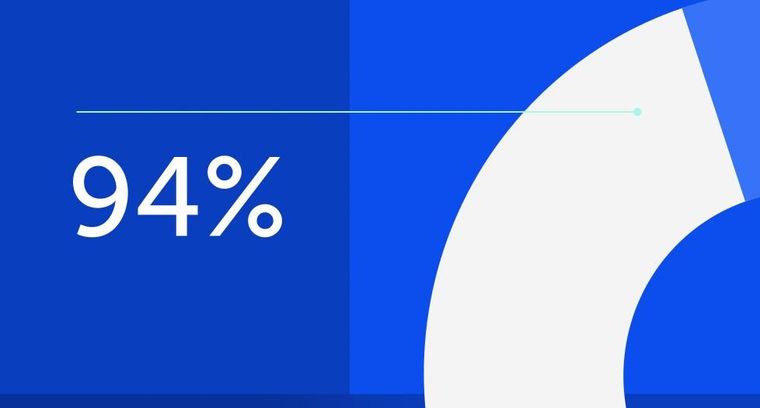
94% of researchers rate our articles as excellent or good
Learn more about the work of our research integrity team to safeguard the quality of each article we publish.
Find out more
SYSTEMATIC REVIEW article
Front. Hum. Neurosci., 29 March 2022
Sec. Brain-Computer Interfaces
Volume 16 - 2022 | https://doi.org/10.3389/fnhum.2022.798883
This article is part of the Research TopicAI-based computer-aided diagnosis and prognosis for psychiatric disordersView all 5 articles
Objective: This study aimed to examine the effectiveness and safety of the Brain-computer interface (BCI) in treatment of upper limb dysfunction after stroke.
Methods: English and Chinese electronic databases were searched up to July 2021. Randomized controlled trials (RCTs) were eligible. The methodological quality was assessed using Cochrane’s risk-of-bias tool. Meta-analysis was performed using RevMan 5.4.
Results: A total of 488 patients from 16 RCTs were included. The results showed that (1) the meta-analysis of BCI-combined treatment on the improvement of the upper limb function showed statistical significance [standardized mean difference (SMD): 0.53, 95% CI: 0.26–0.80, P < 0.05]; (2) BCI treatment can improve the abilities of daily living of patients after stroke, and the analysis results are statistically significant (SMD: 1.67, 95% CI: 0.61–2.74, P < 0.05); and (3) the BCI-combined therapy was not statistically significant for the analysis of the Modified Ashworth Scale (MAS) (SMD: −0.10, 95% CI: −0.50 to 0.30, P = 0.61).
Conclusion: The meta-analysis indicates that the BCI therapy or BCI combined with other therapies such as conventional rehabilitation training and motor imagery training can improve upper limb dysfunction after stroke and enhance the quality of daily life.
In the past two decades, the number of patients with stroke is increasing (Van de Port et al., 2009). Stroke leads to various functional disorders, and the upper limb dysfunction is one of the most common disorders. Upper limb dysfunction not only reduces the quality of patients’ daily life but also makes it difficult to carry out normal rehabilitation training (Feigin et al., 2014). In contrast, it prolongs hospital stay and increases medical costs to patients. Conventional therapies for upper limb dysfunction include postural interventions, functional maneuvers, and exercises. Even though the abovementioned treatments have been widely applied in clinical practice, there is not enough clinical evidence to prove their efficacy. In addition, the single and repeated rehabilitation training are difficult to achieve the expected effect for some patients, and the limited nerve rehabilitation model can no longer meet the increasing rehabilitation needs of patients with stroke (Wang et al., 2010). Therefore, it is very important to find an effective rehabilitation method for stroke.
Brain-computer interface (BCI), an artificial intelligence technology applied in the medical field, is one of the auxiliary means of rehabilitation treatment (Lopez-Larraz et al., 2018). By acting directly on the brain, it can induce the plasticity of the brain and promote the functional reorganization of the brain (Spuler et al., 2018). As we all know, stroke will cause functional or structural changes in the human brain, which will lead to abnormal electroencephalogram (EEG) activity or changes in interhemispheric cerebral excitability (Bolognini et al., 2011; Montenegro et al., 2016). BCI can use brain signals as an alternative channel for communication or equipment control to bypass the brain’s normal output channels of peripheral nerves and muscles. Maybe potentially as a way to influence the neural plasticity process of the brain so as to induce the recovery of normal movement (Daly and Wolpaw, 2008). Therefore, it is becoming a growing area of interest for rehabilitation after stroke (Spuler et al., 2018).
Reviewing previous studies of BCI as treatment of patients with stroke, most clinical research of BCI therapy mainly focused on treatment of upper limb dysfunction after stroke, and several studies reported that BCI has positive findings in clinical. Varkuti et al. (2013) used BCI technology based on motor imagination to treat six patients with chronic stroke and found that five patients were significantly improved in Fugl-Meyer’s upper limb function score. Ang et al.’s (2011) study reported that the application of BCI as treatment of stroke patients can effectively improve the upper limb motor function of patients and can promote the rehabilitation of affected hand and wrist in the sequelae period.
However, the recovery mechanism of BCI on upper limb function after stroke and the effectiveness of BCI treatment are not clear. Besides, the formulation of exercise prescription for BCI treatment may affect treatment effect from many aspects (Rayegani et al., 2014). Therefore, we systematically reviewed the current status of the BCI technology and its application in upper limb dysfunction after stroke. Also, we discussed the efficacy and safety of BCI in treatment of patients after stroke.
The following electronic databases that include Chinese and English were searched from their inception up to July 2021. The Chinese databases contained China National Knowledge Infrastructure (CNKI), Wanfang Database, and Chinese Biology Medical disc (CBMdisc). Also, the English databases were as follows: the Cochrane Library, PubMed, EMBASE, and ScienceDirect. At the same time, this meta-analysis also manually searched the references cited in the relevant studies. The research subjects were not limited to Chinese and English. The search terms were as follows: (Brain-computer interface OR Brain-Machine interface OR BCI OR BMI) AND (stroke OR cerebral infarction OR cerebral hemorrhage OR cerebral vascular accident) AND (Randomized controlled trials OR RCTs).
We have established strict study criteria for inclusion and exclusion criteria based on study design principles, participants, interventions, and outcomes.
Randomized controlled trials (RCTs) of the effects of BCI on diseases of the central nervous system were first considered. Non-RCT comparative studies were excluded. In addition, case reports, conference papers, and reviews were also excluded. Some incomplete report data, unrelated to research, or unable to convert required data were part of exclusion criteria.
Patients clinically diagnosed as stroke was based on the standard diagnostic criteria. There were no restrictions on the age and sex of participants. Participants with significant cognitive, language or psychiatric illnesses, and unwillingness to participate in the experiment were excluded.
The studies contained an experimental group that was intervened by BCI or the combination of BCI with some conventional treatments, while the control group was treated by routine treatment or blank control or other treatment without BCI.
Studies that assessed upper limb function such as Fugl-Meyer Assessment for Upper Extremity (FMA-UE) Scale as the primary outcome measures were considered eligible. Other qualities of life and activities of daily living scales were included if they were relevant to the assessment of symptoms.
Two authors (YP and JW) independently assessed the titles and abstracts of articles retrieved and evaluated the full-text articles. The data from these articles were validated and extracted according to the following predefined criteria: author, year of publication, characteristics of participants, experimental intervention, control intervention, outcome measures, and adverse events. Any disagreement was resolved by a third party (PW).
To evaluate the quality of included studies, two authors independently assessed the risk of bias using Cochrane’s risk-of-bias tool (Varkuti et al., 2013). Specifically, each domain was judged as belonging to one out of three items: “low risk of bias,” “unclear risk of bias,” and “high risk of bias.”
If the eligible outcomes constituted dichotomous data, the relative risk (RR) was calculated, with 95% confidence intervals (CIs). For the clinical effects reported in included studies, the mean change scores and standard deviations (SDs) of the outcomes pre- and post-intervention were extracted for the meta-analyses, as well as the standardized mean difference (SMD), with 95% CIs, was calculated using RevMan 5.4. When there was not enough information about the SDs of the changes, the final values were imputed, as suggested by the Cochrane Handbook for Systematic Reviews of Interventions. The data were then pooled across studies using a random-effects model, with the assumption that each study was not homogeneous. All P-values < 0.05 were considered statistically significant. To assess heterogeneity, the chi-square test and Higgins I2 statistics were used; an I2 value of 50% or more was considered an indicator of significant heterogeneity. To determine the robustness of the meta-analysis results with a higher degree of certainty, a sensitivity analysis was implemented. Only studies that had a low risk of bias for random sequence generation and allocation concealment were analyzed.
A total of 762 potentially relevant studies were identified in the electronic databases. The other four additional studies were identified through other sources. After 253 articles were excluded due to duplication, 513 records were screened according to the title and author names. Based on titles and abstracts, 361 records were excluded because they did not meet the inclusion criteria, and 152 articles were read in full to establish eligibility. Among the full-text articles assessed, 87 were excluded. Ultimately, 65 studies were included in the qualitative synthesis, and only 16 studies achieved sufficient homogeneity in participants, interventions, and outcome measures for inclusion in the quantitative synthesis (Mihara et al., 2013; Li et al., 2014; Ang et al., 2015; Pichiorri et al., 2015; Jang et al., 2016; Kim et al., 2016; Shugeng et al., 2016; Biasiucci et al., 2018; Ying et al., 2018; Ramos-Murguialday et al., 2019; Chen et al., 2020; Hai and Zhiming, 2020; Lee et al., 2020; Sijie et al., 2020; Xianwen et al., 2020; Qiantuo and Tong, 2021). The remaining 49 studies had different outcome measures, improper participants, and duplicate data. Hence, we were unable to perform a meta-analysis on those studies. Figure 1 presents the flowchart of the trial selection process in terms of the Preferred Reporting Items for Systematic Reviews and Meta-Analyses (PRISMA; Cumpston et al., 2019).
A summary of included studies is presented in Table 1.
Table 1. Characteristics of the included clinical trials involving brain-computer interface for the treatment of central nervous system disease.
Figures 2, 3 demonstrate the risk of bias in the included studies.
Figure 3. Summary of the risk of bias: review authors’ judgments about each risk-of-bias item in each included study. “+,” low risk of bias; “?,” unclear risk of bias; “–,” high risk of bias.
In this study, all forest plots evaluated immediate effects after treatment.
Figures 4, 5 demonstrate the Fugl-Meyer Assessment (FMA) score.
Figure 4. Forest plot of the Fugl-Meyer Assessment (FMA) score to evaluate the effect of brain-computer interface (BCI) on upper limb dysfunction after stroke.
Figure 5. Forest plot of the FMA score after omitting Xianwen Xiang’s study to evaluate the effect of BCI on upper limb dysfunction after stroke.
Fifteen studies used the FMA to assess the effectiveness of BCI as an intervention to upper limb dysfunction after stroke (Figure 4). We found an SMD of 0.89 (95% CI: 0.23–1.55), meaning that the average FMA-UE score of the experimental group is separated by 89% of the pooled SD from the control group. But the result had a high heterogeneity (I2 = 90%).
Therefore, we conducted a sensitivity analysis by omitting one study in each term to assess the influence of individual studies on the pooled result. Finally, we removed Xianwen Xiang’s studies, which have a great impact on heterogeneity, and recalculated the combined estimate on the remaining studies (Figure 5). As a result, we demonstrated that BCI treatment rather than the control group had a significantly greater effect on upper limb recovery after stroke in the FMA-UE score (SMD: 0.53, 95% CI: 0.26–0.80, P = 0.0001). Also, the heterogeneity was not significant (I2 = 36%, P = 0.09).
Figure 6 demonstrates the Modified Barthel Index (MBI) score.
Figure 6. Forest plot of the Modified Barthel Index (MBI) score to evaluate the effect of BCI on upper limb dysfunction after stroke.
In seven studies that used the MBI scale, our meta-analysis showed that SMD = 1.67 with 95% CI: 0.61–2.74, I2 = 93% (Figure 6). Similarly, we conducted a sensitivity analysis, in which 1 study was removed at each time, which was performed to evaluate the stability of the results. But there was no apparent fluctuation. This analysis confirmed the stability of the results. According to the actual MBI scores in these studies, both experimental groups and control groups showed improvement after intervention in these seven studies. Furthermore, the experimental groups recovered more in terms of the MBI scale than the control groups.
Figure 7 demonstrates the Modified Ashworth Scale (MAS) score.
Figure 7. Modified Ashworth Scale (MAS) to evaluate the effect of BCI on upper limb dysfunction after stroke.
Six studies compared the effects of BCI treatment using the MAS score (Figure 7). The meta-analysis showed that the BCI intervention was not significantly superior to conventional treatment on the amount of use of their affected hand (SMD: −0.10, 95% CI: −0.50 to 0.30, P = 0.61). The heterogeneity was not significant (I2 = 26%). Regarding actual MAS scores, Pichiorri et al. (2015), Biasiucci et al. (2018), and Ramos-Murguialday et al. (2019) studies found an increase in MAS (i.e., increase in spasticity). Also, the last three studies showed a decrease in the MAS scores. Among these six studies, only Shugeng et al. (2016), Ramos-Murguialday et al. (2019), and Sijie et al. (2020) results showed that the BCI intervention was superior to conventional treatments. However, the analysis results are not conclusive due to the low number of studies. Therefore, it needs to be discussed and analyzed carefully.
This study aims to analyze the effectiveness of BCI in improving upper limb function after stroke. This updated meta-analysis included a total of 16 studies, which also included 488 patients. All the included studies showed that BCI played a positive role in promoting the upper limb rehabilitation of patients after stroke. In this study, only Li et al. (2014) indicated that subjects were allergic to electrode pads. Ang et al. (2015) indicated one case of shoulder joint pain. In addition, most studies did not mention adverse reactions that occurred.
Bai et al. (2020) conducted the meta-analysis investigating the immediate effect as well as the long-term effect of BCI and observed a positive and significant benefit of BCI with 18 single-group studies and 15 controlled studies. Another meta-analysis published by Kruse et al. (2020) indicated that BCI training enhanced brain function recovery and upper limb function after stroke by analyzing 14 RCTs. A total of 14 RCTs were included in these 2 meta-analyses. With 2 additional RCTs being included, our study updated the databases of the trial, and our study was able to investigate the specific performance of upper limb function in common scales such as FMA, MBI, and MAS after intervention.
The BCI repairs functional control connections between external limbs and the brain by rebuilding the cortex of the damaged brain. The process of neural recovery is an important basis for a variety of functions, and certain neuropathological changes are leading to different dysfunctions in many nervous system diseases. BCI has been verified to have a positive effect based on the functional mechanisms such as cortical excitability (Moher et al., 2009), cerebral plasticity, and functional connectivity (Daly and Wolpaw, 2008; Sawaki et al., 2014; Shimamura et al., 2017). Therefore, BCI is transforming to a rehabilitation method of upper limb dysfunction based on the principle of neuroplasticity.
The FMA of the upper limb included eight subitems, namely, reflex, flexor cooperative movement, extensor cooperative movement, activity with the cooperative movement, activity out of the cooperative movement, normal reflex, wrist joint stability, and hand movement and coordination ability and speed. The difference between the experimental group and the control group was statistically significant (P < 0.05) when the FMA score was used as the outcome measure in our study. Ang et al.’s (2015) study showed that the performance of the BCI group was slightly lower than that of the control group. But only 13% of repetitions in the BCI group compared with those in the control group, which may lead to this result. Therefore, we can conclude that safe and effective BCI intervention can improve the functional performance of upper limb dysfunction after stroke. However, one of the most significant difficulties during rehabilitation after stroke is the recovery of fine upper limb skills (Jacquin-Courtois, 2015). Shugeng et al.’s (2016) experiment showed that the functional improvement of patients was mainly reflected in the hand after BCI therapy. Besides, Mihara et al. (2013) performed the neurofeedback only affected in the distal part of the affected arm. However, Ang et al.’s (2015) and Kim et al.’s (2016) studies showed that the rehabilitation led to positive effects on shoulder and elbow. From another perspective, Mihara et al. (2013), Li et al. (2014), and Frolov et al. (2017) found that there was no significant effect on the Action Research Arm Test (ARAT) scores between BCI groups and control groups after intervention. However, Mihara et al. (2013) found that the cortical activation change was correlated with the recovery of the hand function, suggesting that the modulation of the excitability in the BCI and networks augments the functional recovery. Consistent with our findings, we cannot clarify that BCI has a better effect on improving the wrist in the upper limb after stroke, but improving the function of the whole upper limb is explicit. In addition, Ang et al. (2015) found that it is a greater improvement in the FMA scores at 4 weeks of BCI intervention during treatment and the follow-up time. Improvement in the FMA scores following BCI intervention was also demonstrated in Mane et al.’s (2019) study, which showed that the FMA scores improved better at the follow-up of 4 weeks. In most of the included experiments, the intervention time of BCI was mostly 4 weeks. The efficacy of a 4-week intervention with BCI was also demonstrated in the profile.
The ultimate goal of rehabilitation is to improve the patient’s quality of life and to return to society. The MBI scores (SMD = 1.67) were evaluated in seven studies. The results of the analysis showed that the BCI intervention in treatment of poststroke upper limb dysfunction has improved the quality of life of patients and their ability to take care of themselves at the same time, which was consistent with Lin’s finding (Lin et al., 2021).
This meta-analysis may have some heterogeneity in methodological quality. It is because some studies did not mention the details of these patients with stroke, such as stroke location and so on. There are also some differences in the outcome indicators of each study. In addition, to study the best treatment of BCI in improving dysfunction, it is necessary to expand the sample size and conduct the long-term follow-up for further analysis to determine the clinical value of BCI.
Our updated meta-analysis with 16 RCTs indicated that BCI therapy or combined with other therapies such as routine rehabilitation training had favorable effects on upper limb function recovery after stroke. These findings also suggest that intervention duration for 4 weeks had the largest effect size. We also observed that BCI is a safe neuromodulator intervention to improve upper limb functions in patients with stroke.
The original contributions presented in the study are included in the article/supplementary material, further inquiries can be directed to the corresponding authors.
HL and PW designed the study. YP and JW negotiated article methodology and executed search strategy. XW managed the search results of the database. YP and ZL performed the data analysis. LZ and XG provided suggestions for the revision of the manuscript. All authors contributed to screening procedures, data curation, and additional supplementary material.
The authors declare that the research was conducted in the absence of any commercial or financial relationships that could be construed as a potential conflict of interest.
All claims expressed in this article are solely those of the authors and do not necessarily represent those of their affiliated organizations, or those of the publisher, the editors and the reviewers. Any product that may be evaluated in this article, or claim that may be made by its manufacturer, is not guaranteed or endorsed by the publisher.
We would like to thank the research authors included in this systematic review for their support and contribution to this article.
Ang, K. K., Chua, K. S., Phua, K. S., Wang, C., Chin, Z. Y., Kuah, C. W., et al. (2015). Trial of EEG-based motor imagery brain-computer interface robotic rehabilitation for stroke. Clin. EEG Neurosci. 46, 310–320. doi: 10.1177/1550059414522229
Ang, K. K., Guan, C., Chua, K. S., Ang, B. T., Kuah, C. W., Wang, C., et al. (2011). A large clinical study on the ability of stroke patients to use an EEG-based motor imagery brain-computer interface. Clin. EEG Neurosci. 42, 253–258. doi: 10.1177/155005941104200411
Bai, Z., Fong, K. N. K., Zhang, J. J., Chan, J., and Ting, K. H. (2020). Immediate and long-term effects of BCI-based rehabilitation of the upper extremity after stroke: a systematic review and meta-analysis. J. NeuroengRehabil. 17:57. doi: 10.1186/s12984-020-00686-2
Biasiucci, A., Leeb, R., Iturrate, I., Perdikis, S., Al-Khodairy, A., Corbet, T., et al. (2018). Brain-actuated functional electrical stimulation elicits lasting arm motor recovery after stroke. Nat. Commun. 9:2421. doi: 10.1038/s41467-018-04673-z
Bolognini, N., Vallar, G., Casati, C., Latif, L. A., El-Nazer, R., Williams, J., et al. (2011). Neurophysiological and behavioral effects of tDCS combined with constraint-induced movement therapy in poststroke patients. Neurorehabil. Neural. Repair. 25, 819–829. doi: 10.1177/1545968311411056
Chen, S., Cao, L., Shu, X., Wang, H., Ding, L., Wang, S. H., et al. (2020). Longitudinal electroencephalography analysis in subacute stroke patients during intervention of brain-computer interface with exoskeleton feedback. Front. Neurosci. 14:809. doi: 10.3389/fnins.2020.00809
Cumpston, M., Li, T., Page, M. J., Chandler, J., Welch, V. A., Higgins, J. P., et al. (2019). Updated guidance for trusted systematic reviews: a new edition of the cochrane handbook for systematic reviews of interventions. Cochrane. Database Syst. Rev. 10:142. doi: 10.1002/14651858.ED000142
Daly, J. J., and Wolpaw, J. R. (2008). Brain–computer interfaces in neurological rehabilitation. Lancet Neurol. 7, 1032–1043. doi: 10.1016/S1474-4422(08)70223-0
Feigin, V. L., Forouzanfar, M. H., Krishnamurthi, R., Mensah, G. A., Connor, M., and Bennett, D. A. (2014). Global and regional burden of stroke during 1990–2010: findings from the global burden of disease study 2010. Lancet 383, 245–254.
Frolov, A. A., Mokienko, O., Lyukmanov, R., Biryukova, E., Kotov, S., Turbina, L., et al. (2017). Post-stroke rehabilitation training with a motor-imagery-based brain-computer interface (BCI)-Controlled hand exoskeleton: a randomized controlled multicenter trial. Front. Neurosci. 11:400. doi: 10.3389/fnins.2017.00400
Hai, R., and Zhiming, X. (2020). Effect of Brain-Computer Interface technology on upper limb motor function rehabilitation for stroke patients. China Pract. Med. 15, 181–183.
Jacquin-Courtois, S. (2015). Hemi-spatial neglect rehabilitation using non-invasive brain stimulation: or how to modulate the disconnection syndrome? Ann. PhysRehabil. Med. 58, 251–258. doi: 10.1016/j.rehab.2015.07.388
Jang, Y. Y., Kim, T. H., and Lee, B. H. (2016). Effects of brain-computer interface-controlled functional electrical stimulation training on shoulder subluxation for patients with stroke: a randomized controlled trial. OccupTherInt 23, 175–185. doi: 10.1002/oti.1422
Kim, T., Kim, S., and Lee, B. (2016). Effects of action observational training plus brain-computer interface-based functional electrical stimulation on paretic arm motor recovery in patient with stroke: a randomized controlled trial. OccupTherInt 23, 39–47. doi: 10.1002/oti.1403
Kruse, A., Suica, Z., Taeymans, J., and Schuster-Amft, C. (2020). Effect of brain-computer interface training based on non-invasive electroencephalography using motor imagery on functional recovery after stroke - a systematic review and meta-analysis. BMC Neurol. 20:385. doi: 10.1186/s12883-020-01960-5
Lee, S. H., Kim, S. S., and Lee, B. H. (2020). Action observation training and brain-computer interface controlled functional electrical stimulation enhance upper extremity performance and cortical activation in patients with stroke: a randomized controlled trial. Physiother. Theory Pract. 2020, 1–9. doi: 10.1080/09593985.2020.1831114
Li, M., Liu, Y., Wu, Y., Liu, S., Jia, J., and Zhang, L. (2014). Neurophysiological substrates of stroke patients with motor imagery-based Brain-Computer Interface training. Int. J. Neurosci. 124, 403–415. doi: 10.3109/00207454.2013.850082
Lin, C., Martin, K., Arevalo, Y. A., Harvey, R. L., and Prabhakaran, S. (2021). Association of proportional recovery after stroke with health-related quality of life. Stroke 52, 2968–2971. doi: 10.1161/STROKEAHA.120.033672
Lopez-Larraz, E., Sarasola-Sanz, A., Irastorza-Landa, N., Birbaumer, N., and Ramos-Murguialday, A. (2018). Brain-machine interfaces for rehabilitation in stroke: a review. NeuroRehabilitation 43, 77–97. doi: 10.3233/NRE-172394
Mane, R., Chew, E., Phua, K. S., Ang, K. K., Robinson, N., Vinod, A. P., et al. (2019). Prognostic and monitory EEG-Biomarkers for BCI upper-limb stroke rehabilitation. IEEE Trans. Neural. SystRehabilEng. 27, 1654–1664. doi: 10.1109/TNSRE.2019.2924742
Mihara, M., Hattori, N., Hatakenaka, M., Yagura, H., Kawano, T., Hino, T., et al. (2013). Near-infrared spectroscopy-mediated neurofeedback enhances efficacy of motor imagery-based training in poststroke victims: a pilot study. Stroke 44, 1091–1098. doi: 10.1161/STROKEAHA.111.674507
Moher, D., Liberati, A., Tetzlaff, J., Altman, D. G., and Group, P. (2009). Preferred reporting items for systematic reviews and meta-analyses: the PRISMA statement. PLoS Med 6:e1000097.
Montenegro, R. A., Midgley, A., Massaferri, R., Bernardes, W., Okano, A. H., and Farinatti, P. (2016). Bihemispheric motor cortex transcranial direct current stimulation improves force steadiness in post-stroke hemiparetic patients: a randomized crossover controlled trial. Front. Hum. Neurosci. 10:426. doi: 10.3389/fnhum.2016.00426
Pichiorri, F., Morone, G., Petti, M., Toppi, J., Pisotta, I., Molinari, M., et al. (2015). Brain-computer interface boosts motor imagery practice during stroke recovery. Ann. Neurol. 77, 851–865. doi: 10.1002/ana.24390
Qiantuo, T., and Tong, Z. (2021). Rehabilitation effect of Functional Electrical Stimulation controlled by Brain-Computer Interface on upper limb dysfunction in stroke patients. Chin. J. Rehab. Theory Pract. 27, 802–806.
Ramos-Murguialday, A., Curado, M. R., Broetz, D., Yilmaz, O., Brasil, F. L., Liberati, G., et al. (2019). Brain-machine interface in chronic stroke: randomized trial long-term follow-up. Neurorehabil. Neural. Repair. 33, 188–198. doi: 10.1177/1545968319827573
Rayegani, S. M., Raeissadat, S. A., Sedighipour, L., Rezazadeh, I. M., Bahrami, M. H., Eliaspour, D., et al. (2014). Effect of neurofeedback and electromyographic-biofeedback therapy on improving hand function in stroke patients. Top Stroke Rehabil. 21, 137–151. doi: 10.1310/tsr2102-137
Sawaki, L., Butler, A. J., Leng, X., Wassenaar, P. A., Mohammad, Y. M., Blanton, S., et al. (2014). Differential patterns of cortical reorganization following constraint-induced movement therapy during early and late period after stroke: a preliminary study. NeuroRehabilitation 35, 415–426. doi: 10.3233/NRE-141132
Shimamura, N., Katagai, T., Kakuta, K., Matsuda, N., Katayama, K., Fujiwara, N., et al. (2017). Rehabilitation and the neural network after stroke. Transl. Stroke Res. 8, 507–514.
Shugeng, C., Xiaokang, S., and Jie, J. (2016). Research on hand function rehabilitation of stroke patients based on closed-loop brain computer interface. Chin. J. Rehabil. Med. 31, 1189–1194.
Sijie, L., Yulian, Z., Weining, W., and Min, Z. (2020). Application of brain computer interface technology in the rehabilitation of upper limb dysfunction in stroke patients. Clin. Res. 35, 185–188.
Spuler, M., Lopez-Larraz, E., and Ramos-Murguialday, A. (2018). On the design of EEG-based movement decoders for completely paralyzed stroke patients. J. NeuroengRehabil. 15:110. doi: 10.1186/s12984-018-0438-z
Van de Port, I. G., Wevers, L., Roelse, H., van Kats, L., Lindeman, E., and Kwakkel, G. (2009). Cost-effectiveness of a structured progressive task-oriented circuit class training programme to enhance walking competency after stroke: the protocol of the FIT-Stroke trial. BMC Neurol. 9:43. doi: 10.1186/1471-2377-9-43
Varkuti, B., Guan, C., Pan, Y., Phua, K. S., Ang, K. K., Kuah, C. W., et al. (2013). Resting state changes in functional connectivity correlate with movement recovery for BCI and robot-assisted upper-extremity training after stroke. Neurorehabil. Neural. Repair. 27, 53–62. doi: 10.1177/1545968312445910
Wang, W., Collinger, J. L., Perez, M. A., Tyler-Kabara, E. C., Cohen, L. G., Birbaumer, N., et al. (2010). Neural interface technology for rehabilitation: exploiting and promoting neuroplasticity. Phys. Med. RehabilClin. N Am. 21, 157–178. doi: 10.1016/j.pmr.2009.07.003
Xianwen, X., Jiaying, Z., Yuanbiao, S., and Fengyou, S. (2020). Clinical study of Brain-Computer Interface in the treatment of upper limb dysfunction in the convalescent stage of ischemic stroke. Med. Innov. China 17, 154–158.
Keywords: stroke, brain-computer interface, BCI, upper limb dysfunction, meta-analysis
Citation: Peng Y, Wang J, Liu ZC, Zhong LD, Wen X, Wang P, Gong XQ and Liu HY (2022) The Application of Brain-Computer Interface in Upper Limb Dysfunction After Stroke: A Systematic Review and Meta-Analysis of Randomized Controlled Trials. Front. Hum. Neurosci. 16:798883. doi: 10.3389/fnhum.2022.798883
Received: 20 October 2021; Accepted: 17 February 2022;
Published: 29 March 2022.
Edited by:
Do-Won Kim, Chonnam National University, South KoreaCopyright © 2022 Peng, Wang, Liu, Zhong, Wen, Wang, Gong and Liu. This is an open-access article distributed under the terms of the Creative Commons Attribution License (CC BY). The use, distribution or reproduction in other forums is permitted, provided the original author(s) and the copyright owner(s) are credited and that the original publication in this journal is cited, in accordance with accepted academic practice. No use, distribution or reproduction is permitted which does not comply with these terms.
*Correspondence: Pu Wang, d2FuZ3B1XzAzQDEyNi5jb20=; Xiaoqian Gong, MTM4MjYzODA2NzhAMTM5LmNvbQ==; Huiyu Liu, bGl1aHVpeXVkb2N0b3JAc29odS5jb20=
†These authors have contributed equally to this work
Disclaimer: All claims expressed in this article are solely those of the authors and do not necessarily represent those of their affiliated organizations, or those of the publisher, the editors and the reviewers. Any product that may be evaluated in this article or claim that may be made by its manufacturer is not guaranteed or endorsed by the publisher.
Research integrity at Frontiers
Learn more about the work of our research integrity team to safeguard the quality of each article we publish.