medial wall: A review of their location and functional activation. Cereb. Cortex 6, 342–353. doi: 10.1093/cercor/6.3.342
Power, J. D., Barnes, K. A., Snyder, A. Z., Schlaggar, B. L., and Petersen, S. E. (2012). Spurious but systematic correlations in functional connectivity MRI networks arise from subject motion. Neuroimage 59, 2142–2154. doi: 10.1016/j.neuroimage.2011.10.018
Power, J. D., Plitt, M., Laumann, T. O., and Martin, A. (2017). Sources and implications of whole-brain fMRI signals in humans. Neuroimage 146, 609–625. doi: 10.1016/j.neuroimage.2016.09.038
Raichle, M. E. (2010). Two views of brain function. Trends Cogn. Sci. 14, 180–190. doi: 10.1016/j.tics.2010.01.008
Raichle, M. E. (2015). The restless brain: How intrinsic activity organizes brain function. Philos. Trans. R. Soc. Lond. B Biol. Sci. 370:20140172. doi: 10.1098/rstb.2014.0172
Reyes, A., Thesen, T., Wang, X., Hahn, D., Yoo, D., Kuzniecky, R., et al. (2016). Resting-state functional MRI distinguishes temporal lobe epilepsy subtypes. Epilepsia 57, 1475–1484. doi: 10.1111/epi.13456
Rosch, K. S., and Mostofsky, S. (2019). Development of the frontal lobe. Handb. Clin. Neurol. 163, 351–367. doi: 10.1016/B978-0-12-804281-6.00019-7
Rostomily, R. C., Berger, M. S., Ojemann, G. A., and Lettich, E. (1991). Postoperative deficits and functional recovery following removal of tumors involving the dominant hemisphere supplementary motor area. J. Neurosurg. 75, 62–68. doi: 10.3171/jns.1991.75.1.0062
Rudebeck, P. H., and Rich, E. L. (2018). Orbitofrontal cortex. Curr. Biol. 28, R1083–R1088. doi: 10.1016/j.cub.2018.07.018
Sakoğlu, U., Pearlson, G. D., Kiehl, K. A., Wang, Y. M., Michael, A. M., and Calhoun, V. D. (2010). A method for evaluating dynamic functional network connectivity and task-modulation: Application to schizophrenia. MAGMA 23, 351–366. doi: 10.1007/s10334-010-0197-8
Schacher, M., Winkler, R., Grunwald, T., Kraemer, G., Kurthen, M., Reed, V., et al. (2006). Mesial temporal lobe epilepsy impairs advanced social cognition. Epilepsia 47, 2141–2146. doi: 10.1111/j.1528-1167.2006.00857.x
Schwartz, T. H., and Bonhoeffer, T. (2001). In vivo optical mapping of epileptic foci and surround inhibition in ferret cerebral cortex. Nat. Med. 7, 1063–1067. doi: 10.1038/nm0901-1063
Shi, K., Pang, X., Wang, Y., Li, C., Long, Q., and Zheng, J. (2021). Altered interhemispheric functional homotopy and connectivity in temporal lobe epilepsy based on fMRI and multivariate pattern analysis. Neuroradiology 63, 1873–1882. doi: 10.1007/s00234-021-02706-x
Singh, T. B., Aisikaer, A., He, C., Wu, Y., Chen, H., Ni, H., et al. (2020). The assessment of brain functional changes in the temporal lobe epilepsy patient with cognitive impairment by resting-state functional magnetic resonance imaging. J. Clin. Imaging Sci. 10:50. doi: 10.25259/JCIS_55_2020
Spencer, S. S. (2002). Neural networks in human epilepsy: Evidence of and implications for treatment. Epilepsia 43, 219–227. doi: 10.1046/j.1528-1157.2002.26901.x
Thijs, R. D., Surges, R., O’Brien, T. J., and Sander, J. W. (2019). Epilepsy in adults. Lancet 393, 689–701. doi: 10.1016/S0140-6736(18)32596-0
Thompson, G. J., Magnuson, M. E., Merritt, M. D., Schwarb, H., Pan, W. J., McKinley, A., et al. (2013). Short-time windows of correlation between large-scale functional brain networks predict vigilance intraindividually and interindividually. Hum. Brain Mapp. 34, 3280–3298. doi: 10.1002/hbm.22140
Trimmel, K., van Graan, A. L., Caciagli, L., Haag, A., Koepp, M. J., Thompson, P. J., et al. (2018). Left temporal lobe language network connectivity in temporal lobe epilepsy. Brain 141, 2406–2418. doi: 10.1093/brain/awy164
Tuchscherer, V., Seidenberg, M., Pulsipher, D., Lancaster, M., Guidotti, L., and Hermann, B. (2010). Extrahippocampal integrity in temporal lobe epilepsy and cognition: Thalamus and executive functioning. Epilepsy Behav. 17, 478–482. doi: 10.1016/j.yebeh.2010.01.019
Van Paesschen, W., Dupont, P., Van Driel, G., Van Billoen, H., and Maes, A. (2003). SPECT perfusion changes during complex partial seizures in patients with hippocampal sclerosis. Brain 126(Pt 5), 1103–1111. doi: 10.1093/brain/awg108
Vaughan, D. N., Rayner, G., Tailby, C., and Jackson, G. D. (2016). MRI-negative temporal lobe epilepsy: A network disorder of neocortical connectivity. Neurology 87, 1934–1942. doi: 10.1212/WNL.0000000000003289
Viard, A., Piolino, P., Desgranges, B., Chételat, G., Lebreton, K., Landeau, B., et al. (2007). Hippocampal activation for autobiographical memories over the entire lifetime in healthy aged subjects: An fMRI study. Cereb. Cortex 17, 2453–2467. doi: 10.1093/cercor/bhl153
Wang, K., Zhang, X., Song, C., Ma, K., Bai, M., Zheng, R., et al. (2021). Decreased intrinsic neural timescales in mesial temporal lobe epilepsy. Front. Hum. Neurosci. 15:772365. doi: 10.3389/fnhum.2021.772365
Xia, M., Wang, J., and He, Y. (2013). BrainNet viewer: A network visualization tool for human brain connectomics. PLoS One 8:e68910. doi: 10.1371/journal.pone.0068910
Xu, Q., Zhang, Z., Liao, W., Xiang, L., Yang, F., Wang, Z., et al. (2014). Time-shift homotopic connectivity in mesial temporal lobe epilepsy. AJNR Am. J. Neuroradiol. 35, 1746–1752. doi: 10.3174/ajnr.A3934
Yan, C. G., Wang, X. D., Zuo, X. N., and Zang, Y. F. D. P. A. B. I. (2016). Data processing & analysis for (resting-state) brain imaging. Neuroinformatics 14, 339–351. doi: 10.1007/s12021-016-9299-4
Yan, C. G., Yang, Z., Colcombe, S. J., Zuo, X. N., and Milham, P. (2017). Concordance among indices of intrinsic brain function:Insights from inter individual variation and temporal dynamics. Sci. Bull. 62, 1572–1584. doi: 10.1016/j.scib.2017.09.015
Yang, H., Long, X. Y., Yang, Y., Yan, H., Zhu, C. Z., Zhou, X. P., et al. (2007). Amplitude of low frequency fluctuation within visual areas revealed by resting-state functional MRI. Neuroimage 36, 144–152. doi: 10.1016/j.neuroimage.2007.01.054
Yang, H., Zhang, C., Liu, C., Yu, T., Zhang, G., Chen, N., et al. (2018). Brain network alteration in patients with temporal lobe epilepsy with cognitive impairment. Epilepsy Behav. 81, 41–48. doi: 10.1016/j.yebeh.2018.01.024
Zang, Y., Jiang, T., Lu, Y., He, Y., and Tian, L. (2004). Regional homogeneity approach to fMRI data analysis. Neuroimage 22, 394–400. doi: 10.1016/j.neuroimage.2003.12.030
Zang, Y. F., He, Y., Zhu, C. Z., Cao, Q. J., Sui, M. Q., Liang, M., et al. (2007). Altered baseline brain activity in children with ADHD revealed by resting-state functional MRI [published correction appears in Brain Dev. 2012 Apr;34(4):336]. Brain Dev. 29, 83–91. doi: 10.1016/j.braindev.2006.07.002
Zeng, H., Pizarro, R., Nair, V. A., La, C., and Prabhakaran, V. (2013). Alterations in regional homogeneity of resting-state brain activity in mesial temporal lobe epilepsy. Epilepsia 54, 658–666. doi: 10.1111/epi.12066
Zhang, Z., Lu, G., Zhong, Y., Tan, Q., Chen, H., Liao, W., et al. (2010). fMRI study of mesial temporal lobe epilepsy using amplitude of low-frequency fluctuation analysis. Hum. Brain Mapp. 31, 1851–1861. doi: 10.1002/hbm.20982
Zhang, Z., Lu, G., Zhong, Y., Tan, Q., Liao, W., Chen, Z., et al. (2009). Impaired perceptual networks in temporal lobe epilepsy revealed by resting fMRI. J. Neurol. 256, 1705–1713. doi: 10.1007/s00415-009-5187-2
Zhao, B., Yang, B., Tan, Z., Hu, W., Sang, L., Zhang, C., et al. (2020). Intrinsic brain activity changes in temporal lobe epilepsy patients revealed by regional homogeneity analysis. Seizure 81, 117–122. doi: 10.1016/j.seizure.2020.07.030
Zou, Q. H., Zhu, C. Z., Yang, Y., Zuo, X. N., Long, X. Y., Cao, Q. J., et al. (2008). An improved approach to detection of amplitude of low-frequency fluctuation (ALFF) for resting-state fMRI: Fractional ALFF. J. Neurosci. Methods 172, 137–141. doi: 10.1016/j.jneumeth.2008.04.012
Zuo, X. N., Ehmke, R., Mennes, M., Imperati, D., Castellanos, F. X., Sporns, O., et al. (2012). Network centrality in the human functional connectome. Cereb. Cortex 22, 1862–1875. doi: 10.1093/cercor/bhr269
Keywords: cognition, dynamic, intrinsic brain activity, resting-state functional magnetic resonance imaging, temporal lobe epilepsy
Citation: Song C, Zhang X, Han S, Ma K, Wang K, Mao X, Lian Y, Zhang X, Zhu J, Zhang Y and Cheng J (2022) More than just statics: Static and temporal dynamic changes in intrinsic brain activity in unilateral temporal lobe epilepsy. Front. Hum. Neurosci. 16:971062. doi: 10.3389/fnhum.2022.971062
Received: 16 June 2022; Accepted: 01 August 2022;
Published: 31 August 2022.
Edited by:
Tamer Demiralp, Istanbul University, TurkeyReviewed by:
Masoud Seraji, University of Texas at Austin, United StatesCopyright © 2022 Song, Zhang, Han, Ma, Wang, Mao, Lian, Zhang, Zhu, Zhang and Cheng. This is an open-access article distributed under the terms of the Creative Commons Attribution License (CC BY). The use, distribution or reproduction in other forums is permitted, provided the original author(s) and the copyright owner(s) are credited and that the original publication in this journal is cited, in accordance with accepted academic practice. No use, distribution or reproduction is permitted which does not comply with these terms.
*Correspondence: Jingliang Cheng, ZmNjY2hlbmdqbEB6enUuZWR1LmNu; Yong Zhang, enp1emhhbmd5b25nMjAxM0AxNjMuY29t
†These authors have contributed equally to this work
Disclaimer: All claims expressed in this article are solely those of the authors and do not necessarily represent those of their affiliated organizations, or those of the publisher, the editors and the reviewers. Any product that may be evaluated in this article or claim that may be made by its manufacturer is not guaranteed or endorsed by the publisher.
Research integrity at Frontiers
Learn more about the work of our research integrity team to safeguard the quality of each article we publish.