- 1Laboratory for Cognition and Neural Stimulation, Department of Neurology, University of Pennsylvania, Philadelphia, PA, United States
- 2Moss Rehabilitation Research Institute, Elkins Park, PA, United States
- 3Department of Neurology, University of Pennsylvania, Philadelphia, PA, United States
- 4Penn Frontotemporal Degeneration Center, University of Pennsylvania, Philadelphia, PA, United States
- 5Department of Biostatistics, Epidemiology and Informatics, University of Pennsylvania, Philadelphia, PA, United States
Objectives: We hypothesized that measures of cortical thickness and volume in language areas would correlate with response to treatment with high-definition transcranial direct current stimulation (HD-tDCS) in persons with primary progressive aphasia (PPA).
Materials and Methods: In a blinded, within-group crossover study, PPA patients (N = 12) underwent a 2-week intervention HD-tDCS paired with constraint-induced language therapy (CILT). Multi-level linear regression (backward-fitted models) were performed to assess cortical measures as predictors of tDCS-induced naming improvements, measured by the Western Aphasia Battery-naming subtest, from baseline to immediately after and 6 weeks post-intervention.
Results: Greater baseline thickness of the pars opercularis significantly predicted naming gains (p = 0.03) immediately following intervention, while greater thickness of the middle temporal gyrus (MTG) and lower thickness of the superior temporal gyrus (STG) significantly predicted 6-week naming gains (p’s < 0.02). Thickness did not predict naming gains in sham. Volume did not predict immediate gains for active stimulation. Greater volume of the pars triangularis and MTG, but lower STG volume significantly predicted 6-week naming gains in active stimulation. Greater pars orbitalis and MTG volume, and lower STG volume predicted immediate naming gains in sham (p’s < 0.05). Volume did not predict 6-week naming gains in sham.
Conclusion: Cortical thickness and volume were predictive of tDCS-induced naming improvement in PPA patients. The finding that frontal thickness predicted immediate active tDCS-induced naming gains while temporal areas predicted naming changes at 6-week suggests that a broader network of regions may be important for long-term maintenance of treatment gains. The finding that volume predicted immediate naming performance in the sham condition may reflect the benefits of behavioral speech language therapy and neural correlates of its short-lived treatment gains. Collectively, thickness and volume were predictive of treatment gains in the active condition but not sham, suggesting that pairing HD-tDCS with CILT may be important for maintaining treatment effects.
Introduction
Primary progressive aphasia (PPA) is a debilitating syndrome marked by progressive loss of language skills resulting from underlying neurodegenerative diseases. Unfortunately, speech language therapy (SLT), the current standard-of-care, is only modestly beneficial (Beeson et al., 2011; Tippett et al., 2015; Gervits et al., 2017). A growing body of evidence suggests that transcranial direct current stimulation (tDCS), a form of non-invasive neuromodulation, can enhance language outcomes in persons with PPA when paired with SLT (Tsapkini et al., 2014; Gervits et al., 2017; Fenner et al., 2019; Cotelli et al., 2020; Nissim et al., 2020). TDCS involves the delivery of weak electrical currents via electrodes placed on the scalp (Nitsche and Paulus, 2000), which alter the resting membrane potential of neurons and enable the modulation of neuronal excitability (facilitation or suppression) of targeted cortical regions (Nitsche and Paulus, 2000; Nitsche et al., 2005; Vecchio et al., 2016). TDCS has shown promise in facilitating behaviorally beneficial neuroplasticity when targeting a brain region responsible for a particular cognitive process, particularly when paired with a task that engages the targeted brain system (Gill et al., 2015; Pellicciari and Miniussi, 2018; Dubreuil-Vall et al., 2019; Kronberg et al., 2020). However, responses to tDCS vary considerably, which limits its advancement as a potential intervention for persons with PPA. The causes of this variability remain poorly understood (Datta et al., 2012; Horvath et al., 2014; Tsapkini et al., 2014), in part because characteristics that predict responsiveness to tDCS treatment in PPA patients are relatively unexplored.
A pathological hallmark of PPA is cortical atrophy in key regions of the brain’s language network (Mesulam, 2003; Sreepadma et al., 2003; Gorno-Tempini et al., 2011; Rogalski et al., 2011; Norise and Hamilton, 2017). Different patterns of cortical atrophy are observed in the three known subtypes of PPA. Atrophy is observed in the left inferior frontal lobe and insula in persons with non-fluent/agrammatic PPA (naPPA), who experience difficulties with language composition at the level of words and grammar. Individuals with logopenic variant PPA (lvPPA) show atrophy of the left posterior temporal and parietal lobes, and experience difficulties in lexical retrieval and phonological loop functions. Semantic variant PPA (svPPA) involves anterior and ventral temporal lobe atrophy that account for deficits in processing conceptual information (Grossman and Ash, 2004; Grossman, 2012, 2018; Agosta et al., 2013; Giannini et al., 2017).
A gap in knowledge exists in understanding the contribution of both cortical thickness and volume atrophy and its relationship to tDCS-related language gains in PPA. Here, we are interested in exploring whether cortical thickness and/or volume across PPA subtypes may be predictive of tDCS-induced language gains. We operationalize cortical volume and thickness, where the former is defined as the amount of gray matter that lies between the gray-white boundary and the pia mater and the latter as the width of gray matter structure (i.e., cortical ribbon), respectively (Winkler et al., 2010; Nissim et al., 2017). Volume is quantified by both thickness and surface area and represents of the amount and size of neurons and dendritic processes (Makris et al., 2007; Dickerson and Wolk, 2012). Thickness serves as a marker of the integrity of the cerebral cortex and relates to the size, number, and density of cells in a cortical column (Rakic, 1988). Numerous studies have shown that cortical thickness is an important parameter related with cognitive abilities (Engvig et al., 2010; McGugin et al., 2016; Schmidt et al., 2016), and thinner cortex is a reliable index of atrophy in neurodegenerative diseases (Dickerson et al., 2009). Yet, to our knowledge, prior research in PPA has largely focused on cortical volume as a predictor of response to tDCS-induced language gains (Cotelli et al., 2016; de Aguiar et al., 2020). We hypothesized that the degree of cortical thickness and volume atrophy in language-relevant brain regions could strongly influence tDCS treatment response in persons with PPA.
There are at least two reasons to suspect that cortical atrophy could be a strong predictor of tDCS efficacy in persons with PPA. First, because tDCS-induced long-term changes in brain function are thought to be mediated by modulation of synaptic plasticity (Monte-Silva et al., 2013; Rahman et al., 2013; Pelletier and Cicchetti, 2015), they are theoretically dependent on the density of synapses and overall neuronal connectivity in stimulated areas, both of which are markedly reduced in brain regions that have undergone neurodegenerative atrophy (Pini et al., 2016, 2018). Secondly, the amount and distribution of current that reaches brain tissue during tDCS administration may depend on individual anatomy (Kim et al., 2014; Unal et al., 2020). Some computational modeling studies have suggested that cortical atrophy (i.e., decreased gray matter and increased cerebrospinal fluid) reduces the current density that reaches atrophied parts of the brain (Mahdavi and Towhidkhah, 2018), although more recent studies have called this finding into question (Unal et al., 2020). Thus, cortical atrophy may reduce the effects of tDCS, both because atrophied areas contain less substrate to stimulate and likely receive less current than relatively spared brain regions.
The potential of tDCS to elicit adaptive neuroplasticity in patients with aphasia has previously been associated with two possible and not mutually exclusive mechanisms: (1) the activation (or re-activation) of canonical networks that become dysfunctional due to disease pathology; or (2) the recruitment of compensatory networks that engage brain regions previously uninvolved in language processing (Chrysikou and Hamilton, 2011; Cotelli et al., 2016). Current research in persons with PPA supports the notion that tDCS engages pre-existing neural substrates within the damaged language network, as evidence shows that partially spared cortical regions involved in language are essential to confer tDCS-induced language enhancement (Turkeltaub et al., 2011; Norise and Hamilton, 2017). This has been demonstrated in prior PPA studies focusing on naming improvement, where cortical volume predicted conventional tDCS-induced improvements after a written naming and spelling therapy (de Aguiar et al., 2020) and Individualized Computerized Anomia Training (ICAT; Cotelli et al., 2016).
In the current double-blind, within-subject crossover exploratory pilot study, we examined whether baseline cortical thickness (i.e., width of cortical gray matter) and/or volume (i.e., a representation of the amount and size of neurons and dendritic processes) of left hemisphere language regions predict improvements in language abilities after a 2-week intervention of active versus sham high-definition tDCS (HD-tDCS) in PPA patients. Cortical thinning corresponds well with clinical manifestations in PPA (Thanprasertsuk and Likitjaroen, 2021), while cortical volume atrophy has shown associations with lower naming accuracy in PPA patients (Meyer et al., 2017); thus, we were interested in examining the relationship between tDCS-induced naming improvement and cortical measures at baseline. HD-tDCS, which affords increased stimulation focality, and to our knowledge, has not yet been explored in PPA, was paired with constraint induced language therapy (CILT) during ten daily sessions. CILT was selected as it has previously demonstrated to be efficacious in persons with PPA (Hameister et al., 2017). Language abilities were assessed using the Western Aphasia Battery-Revised (WAB-R; Kertesz, 2006) at baseline, immediately after intervention (0-week) and 6-week post-intervention to assess maintenance of tDCS-induced treatment gains. We hypothesized that left hemisphere language regions with greater cortical thickness or volume at baseline would predict language gains at post-intervention time points in the active condition, but not sham, when compared to cortical atrophy of a control site and/or cortical atrophy more generally (i.e., global cortical volume/thickness).
Materials and Methods
Participants
Twelve individuals with PPA (lvPPA = 8, svPPA = 2, naPPA = 2) were included in this study [4 females; mean age = 66.92(±6.37); mean education = 17.17(±1.95)]. Participants were recruited from the Penn Frontotemporal Degeneration Center (FTDC) and were diagnosed according to Gorno-Tempini et al. (2011) guidelines. At the time of enrollment, mean time since diagnosis was 3.42(±1.71) years. Participants were enrolled if they met the following inclusion criteria: right-handedness, native English-speakers, no contraindications to undergoing tDCS or MRI, no other active neurologic conditions, and a Mini Mental Status Examination (MMSE; Folstein et al., 1975) score ≥15 prior to enrollment [mean MMSE = 24.5(±2.88); range = 19–29] to ensure that cognitive impairment did not impede engagement in the study protocol. All participants provided informed consent in accordance with the University of Pennsylvania’s Institutional Review Board (IRB). Table 1 summarizes demographic data.
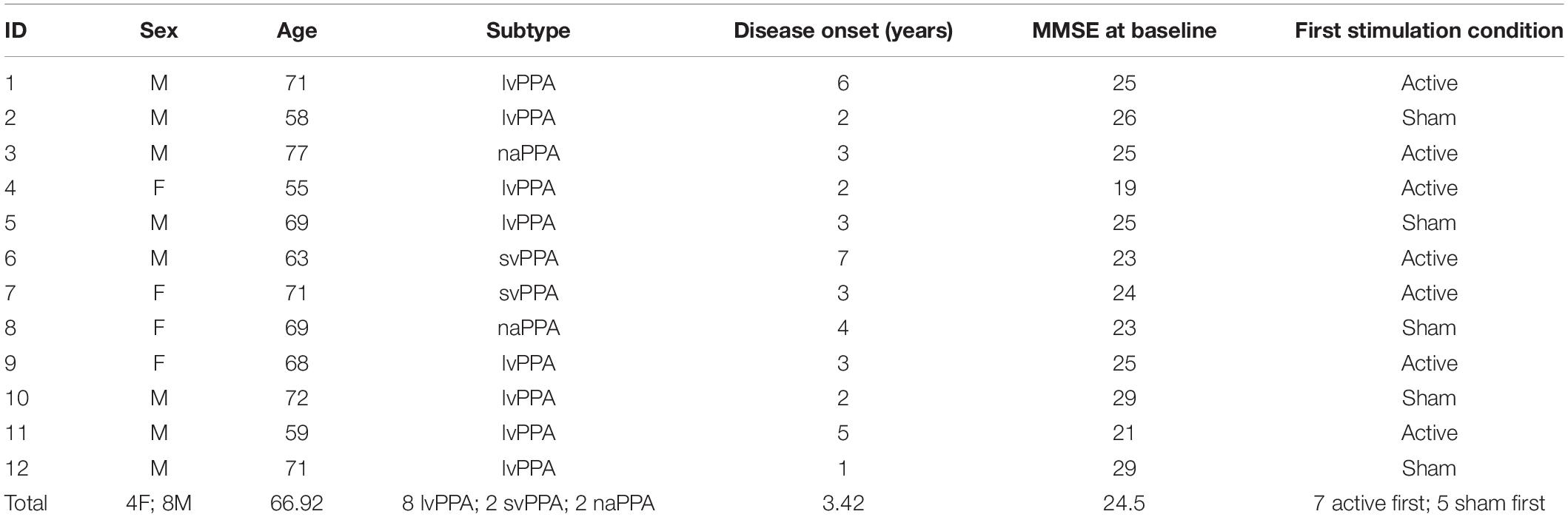
Table 1. Demographics and characteristics of each participant and the total sample (mean age, sex, PPA subtype, years since onset, baseline MMSE score, order of stimulation condition).
Intervention Timeline
Participants were randomly assigned to receive a 10-day course of CILT for 1 h paired with 20 min of active (or sham) HD-tDCS in the first (or second) arm (counterbalanced for stimulation condition). Participants (N = 12) completed the WAB-R prior to each treatment arm and again immediately after (0-week) and 6-week post-intervention. To avoid carryover effects, arm 2 baseline assessment was administered 12-week following arm 1 treatment. One participant (ID#12) was excluded from behavioral analyses due to missing the 6-week time point, but was included in imaging analyses. Both treatment arms followed identical procedures, differing only with respect to the stimulation condition (see Figure 1). The FTDC obtained T1-weighted MRI scans and administered the MMSE within ∼7 months prior to enrollment. At enrollment, the MMSE was readministered. A paired-samples t-test revealed no significant decline in cognitive status from the time of the scan and enrollment in the study [t(11) = −1.43, p = 0.2].

Figure 1. Overview of study design. The color differentiation indicates arm 1 crossover to arm 2 and arm 2 crossover to arm 1.
Neuroimaging Acquisition and Processing
Participants underwent structural neuroimaging on a 3-Tesla Siemens magnetic resonance imaging (MRI) scanner. MRI data for 6 participants were collected with a T1-weighted sagittal MP-RAGE sequence with in-plane voxel sizes of 1.05 mm × 1.05 mm, slice thickness = 1.2 mm, repetition time = 2300 ms, echo time = 2.91 ms, and inversion time = 900 ms. The remaining participants underwent a multi-echo T1-weighted MPRAGE with in-plane voxel sizes of 0.8 mm × 0.8 mm, slice thickness = 0.8 mm, repetition time = 2400 ms, inversion time = 1020 ms, and echo times of 1.96 ms, 3.88 ms, 5.8 ms, and 7.72 ms (Tisdall et al., 2016). This difference in scanner parameters is within an acceptable deviation range (Mulder et al., 2019), and therefore likely does not affect analyses conducted on the full sample. Images were processed using Advanced Normalization Tools (ANTs), which has been extensively validated against surface-based methods such as FreeSurfer (Tustison et al., 2014). Images underwent intensity normalization and were spatially normalized to a healthy control template from the Open Access Series of Imaging Studies (OASIS) dataset using a symmetric diffeomorphic algorithm (Klein et al., 2009; Avants et al., 2011; Phillips et al., 2018). Images were then segmented into six tissue classes using template-based priors: cortical gray matter, deep gray matter, white matter, CSF, brainstem, and cerebellum. Tissue segmentation posteriors were then used to estimate cortical thickness. The Lausanne atlas (scale 60) was aligned to each T1-weighted image and intersected with gray matter probability maps to obtain gray matter volume estimates for each parcellated region. Volume was computed from voxels in each region with a gray matter probability of 50% or higher. Gray matter volumes were normalized by intracranial volume and converted to z-scores based on gray matter volume in control images. All scans were visually inspected for image quality, and outlier analyses were performed (i.e., z-scores > 3.0) to identify extreme values due to segmentation error or other artifacts.
Brain Regions of Interest (ROIs)
To avoid multiple comparison issues, we selected a priori seven regions that exhibit predominant pathology across all PPA subtypes in the frontal and temporal left hemisphere to represent the language network (Mesulam et al., 2014; Thanprasertsuk and Likitjaroen, 2021; Figure 2). This included regions involved in confrontation naming, speech production, comprehension, and general language processing (Ojemann et al., 1988; Kan and Thompson-Schill, 2004; Newhart et al., 2007; MacDonald et al., 2015; Walenski et al., 2019): inferior frontal gyrus (IFG) parcellated into the pars opercularis (IFGop), pars orbitalis (IFGorb), and pars triangularis (IFGtr), middle temporal gyrus (MTG) and superior temporal gyrus parcellated into an anterior and posterior region (aMTG; pMTG; aSTG; pSTG). The left occipital cortex was assessed as a control region (separately for thickness and volume; divided into 5 ROIs).
High-Definition tDCS (HD-tDCS) Parameters
Participants received HD-tDCS (SoterixMedical 1 × 1 low intensity transcranial DC Stimulator and 4 × 1 Multi-Channel Stimulation Interface) at 1.5 mA intensity for 20-min in the active condition with 30 s current ramp-up and -down. Electrodes were placed following the International 10-10 EEG system. The montage targeted the left frontotemporal region (FT7); electrode placement involved a central anode electrode over FT7 and four surrounding cathode electrodes F7, T7, FC5, FT9 (−0.375 mA each) arranged in a ring configuration. Figure 3 displays the predicted current flow and electrode montage. Sham involved a 30 s ramp-up/ramp-down stimulation procedure using the same montage as active (Palm et al., 2013) which enabled blinding of the stimulation condition.
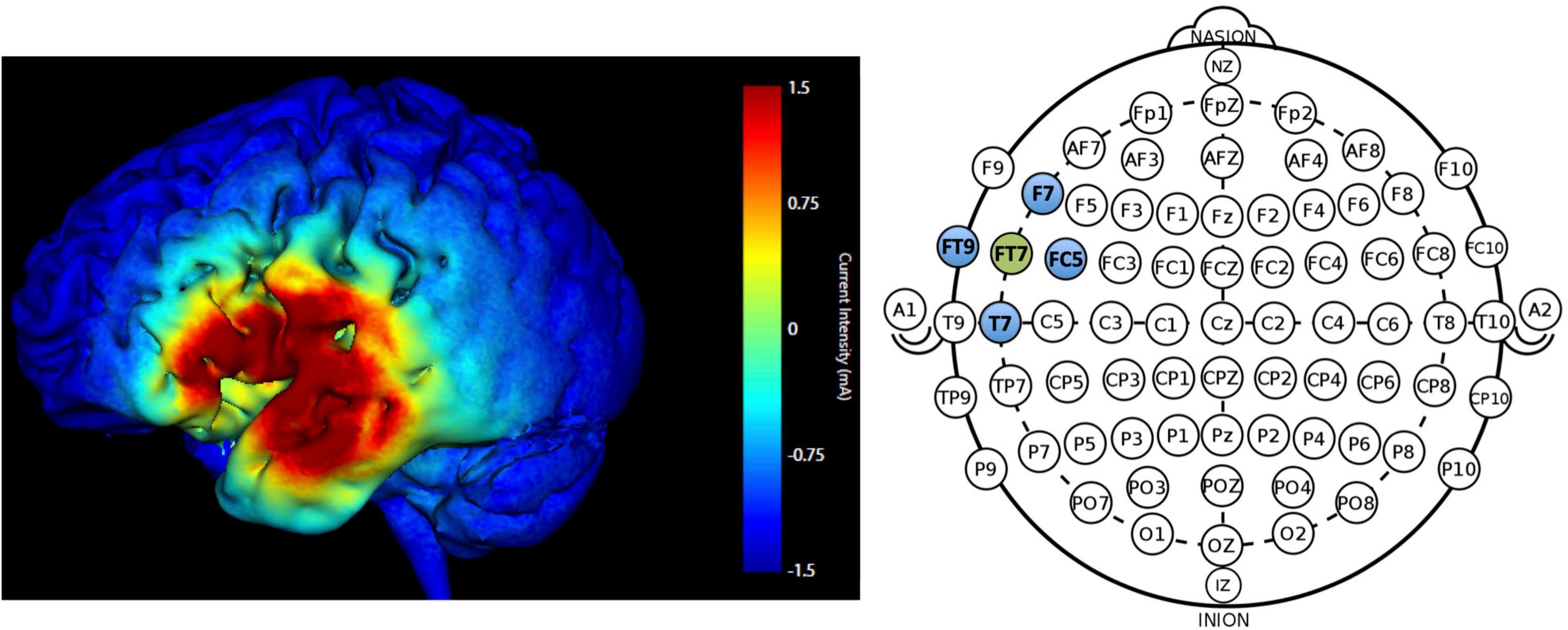
Figure 3. Predicted current modeling simulation at 1.5 mA intensity for electrode placement at FT7 (anode; green) and F7, T7, FC5, FT9 (cathode electrodes; blue). The color legend indicates current intensity (warmer colors = higher current intensity; max = 1.5 mA).
Constraint Induced Language Therapy (CILT)
Constraint induced language therapy is a behavioral SLT that targets speech production by requiring communicative interactions using only spoken output while constraining the use of non-verbal forms of communication, such as gesturing (Maher et al., 2006). Participants used spoken output to request cards depicting everyday objects and actions (Kirmess and Maher, 2010; Ciccone et al., 2015). If unsuccessful, a semantic cue was provided, followed by a phonetic cue, then the correct response. Over the course of therapy, difficulty increased from single noun production (object stimuli) to a single verb response (action stimuli) followed by generating sentences for the object and action stimuli. CILT administration followed prior studies, as described elsewhere (Pulvermüller et al., 2001; Maher et al., 2006).
Western Aphasia Battery-Revised (WAB-R) Parameters
The WAB-R is a widely employed, standard assessment of aphasia characteristics and severity that shows high test-retest reliability (Kertesz, 2006). The WAB Aphasia Quotient (WAB-AQ)–a measure of overall severity–served as a primary outcome measure. WAB subtests were examined separately for spontaneous speech, auditory-verbal comprehension, repetition, and naming. Imaging analyses focused on outcome measures demonstrating stimulation-induced changes from baseline.
Statistical Analyses
Western Aphasia Battery-Revised Behavioral Performance
Repeated-measures analyses of variance (RM-ANOVAs) were conducted on change scores reflecting the difference between baseline to 0- and 6-week post-treatment to eliminate confounds from potential carry over effects on the WAB-AQ and WAB subtests, with tDCS condition (active, sham) and time point (0-week, 6-week) as within-subject factors. An additional analysis was performed with randomization order (first arm: active, sham) as a between-subject factor. Data were analyzed with SPSS v26.
Identifying Cortical Predictors of tDCS Effects
Using a two-step approach, we conducted multiple linear regression analyses to explore (1) the relationship between tDCS-induced language gains and baseline cortical thickness or volume as predictors of treatment response for outcome measures demonstrating significant improvement; and (2) the predictive value of significant ROIs at each time point while accounting for global left hemisphere and whole brain thickness and volume.
Given the exploratory nature of these analyses, we conducted separate backward-stepwise regression analyses including all seven ROIs for each cortical measure (thickness and volume), time point (change from 0- to 6-week), and stimulation condition (active and sham). The backward regression enabled the assessment of all variables in a single model and removed each ROI from subsequent models to identify the best model fit.
To ensure that the findings, were not driven by overall brain atrophy, additional regression analyses utilizing the enter approach were conducted on significant regions alongside covariates in the models (global left hemisphere and whole brain cortical thickness and volume).
Lastly, similar regression analyses were performed on a control region, (i.e., occipital cortex; 5 ROIs total for thickness and volume). Additional control analyses were performed using Bayesian linear regression in JASP v0.16.
Results
Behavior
No significant change in WAB-AQ as a function of tDCS condition was observed [F(1,10) = 2.53, p = 0.14], although there was a numerical increase from mean baseline WAB-AQ to 0-week post-intervention following active [M(±SD) = 83.20(±9.57) to 85.49(±8.63)] compared to sham stimulation [M(±SD) = 82.28(9.75) to 83.53(10.19)]. The WAB-R naming subtest revealed a significant effect of tDCS condition [F(1,10) = 7.11, p = 0.02, ηp2 = 0.42; power = 0.68], reflecting performance gains in the active condition at the 0-week [M(±SD) = 0.6(0.42)] and 6-week [M(±SD) = 0.21(0.63)] time points when compared to sham [0-week: M(±SD) = 0.21(0.13); 6-week: M(±SD) = 0.03(0.06); see Table 2 for WAB scores and Supplementary Table 1 for WAB statistics]. By contrast, change in performance on the auditory-verbal comprehension subtest was significantly lower in the active versus sham condition [F(1,10) = 6.42, p = 0.03]. However, further assessment suggests this finding does not represent meaningful change. Specifically, baseline scores in the active condition tended to be closer to ceiling. Furthermore, including randomization order as a covariate yielded no effect of stimulation condition (p = 0.06). No other WAB-R subtests significantly changed (p’s > 0.05). This and the finding that the auditory-verbal comprehension subset slightly, but significantly, declined following active versus sham stimulation potentially explains the lack of tDCS-induced changes on overall WAB-AQ. Randomization order was not significant in any analysis (p’s > 0.05). Lastly, there were no significant differences between baseline arm 1 versus arm 2 (paired samples t-test p > 0.8).
Cortical Thickness and Volume
Backward-Stepwise Linear Regressions
Greater cortical thickness of the IFGop significantly predicted naming gains at the 0-week time point in the active condition [F(1,10) = 6.08; b = 0.62; p = 0.03; R2Adjusted = 0.32; Figure 4]. Analyses of naming gains 6-week post-active stimulation revealed two significant predictors of tDCS-induced naming gains: greater cortical thickness of the pMTG and lower thickness of the pSTG [F(4,6) = 7.46; b = 0.92, −0.202; p = 0.02; R2Adjusted = 0.72; Figure 5]. Cortical thickness did not significantly predict naming performance following sham stimulation at either time point (p’s > 0.05).
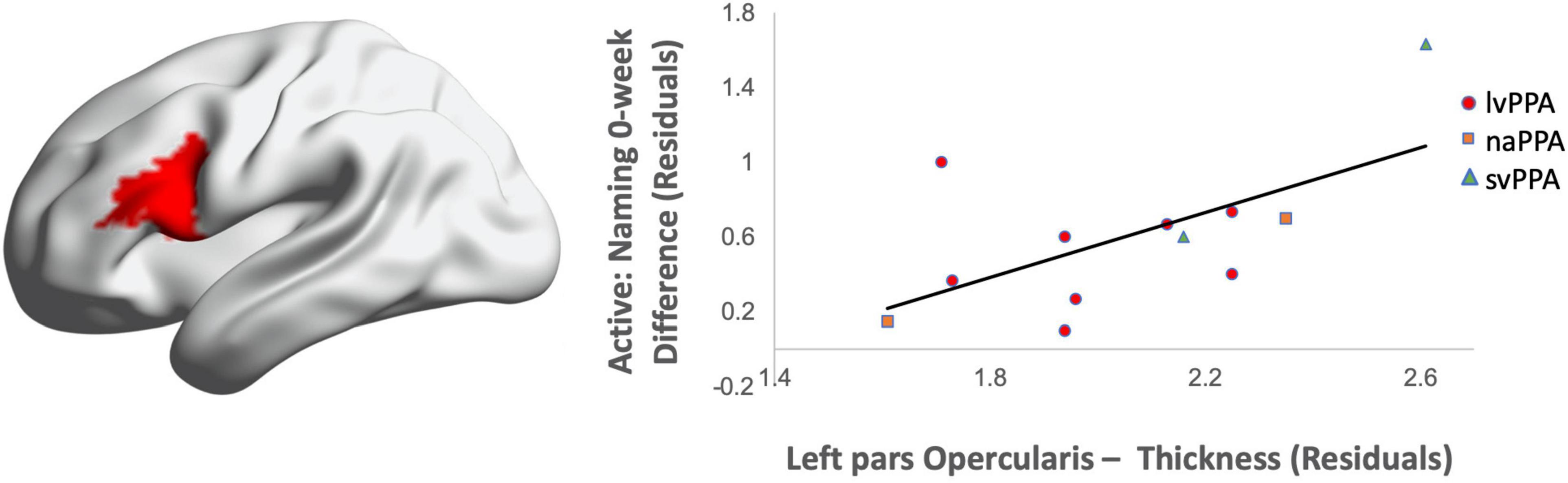
Figure 4. Greater baseline cortical thickness of the IFGop (highlighted in red) significantly predicted naming gains in the active condition at 0-week post-intervention (red = greater thickness; R2 = 0.32).
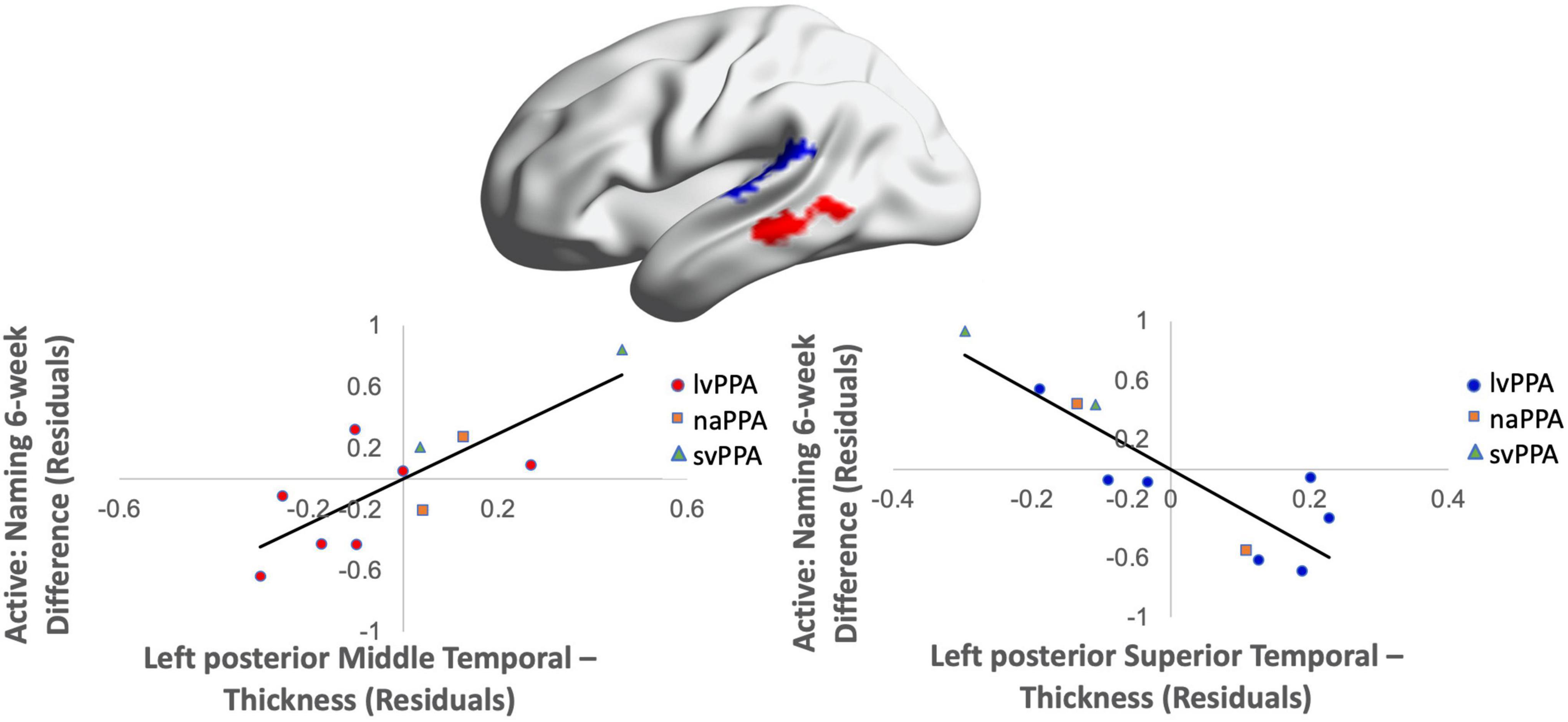
Figure 5. Greater baseline cortical thickness of the MTG (highlighted in red) and lower thickness of the pSTG (highlighted in blue) predicted naming gains in the active condition at 6-week post-intervention (red = greater thickness; blue = lower thickness; R2 = 0.72).
Cortical volume did not predict naming performance 0-week post-active stimulation (p’s > 0.05); however, baseline cortical volume of frontal and temporal regions significantly predicted naming gains at 6-week post-intervention: greater left IFGtr and pMTG volume and lower aSTG and pSTG volume [F(4,6) = 8.01; b = 0.76, 0.71, −0.57, −0.70; p = 0.014; R2Adjusted = 0.74; Figure 6]. In the sham condition, at the 0-week time point, greater cortical volume of the IFGorb and pMTG, but lower volume of the pSTG predicted naming gains [F(3,8) = 5.71; b’s = 0.697, 0.613, −0.836; p = 0.02; R2Adjusted = 0.56; Figure 7]. Cortical volume did not significantly predict naming performance 6-week post-sham (p’s > 0.05).
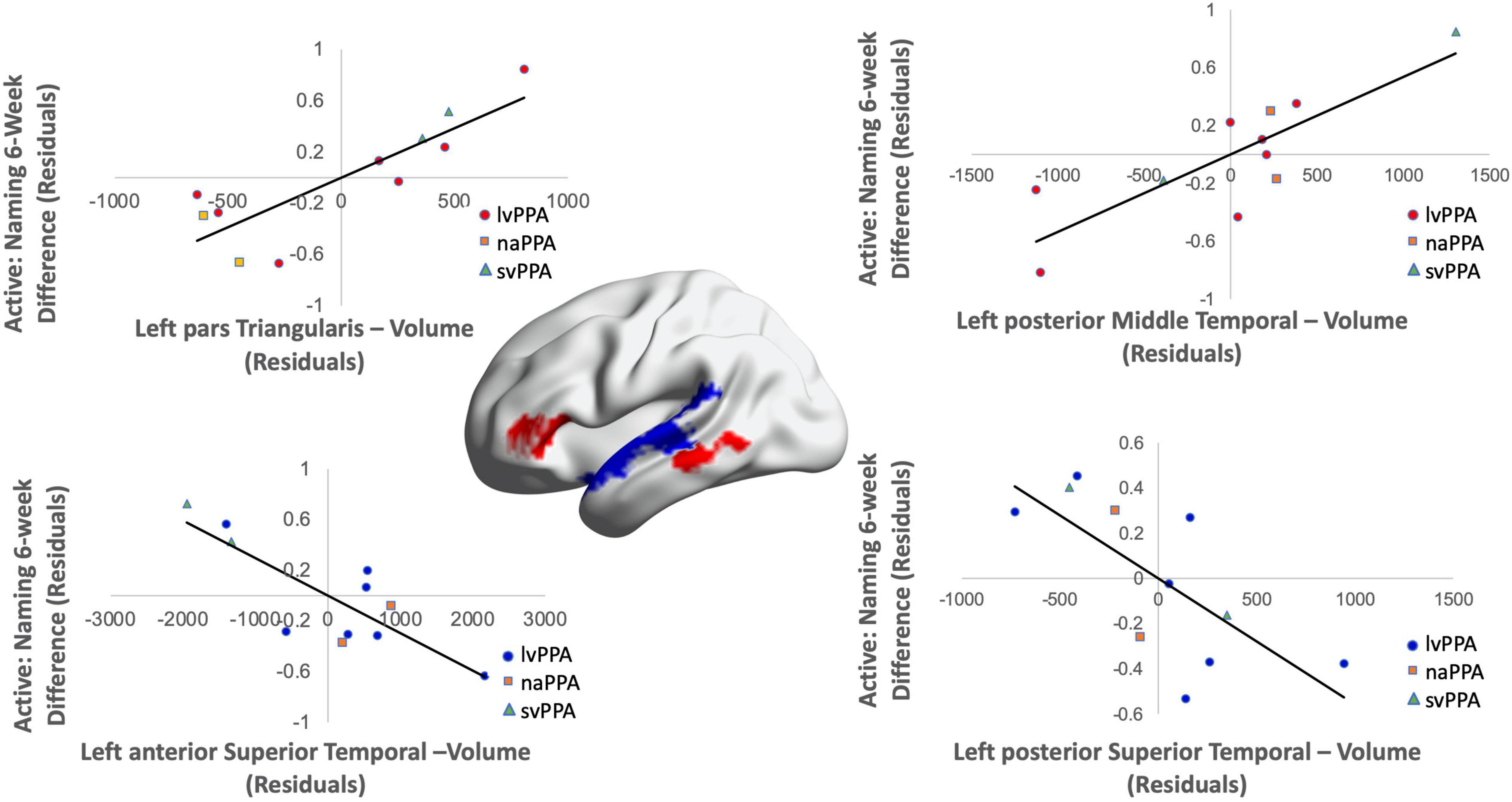
Figure 6. Greater baseline cortical volume of the IFGtr (highlighted in red), MTG (highlighted in red), and lower volume of aSTG and pSTG (highlighted in blue) predicted naming gains in the active condition at 6-week post-intervention (red = greater thickness; blue = lower thickness; R2 = 0.74).
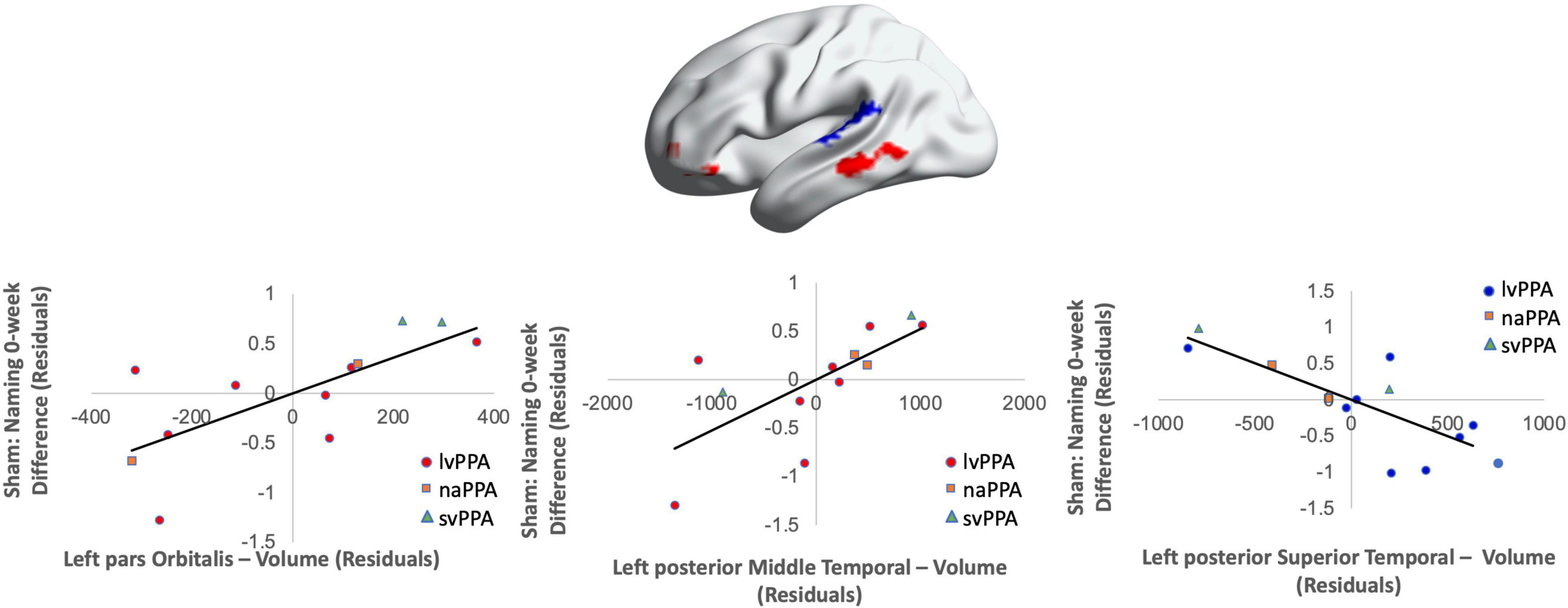
Figure 7. Greater baseline cortical thickness of the IFGorb (highlighted in red), MTG (highlighted in red), and lower thickness of the pSTG (highlighted in blue) predicted sham naming gains at 0-week post-intervention (red = greater thickness; blue = lower thickness; R2 = 0.56).
See Table 3 for a complete summary of regression model results. See Supplementary Figures 1, 2 for nonsignificant sham results.
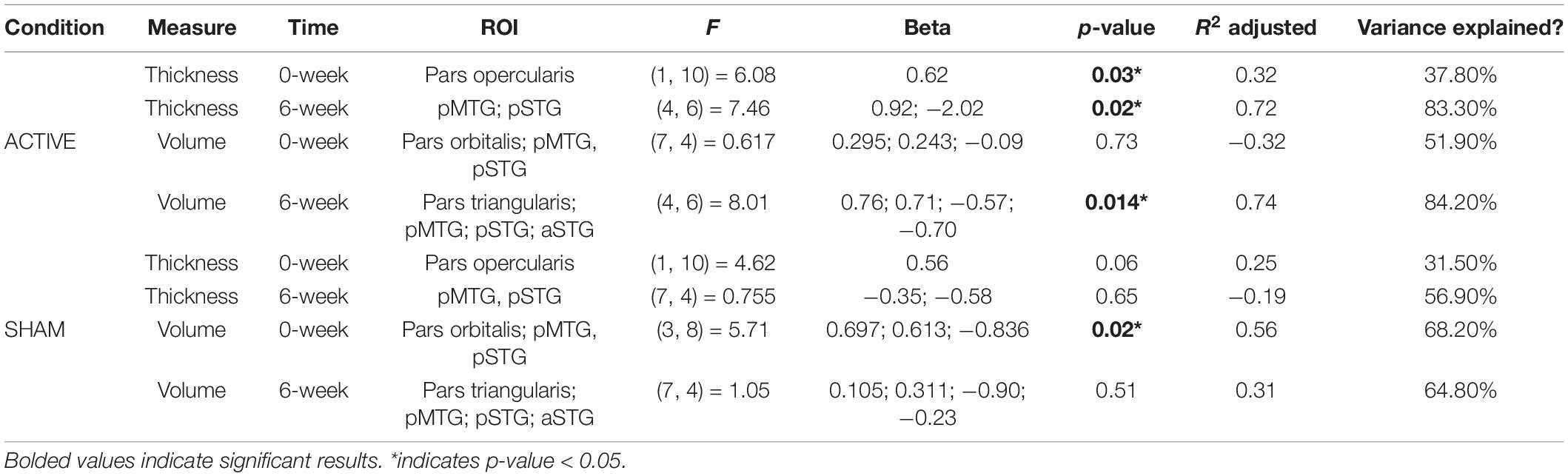
Table 3. Summary of linear regression (backward-fitted model) results for significant thickness and volume ROIs at the 0-week and 6-week time points.
Linear Regressions With Covariates
Global (total left hemisphere and whole brain) cortical thickness measures did not improve model fit above and beyond the significant ROIs that predicted active 0-week naming gains (IFGop; p > 0.05) or active 6-week naming gains (IFGtr, IFGorb, pMTG, pSTG; p’s > 0.05).
Similarly, global brain volume measures did not improve model fit above and beyond the significant ROIs that predicted active 6-week naming gains (IFGtr, pMTG, aSTG, pSTG; p > 0.05). Thus, disease severity expressed in volume loss does not explain tDCS-induced naming gains observed 6-week post-stimulation. Lastly, adding the global covariates to the model containing significant ROIs for the 0-week sham condition (IFGorb, pMTG, pSTG) did not improve model fit (p > 0.05). Together, these results provide further support for cortical thickness and volume of specific ROIs mediating short- and long-term active tDCS-induced naming gains.
Control Regions of Interest
Cortical thickness and volume of occipital cortex assessed as control ROIs did not significantly predict language gains at either the 0- or 6-week time points in either stimulation condition (p’s > 0.05). Similarly, Bayesian linear regression results confirm anecdotal to moderate evidence indicating an absence of effect in occipital ROIs (thickness and volume for active: 0-week BF10 = 0.36 and BF10 = 0.75, 6-week BF10 = 0.17 and BF10 = 0.20, respectively; sham 0-week BF10 = 0.19 and BF10 = 0.29, 6-week BF10 = 0.27 and 6-week BF10 = 0.23, respectively).
Discussion
The current study examined whether cortical thickness or volume of left hemisphere brain structures involved in language processing can predict tDCS-induced naming improvements in persons with PPA when compared to a control region and global atrophy measures more generally. Consistent with previous literature (Tsapkini et al., 2014, 2018; Cotelli et al., 2016; McConathey et al., 2017; Ficek et al., 2018; Fenner et al., 2019), we identified a significant enhancement in naming performance after active stimulation that was not observed in the sham condition. Baseline cortical thickness and volume of frontal and temporal regions involved in language predicted tDCS-induced enhancements in naming. Differential effects were observed at post-intervention time points such that improved naming performance was associated with greater cortical thickness and volume in certain regions (IFGop, IFGtr, MTG) and lower cortical thickness and volume in other regions (aSTG, pSTG). Moreover, baseline cortical thickness predicted naming gains immediately after therapy in anterior frontal regions and included posterior temporal regions at 6-week post-intervention. This suggests that a broader network may be necessary in the maintenance of tDCS-induced treatment gains. Collectively, these findings indicate that baseline cortical thickness and volume in language processing centers may be important factors for predicting response to brain stimulation in persons with PPA.
Cortical thickness is thought be a proxy for the integrity of the cerebral cortex and relates to the size, number, and density of cells in cortical structures (Rakic, 1988; Dickerson and Wolk, 2012), while volume is thought to be a proxy of neural reserve (Arenaza-Urquijo et al., 2013), or the extent to which the brain can harbor neuropathology without an individual experiencing functional impairments (Stern, 2002, 2010; Murray et al., 2011; Medaglia et al., 2017). Correspondingly, neuroimaging studies in patients with neurodegenerative disorders demonstrate that cortical thickness associates with neuronal loss (Shefer, 1973; Fischl and Dale, 2000), while lower volume associates with worse cognitive performance (Vollmer et al., 2016). Thus, our primary focus was on cortical thickness and volume of the left hemisphere language network to demonstrate whether baseline cortical measures could predict treatment-induced language gains. The fact that we identified strong correlations between cortical thickness and volume in language-relevant brain regions and tDCS-induced naming benefits experienced by persons with PPA aligns well with the roles that these brain areas are understood to have in naming and in language processing more broadly.
The IFG, a critical region for speech production, includes the two components of Broca’s area, the IFGop and IFGtr (Foundas et al., 1998; Holland et al., 2011). IFG recruitment occurs during propositional speech, verb generation (Blacker et al., 2006; Costafreda et al., 2006; Harrington et al., 2006), picture naming (Moore and Price, 1999; Harrington et al., 2006; Breier and Papanicolaou, 2008; Delikishkina et al., 2020), and action observation (Molnar-Szakacs et al., 1991). Given the essential nature and abundant connections of the IFG to other language-relevant regions (Friederici and Gierhan, 2013; Hertrich et al., 2020), it may be an important target for stimulation. Here, greater baseline cortical thickness and volume of the IFGop and IFGtr, respectively, associated with naming improvement after active, but not sham, stimulation. These findings suggest that spared or partially spared regions within the IFG are necessary for tDCS-induced enhancement of naming performance. Moreover, these findings lend support for targeting the IFG in the context of brain stimulation, and similarly are consistent with previous research showing that active tDCS over the IFG paired with SLT conferred improvements in naming and other aspects of language in PPA patients (Webster et al., 2016; Fenner et al., 2019; de Aguiar et al., 2020).
We also observed predicted results in the MTG, but encountered surprising results in the STG. Specifically, greater MTG but lower STG thickness and volume associated with naming gains in the active group. Neuroimaging studies demonstrate MTG activation during lexico-semantic retrieval (Baldo and Dronkers, 2006; Oppenheim et al., 2010; Harvey and Schnur, 2015), picture naming (Indefrey and Levelt, 2004), and processing complex action knowledge (Wallentin et al., 2011). This suggests that increased thickness and volume of the MTG may allow for stimulation-induced enhancements of semantic-to-lexical mapping critical for picture naming tasks (Schwartz et al., 2009). The STG, which is involved in word recognition and naming, comprises Wernicke’s area, a region vital for language comprehension. The findings that lower cortical measures of the STG predicted naming improvements in active group was somewhat unexpected given that regions with greater cortical atrophy have shown associations with decrements in language abilities (Rogalski and Mesulam, 2009; Rogalski et al., 2011; Rohrer, 2012; Rohrer et al., 2013). One explanation for the negative correlation between naming gains and lower STG thickness and volume may be that there is greater capacity for tDCS-induced improvement in individuals with greater baseline impairment. This aligns with previous research demonstrating that more impaired PPA patients at baseline may respond more positively to tDCS-related language therapies (McConathey et al., 2017). Our results also align with research demonstrating that lower baseline cortical volume of some language-related regions predicted improvement in written naming and spelling after tDCS intervention targeting the IFG in PPA patients (de Aguiar et al., 2020). In that study, letter accuracy improvements observed in active, but not sham, tDCS administered during therapy were associated with lower volume of the left angular gyrus (de Aguiar et al., 2020). Prior research in healthy participants has also confirmed that individual differences in cortical morphology of the left versus right prefrontal cortex associate with differences of tDCS effects on cognition in the context of decision-making (Filmer et al., 2019).
This study has several limitations. The sample size was small (N = 12) which reduces statistical power. However, this sample size is in line with previously published research involving tDCS in PPA patients that also report positive results on language tasks (Gervits et al., 2017; Fenner et al., 2019; Hosseini et al., 2019), suggesting it is a promising intervention in this population. It is possible that stimulation could increase general arousal. We find this unlikely in the current study, as previous research in healthy individuals assessing tDCS on semantic and phonemic fluency after left IFG stimulation have shown that active versus sham stimulation increases word production and control experiments confirmed that these findings were not dependent on levels of general arousal (Cattaneo et al., 2011). Although all PPA variants were included, the majority of participants were of the lvPPA subtype. Thus, differences in neuroanatomical predictors of stimulation effects across variants could not be assessed; results may not be as applicable to naPPA and svPPA patients. The range in values for lvPPA patients suggest results are not driven by categorical differences between variant subtype, but rather reflect variability predominantly in the lvPPA patients. Randomization was not well balanced for stimulation condition within and across variants. The current study findings are preliminary and should be validated in a larger sample of PPA patients with equal variant subtypes, severity, and randomization of stimulation condition. We selected cortical areas for analysis that are central in language processing, but it is possible that different regions in the language network would better predict tDCS-induced naming improvement. However, this explanation is unlikely because the inclusion of all left hemispheric cortical regions and the whole brain as covariates in our models as well as analysis of a control region, i.e., the occipital lobe, did not yield significant results, suggesting that other candidate left-hemispheric language regions or disease severity more generally do not account for the current findings. Thus, the functional role of stimulated regions and their connectivity with other regions likely plays an important role in determining response to stimulation.
Conclusion
Differential findings were observed in terms of thickness and volume, where some regions with greater or lower cortical measures at baseline were predictive of naming improvements after intervention. Nevertheless, these findings indicate that the degree and location of atrophy are important factors in response to naming-based enhancement from tDCS in PPA. Our findings provide insight into cortical predictors of tDCS-induced naming gains and lend support for stimulation of the left IFG as a promising target for improving SLT outcomes in persons with PPA. Taken together, these results provide guidance toward the application of HD-tDCS in PPA for rehabilitation protocols.
Data Availability Statement
The original contributions presented in this study are included in the article/Supplementary Material, further inquiries can be directed to the corresponding author.
Ethics Statement
The studies involving human participants were reviewed and approved by the University of Pennsylvania Institutional Review Board (IRB). The patients/participants provided their written informed consent to participate in this study.
Author Contributions
NN was responsible for the data analyses, figures, and prepared the original draft of the manuscript. DH aided in data analyses, figures, and manuscript edits. CH and LF aided in data acquisition and contributed to the original draft of the manuscript. PB, GL, JP, KC, and MG contributed to the neuroimaging acquisition, preprocessing, and edits the manuscript. SX aided in data analyses. RH was responsible for the intellectual contribution to the study design and data acquisition, and contributed to the data analyses, figures, and manuscript edits. All authors reviewed and approved the final manuscript.
Funding
This research was supported by the National Institute on Aging (R01AG059763 to RH), Laboratory for Cognition and Neural Stimulation, and Penn Frontotemporal Degeneration Center at the University of Pennsylvania. The T32 Moss Rehabilitation Research Institute/University of Pennsylvania post-doctoral training fellowship (NIH 5T32HD071844-05) also supported this work.
Conflict of Interest
The authors declare that the research was conducted in the absence of any commercial or financial relationships that could be construed as a potential conflict of interest.
Publisher’s Note
All claims expressed in this article are solely those of the authors and do not necessarily represent those of their affiliated organizations, or those of the publisher, the editors and the reviewers. Any product that may be evaluated in this article, or claim that may be made by its manufacturer, is not guaranteed or endorsed by the publisher.
Acknowledgments
Brain images were visualized with the BrainNet Viewer software (Xia et al., 2013, http://www.nitrc.org/projects/bnv/).
Supplementary Material
The Supplementary Material for this article can be found online at: https://www.frontiersin.org/articles/10.3389/fnhum.2022.907425/full#supplementary-material
References
Agosta, F., Galantucci, S., Canu, E., Cappa, S. F., Magnani, G., Franceschi, M., et al. (2013). Disruption of structural connectivity along the dorsal and ventral language pathways in patients with nonfluent and semantic variant primary progressive aphasia: a DT MRI study and a literature review. Brain Lang. 127, 157–166. doi: 10.1016/j.bandl.2013.06.003
Arenaza-Urquijo, E. M., Molinuevo, J. L., Sala-Llonch, R., Solé-Padullés, C., Balasa, M., Bosch, B., et al. (2013). Cognitive reserve proxies relate to gray matter loss in cognitively healthy elderly with abnormal cerebrospinal fluid amyloid-β levels. J. Alzheimers Dis. 35, 715–726. doi: 10.3233/JAD-121906
Avants, B. B., Tustison, N. J., Song, G., Cook, P. A., Klein, A., and Gee, J. C. (2011). A reproducible evaluation of ANTs similarity metric performance in brain image registration. Neuroimage 54, 2033–2044. doi: 10.1016/j.neuroimage.2010.09.025
Baldo, J. V., and Dronkers, N. (2006). The role of inferior parietal and inferior frontal cortex in workng memory. Neuropsychology 20, 529–538. doi: 10.1037/0894-4105.20.5.529
Beeson, P. M., King, R. M., Bonakdarpour, B., Henry, M. L., Cho, H., and Rapcsak, S. Z. (2011). Positive effects of language treatment for the logopenic variant of primary progressive aphasia. J. Mol. Neurosci. 45, 724–736. doi: 10.1007/s12031-011-9579-2
Blacker, D., Byrnes, M. L., Mastaglia, F. L., and Thickbroom, G. W. (2006). Differential activation of frontal lobe areas by lexical and semantic language tasks: a functional magnetic resonance imaging study. J. Clin. Neurosci. 13, 91–95. doi: 10.1016/j.jocn.2005.02.009
Breier, J., and Papanicolaou, A. (2008). Spatiotemporal patterns of brain activation during an action naming task using magnetoencephalography. J. Clin. Neurophysiol. 25, 7–12. doi: 10.1097/WNP.0b013e318163ccd5
Cattaneo, Z., Pisoni, A., and Papagno, C. (2011). Transcranial direct current stimulation over Broca’s region improves phonemic and semantic fluency in healthy individuals. Neuroscience 183, 64–70. doi: 10.1016/j.neuroscience.2011.03.058
Chrysikou, E. G., and Hamilton, R. H. (2011). Noninvasive brain stimulation in the treatment of aphasia: exploring interhemispheric relationships and their implications for neurorehabilitation. Restor. Neurol. Neurosci. 29, 375–394. doi: 10.3233/RNN-2011-0610
Ciccone, N., West, D., Cream, A., Cartwright, J., Rai, T., Granger, A., et al. (2015). Constraint-induced aphasia therapy (CIAT): a randomised controlled trial in very early stroke rehabilitation. Aphasiology 30, 566–584. doi: 10.1080/02687038.2015.1071480
Costafreda, S. G., Fu, C. H. Y., Lee, L., Everitt, B., Brammer, M. J., and David, A. S. (2006). A systematic review and quantitative appraisal of fMRI studies of verbal fluency: role of the left inferior frontal gyrus. Hum. Brain Map. 27, 799–810. doi: 10.1002/hbm.20221
Cotelli, M., Manenti, R., Ferrari, C., Gobbi, E., Macis, A., and Cappa, S. F. (2020). Effectiveness of language training and non-invasive brain stimulation on oral and written naming performance in Primary Progressive Aphasia: a meta-analysis and systematic review. Neurosci. Biobehav. Rev. 108, 498–525. doi: 10.1016/j.neubiorev.2019.12.003
Cotelli, M., Manenti, R., Paternico, M., Brambilla, M., Petesi, M., Premi, E., et al. (2016). Grey matter density predicts the improvement of naming abilities after tDCS intervention in agrammatic variant of primary progressive aphasia. Brain Topogr. 29, 738–751. doi: 10.1007/s10548-016-0494-2
Datta, A., Truong, D., Minhas, P., Parra, L. C., and Bikson, M. (2012). Inter-individual variation during transcranial direct current stimulation and normalization of dose using MRI-derived computational models. Front. Psychiatry 3:91. doi: 10.3389/fpsyt.2012.00091
de Aguiar, V., Zhao, Y., Faria, A., Ficek, B., Webster, K. T., Wendt, H., et al. (2020). Brain volumes as predictors of tDCS effects in primary progressive aphasia. Brain Lang. 200:104707 doi: 10.1016/j.bandl.2019.104707
Delikishkina, E., Lingnau, A., and Miceli, G. (2020). Neural correlates of object and action naming practice. Cortex 131, 87–102. doi: 10.1016/j.cortex.2020.05.005
Dickerson, B. C., Bakkour, A., Salat, D. H., Feczko, E., Pacheco, J., Greve, D. N., et al. (2009). The cortical signature of Alzheimer’s disease: regionally specific cortical thinning relates to symptom severity in very mild to mild AD dementia and is detectable in asymptomatic amyloid-positive individuals. Cereb. Cortex 19, 497–510. doi: 10.1093/cercor/bhn113
Dickerson, B. C., and Wolk, D. A. (2012). MRI cortical thickness biomarker predicts AD-like CSF and cognitive decline in normal adults. Neurology 78, 84–90. doi: 10.1212/WNL.0b013e31823efc6c
Dubreuil-Vall, L., Chau, P., Ruffini, G., Widge, A. S., and Camprodon, J. A. (2019). tDCS to the left DLPFC modulates cognitive and physiological correlates of executive function in a state-dependent manner. Brain Stimulat. 12, 1456–1463. doi: 10.1016/j.brs.2019.06.006
Engvig, A., Fjell, A. M., Westlye, L. T., Moberget, T., Sundseth, O., Larsen, V. A., et al. (2010). Effects of memory training on cortical thickness in the elderly. Neuroimage 52, 1667–1676. doi: 10.1016/j.neuroimage.2010.05.041
Fenner, A. S., Webster, K. T., Ficek, B. N., Frangakis, C. E., and Tsapkini, K. (2019). Written verb naming improves after tDCS over the left IFG in primary progressive aphasia. Front. Psychol. 10:1396. doi: 10.3389/fpsyg.2019.01396
Ficek, B. N., Wang, Z., Zhao, Y., Webster, K. T., Desmond, J. E., Hillis, A. E., et al. (2018). The effect of tDCS on functional connectivity in primary progressive aphasia. Neuroimage 19, 703–715. doi: 10.1016/j.nicl.2018.05.023
Filmer, H. L., Ehrhardt, S. E., Shaw, T. B., Mattingley, J. B., and Dux, P. E. (2019). The efficacy of transcranial direct current stimulation to prefrontal areas is related to underlying cortical morphology. Neuroimage 196, 41–48. doi: 10.1016/j.neuroimage.2019.04.026
Fischl, B., and Dale, A. M. (2000). Measuring the thickness of the human cerebral cortex from magnetic resonance images. Proc. Natl. Acad. Sci. U. S. A. 97, 11050–11055. doi: 10.1073/pnas.200033797
Folstein, M. F., Folstein, S. E., and McHugh, P. R. (1975). “Mini-mental state”: a practical method for grading the cognitive state of patients for the clinician. J. Psychiatr. Res. 12, 189–198. doi: 10.1016/0022-3956(75)90026-6
Foundas, A. L., Eure, K. F., Luevano, L. F., and Weinberger, D. R. (1998). MRI asymmetries of Broca’s area: the pars triangularis and pars opercularis. Brain Lang. 64, 282–296. doi: 10.1006/brln.1998.1974
Friederici, A. D., and Gierhan, S. M. E. (2013). The language network. Curr. Opin. Neurobiol. 23, 250–254. doi: 10.1016/j.conb.2012.10.002
Gervits, F., Ash, S., Coslett, H. B., Rascovsky, K., Grossman, M., and Hamilton, R. (2017). Transcranial direct current stimulation for the treatment of primary progressive aphasia: an open-label pilot study. Brain Lang. 47, 549–562. doi: 10.1097/CCM.0b013e31823da96d.Hydrogen
Giannini, L. A. A., Irwin, D. J., Mcmillan, C. T., Ash, S., Rascovsky, K., Wolk, D. A., et al. (2017). Clinical marker for Alzheimer disease pathology in logopenic primary progressive aphasia. Neurology 88, 2276–2284. doi: 10.1212/WNL.0000000000004034
Gill, J., Shah-Basak, P. P., and Hamilton, R. (2015). It’s the thought that counts: examining the task-dependent effects of transcranial direct current stimulation on executive function. Brain Stimulat. 8, 253–259. doi: 10.1016/j.brs.2014.10.018
Gorno-Tempini, M. L., Hillis, A. E., Weintraub, S., Kertesz, A., Mendez, M., Cappa, S. F., et al. (2011). Classification of primary progressive aphasia and its variants. Neurology 76, 1006–1014. doi: 10.1212/WNL.0b013e31821103e6
Grossman, M. (2012). The non-fluent/agrammatic variant of primary progressive aphasia. Lancet Neurol. 11, 545–555. doi: 10.1016/S1474-4422(12)70099-6.THE
Grossman, M. (2018). Linguistic aspects of primary progressive aphasia. Annu. Rev. Linguist. 4, 377–403. doi: 10.1146/annurev-linguistics-011516-034253
Grossman, M., and Ash, S. (2004). Primary progressive aphasia: a review. Neurocase 10, 3–18. doi: 10.1080/13554790490960440
Hameister, I., Nickels, L., Abel, S., and Croot, K. (2017). “Do you have mowing the lawn?”–improvements in word retrieval and grammar following constraint-induced language therapy in primary progressive aphasia. Aphasiology 31, 308–331. doi: 10.1080/02687038.2016.1197558
Harrington, G. S., Buonocore, M. H., and Tomaszewski Farias, S. (2006). Intrasubject reproducibility of functional MR imaging activation in language tasks. Am. J. Neuroradiol. 27, 938–944.
Harvey, D. Y., and Schnur, T. T. (2015). Distinct loci of lexical and semantic access deficits in aphasia: evidence from voxel-based lesion-symptom mapping and diffusion tensor imaging. Cortex 67, 37–58. doi: 10.1016/j.cortex.2015.03.004
Hertrich, I., Dietrich, S., and Ackermann, H. (2020). The margins of the language network in the brain. Front. Commun. 5:519955. doi: 10.3389/fcomm.2020.519955
Holland, R., Leff, A. P., Josephs, O., Galea, J. M., Desikan, M., Price, C. J., et al. (2011). Speech facilitation by left inferior frontal cortex stimulation. Curr. Biol. 21, 1403–1407. doi: 10.1016/j.cub.2011.07.021
Horvath, J. C., Carter, O., and Forte, J. D. (2014). Transcranial direct current stimulation: five important issues we aren’t discussing (but probably should be). Front. Syst. Neurosci. 8:2. doi: 10.3389/fnsys.2014.00002
Hosseini, M., McConathey, E. M., Ungrady, M., Grossman, M., Coslett, H. B., and Hamilton, R. H. (2019). Proceedings #10: transcranial direct current stimulation mediates improvements in verbal fluency for patients with primary progressive aphasia. Brain Stimulat. 12, e69–e71. doi: 10.1016/j.brs.2018.12.179
Indefrey, P., and Levelt, W. J. M. (2004). The spatial and temporal signatures of word production components. Cognition 92, 101–144. doi: 10.1016/j.cognition.2002.06.001
Kan, I. P., and Thompson-Schill, S. L. (2004). Effect of name agreement on prefrontal activity during overt and covert picture naming. Cognit. Affect. Behav. Neurosci. 4, 43–57. doi: 10.3758/CABN.4.1.43
Kim, J.-H., Kim, D.-W., Chang, W. H., Kim, Y.-H., Kim, K., and Im, C.-H. (2014). Inconsistent outcomes of transcranial direct current stimulation may originate from anatomical differences among individuals: electric field simulation using individual MRI data. Neurosci. Lett. 564, 6–10. doi: 10.1016/j.neulet.2014.01.054
Kirmess, M., and Maher, L. M. (2010). Constraint induced language therapy in early aphasia rehabilitation. Aphasiology 24, 725–736. doi: 10.1080/02687030903437682
Klein, A., Andersson, J., Ardekani, B. A., Ashburner, J., Avants, B., Chiang, M. C., et al. (2009). Evaluation of 14 nonlinear deformation algorithms applied to human brain MRI registration. Neuroimage 46, 786–802. doi: 10.1016/j.neuroimage.2008.12.037.Evaluation
Kronberg, G., Rahman, A., Sharma, M., Bikson, M., and Parra, L. C. (2020). Direct current stimulation boosts hebbian plasticity in vitro. Brain Stimulat. 13, 287–301. doi: 10.1016/j.brs.2019.10.014
MacDonald, A. D., Heath, S., McMahon, K. L., Nickels, L., Angwin, A. J., van Hees, S., et al. (2015). Neuroimaging the short- and long-term effects of repeated picture naming in healthy older adults. Neuropsychologia 75, 170–178. doi: 10.1016/j.neuropsychologia.2015.06.007
Mahdavi, S., and Towhidkhah, F. (2018). Computational human head models of tDCS: influence of brain atrophy on current density distribution. Brain Stimulat. 11, 104–107. doi: 10.1016/j.brs.2017.09.013
Maher, L. M., Kendall, D., Swearengin, J. A., Rodriguez, A., Leon, S. A., Pingel, K., et al. (2006). A pilot study of use-dependent learning in the context of constraint induced language therapy. J. Int. Neuropsychol. Soc. 12, 843–852. doi: 10.1017/S1355617706061029
Makris, N., Biederman, J., Valera, E. M., Bush, G., Kaiser, J., Kennedy, D. N., et al. (2007). Cortical thinning of the attention and executive function networks in adults with attention-deficit/hyperactivity disorder. Cereb. Cortex 17, 1364–1375. doi: 10.1093/cercor/bhl047
McConathey, E. M., White, N. C., Gervits, F., Ash, S., Coslett, H. B., Grossman, M., et al. (2017). Baseline performance predicts tDCS-mediated improvements in language symptoms in primary progressive aphasia. Front. Hum. Neurosci. 11:347. doi: 10.3389/fnhum.2017.00347
McGugin, R. W., Van Gulick, A. E., and Gauthier, I. (2016). Cortical thickness in fusiform face area predicts face and object recognition performance. J. Cognit. Neurosci. 28, 282–294. doi: 10.1162/jocn_a_00891
Medaglia, J. D., Pasqualetti, F., Hamilton, R. H., Thompson-Schill, S. L., and Bassett, D. S. (2017). Brain and cognitive reserve: translation via network control theory. Neurosci. Biobehav. Rev. 75, 53–64. doi: 10.1016/j.neubiorev.2017.01.016
Mesulam, M. M. (2003). Primary progressive aphasia — a language-based dementia. N. Engl. J. Med. 349, 1535–1542. doi: 10.1056/NEJMra022435
Mesulam, M. M., Weintraub, S., Rogalski, E. J., Wieneke, C., Geula, C., and Bigio, E. H. (2014). Asymmetry and heterogeneity of Alzheimer’s and frontotemporal pathology in primary progressive aphasia. Brain 137, 1176–1192. doi: 10.1093/brain/awu024
Meyer, A. M., Faria, A. V., Tippett, D. C., Hillis, A. E., and Friedman, R. B. (2017). The relationship between baseline volume in temporal areas and post-treatment naming accuracy in primary progressive aphasia. Aphasiology 31, 1059–1077. doi: 10.1080/02687038.2017.1296557
Molnar-Szakacs, I., Iacoboni, M., Koski, L., and Mazziotta, J. C. (1991). Functional segregation within pars opercularis of the inferior frontal gyrus: evidence from fMRI studies of imitation and action observation. Cereb. Cortex 2005, 986–994. doi: 10.1093/cercor/bhh199
Monte-Silva, K., Kuo, M. F., Hessenthaler, S., Fresnoza, S., Liebetanz, D., Paulus, W., et al. (2013). Induction of late LTP-like plasticity in the human motor cortex by repeated non-invasive brain stimulation. Brain Stimulat. 6, 424–432. doi: 10.1016/j.brs.2012.04.011
Moore, C. J., and Price, C. J. (1999). Three distinct ventral occipitotemporal regions for reading and object naming. Neuroimage 10, 181–192. doi: 10.1006/nimg.1999.0450
Mulder, M. J., Keuken, M. C., Bazin, P. L., Alkemade, A., and Forstmann, B. U. (2019). Size and shape matter: the impact of voxel geometry on the identification of small nuclei. PLoS One 14:e0215382. doi: 10.1371/journal.pone.0215382
Murray, A. D., Staff, R. T., McNeil, C. J., Salarirad, S., Ahearn, T. S., Mustafa, N., et al. (2011). The balance between cognitive reserve and brain imaging biomarkers of cerebrovascular and Alzheimer’s diseases. Brain 134, 3684–3693. doi: 10.1093/brain/awr259
Newhart, M., Ken, L., Kleinman, J. T., Heidler-Gary, J., and Hillis, A. E. (2007). Neural networks essential for naming and word comprehension. Cognit. Behav. Neurol. 20, 25–30. doi: 10.1097/WNN.0b013e31802dc4a7
Nissim, N. R., Moberg, P. J., and Hamilton, R. H. (2020). Efficacy of Noninvasive Brain Stimulation (tDCS or TMS) paired with language therapy in the treatment of primary progressive aphasia: an exploratory meta-analysis. Brain Sci. 10, 1–18. doi: 10.3390/brainsci10090597
Nissim, N. R., O’Shea, A. M., Bryant, V., Porges, E. C., Cohen, R., and Woods, A. J. (2017). Frontal structural neural correlates of working memory performance in older adults. Front. Aging Neurosci. 8:328. doi: 10.3389/fnagi.2016.00328
Nitsche, M. A., and Paulus, W. (2000). Excitability changes induced in the human motor cortex by weak transcranial direct current stimulation. J. Physiol. 527(Pt 3), 633–639. doi: 10.1111/j.1469-7793.2000.t01-1-00633.x
Nitsche, M. A., Seeber, A., Frommann, K., Klein, C. C., Rochford, C., Nitsche, M. S., et al. (2005). Modulating parameters of excitability during and after transcranial direct current stimulation of the human motor cortex. J. Physiol. 568, 291–303. doi: 10.1113/jphysiol.2005.092429
Norise, C., and Hamilton, R. H. (2017). Non-invasive brain stimulation in the treatment of post-stroke and neurodegenerative aphasia: parallels, differences, and lessons learned. Front. Hum. Neurosci. 10:675. doi: 10.3389/fnhum.2016.00675
Ojemann, G. A., Creutzfeldt, O., Lettich, E., and Haglund, M. M. (1988). Neuronal activity in human lateral temporal cortex related to short-term verbal memory, naming and reading. Brain 111, 1383–1403. doi: 10.1093/brain/111.6.1383
Oppenheim, G. M., Dell, G. S., and Schwartz, M. F. (2010). The dark side of incremental learning: a model of cumulative semantic interference during lexical access in speech production. Cognition 114, 227–252. doi: 10.1016/j.cognition.2009.09.007
Palm, U., Reisinger, E., Keeser, D., Kuo, M.-F., Pogarell, O., Leicht, G., et al. (2013). Evaluation of sham transcranial direct current stimulation for randomized, placebo-controlled clinical trials. Brain Stimulat. 6, 690–695. doi: 10.1016/j.brs.2013.01.005
Pelletier, S. J., and Cicchetti, F. (2015). Cellular and molecular mechanisms of action of transcranial direct current stimulation: evidence from in vitro and in vivo models. Int. J. Neuropsychopharmacol. 18:pyu047. doi: 10.1093/ijnp/pyu047
Pellicciari, M. C., and Miniussi, C. (2018). Transcranial direct current stimulation in neurodegenerative disorders. J. ECT 34, 193–202. doi: 10.1097/YCT.0000000000000539
Phillips, J. S., Da Re, F., Dratch, L., Xie, S. X., Irwin, D. J., McMillan, C. T., et al. (2018). Neocortical origin and progression of gray matter atrophy in nonamnestic Alzheimer’s disease. Neurobiol. Aging 63, 75–87. doi: 10.1016/j.neurobiolaging.2017.11.008
Pini, L., Manenti, R., Cotelli, M., Pizzini, F. B., Frisoni, G. B., and Pievani, M. (2018). Non-invasive brain stimulation in dementia: a complex network story. Neurodegen. Dis. 18, 281–301. doi: 10.1159/000495945
Pini, L., Pievani, M., Bocchetta, M., Altomare, D., Bosco, P., Cavedo, E., et al. (2016). Brain atrophy in Alzheimer’s Disease and aging. Ageing Res. Rev. 30, 25–48. doi: 10.1016/j.arr.2016.01.002
Pulvermüller, F., Neininger, B., Elbert, T., Mohr, B., Rockstroh, B., Koebbel, P., et al. (2001). Constraint-induced therapy of chronic aphasia after stroke. Stroke 32, 1621–1626. doi: 10.1161/01.STR.32.7.1621
Rahman, A., Reato, D., Arlotti, M., Gasca, F., Datta, A., Parra, L. C., et al. (2013). Cellular effects of acute direct current stimulation: somatic and synaptic terminal effects. J. Physiol. 591, 2563–2578. doi: 10.1113/jphysiol.2012.247171
Rakic, P. (1988). Specification of cerebral cortical areas. Science 241, 170–176. doi: 10.1126/science.3291116
Rogalski, E. J., Cobia, D., Harrison, T. M., Wieneke, C., Thompson, C. K., Weintraub, S., et al. (2011). Anatomy of language impairments in primary progressive aphasia. J. Neurosci. 31, 3344–3350. doi: 10.1523/JNEUROSCI.5544-10.2011
Rogalski, E. J., and Mesulam, M. M. (2009). Clinical trajectories and biological features of primary progressive aphasia (PPA). Curr. Alzheimer Res. 6, 331–336. doi: 10.1038/jid.2014.371
Rohrer, J. D. (2012). Structural brain imaging in frontotemporal dementia. Biochim. Biophys. Acta 1822, 325–332. doi: 10.1016/j.bbadis.2011.07.014
Rohrer, J. D., Caso, F., Mahoney, C., Henry, M., Rosen, H. J., Rabinovici, G., et al. (2013). Patterns of longitudinal brain atrophy in the logopenic variant of primary progressive aphasia. Brain Lang. 127, 121–126. doi: 10.1016/j.bandl.2012.12.008
Schmidt, E. L., Burge, W., Visscher, K. M., and Ross, L. A. (2016). Cortical thickness in frontoparietal and cingulo-opercular networks predicts executive function performance in older adults. Neuropsychology 30, 322–331. doi: 10.1037/neu0000242
Schwartz, M. F., Kimberg, D. Y., Walker, G. M., Faseyitan, O., Brecher, A., Dell, G. S., et al. (2009). Anterior temporal involvement in semantic word retrieval: voxel-based lesion-symptom mapping evidence from aphasia. Brain 132, 3411–3427. doi: 10.1093/brain/awp284
Shefer, V. F. (1973). Absolute number of neurons and thickness of the cerebral cortex during aging, senile and vascular dementia, and Pick's and Alzheimer's diseases. Neurosci. Behav. Physiol. 6, 319–324.
Sreepadma, P. S., Mesulam, M.-M., Cynthia, K. T., Nancy, A. J., Sandra, W., Todd, B. P., et al. (2003). Primary progressive aphasia: PPA and the language network. Ann. Neurol. 53, 35–49. doi: 10.1002/ana.10390
Stern, Y. (2002). What is cognitive reserve? Theory and research application of the reserve concept. J. Int. Neuropsychol. Soc. 8, 448–460. doi: 10.1017/S1355617702813248
Stern, Y. (2010). Cognitive reserve. Neuropsychologia 47, 2015–2028. doi: 10.1016/j.neuropsychologia.2009.03.004.Cognitive
Thanprasertsuk, S., and Likitjaroen, Y. (2021). Pattern of cortical thinning in logopenic progressive aphasia patients in Thailand. BMC Neurol. 21:22. doi: 10.1186/s12883-020-02039-x
Tippett, D. C., Hillis, A. E., and Tsapkini, K. (2015). Treatment of primary progressive aphasia. Curr. Treat. Options Neurol. 17:362. doi: 10.1007/s11940-015-0362-5
Tisdall, M., Reuter, M., Qureshi, A., Buckner, R., Fischl, B., and van der Kouwe, A. (2016). Prospective motion correction with volumetric navigators (vNavs) reduces the bias and variance in brain morphometry induced by subject motion. Neuroimage 127, 11–22. doi: 10.1016/j.neuroimage.2015.11.054.Prospective
Tsapkini, K., Frangakis, C., Gomez, Y., Davis, C., and Hillis, A. E. (2014). Augmentation of spelling therapy with transcranial direct current stimulation in primary progressive aphasia: preliminary results and challenges. Aphasiology 28, 1112–1130. doi: 10.1080/02687038.2014.930410
Tsapkini, K., Webster, K. T., Ficek, B. N., Desmond, J. E., Onyike, C. U., Rapp, B., et al. (2018). Electrical brain stimulation in different variants of primary progressive aphasia: a randomized clinical trial. Alzheimers Dement. 4, 461–472. doi: 10.1016/j.trci.2018.08.002
Turkeltaub, P. E., Messing, S., Norise, C., and Hamilton, R. H. (2011). Are networks for residual language function and recovery consistent across aphasic patients? Neurology 76, 1726–1734. doi: 10.1212/WNL.0b013e31821a44c1
Tustison, N. J., Cook, P. A., Klein, A., Song, G., Das, S. R., Duda, J. T., et al. (2014). Large-scale evaluation of ANTs and FreeSurfer cortical thickness measurements. Neuroimage 99, 166–179. doi: 10.1016/j.neuroimage.2014.05.044
Unal, G., Ficek, B., Webster, K., Shahabuddin, S., Truong, D., Hampstead, B., et al. (2020). Impact of brain atrophy on tDCS and HD-tDCS current flow: a modeling study in three variants of primary progressive aphasia. Neurol. Sci. 41, 1781–1789. doi: 10.1007/s10072-019-04229-z
Vecchio, F., Pellicciari, M. C., Miraglia, F., Brignani, D., Miniussi, C., and Rossini, P. M. (2016). Effects of transcranial direct current stimulation on the functional coupling of the sensorimotor cortical network. Neuroimage 140, 50–56. doi: 10.1016/j.neuroimage.2016.01.051
Vollmer, T., Huynh, L., Kelley, C., Galebach, P., Signorovitch, J., DiBernardo, A., et al. (2016). Relationship between brain volume loss and cognitive outcomes among patients with multiple sclerosis: a systematic literature review. Neurol. Sci. 37, 165–179. doi: 10.1007/s10072-015-2400-1
Walenski, M., Europa, E., Caplan, D., and Thompson, C. K. (2019). Neural networks for sentence comprehension and production: an ALE-based meta-analysis of neuroimaging studies. Hum. Brain Map. 40, 2275–2304. doi: 10.1002/hbm.24523
Wallentin, M., Nielsen, A. H., Vuust, P., Dohn, A., Roepstorff, A., and Lund, T. E. (2011). BOLD response to motion verbs in left posterior middle temporal gyrus during story comprehension. Brain Lang. 119, 221–225. doi: 10.1016/j.bandl.2011.04.006
Webster, K. T., Chakravarty, T., Ficek, B., Onyike, C., Frangakis, C. F., Hillis, A. E., et al. (2016). Electrostimulation effects in lvPPA and nfvPPA. Ann. Neurol. 80:S1-S432. doi: 10.1002/ana.24759
Winkler, A. M., Kochunov, P., Blangero, J., Almasy, L., Zilles, K., Fox, P. T., et al. (2010). Cortical thickness or grey matter volume? The importance of selecting the phenotype for imaging genetics studies. Neuroimage 53, 1135–1146. doi: 10.1016/j.neuroimage.2009.12.028
Keywords: transcranial direct current stimulation (tDCS), primary progressive aphasia (PPA), constraint induced language therapy (CILT), structural neuroimaging, western aphasia battery-revised (WAB-R)
Citation: Nissim NR, Harvey DY, Haslam C, Friedman L, Bharne P, Litz G, Phillips JS, Cousins KAQ, Xie SX, Grossman M and Hamilton RH (2022) Through Thick and Thin: Baseline Cortical Volume and Thickness Predict Performance and Response to Transcranial Direct Current Stimulation in Primary Progressive Aphasia. Front. Hum. Neurosci. 16:907425. doi: 10.3389/fnhum.2022.907425
Received: 29 March 2022; Accepted: 02 June 2022;
Published: 07 July 2022.
Edited by:
Ferdinand Binkofski, RWTH Aachen University, GermanyReviewed by:
Dirk B. Den Ouden, University of South Carolina, United StatesTed Maldonado, Indiana State University, United States
Copyright © 2022 Nissim, Harvey, Haslam, Friedman, Bharne, Litz, Phillips, Cousins, Xie, Grossman and Hamilton. This is an open-access article distributed under the terms of the Creative Commons Attribution License (CC BY). The use, distribution or reproduction in other forums is permitted, provided the original author(s) and the copyright owner(s) are credited and that the original publication in this journal is cited, in accordance with accepted academic practice. No use, distribution or reproduction is permitted which does not comply with these terms.
*Correspondence: Roy H. Hamilton, Um95LkhhbWlsdG9uQHBlbm5tZWRpY2luZS51cGVubi5lZHU=