- 1Department of Neurology With Experimental Neurology, Charité–Universitätsmedizin Berlin, Berlin, Germany
- 2Control Systems Group, Technische Universität Berlin, Berlin, Germany
- 3Berlin School of Mind and Brain, Charité–Universitätsmedizin Berlin, Berlin, Germany
- 4NeuroCure Clinical Research Centre, Charité–Universitätsmedizin Berlin, Berlin, Germany
- 5German Center for Neurodegenerative Diseases, DZNE, Berlin, Germany
The understanding of locomotion in neurological disorders requires technologies for quantitative gait analysis. Numerous modalities are available today to objectively capture spatiotemporal gait and postural control features. Nevertheless, many obstacles prevent the application of these technologies to their full potential in neurological research and especially clinical practice. These include the required expert knowledge, time for data collection, and missing standards for data analysis and reporting. Here, we provide a technological review of wearable and vision-based portable motion analysis tools that emerged in the last decade with recent applications in neurological disorders such as Parkinson's disease and Multiple Sclerosis. The goal is to enable the reader to understand the available technologies with their individual strengths and limitations in order to make an informed decision for own investigations and clinical applications. We foresee that ongoing developments toward user-friendly automated devices will allow for closed-loop applications, long-term monitoring, and telemedical consulting in real-life environments.
1. Introduction
The widespread application of technologies for gait analysis has contributed greatly to our current understanding of healthy and pathological locomotion (Celik et al., 2021). On one hand, instrumented gait analysis complements the quantification of long-established clinical scales [e.g., Berg Balance Scale (Berg et al., 1989), Timed-up-and-go test (Podsiadlo and Richardson, 1991)] and patient self-reports [e.g., Freezing of Gait Questionnaire (Giladi et al., 2000)]. On the other hand, portable technologies for gait analysis may improve diagnosis, follow-up, and treatment of gait disorders through continuous monitoring in activities of daily living (Tzallas et al., 2014; Filli et al., 2018; Ancona et al., 2021). In concert with functional neuroimaging and neuromodulation, gait analysis technologies can enhance our knowledge of healthy and pathological gait function (Maetzler et al., 2009; Artusi et al., 2018; Buckley et al., 2019).
Gait and postural control disorders in the context of neurological diseases, such as Parkinson's Disease (PD) and Multiple Sclerosis (MS), have an immense impact on affected people's quality of life (Snijders et al., 2007). Parkinson's Disease is the second most common neurodegenerative disease in the elderly in Europe (Deuschl et al., 2020). Patients frequently suffer from slow movements (bradykinesia), pathological gait patterns including reduced step length and freezing of gait (FoG) (Nutt et al., 2011), as well as difficulties in postural control (Schlenstedt et al., 2016). Numerous publications have shown that gait parameters extracted with optical motion capturing, force plates, or inertial sensors correlate with clinical assessments of disease severity and levodopa responsiveness in PD (Horak and Mancini, 2013). In patients with MS, leg paresis, loss of coordination, and spasticity often manifest as gait dysfunction and changes in balance control (Comber et al., 2017). MS related gait and balance impairments can occur at very early disease stages, however, so minor that they may be difficult to see with the bare eye (Kieseier and Pozzilli, 2012). Advanced movement analysis techniques can measure these subtle changes and could be used to identify the risk of mobility loss (Shanahan et al., 2018). In this narrative review on gait analysis technologies in neurological disorders, we will discuss PD and MS as illustrative examples because of their distinct gait characteristics and clinical relevance in different age groups.
Recently, there has been a growing research interest in contact-free human motion tracking with available clinical equipment (e.g., standard camera) using machine learning (ML). Telemedical patient care options play an increasing role in times of global pandemics (Sibley et al., 2021). Therefore, the literature published on the topic of reliable, easily accessible, and easy to use measurement systems for gait and balance analysis is extensive and can be overwhelming. This narrative review aims at building a technical understanding of emerging portable gait analysis technologies for neurological disorders, which can be classified into non-wearable and wearable devices (wearables) (de-la Herran et al., 2014). Our goal is to enable the reader to understand the strengths and limitations of available technologies and thereby support decision-making for planning applications in research and diagnostics. After briefly introducing gait and postural control measures, wearable and recent non-wearable systems from the last decade are discussed in detail for their functionality, usefulness, and usability in practice. Future applications and trends are identified.
2. Measures of Gait and Postural Control
2.1. Gait Measures
Gait results from cyclical limb movement. For its analysis, parameters are often defined in the dimensions time and space, as illustrated in Figure 1. Despite the two displayed main phases, stance phase and swing phase, the gait cycle can be divided into up to eight phases with regard to leg position, foot position, and load (namely initial contact, loading response, mid stance, terminal stance, pre-swing, initial swing, mid swing, and terminal swing) (Taborri et al., 2016). Resulting spatiotemporal features such as gait cycle and gait velocity are commonly expressed as the average of several strides. Dynamic features of gait represent the stride-to-stride variability of these measures in the form of intra-subject standard deviation or coefficient of variation (Lord et al., 2013; Buckley et al., 2019). Further variables can be extracted such as parameters in the frequency domain or sub-task-specific parameters, e.g., the rotational velocity of turns (Horak and Mancini, 2013). The evaluation of sub-tasks in standard clinical tests may be relevant for investigating specific symptoms. For example, sequential tasks of turning and passing through narrow doors have been designed to provoke episodic FoG symptom in PD (Ziegler et al., 2010; Reches et al., 2020). In addition, kinematic parameters such as knee joint angles or range of motion (ROM) as well as kinetic parameters such as ground reaction forces (GRF) can be extracted from certain portable systems to monitor disease progression (Baker, 2013; Veeraragavan et al., 2020). A list of frequently reported gait and balance parameters is presented in Table 1.
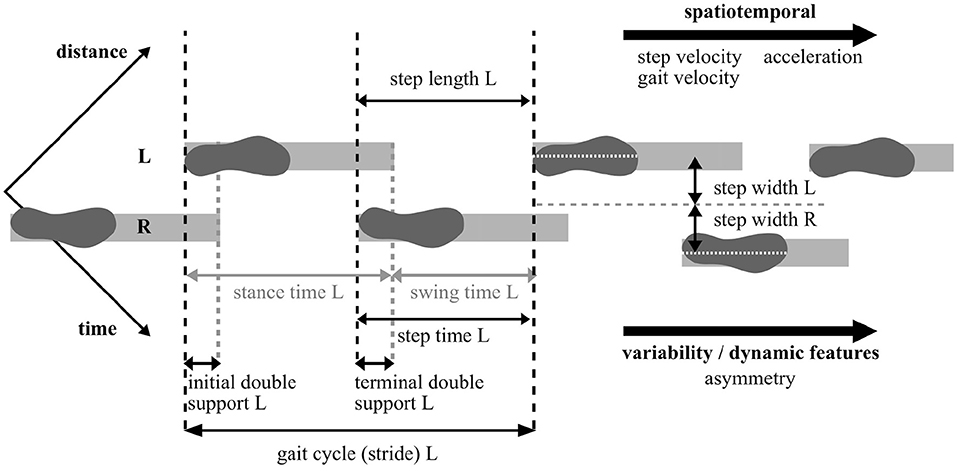
Figure 1. Illustration of basic spatiotemporal and dynamic gait parameter definitions. Note that the footprint indicates the heel strike event. L, Left foot; R, Right foot.
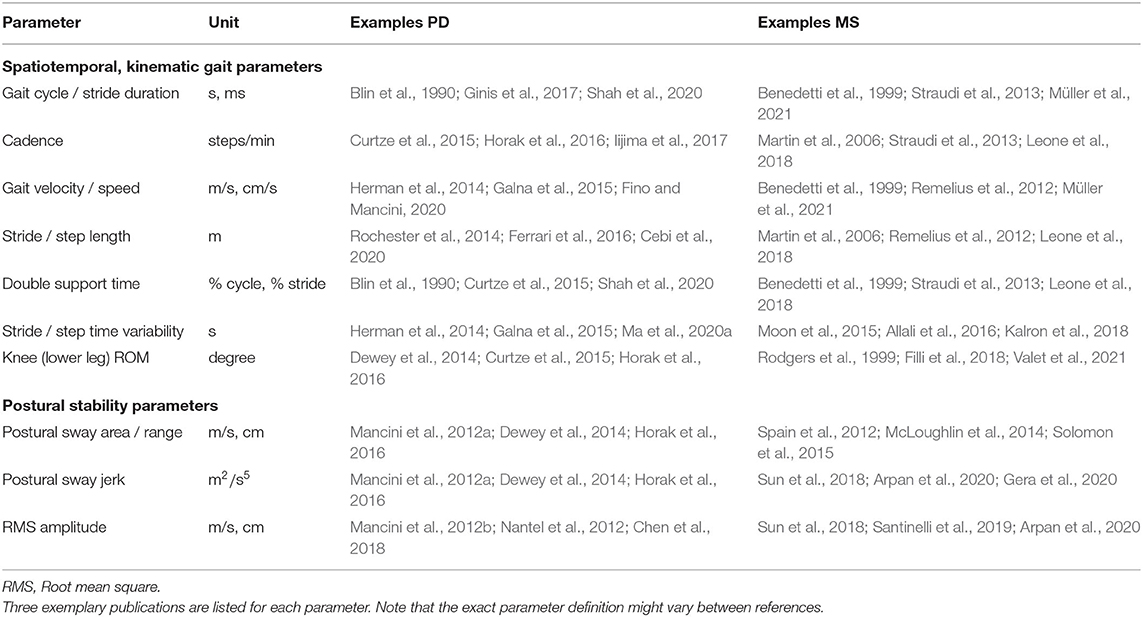
Table 1. Examples of commonly derived measures of gait and postural control from instrumented analysis technologies.
With the high amount of redundant and covariant available parameters from instrumented gait analysis, methods have been suggested to summarize parameters for better interpretation: For example, the Gait Variability Index (GVI) was introduced by Gouelle et al. (2013) combining nine weighted gait parameters based on results of a principal component analysis (PCA) in comparison with a reference group. Morris et al. (2017) proposed a model of unrestricted gait based on data from wearables on 103 elderly controls and 67 PD patients. Four gait domains were derived from 14 gait parameters by applying a PCA: pace, rhythm, variability, and asymmetry. Further models are summarized in Celik et al. (2021). Although simplifying the complexities of instrumented gait assessment would be helpful, the prevalence of these higher-order parameters in clinical trials has been low to date, possibly due to the complexity of their analysis and interpretation.
Since the number of kinematic gait analysis technologies has grown excessively in recent years, we focus on the assessment of kinematic parameters in this review. However, additional investigations of the phasic contribution of muscles in a gait cycle can be obtained from surface electromyography (EMG), integrated into many studies on human locomotion and neurological disease characteristics (e.g. Winter, 1989; Mickelborough et al., 2004; Gnther et al., 2019; Cofré Lizama et al., 2020). A detailed overview of standardized clinical tasks and protocols for the assessment of gait, such as the timed 10m walking test or the timed-up-and-go test (TUG), can be found in Graham et al. (2008) or de-la Herran et al. (2014).
2.2. Balance Measures
Depending on the measurement modality, either the center of pressure (COP) or the center of mass (COM) is tracked in balance assessments during standing in different conditions (Buckley et al., 2019). Often utilized conditions are standing on hard surfaces vs. foam surfaces [modified clinical test of sensory interaction on balance (Horn et al., 2015)] or eyes-open vs. eyes-closed. Multiple parameters are determined describing the displacement of COP or COM over a defined amount of time as illustrated in Figure 2. Postural sway captures the horizontal acceleration of the person's center in all directions, most often in the mediolateral and anterior-posterior planes. Typically, sway area, sway range, sway velocity, and jerk, defined as the smoothness of the trunk sway (rate of change), are extracted and analyzed regarding asymmetry and variability between different conditions (Martinez-Mendez et al., 2012).
Balance and gait may represent independent domains of mobility in neurological diseases (Horak et al., 2016). Thus, no single measure of either balance or gait can fully characterize mobility impairments, although gait parameters facilitate statements on the balance capabilities of a person. Longer stance phases, expanded step width and deviations from walking a straight line were reported in people with balance disorders (Spain et al., 2014; Diaz et al., 2020).
2.3. Measures/Biomarkers in PD
Parkinsonian gait differs from the gait of the healthy elderly even in the early stages of the disease as revealed by kinematic measures. Galna et al. (2015) found an impairment across the gait domains pace, variability, rhythm, asymmetry, and postural control in recently diagnosed PD patients compared to age-matched healthy controls. PD patients walked at a slower pace, with decreased step length, and showed increased asymmetry and step-to-step variability. Others reported a set of 20 gait kinematic variables, such as stride length or gait velocity, that differentiates parkinsonian gait from the gait of controls, and a set of variables correlating with symptom severity, potentially serving as markers of PD progression (Dewey et al., 2014). Recently, Ghislieri et al. (2021) used foot-switch sensors to assess gait parameters in PD patients and age-matched controls during walking and reported a 42%-increase in atypical gait cycles in PD, which correlated with motor symptom severity1. Veeraragavan et al. (2020) showed that a neural network approach with features extracted from the vertical ground reaction force can differentiate PD from controls as well as predict disease severity (Hoehn & Yahr stage). Furthermore, postural instability is increased in early PD and deteriorates within 12 months of diagnosis, thus providing a potential marker for motor function decline (Mancini et al., 2011, 2012a). Increased gait variability and sagittal trunk movement might predict an increased risk of falls (Ma et al., 2020a). Yet, no standardized set of gait kinematic biomarkers that signifies gait improvement in PD exists (Horak and Mancini, 2013).
2.4. Measures/Biomarkers in MS
Differences in gait and balance parameters between neurologically intact controls and MS patients were reported (Shanahan et al., 2018): Reduced gait speed and stride length, a prolonged double support time, as well as changes in kinematic characteristics of the hip, knee, and ankle joint were found to correlate with disease severity in patients with relapsing-remitting, primary or secondary progressive MS with no to moderate impairments2 (Benedetti et al., 1999; Martin et al., 2006; Kelleher et al., 2010; Remelius et al., 2012). Additionally, a relationship between a reduced dorsiflexion angle at initial contact and walking induced fatigue as well as an increased power absorption at the hip, knee, and ankle have been reported in MS patients with moderate disabilities (EDSS 3–6) (McLoughlin et al., 2016). Studies on balance in MS patients with mild to moderate impairments (EDSS 0–5.5) showed an increased mediolateral sway path length, mediolateral sway range (Solomon et al., 2015), and sway area (Spain et al., 2014). This is also reflected by a wider stride width in patients with EDSS 2.5–6 compared to controls (Remelius et al., 2012). Clinical tests including turns were recommended to reveal important markers of balance confidence and walking abilities in MS (Adusumilli et al., 2018).
3. Wearable Technologies
3.1. Inertial Sensors
3.1.1. Technology
The progress in micro-electromechanical system (MEMS) technology resulted in the availability of small, lightweight, and low-cost inertial measurement units (IMUs) conquering the motion tracking market (Seel et al., 2020). Therefore, IMUs are the most widely used type of wearable sensors for gait and balance analysis (de-la Herran et al., 2014; Shanahan et al., 2018); the quantification of gait with IMUs is sometimes referred to as InertiaLocoGraphy (ILG) (Vienne-Jumeau et al., 2020). IMUs typically consist of a combination of multi-dimensional gyroscopes, accelerometers, and often magnetometer sensors allowing the estimation of joint angles, gait and angular velocities, position and orientation in space via sensor fusion techniques (Sabatini, 2006). The wireless sensors can be mounted on various parts of the patient's body, for example, foot, lower leg, pelvis, torso, or integrated into garments and insoles, in order to measure movements of a specific body segment.
Accelerometers are most commonly used in motion analysis and assess the one-, two-, or three-dimensional acceleration of the sensor in terms of externally applied acceleration forces (Diaz et al., 2020). The measured signal is the sum of (1) the linear acceleration, namely the translation- and/or rotation-related instantaneous change of velocity, and (2) the earth's gravitational acceleration, which is approximately 9.81 m/s2 in vertical direction near the earth's surface. However, these two components can only be differentiated completely in quasi-stationary scenarios.
Gyroscopes provide the one-, two-, or three-dimensional angular velocities of the body segment to which they are attached. The design typically relies on the Coriolis effect whereby a body moving freely in a rotating frame of reference experiences the Coriolis force acting perpendicular to the direction of applied motion and to the axis of rotation. Segment orientations and joint angles can be determined by integration of the resulting angular rates if initial values are known and measurement biases are removed. However, the biases of MEMS-based gyroscopes are temperature-dependent and time-varying, which makes it difficult to estimate them during movements (Woodman, 2007).
To overcome the disadvantages of both sensor types, accelerometers and gyroscopes, magnetometers are often included in IMUs [also referred to as magneto inertial measurement units (MIMUs)] to improve orientation measurements, namely heading. Heading describes the angle of the sensor with respect to the horizontal direction of the magnetic north. In a magnetically undisturbed environment, magnetometers measure this component and a vertical component of the local earth's magnetic field. However, these readings are typically noisy and affected by magnetic disturbances originating from objects containing ferromagnetic material or emitting magnetic fields as usually the case in indoor environments (Schauer, 2017).
As the measurement signals of inertial sensors are well-known to be subject to errors such as time-variant sensor biases and measurement noise, reliable motion tracking requires computationally complex algorithms including state estimation methods and kinematic models (Seel et al., 2020). Three-dimensional strap-down integration and suitable sensor fusion algorithms combining the different signals are applied to estimate the real-time orientation of an attached IMU (Schauer, 2017), as illustrated in Figure 3. More precisely, the orientation of the inertial coordinate system, which is aligned with the housing of the sensor, is estimated with respect to a three-dimensional inertial reference coordinate system. The accuracy and precision of a wearable sensor system depend on how many sensors are used, where and how the sensors are mounted, and on the utilized algorithms. For example, the sensor coordinate systems should be sufficiently well-aligned with a meaningful coordinate system of the body part of interest, to which the sensor is attached, or methods for non-restrictive sensor-to-segment calibration or automatic anatomical calibration should be applied (Seel et al., 2014b).
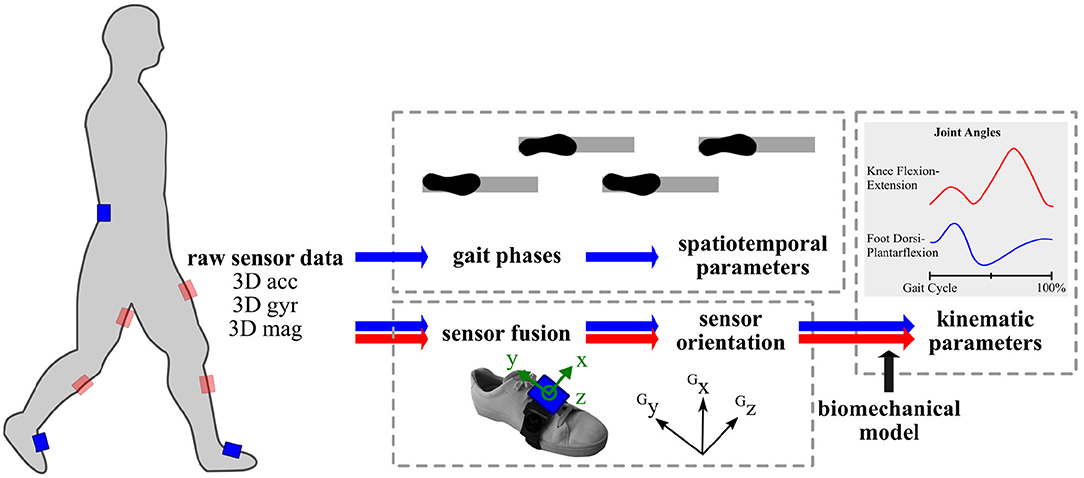
Figure 3. Methods overview for instrumented gait analysis with inertial sensors in commonly used positions on pelvis and lower limbs. acc, accelerometer readings; G, Global coordinate system; gyr, gyroscope readings; mag, magnetometer readings.
For clinical gait data analysis, further mathematical tools are required to extract spatiotemporal gait parameters and often anatomical models are utilized for extracting kinematic parameters. Commonly in a two-stage approach, first gait events and phases are detected and, secondly, spatial parameters are determined. Various approaches exist on how and to what detail gait phases are detected from IMU recordings. Reliable gait detection can be achieved by exploiting the angular rates from the gyroscopes (Bertoli et al., 2018) or by combining them with the accelerometer measurements using peak detection algorithms (Mariani et al., 2013). Automated methods deploy adaptive thresholds based on the subject's walking style (Bejarano et al., 2014; Seel et al., 2014a). Spatial parameters are obtained by either signal integration, kinematic gait models, or ML techniques (Yang and Li, 2012; Caldas et al., 2017). Major gait parameters, for example, stride length, walking speed, can be derived with the most commonly used setup of two inertial sensors that are placed on the feet/shoes (e.g., Schlachetzki et al., 2017) or on the shank. Especially when postural control and balance parameters shall be extracted, a third sensor is added either on the chest, pelvis or lumbar spine and the acceleration is used to calculate COM and sway parameters (Mancini et al., 2012b; Curtze et al., 2015; Hsieh and Sosnoff, 2021). Recently, it has been shown that several gait events can be obtained from a single IMU at the pelvis even in individuals with neurological conditions (Pham et al., 2017). Tracking of the lower body or full body motion tracking is facilitated by further sensors (e.g., Schepers et al., 2018; Teufl et al., 2019).
Available commercial systems for gait and balance analysis applied in neurological patients are, for example, Xsens MVN (Xsens Technologies B.V., Enschede, Netherlands) for full-body motion tracking with up to 17 sensors, Mobility Lab (APDM, Inc., Portland, OR, USA) with six IMUs and data analysis software for different test scenarios (Dewey et al., 2014; Mancini and Horak, 2016; Morris et al., 2019), or RehaGait (HASOMED, Magdeburg, Germany) with up to seven inertial sensors and gait data analysis software (Donath et al., 2016). The sensors and systems diverge in their software, namely algorithms for sensor fusion and parameter estimation, as well as in their communication and housing. The housing and its dimensions vary depending on the battery capacities and on-sensor storage (Diaz et al., 2020). Furthermore, different data collection modes are available, such as real-time streaming or post-recording data download, and their transmission to a computer or smart device. The provided sampling rates correlate with the number of utilized sensors and show a large range (22–320 Hz), although this parameter has a high impact on the accuracy (Caldas et al., 2017). Besides, the pricing for IMU-based gait analysis systems varies strongly with individual wireless inertial sensor being available at affordable prices. However, the more detailed the gait analysis software has been evaluated, the more expensive it is. This is one reason for the trend toward own investigations and open-source gait analysis software (e.g., Gurchiek et al., 2019). Therefore, before deciding on a sensor system, the complete application scenario and budget should be outlined and recent developments need to be taken into account.
3.1.2. Applications
Inertial sensors in PD. Inertial sensor technology applied in PD was able to reproduce the findings of distinct spatiotemporal gait characteristics including short steps, shuffling gait, and postural instability, specific for different disease stages and levels of motor impairment (Schlachetzki et al., 2017); cf. Section 2.3. A typical application is the instrumentalization of established clinical tests with IMUs with the goal of making the assessment rater-independent and gaining additional information (Palmerini et al., 2013). For example, Dewey et al. (2014) used the Mobility Lab system in an instrumented TUG and instrumented sway assessment in 135 PD subjects and 66 age-matched controls. For both tests, they identified multiple variables (e.g., stride length, turn duration, total sway area) that correlate with PD severity measures and differentiate PD subjects from controls. Instrumented gait analysis with IMUs offers the possibility to examine the differential effects of established and novel PD treatments on gait. A selection of exemplary studies is presented in Table 2.
For instance, Curtze et al. (2015) studied the effect of levodopa treatment on gait in a large cohort of patients and found that pace-related gait measures responded well to levodopa treatment, while balance parameters did not improve in the ON- compared to the OFF-state. Iijima et al. (2017) used 24 h single-accelerometer measurements from the trunk in order to track improvements in the gait fluctuations of PD patients after the addition/increase in dose of selegiline, showing a higher sensitivity than clinical scores. Recently, Cebi et al. (2020) used gait kinematics derived from IMU sensors placed at the hip and ankles to examine the therapeutic outcome of deep brain stimulation of the Nucleus subthalamicus (STN-DBS) on gait disorders in PD. Time to complete a 7 m walking task and number of steps were reduced and gait kinematics improved (stride length, ROM) 8 weeks after STN-DBS surgery in the DBS-ON compared to the DBS-OFF condition. In addition, freezers with a pre-surgical levodopa response of gait kinematics responded better to STN-DBS, indicating that the assessment with IMUss might be useful to predict the outcome of such treatments in specific patient subgroups.
Another popular application of IMUs in PD is as a tool to recognize and quantify FoG, a symptom which is rarely observed during clinical consultations since it occurs episodically. Freezing of gait usually appears in everyday life situations, i.e., during turning or walking through narrow doorways, and is associated with an increased risk of falls (Gray and Hildebrand, 2000; Bloem et al., 2004). Various sensor-based methods have been developed to objectively measure FoG in terms of number of episodes and episode duration (Moore et al., 2008; Rodrguez-Martn et al., 2017; Silva de Lima et al., 2017; Suppa et al., 2017; Pardoel et al., 2019). Sensor-based FoG detection opens up the possibility of monitoring FoG in the home environment of patients, which could facilitate the diagnosis and treatment of FoG (Suppa et al., 2017; Mancini et al., 2021).
Furthermore, IMUs are integrated in novel therapeutic cueing devices, which aim to monitor and treat gait disorders in PD. Cueing was shown to be effective in improving gait function in PD and a multitude of cueing paradigms exists (Muthukrishnan et al., 2019). Table 2 includes examples of cueing devices using IMUs to either administer gait-synchronized cues or to analyze the gait pattern in response to treatment. For example, the gait training tool CuPiD-system consists of wearable IMUs, a smartphone, and headphones to deliver intelligent auditory feedback on gait (Casamassima et al., 2014; Ferrari et al., 2016). Patients using the device showed improvements in maintaining cadence during prolonged walking, improved balance, and quality of life (Ginis et al., 2016, 2017). The GaitAssist system applies adaptive, rhythmic auditory cues and was used in the home environment of PD patients, who showed a trend toward reduced FoG episodes after several days of gait training with the system (Mazilu et al., 2015). Other cueing systems administer gait-synchronized sensory stimulation, which process IMU data online to analyze the gait while walking: Mancini and colleagues examined the effect of vibrotactile cueing at the wrist (VibroGait) and found reduced FoG, improved turning and trunk stability, increased first step duration, but reductions in gait speed and stride length (Harrington et al., 2016; Mancini et al., 2018; Fino and Mancini, 2020; Schlenstedt et al., 2020). Sijobert et al. (2016) developed a smart cueing device applying sensory, electrical stimulation at the lower leg via skin electrodes and found that the time to complete a walking task and the number of FoG episodes decreased.
These studies show that sensor-based gait measurements (1) might help to objectively examine treatment effects on gait disorders, (2) might facilitate the monitoring of treatment outcomes over longer follow-up periods, (3) may be used to predict the outcome of treatments in specific patient subgroups, and (4) could become integral part of new therapeutic methods.
Inertial sensors in MS. Shah et al. (2020) postulated that daily life monitoring with IMUs might be more sensitive to impairments from neurological diseases than laboratory IMU-based gait measures but that the analyzed neurological diseases (PD and MS) might require different gait outcome measures. Trunk-, shank-, or foot-placed IMUs have been frequently applied to measure gait and, especially, balance dysfunction in MS patients, commonly in the form of the instrumented TUG test (Shanahan et al., 2018): Spain et al. (2014) utilized IMUs to differentiate between mild MS, moderate MS, and control groups based on the variability in gait velocity, trunk motion, and sway (range, area). Craig et al. (2017) showed the reproducibility of the instrumented TUG results over two sessions and that stride velocity, cadence, and cycle time correlate significantly with disease severity and number of recent falls. IMU-based analysis has been found useful to detect even early changes in gait and balance in MS (Spain et al., 2012). Measurements with IMUs were able to reflect intra-individual changes in identified biomarkers associated with a change in clinical severity scores in a 12-month prospective study by Galea et al. (2017). Therefore, objective gait analysis with IMUs might increase the sensitivity of clinical and performance tests to monitor gait dysfunction in MS (Vienne-Jumeau et al., 2020). Moreover, spatiotemporal parameters from walking have been used to objectively measure MS disease specific characteristics, such as muscle fatigue, which could be helpful in monitoring and evaluating rehabilitation and treatment efficacy (Motta et al., 2016; Ibrahim et al., 2020). In the area of therapeutic aids and home-care, IMU-based fall detection is an emerging application for various gait disorders (Wang et al., 2020).
3.2. Smart Devices
3.2.1. Technology
Although smart devices, such as smartphones or smartwatches, use inertial sensors as a technique, they are presented separately in this section due to their high presence and popularity in everyday life that makes them particularly interesting for long-term monitoring in home environments. Smart devices can be used as single sensor units like previously described IMUs, for example, by wearing a smart device on the hip for a postural control assessment (Kosse et al., 2015). The data can then be transferred and processed in the same way as previously described for IMUs. Usually, measurement setups are limited to two measurement locations, for example, one smartphone and a paired smartwatch. However, most frequently only one device is utilized (e.g., Chomiak et al., 2019; Hsieh et al., 2019). Available sampling rates for smart-device-based IMUs depend on the hardware (e.g., for Apple products3 (Cupertina, CA, USA), it is supposed to be at least 100 Hz), and can limit the range of applications. The reliability of smart device measurements for motion tracking is still being investigated (Vohralik et al., 2015).
In addition to regular IMUs, smart devices come with an integrated interface and specific software (“apps”) facilitating a user-friendly operation of the data assessment. Different apps provide different data collection modes, such as real-time streaming, recording, and post-recording wireless data download. In literature, mostly customized apps were used to record the sensor data and calculate gait or balance parameters on- or offline (Franco et al., 2012; Kosse et al., 2015; Chomiak et al., 2019), or to upload the data to cloud servers for offline gait analysis (Manor et al., 2018). As an advantage to standalone IMUs, smart devices promote direct biofeedback in the form of visual, auditory, or haptic signals. Due to these features, smartphones are often combined with IMUs for gait monitoring and therapy systems (e.g., Ferrari et al., 2016; Palmerini et al., 2017).
3.2.2. Applications
Although the use of smart devices to assess gait and balance is under extensive investigation, most applications are still under development. Multiple studies explore smart devices for balance assessments measuring trunk movements and postural stability but so far mostly in neurologically intact participants (e.g., Alberts et al., 2015a,b; Kosse et al., 2015; Hsieh et al., 2019). Roeing et al. (2017) reviewed 13 studies and found that five evaluated the validity of their smartphone applications for balance and risk of falls assessment; the results demonstrated strong concurrent validity with standalone accelerometry, 3D motion capture, and force plate measurements. Three of these studies included a measure of reliability revealing high ICC values for mixed variables (Mellone et al., 2012; Cerrito et al., 2015; Kosse et al., 2015; Roeing et al., 2017). Standardized clinical assessments, such as sit-to-stand evaluation (Cerrito et al., 2015; Marques et al., 2021), the TUG (Mellone et al., 2012; Ponciano et al., 2020), and postural balance (Hsieh and Sosnoff, 2021), were instrumented using a single smart device. Also applications in rehabilitation in the form of biofeedback loops with potential use at home, e.g., as a smartphone-based audio-biofeedback in order to improve balance during bipedal standing (Franco et al., 2012), are being evaluated. However, special research interest is on utilizing smart devices for gait assessment as there lies a great potential for long-term monitoring in everyday activities. Fall detection with smart devices is already available on the market in the form of Apple Watch Series 4–6 (Apple Inc, 2020). The accelerometer and gyroscope readings from the wrist are used in combination with a fall detection threshold yielding a high false-positive rate (Wang et al., 2020).
Most approaches aim at extracting gait parameters from the use of a single smart device (Ellis et al., 2015; Kosse et al., 2015; Manor et al., 2018). For example, Manor et al. (2018) created an app for systematic gait data recording and analysis that can be performed independently by the user either in the laboratory or at home with the smartphone placed in the trousers' front pocket. When comparing normal and dual-task trials in neurologically intact volunteers, average stride times derived from the app demonstrated high correlation with the simultaneously used instrumented mat in the laboratory. Lipsmeier et al. (2018) explored the potential of smartphones for assessing biomarkers in PD that might serve as outcome measures in clinical trials. The authors presented moderate to strong retest reliability and successful discrimination between PD and controls with increased sensitivity compared to traditional clinical scales (Buckley et al., 2019).
Despite regular gait assessment, smart devices were evaluated for continuous monitoring in PD for FoG detection and fall prevention. For example, Ellis et al. (2015) developed a mobile application with the smartphone at the front waist to track gait and its variability, an indicator for FoG in PD, presenting it as an alternative to conventional gait analysis technologies. Chomiak et al. (2019) utilized an iPod Touch, worn on the thigh, and ML to identify gait-cycle breakdown and freezing episodes of varying duration. Ahn et al. (2017) presented a system for FoG detection and visual cueing based on smart glasses (Android): The subject's movements are tracked using the inertial sensor from the glasses, which projects visual patterns in the case of a recognized FoG event. Furthermore, numerous smartphone applications have been designed for the assessment and monitoring of multiple health parameters in patients with PD (Monje et al., 2019). The apps combine questionnaires, cognitive, voice, and motor tasks providing repeated measures of the patients motor state along with valid and clinically meaningful knowledge of symptom evolution (Bot et al., 2016; Lakshminarayana et al., 2017; Lipsmeier et al., 2018). Similar applications are available for monitoring MS patients [e.g., elevateMS by Pratap et al., 2020].
These diverse applications of smart devices in gait and balance assessments reveal their future potential to be utilized for objective evaluation of treatments over short and long follow-up periods, closed-loop applications, and telemedical consulting in real-life environments.
3.3. Instrumented Insoles
3.3.1. Technology
Instrumented insoles are insoles that have integrated force or pressure sensors to measure changes in pressure between the foot and the ground. Force sensors measure the applied force discriminating the component of each axis that is measured, whereas pressure sensors are non-discriminating and thereby measure the combined ground reaction force (de-la Herran et al., 2014). Most commonly used insole sensors are capacitive, resistive piezoelectric, and piezoresistive sensors (de-la Herran et al., 2014). The measurement principle is based on the detection of voltage changes caused by fluctuations in electrical capacity or electrical resistance in semiconductor materials due to stretching or compression (Chen and Yan, 2020). The choice of sensor depends on the desired range of pressure/force, sampling rate, and sensitivity (Diaz et al., 2020). Insoles typically incorporate arrays of sensors measuring a spatial pressure/force profile over the plantar foot surface (Shanahan et al., 2018). The profile varies during the gait cycle and depends on a person's body weight: In healthy gait, the maximum vertical force is applied and, thereby, the maximum pressure occurs when the whole body weight is on one leg/foot during the stance phase (Clarke, 1980). No force is applied during the swing phase. The profile's spatial resolution depends on the number of integrated sensors in the insole, its temporal resolution on the applied sampling rate, and its sensitivity on the utilized sensor and analog-to-digital converter.
Available systems are among others the F-Scan (Tekscan Inc., Boston, MA, USA) with 3.9 force-sensitive resistors per cm2, the Moticon SCIENCE pressure insoles (Moticon, Munich, Germany) with 16 capacitive pressure sensors, or WalkinSense (Kinematix SA, Sheffield, UK) with eight force-sensing piezoresistors. The latter two and other newer insole types often incorporate additional sensors such as an IMU (Arafsha et al., 2018). Besides, the available systems differ in the type of power supply, data transmission and storage, in the user operation, and associated analysis software.
The validity of discrete pressure and force measurements with insoles is comparable to optical motion capture and they show a high reliability within and between trials (Shanahan et al., 2018). From the profiles, spatiotemporal gait parameters (e.g., stride time, gait phases) can be extracted. However, patients with neurological gait disorders tend to walk slowly, shuffle, and perform short and dragged steps making it challenging for automatic gait event detection based on heel strike or initial contact (Pirker and Katzenschlager, 2017; Diaz et al., 2020). For balance analysis, insoles are regularly used to measure the COP to evaluate postural stability (Ma et al., 2016).
3.3.2. Applications
So far, the clinical application of instrumented insoles in PD patients has mostly been limited to the differentiation between PD and controls. Extracted gait and balance parameters have been used successfully for discrimination between the groups (Mazumder et al., 2018; Chatzaki et al., 2021), in line with the findings from established laboratory gait assessments. Furthermore, instrumented insoles have been investigated for their ability to recognize and quantify FoG in PD (Popovic et al., 2010; Shalin et al., 2020; Pardoel et al., 2021). Pardoel et al. (2021) combined features derived from a pressure-sensing insole and IMUs on the leg to detect FoG in 11 PD patients. The authors reported that the combination of both modalities outperformed classification models that used data from a single sensor type. In a small data sample (n = 5), Shalin et al. (2020) demonstrated that foot pressure distributions from 60 × 21 sensor-arrays could be used for FoG prediction (0.5–3 s before FoG onset). Therefore, together with inertial sensors, instrumented insoles could be integrated into therapeutic cueing devices for treating gait disorders in PD (cf. section 3.1).
Few studies utilized insoles to examine gait function in MS patients so far (Shanahan et al., 2018). Viqueira Villarejo et al. (2014) reported an increased plantar pressure during the stance phase and variability in step timing in MS compared to controls. Galea et al. (2017) quantified MS-related gait and balance deterioration over 12 months using EMG and insoles and observed decreases in gait speed and balance scores, and an increase in double support time. Doḿınguez et al. (2020) validated gait velocity and other parameters from a new insole system with an incorporated IMU against a common instrumented walkway in 205 MS patients. The results revealed a high correlation between devices in velocity, ambulation time, cadence, and stride length. Note that spatial parameters, such as stride length and stride wide, can only be derived from the IMU data (Farid et al., 2021).
Although the use of insoles is unobtrusive and, therefore, has a high potential in monitoring daily activities (Diaz et al., 2020), the hesitant use in research and clinical application may have practical reasons. For reliable measurements, diverse sole sizes must be available to cover the variety in foot sizes. Systems with multiple soles and validated analysis software can initially require a five-digit amount. Due to the mechanical stress during walking, the soles' life is limited. People must wear shoes that allow the use of additional insoles. Furthermore, shoes must be taken off and put on again to set up the measurement, an additional obstacle for elderly patient groups such as PD. However, for long-term monitoring in the future, the ease of integration in patients' everyday life could be an advantage.
3.4. Summary and Discussion
Wearable sensor technology is currently being applied and explored in existing and newly developed clinical gait and balance assessments as well as for long-term monitoring of various activities in daily living. For multiple reasons, body-worn sensors are of great value for balance and gait assessments in neurological disorders: Their high level of portability theoretically facilitates unlimited use in laboratory research environments, clinical settings, and home environments. No line-of-sight restrictions apply as in vision-based technologies. The small, lightweight, and wireless devices do not restrict the subject's movement. In contrast to laboratory-based methods, wearable devices might come at low prices and facilitate easier setups. When provided with a graphical user interface and validated analysis software, usability can be as good that patients can record data on their own. The number of gait parameters that can be extracted from wearables has expanded dramatically over the last years and new, more robust algorithms are under permanent development.
However, all these potential advantages are not always met in the available systems. The gait estimation algorithms for IMUs and insoles are often still in exploration, not evaluated to a reliable extent in the desired target group. The optimal sensor layout is still debated and requires a trade-off between usability and accuracy. Furthermore, it is challenging to calculate paths and distances traveled (Buckley et al., 2019). Necessary sensor-to-segment alignment, the need for precise manual sensor attachment, and required calibration movements by many methods halt the advance of inertial sensor techniques into clinical trials. When utilizing magnetometer readings, measurement errors occur in magnetically-disturbed environments, such as typical clinic or home environments containing electronic devices and objects of ferromagnetic material (de Vries et al., 2009). With increasing algorithm complexity, required processing resources rise yielding high energy consumption and waiting times between subsequent recordings. Still, algorithm development is an active area of research tackling these issues (e.g., Marín et al., 2020; Laidig et al., 2021). Also, existing hardware issues, such as limited recording time by battery and storage capacity, and data loss during the wireless transfer from sensors to applications or cloud servers should be a trivial problem in the future. Patient user interfaces continue to improve (Shanahan et al., 2018).
In addition to their use in recording and analyzing gait and balance disorders, wearables can be applied in rehabilitation technologies or therapeutic aids, such as a cueing device to treat gait impairments in PD (cf. Table 2). Besides beneficial therapeutic effects, full-time body-worn sensors allow long-term monitoring and might contribute to the individualization of therapies as well as to telemedicine concepts. The objective tracking and quantification of a PD patient's motor activity over the day is valuable information for the precise adjustment of individual medication plans. Particularly in times of global pandemics, where the number of regular face-to-face visits is reduced (Roy et al., 2020), automatically extracted and shared parameters from wearables have the potential to support clinical decisions. Automatic evaluation methods of data from wearables in clinical gait and balance assessments (e.g., Karatsidis et al., 2017; Nguyen et al., 2019) but also in unrestricted activities of daily living (e.g., Roth et al., 2021) are constantly investigated. Machine learning techniques are the driving force behind this rapid growth of applications. Still, a remaining challenge lies in obtaining validated measures and standardized motor parameters that predict relevant clinical outcomes for each neurological disease (Monje et al., 2019; Shah et al., 2020). Further investigations are required before the analysis of locomotion in everyday activities becomes reliable and thereby clinically relevant (Graham et al., 2008; Lord et al., 2013).
4. Non-Wearable Technologies: Vision-based Motion Analysis
Marker-less optical motion capture systems have become popular with the launch of affordable in-depth cameras. Even though computer gaming and virtual reality serve as the main drivers for this rapid evolution of digital image processing, the practical application in the health sector has been discussed and performed frequently (Clark et al., 2012; Albert et al., 2020). Despite being an older technology, motion tracking with optical markers is generally handled as the gold standard with which newly developed technologies for gait analysis are compared. However, marker-based tracking requires an extensive, expensive, non-portable setup. Similar restrictions apply for instrumented mats, walkways, or treadmills, although they are portable on a large scale and often easier to handle. For the sake of completeness, we mention these approaches as references; overviews of gold standard methods can be found elsewhere (e.g., Shanahan et al., 2018; Celik et al., 2021).
Presently, vision-based non-wearable technologies with standard cameras or depth cameras are increasingly applied in human motion tracking. Image acquisition is most conveniently achieved via standard 2D cameras that output images as 2D pixel grids. Each pixel traditionally carries a red, green, and blue (RGB) value, with intensities ranging from 0 to 255. However, 2D cameras do not provide any spatial depth information on the tracked pose. This information has to be obtained either by performing additional post-capture processing with machine learning (ML) algorithms, by using multiple cameras, or by switching to another technology, such as depth cameras which provide 4D information on the tracked object. Pixels obtained with depth cameras are primarily coupled to the distance of the tracked object from the sensor and are typically paired together with a classical RGB value. Both, standard and depth cameras, are able to extract detailed information required for biomechanical analyses.
4.1. In-depth Camera Technology
There are several types of in-depth cameras that rely on different methods to infer depth, as illustrated in Figure 4. Structured light imaging uses patterned light to capture the 3D topography of a surface (Geng, 2011). Here, the scale and direction of a distorted pattern are used to assess the depth of an object. Furthermore, time-of-flight (ToF) technology measures the time it takes for infrared light to travel toward an object and reflect into the imaging sensor (Kolb et al., 2010). The corresponding phase shift in the signal is subsequently measured and converted into distance. Lastly, stereoscopic vision (also: stereotactic imaging) incorporates two or more stereo cameras to compare two or more simultaneously recorded images for the estimation of depth. Likewise to human eyes, the distance between the cameras is fixed and used to measure the closeness of an object on multiple juxtaposed images obtained by using any type of light.
Two well-known, affordable depth-sensing cameras that are frequently used in medical applications are Kinect (Microsoft, Redmond, WA, USA) and RealSense (Intel, Santa Clara, CA, USA), which are reviewed here as examples due to their manifold occurrence in literature on gait and balance. Other commercially available systems on the market are, e.g., ZED (StereoLabs, San Francisco, CA, USA) or XtionPro (ASUS, Taipeh Taiwan).
4.1.1. Microsoft Kinect
Microsoft's Kinect is a motion-sensing device originally developed for gaming purposes and is one of the earliest motion capture technologies of its kind to be used in medical research. Owing to Kinect's long history in pose estimation, it has been well-assessed in various research settings and utilized in tracking different movement patterns. Throughout the last decade, several versions of Kinect have been produced: The introductory model Kinect 1 (2010) integrates a structured near-infrared light source with an accompanying sensor to capture the reflected light patterns, whereas Kinect 2 (2013) and Azure Kinect (2019) use wide angle ToF cameras (Zhang, 2012).
Although Kinect 1 is a well-established system, when compared to gold standard techniques, it provides only basic motion capture capabilities such as collecting temporal gait parameters, estimating single joint angles, or assessing postural control during reaching and balance tasks (Clark et al., 2012; Schmitz et al., 2014). In kinematic gait recordings, the system generally underestimates joint flexion and overestimates extension during walking in the sagittal plane. Here, stride timing measurements perform surprisingly well with the highest accuracy at low gait speeds, despite a high error in hip and knee displacement (Pfister et al., 2014). Clothing and different body shapes were discussed as possible reasons for measurement errors. Therefore, approaches that use additional multi-layer filtering, where the estimated pose is further refined through a synthetic library of posture variations, can alleviate some tracking inaccuracies, increase parameter precision, and allow for better recognition of occluded body parts (Shotton et al., 2011; Wei et al., 2012; Xu et al., 2013). Moreover, recording frameworks with multiple cameras were able to improve pose estimation accuracy and approximation of occluded segments at the costs of a higher setup effort (Gao et al., 2015).
Nonetheless, Kinect 1 has been used in several clinically-oriented studies to measure lower body biomechanics for determining stride time, length, and speed in healthy individuals (Gabel et al., 2012; Auvinet et al., 2015). In the context of PD, scientists used wavelet-based digital signal processing to analyze gait parameters and quantitatively distinguish gait phases with an accuracy of up to 93% (Muñoz et al., 2018). Spatiotemporal parameters were distinguishable in stage II and III PD patients compared to a control group, reaching a maximum accuracy of 97.2% after classification with a neural network (NN) (Ťupa et al., 2015). Likewise, Kinect 1 technology has been implemented in MS gait analysis to discern MS patients from neurologically intact controls by differences in the average walking speed and lateral body sway (Behrens et al., 2014), or ROM, stride length, and step width (Gholami et al., 2016).
The newer Kinect 2 system uses continuous-wave ToF technology instead of structured light, enabling a more stable data feed with an increased accuracy within the measurement range of 4m (Gonzalez-Jorge et al., 2015). In clinical assessments, Kinect 2 displayed an adequate performance when tracking joint center displacement (Napoli et al., 2017). The second generation demonstrates better accuracy in joint estimation and stays more robust to body rotation as well as occlusions during various movements like walking and jogging (Wang et al., 2015; Guess et al., 2017). Therefore, Kinect 2 seems to outperform Kinect 1 in locomotion tracking except for foot position tracking during standing, where a larger amount of noise is generated, possibly due to ToF artifacts (Otte et al., 2016). Kinect 2 reliably assessed spatiotemporal parameters during comfortable and fast-paced gait (Mentiplay et al., 2015). However, significant performance variations in different motion planes and incompatibility with certain functional movements still exist in Kinect 2 when compared to marker-based systems, especially in the context of compound joint movement (Mentiplay et al., 2015). The validity of lower limb joint kinematics depends on the camera's capture angle for recording the walking subject. Moreover, in treadmill walking, accuracy levels appear to vary across gait parameters, with temporal parameters based on heel strike having fewer errors than those based on toe-off, and their accuracy fluctuates with changing walking speeds (Xu et al., 2015). Linear pelvic and trunk ROM can still be tracked with reasonable precision at 70 and 90% of maximal locomotion speed, providing a reliable reference point across all velocities (Macpherson et al., 2016). In attempts to use multiple Kinect 2 cameras to achieve a higher tracking accuracy, several cameras have to be calibrated together via geometric trilateration. The distance between the subject and at least three recording cameras is measured through signal strength. When used to determine gait parameters, three Kinect 2 sensors show a much higher spatiotemporal reliability compared to a single Kinect 2 camera (Yang et al., 2016).
Kinect 2 has been applied in PD patients, where 92% of freezing episodes, 91% of tremor occurrences, and 99% of falling incidents could be detected with customized algorithms (Bigy et al., 2015). Moreover, Kinect 2 measurements in combination with customized algorithms were able to consistently produce results similar to a marker-based system and output significant differences between PD and control groups for stride length, gait, and swing velocity (Eltoukhy et al., 2017; Sabo et al., 2020). In MS patients, moderate and fast walking speed measurements agree with results derived from marker-based systems, but once more, only if combined with customized software or auxiliary ML-based classifiers (Bethoux et al., 2018; Elkurdi et al., 2018). Indeed, it seems that additional ML algorithms or NNs can frequently increase the validity and reliability with depth cameras (Rocha et al., 2018).
Microsoft's Azure is the most recent Kinect upgrade that supports additional features and several depth-sensing modes. Although part of the same production line, it has been specifically designed for distinctive non-gaming purposes such as research and health care use. Compared to Kinect 2, Azure has a higher angular resolution, lower noise, and better tracking accuracy (Tölgyessy et al., 2021). When used as a dual system consisting of two cameras, Azure outputs precise knee angles and demonstrates an overall improved validity over Kinect 2 (Ma et al., 2020b). During the estimation of sagittal hip and knee joint angles, a single Azure appears to have a superior depth resolution and shows better tracking performance when subjects walk at non-frontal camera viewing angles (Yeung et al., 2021). In treadmill walking, spatial gait parameters (e.g., step length and width) can be measured more reliably with Azure, though the accuracy of temporal parameters (e.g., stride duration) does not change significantly between the two models. Interestingly, Kinect 2 seems to outperform Azure regarding upper body tracking. However, an overall increase in the quality of lower extremity parameters and the additional introduction of integrated deep learning-based body tracking algorithms create appeal for Azure to be used in gait rehabilitation (Albert et al., 2020). As far as the application of Azure in PD and MS studies is concerned, to our knowledge there has not been any material published yet.
4.1.2. Intel RealSense
Intel's RealSense cameras stem from several generations of stereo depth cameras with a production start in 2015. The system comprises a left-right depth stereo camera pair and an additional color camera. The stereo cameras use textured light to ensure unambiguous image matching, which in turn enables more accurate depth measurements. Accordingly, stereotactic systems including RealSense are less sensitive to noise compared to other in-depth camera types, which allows for a more flexible experimental setup (Keselman et al., 2017; Zabatani et al., 2019). In motion analysis, the system can be used to measure a definite amount of spatiotemporal variables, however, joints with multiple degrees of freedom exhibit inaccuracies yielding difficulties for the forthcoming gait data analysis (Mejia-Trujillo et al., 2019). In general, RealSense seems to perform better at slow to normal walking speeds located in small to medium-sized environments (Hausamann et al., 2021). Auxiliary tools can be used to extend the current three-part system to up to six cameras to improve body shape and joint position tracking (Boppana and Anderson, 2019). Intriguingly, despite having an older production age, both Kinect 1 and 2 seem to rival RealSense's signal quality and capture range during walking (Mejia-Trujillo et al., 2019). Moreover, temporal parameters seem to exhibit slightly better accuracy when recorded with RealSense, whereas spatial parameters retain similar values to Kinect measurements (Gutta et al., 2021). While a few gait studies with Intel's RealSense exist, up to the present moment no publications known to the authors have used the technology to assess gait and balance explicitly in either PD or MS patient groups. However, RealSense convincingly holds the potential to be used in clinical research, whether as a new method for motion tracking or as a Kinect substitute (Clark et al., 2019).
4.2. Standard Camera Technology
In daily clinical practice, video recordings are still predominantly recorded with conventional standard cameras. Clinicians may film their patients during outpatient or inpatient visits (e.g., in frequented hallways) or are presented with home videos for neurological evaluation (Sato et al., 2019). However, the material has so far only been used for subjective assessment and documentation, not exploiting its full potential. The use of standard camera material for motion analysis would require a less demanding setup, fewer recording constraints, and would offer more favorable pricing, and integrability into daily research, clinical and telemedical settings. The resulting demand for swift algorithms that accurately determine body part locations on video images drove the development of numerous approaches for pose estimation in standard imaging. In comparison to depth cameras that output distance information without requiring training and often include built-in post-processing software, standard camera footage has to be analyzed offline by a separate learning pipeline for motion tracking. The software pipeline either determines pose coordinates in 2D or deduces depth, in case 3D coordinates are the desired output. In this section, we go over recent findings in pose estimation and discuss available toolboxes designed as ready-to-use software packages for a broader scientific audience that might be applied for gait analysis in neurological disorders.
4.2.1. 2D Pose Estimation
Most recent 2D pose estimation approaches rely heavily on contemporary advances in deep learning, a branch of ML that employs NNs with many layers. With an annotated image data set where objects have been manually labeled, a NN can be trained via supervised learning to classify and track those objects. Pose estimation algorithms frequently use convolutional neural networks (CNNs) as their architectural foundation with multiple layers (e.g., Toshev and Szegedy, 2014). The greatest advantage of CNNs is their ability to learn feature representations directly from the data set in use, which removes the need for additional training data, thus, ensuring a straightforward experimental flow. Their convolutional structure produces 2D probability maps for the location of each body part after they had been trained to recognize image features that belong to specific shapes (e.g., knee, foot) (Wei et al., 2016). This establishes a statistical relationship between the input images and output pose key-points, which can be used to track pose in yet unanalyzed data and make predictions on the spatiotemporal appearance of tracked key-points.
In video data, 2D pose tracking represents a unique set of challenges and numerous network designs have been created to optimize both for speed and reliability in their specific study context. Contrary to static image analysis, images that have been extracted from video frames are often subject to motion blur, frequent body occlusions, unconventional subject positions and further represent large data sets due to the sheer amount of frames in a single video (cf. Figure 5). The continuously increasing amount of new pose algorithms also evokes the demand for largely manually annotated data sets that thematically represent the defined area of research: sports, outdoors, medical research, and many others (Sigal et al., 2010; Ionescu et al., 2013; Andriluka et al., 2014). To withstand these challenges, attempts have been made to increase the quality of parameter supervision by, for example, cross-correlating features in adjacent video frames or integrating various mathematical approaches with NNs, and to reduce the amount of required pre-labeled data (Ouyang et al., 2014; Szegedy et al., 2015; Tompson et al., 2015; Feichtenhofer et al., 2017).
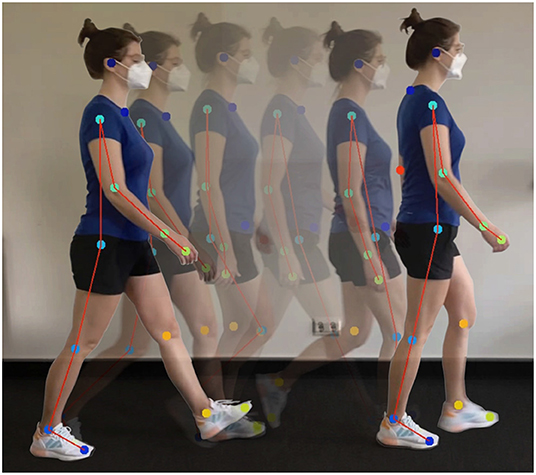
Figure 5. An example of 2D motion tracking performed with DeepLabCut. Here, several joints are being tracked simultaneously to determine the exact limb position during straight walking.
In gait analysis, established preliminary models use standard cameras ranging from simple mobile phone cameras to multiple cameras accompanied with additional sensors such as IMUs or floor sensors (Alharthi et al., 2019; Viswakumar et al., 2019; Vaith et al., 2020; Stenum et al., 2021). The validity, reliability, and processing time of these models vary according to the type and quality of camera footage, computational system architecture, type and amount of training data used as well as many other factors. Therefore, choosing a suitable pose estimation model is strongly influenced by the experimental setting and might depend on the number of tracked legs, frequency of body part occlusions, subjects' clothing, and room background color. Until these and other issues are resolved, 2D pose estimation will not be applied on a wide scale in the clinical field. However, first studies indicating possible applications of this technique in neurological disorders have been published: Li et al. (2018) combined the outcome of convolutional pose machines with ML-based classification for discriminating disease and symptom severity in PD patients in tasks such as toe-tapping and stamping. Hu et al. (2019) successfully established a novel graph CNN to classify freezing episodes from regular gait in the TUG test of 45 Parkinsonian patients, recorded in frontal view.
4.2.2. Single-View 3D Pose Estimation
Occlusion of body parts has continuously presented a challenge to 2D human pose estimation for gait analysis, especially when both legs are tracked simultaneously, as desired, for example, for analyzing gait symmetry in PD. Thus, advances have been made toward setting the pose in a 3D coordinate framework instead of 2D by subsequently generating a 3D environment from images obtained by a single RGB camera. Complementary 3D pose libraries can be used to create 2D projections from virtual camera views. In such cases, 2D pose estimation is performed on input images and then depth is calculated using an additional pre-existing 3D library as a reference (Chen and Ramanan, 2017). However, the employment of 3D libraries requires even larger amounts of annotated data. To address this challenge, specialized CNNs have been implemented to output 2D key-points together with body silhouettes, which are later synchronized with a mathematically generated 3D body mesh model to estimate full 3D pose (Loper et al., 2015; Pavlakos et al., 2018). Moreover, some networks specialize in the detection of individual people from group images and automatically crop out single subjects that are present on the input image, subsequently performing individual 2D pose tracking and later placing the obtained parameters into a virtual 3D environment (Moon et al., 2019). Recently, single-view 3D pose estimation has been integrated into the gait analysis of PD patients, where spatiotemporal parameters including step length, velocity, and cadence evaluated with a deep learning pose estimation algorithm seemed in good agreement with reference data obtained through pressure sensors (ICC > 0.9) (Shin et al., 2021).
4.2.3. Multi-View 3D Pose Estimation
The multi-view approach to 3D pose estimation is an alternative scheme that further reduces training set size and eliminates the need for large 3D libraries. One strategy is to train the network on images from multiple cameras before predicting the 3D pose from images obtained by a single camera (Rhodin et al., 2018). Specifically, a network is trained to predict the same 3D pose regardless of camera perspective and can perform 3D predictions solely based on 2D imagery. Other methods include algebraic and volumetric triangulation that are speculated to be more robust to occlusions or partial body visibility (Iskakov et al., 2019). Further strategies use so-called key-point coordinates instead of heatmaps (Pavllo et al., 2019) or employ a multi-stage architecture to reconstruct the 3D pose from 2D heatmap predictions at each CNN processing stage (Tome et al., 2018).
As multi-view 3D pose estimation approaches effectively deal with body part occlusions and simultaneously alleviate the need for large training libraries, they present a promising tool in gait tracking. Indeed, such models are able to qualitatively reproduce locomotion compared to marker-based motion capture, albeit still producing a small error rate in the final 3D pose (Nakano et al., 2020). Therefore, technical challenges of multi-view systems such as the setup of multiple cameras, triangulation, and more extensive processing make the experimental setting more demanding but at the same time offer an opportunity to improve the quality of gait parameters.
4.2.4. Software Toolboxes
While numerous algorithms have been created in the attempt to improve the performance of 2D and 3D pose estimation algorithms, we will now briefly summarize several that have been pre-packaged as software toolboxes and are being used by a wider, non-specialist scientific community to track human motion promoting new fields of application (Table 3).
One of the earliest of such packages is DeeperCut, a multi-person pose estimation method based on the integer linear programming approach DeepCut (Pishchulin et al., 2016). Here, deep residual neural networks (ResNets) have been adapted inside a convolutional architecture in form of a sliding window-based body part detection (He et al., 2016). ResNets build on constructs known from pyramidal cells in the cerebral cortex: They utilize skip connections, or shortcuts to jump over some network layers and map nonlinearities. Moreover, DeeperCut features image-conditioned pairwise terms or architecture components that indicate the presence of other body parts in the vicinity of a tracked point and group these body parts into a valid pose configuration (Insafutdinov et al., 2016). Published in 2018, DeepLabCut is a more recent tracking toolbox. Although a CNN architecture as well, DeepLabCut significantly differs from its predecessor DeeperCut by implementing pre-trained ResNets, which fine-tune the already existing node weights following the tracked body part. Therefore, DeepLabCut exhibits a faster performance and requires a smaller amount of pre-labeled images for training. After network processing, the user can readily access spatial coordinates and the existential probability of every tracked body part, stored in the form of x- and y-coordinates for each video time frame (Mathis and Warren, 2018; Mathis et al., 2018). Figure 5 shows an example of 2D motion tracking with DeepLabCut. Additional reconstruction of 3D kinematics with DeepLabCut is possible by either establishing individual networks for each camera view or training a single network that generalizes across all views (Nath et al., 2019).
OpenPose is a real-time 2D pose estimation approach developed for motion processing of multiple individuals on a single image. An integral part are Part Affinity Fields (PAFs), a set of 2D vector fields that encode the orientation and location of limbs on the analyzed image. Moreover, PAFs are bottom-up representations of unstructured pairwise relationships between detected body parts that enable the reconstruction of the full-body pose while decreasing the total computational cost. As with any multi-person tracking algorithm, OpenPose faces obstacles like subjects present on the image at different positions or scales and body part occlusions (Cao et al., 2019). OpenPose has recently been implemented with multiple synchronized cameras to evaluate motor performance in a 3D pose framework. Compared to a marker-based system, the mean absolute error of points tracked during walking equaled less than 30 mm, excluding 10% of cases where OpenPose initially failed to recognize the correct body segment during 2D estimation (Nakano et al., 2020).
Several other prominent pose estimation toolboxes exist which have not yet been frequently featured in gait research: Anipose is an open-source toolkit designed to augment the existing 2D tracking methods for accurate pose tracking in a 3D setting. It deploys optimization on the calibration, triangulation, and filtering over multiple camera views that accompanies the processing by antecedent NN packages (Karashchuk et al., 2020). DeepPoseKit aims to resolve the limitations of over-parametrization by pre-trained ResNets and the lack of robustness in GPU-based approaches. The pipeline is based on alternative confidence map processing methods, multi-scale inference, and GPU-oriented convolutional layers (Graving et al., 2019). Lastly, AlphaPose is another open-source multi-pose estimator featuring a regional multi-person pose estimation (RPME) framework (Fang et al., 2017). During training, the RPME pipeline detects single humans on the image by establishing bounding boxes around each individual. Afterwards, single pose estimation is performed on each bounding box and the output is further refined (Xiu et al., 2018; Li et al., 2019).
In conclusion, several toolboxes have already been tested on human footage of walking and running. Among the software packages in Table 3, OpenPose has been most extensively evaluated both in 2D and 3D gait estimation. In 2D video analysis, mean absolute errors of temporal parameters are smaller than differences arising from natural variations in the walking pattern making temporal changes detectable in healthy gait (Stenum et al., 2021). Step length estimation accuracy depends on the participant's position in the camera field of view, with central positions resulting in lower error rates. Unlike gait speed that reaches accuracy levels similar to the gold standard, errors in sagittal hip, knee, and ankle angles are in proximity of test-retest errors in the same plane. In an underwater running setup, the accuracy of predictions for 2D-joint marker positions extracted with DeepLabCut seems to match manual labels with a mean difference of fewer than three pixels (Cronin et al., 2019). Although not compared to a marker-based system, DeepLabCut seemed sensitive enough to differentiate between closely-spaced running cadences with a high test-retest reliability of the mean stride data. In 3D motion capture obtained with OpenPose, DeepLabCut, and AlphaPose, significant kinematic differences at hip and knee occurred in comparison to marker-based systems (Needham et al., 2021). Here, tracking accuracy of the ankle unexpectedly performed better than other joints, possibly owing to more precise manual annotation during training due to its apparent anatomical position. When compared to IMUs, OpenPose seems to exhibit tracking discrepancies in joint angles of up to 14 (Gu et al., 2018; D'Antonio et al., 2020, 2021). Despite these reports, Sato et al. (2019) employed a pipeline with OpenPose to analyze cadence in daily clinical movies recorded from the frontal angle in healthy controls (n = 117) and two PD patients with prominent FoG. The authors reported a discrimination performance for mild PD gait from controls of 0.75–0.96 (area under curve) and for comparing gait sequences before vs. after DBS treatment (n = 1) of 0.98. On the whole, as the demand for efficient and cost-effective technologies for gait analysis grows, deep learning architectures are still lacking in precision but continue to improve rapidly and are increasingly being implemented into clinical studies and home assessments (Xue et al., 2018; Viswakumar et al., 2019; Sibley et al., 2021).
4.3. Summary and Discussion
Non-wearable technologies are becoming an attractive tool for gait and balance analysis due to their advantages compared to wearables. Their availability, portability, easy setup, and complete non-intrusiveness shorten the preparation time significantly and may reduce the stress of the participant. These attributes yield comparatively low pricing, bringing non-wearable marker-less tools distinct advantages over customary gold standard technologies that are costly and difficult to deploy in environments of everyday activities. Conversely, vision-based motion tracking accuracy of non-wearable systems remains lower than that of marker-based systems. While discrepancies in temporal parameters stay at a small scale, spatial parameter differences including joint angles vary from system to system and are significantly influenced by the experimental environment. Indeed, the error rate of most systems depends on the recording conditions as well as movement complexity and speed, which limits data capture to greater constraints and reduces the number of feasible walking assessments. Moreover, depth camera technology remains sensitive to potential light interference from multiple sensors and operates only in certain volume ranges, reducing the amount of suitable settings (Colyer et al., 2018).
At present, the application of in-depth technology in neurological disorders to quantify gait and balance impairments is yet in exploration. Although the performance of the reviewed systems has been exploited in healthy gait, studies on validity and reliability in pathological gait patterns are still rare, especially for most recent developments (Azure). Existing studies showed that spatiotemporal and kinematic parameters from walking and standing can be extracted and used for differentiation between PD/MS individuals and neurologically intact controls (Behrens et al., 2014; Ťupa et al., 2015; Gholami et al., 2016; Eltoukhy et al., 2017; Sabo et al., 2020), as well as for falling, tremor, and freezing detection in PD (Bigy et al., 2015). Furthermore, combining the in-depth camera output with downstream ML methods seems promising for robust gait analysis in the clinical context (Ťupa et al., 2015; Bethoux et al., 2018; Elkurdi et al., 2018; Rocha et al., 2018). However, this comes at the loss of simplicity, and requires expert knowledge in the application.
Intensive research is currently carried out in the area of pose estimation with standard cameras. Yet, the available methods appear too complex for human gait analysis to be applied outside research environments at the moment. Nonetheless, the rapid evolution of these techniques can be predicted due to the high availability of video material and the already distributed toolboxes under creative commons licenses. Once intensively trained networks on large, standardized data sets are available, the application in clinical and home environments will be possible on a larger scale. In conclusion, for current and planned studies on movement disorders, the careful recording of video material, ideally from two or more perspectives, should be an integral part as this could allow a detailed motion analysis in the near future.
Altogether, marker-less vision-based motion tracking offers an exciting new opportunity for capturing gait-related data in the clinical context. Even though the technology is not yet mature, it shows distinct advantages over gold standard methods and might help unfold a new niche of easily accessible, repeated, longitudinal data collection not only in clinical but patients' home environments. The steady transition toward simpler recording technologies also fits impeccably the contact restrictions in the ongoing COVID-19 pandemic pushing the need for remote video measurements and analysis in telemedicine (Sibley et al., 2021).
5. Conclusion and Future Directions
This review examined established and emerging wearable and vision-based portable technologies for objective gait and balance analysis applicable for neurological disorders. The literature published on the topic is extensive reflecting the high demand for reliable, sensitive, easily accessible, easy to use, and mobile measurement systems. New developments aim to reduce monetary and personnel costs, improve accessibility, and allow short as well as long-term assessments in and outside the clinic. Meeting all these demands still poses a challenge, since the continuous detection and characterization of locomotion in various environments is a complex task. Nonetheless, a great number of gait and posture parameters can be captured with inertial sensors, instrumented insoles, smartphones, in-depth cameras, and also to some extent with standard camera technology. Due to the increased sensitivity of these objective parameters, early subtle gait dysfunction or disease progression become measurable (Horak et al., 2015). Therefore, instrumentalized gait and balance analysis will play a major role in prospective diagnosis, prevention, therapy, and monitoring of neurological disorders.
The decision on a suitable measurement and analysis tool for current studies and clinical examinations depends on balancing the requirements for validity, reliability, and usability. The first step is to define the key parameters that are to be measured with high accuracy and sensitivity with respect to the target group and their gait and movement characteristics. For example, step length was shown to be an important biomarker in PD and vision-based tracking methods might be more reliable than wearables in tracking this parameter (de-la Herran et al., 2014). Especially in joint angle tracking, the reviewed technologies still lack reliability compared to laboratory-based systems, which offer the greatest sensitivity and are reliable over a wide spectrum of measures. Secondly, the choice of a measurement instrument is heavily influenced by the given or desired measurement setup. The various technical solutions also offer different operating concepts and workflows. Parameters can be either extracted in real-time, thus being available for immediate biofeedback or adaptive therapies, or parameters are determined offline, often yielding a higher accuracy. The distinct usability aspects must be carefully weighed before deciding to integrate a system into clinical trials, workflows, or home applications.
Provided with the broad overview of literature in this review, we recommend a number of improvements for future research: (1) To overcome the existing inconsistencies in application, reporting, and interpretation of the extracted gait and balance measures, the utilized hardware and software, including the version number, should be reported. (2) When comparing the assessed parameters with values from the literature, one has to be very careful regarding the exact definition of the parameter calculation. At the moment, reported gait and balance parameters vary greatly between studies, making it difficult to compare treatment effects or to choose meaningful parameters for future investigations. Therefore, publications should provide the exact parameter definitions and methods in the supplements, when using self-implemented algorithms, or refer to applied definitions from literature (Benedetti et al., 2013; Siragy and Nantel, 2018). (3) For the same reasons, in any gait data analysis, gait velocity should be included in experiments as a final common expression of gait performance, plus a range of gait variables according to pre-defined criteria (Lord et al., 2013). (4) Due to rapid developments in pose estimation, the careful recording of video material, ideally from two or more perspectives, should be an integral part of any upcoming study as this material could allow a detailed motion analysis soon.
However, before the new systems are integrated into clinical routines, further research into the validity and reliability of each device is essential, preferably with comparative studies in large populations of neurologically intact controls and individuals with neurological disorders (Horak et al., 2015). This requirement contrasts with the advantage that wearable and marker-less vision-based systems are less expensive than gold-standard technologies: The more effort that has been put into the development and validation of a technology, the more expensive commercially available systems become. Despite the required improvements, we hold the opinion that portable systems for objective assessment of gait and balance characteristics are indispensable to support the neurological, face-to-face exam along with imaging and other biomarkers to facilitate individualized, adaptive treatments in the future. We see that there is a vicious circle to be escaped where as long as the technologies for simple and reliable gait analysis are not yet mature, the search for disease-specific biomarkers will be held up yielding skepticism toward the usefulness of these techniques. One future direction is the integration of several and novel sensor modalities (Buckley et al., 2019; Espay et al., 2019; Morita et al., 2020). Multiple sensors can provide redundant information and their fusion might reduce uncertainty, which can increase reliability in case of a sensor failure. The different modalities can provide objective, real-world data about the clinical phenotypes of individual patients over flexible amounts of time, possibly boosting our knowledge of locomotion and disease pathologies in the concept of deep phenotyping (Dorsey et al., 2020). The creation of normative databases (big data approaches) will yield an increased understanding of pathologies, enhancing the evaluation of therapies, and improve patient care (Buckley et al., 2019; Monje et al., 2019). In the long term, the emerging techniques for gait and balance tracking might be used for continuous monitoring and predicting disability such as fall risks in real-world environments (Weiss et al., 2015) and can be integrated into new, personalized therapeutic interventions. In the context of tele-consultations, tele-therapy and -rehabilitation, wearable and vision-based technologies can be utilized to report and monitor movement conditions and compliance with treatments. In the development of these telemedical applications, a strong focus should be on usability such that target user groups suffering from motor as well as mild cognitive impairments can use the technologies safely and reliably.
Ethics Statement
Written informed consent was obtained from the individual(s) for the publication of any potentially identifiable images or data included in this article.
Author Contributions
CS-H, MS, and MJ drafted the work (Introduction: CS-H, Measures of Gait and Postural Control and Wearable Technologies: CS-H and MJ, Non-wearable Technologies: Vision-Based Motion Analysis: MS and CS-H, Conclusion and Future Directions CS-H). All authors contributed to the conception, design of this work, and revised it critically for important intellectual content. All authors gave final approval of the version to be published and agree to be accountable for all aspects of the work.
Funding
This work was funded by the German Federal Ministry of Education and Research (BMBF) within the project Mobil4Park—project ID FKZ16SV81 68—and the Deutsche Forschungsgemeinschaft (DFG, German Research Foundation)—project ID 424778381—TRR 295. NW is a Freigeist-Fellow supported by the Volkswagen Foundation, and a participant in the BIH Charité Clinician Scientist Program. NW and MS are supported by Hertie Academy of Clinical Neuroscience.
Conflict of Interest
Author TS is co-founder of the SensorStim Neurotechnology GmbH, a company developing sensor-based stimulation technologies.
The remaining authors declare that the research was conducted in the absence of any commercial or financial relationships that could be construed as a potential conflict of interest.
Publisher's Note
All claims expressed in this article are solely those of the authors and do not necessarily represent those of their affiliated organizations, or those of the publisher, the editors and the reviewers. Any product that may be evaluated in this article, or claim that may be made by its manufacturer, is not guaranteed or endorsed by the publisher.
Footnotes
1. ^Unified Parkinson's Disease Rating Scale - Part III (UPDRS-III).
2. ^Expanded Disability Status Scale (EDSS) 0–2.5 (Benedetti et al., 1999; Martin et al., 2006) and 2.5–6 (Remelius et al., 2012); Hauser Ambulation Index (HAI) 0–2 (group 1) and 3–4 (group 2) (Kelleher et al., 2010).
3. ^https://developer.apple.com/documentation/coremotion/getting_raw_accelerometer_events [accessed July 6, 2021].
References
Adusumilli, G., Lancia, S., Levasseur, V. A., Amblee, V., Orchard, M., Wagner, J. M., et al. (2018). Turning is an important marker of balance confidence and walking limitation in persons with Multiple Sclerosis. PLoS ONE 13:e0198178. doi: 10.1371/journal.pone.0198178
Ahn, D., Chung, H., Lee, H. W., Kang, K., Ko, P. W., Kim, N. S., et al. (2017). Smart gait-aid glasses for Parkinson's Disease patients. IEEE Trans. Biomed. Eng. 64, 2394–2402. doi: 10.1109/TBME.2017.2655344
Albert, J. A., Owolabi, V., Gebel, A., Brahms, C. M., Granacher, U., and Arnrich, B. (2020). Evaluation of the pose tracking performance of the Azure Kinect and Kinect v2 for gait analysis in comparison with a gold standard: a pilot study. Sensors 20:5104. doi: 10.3390/s20185104
Alberts, J. L., Hirsch, J. R., Koop, M. M., Schindler, D. D., Kana, D. E., Linder, S. M., et al. (2015a). Using accelerometer and gyroscopic measures to quantify postural stability. J. Athl. Train. 50, 578–588. doi: 10.4085/1062-6050-50.2.01
Alberts, J. L., Thota, A., Hirsch, J., Ozinga, S., Dey, T., Schindler, D. D., et al. (2015b). Quantification of the balance error scoring system with mobile technology. Med. Sci. Sports Exerc. 47:2233. doi: 10.1249/MSS.0000000000000656
Alharthi, A. S., Yunas, S. U., and Ozanyan, K. B. (2019). Deep learning for monitoring of human gait: a review. IEEE Sens. J. 19, 9575–9591. doi: 10.1109/JSEN.2019.2928777
Allali, G., Laidet, M., Armand, S., Elsworth-Edelsten, C., Assal, F., and Lalive, P. H. (2016). Stride time variability as a marker for higher level of gait control in multiple sclerosis: its association with fear of falling. J. Neural Transm. 123, 595–599. doi: 10.1007/s00702-016-1551-4
Ancona, S., Faraci, F. D., Khatab, E., Fiorillo, L., Gnarra, O., Nef, T., et al. (2021). Wearables in the home-based assessment of abnormal movements in Parkinson's disease: a systematic review of the literature. J. Neurol. 269, 100–110. doi: 10.1007/s00415-020-10350-3
Andriluka, M., Pishchulin, L., Gehler, P., and Schiele, B. (2014). “2D human pose estimation: New benchmark and state of the art analysis,” in Proceedings of the IEEE Conference on Computer Vision and Pattern Recognition (Columbus, OH: IEEE), 3686–3693.
Apple Inc. (2020). Use fall detection with Apple Watch. Available online at: https://support.apple.com/en-us/HT208944 (accessed August 27, 2021).
Arafsha, F., Hanna, C., Aboualmagd, A., Fraser, S., and El Saddik, A. (2018). Instrumented wireless smartinsole system for mobile gait analysis: a validation pilot study with tekscan strideway. J. Sens. Actuator Netw. 7:36. doi: 10.3390/jsan7030036
Arpan, I., Fling, B., Powers, K., Horak, F. B., and Spain, R. I. (2020). Structural neural correlates of impaired postural control in people with secondary progressive multiple sclerosis. Int. J. MS Care 22, 123–128. doi: 10.7224/1537-2073.2019-004
Artusi, C. A., Mishra, M., Latimer, P., Vizcarra, J. A., Lopiano, L., Maetzler, W., et al. (2018). Integration of technology-based outcome measures in clinical trials of Parkinson and other neurodegenerative diseases. Parkinsonism Relat. Disord. 46, S53–S56. doi: 10.1016/j.parkreldis.2017.07.022
Auvinet, E., Multon, F., Aubin, C.-E., Meunier, J., and Raison, M. (2015). Detection of gait cycles in treadmill walking using a kinect. Gait Posture 41, 722–725. doi: 10.1016/j.gaitpost.2014.08.006
Baker, R. (2013). Measuring Walking: A Handbook of Clinical Gait Analysis, 1st Edn. London: Mac Keith Press.
Behrens, J., Pfüller, C., Mansow-Model, S., Otte, K., Paul, F., and Brandt, A. U. (2014). Using perceptive computing in multiple sclerosis-the short maximum speed walk test. J. Neuroeng. Rehabil. 11, 1–10. doi: 10.1186/1743-0003-11-89
Bejarano, N. C., Ambrosini, E., Pedrocchi, A., Ferrigno, G., Monticone, M., and Ferrante, S. (2014). A novel adaptive, real-time algorithm to detect gait events from wearable sensors. IEEE Trans. Neural Syst. Rehabil. Eng. 23, 413–422. doi: 10.1109/TNSRE.2014.2337914
Benedetti, M., Merlo, A., and Leardini, A. (2013). Inter-laboratory consistency of gait analysis measurements. Gait Posture 38, 934–939. doi: 10.1016/j.gaitpost.2013.04.022
Benedetti, M., Piperno, R., Simoncini, L., Bonato, P., Tonini, A., and Giannini, S. (1999). Gait abnormalities in minimally impaired multiple sclerosis patients. Multiple Sclerosis J. 5, 363–368. doi: 10.1177/135245859900500510
Berg, K., Wood-Dauphine, S., Williams, J. I., and Gayton, D. (1989). Measuring balance in the elderly: preliminary development of an instrument. Physiotherapy Canada 41, 304–311. doi: 10.3138/ptc.41.6.304
Bertoli, M., Cereatti, A., Trojaniello, D., Avanzino, L., Pelosin, E., Del Din, S., et al. (2018). Estimation of spatio-temporal parameters of gait from magneto-inertial measurement units: multicenter validation among Parkinson, mildly cognitively impaired and healthy older adults. Biomed. Eng. Online 17, 1–14. doi: 10.1186/s12938-018-0488-2
Bethoux, F., Varsanik, J. S., Chevalier, T. W., Halpern, E. F., Stough, D., and Kimmel, Z. M. (2018). Walking speed measurement with an ambient measurement system (ams) in patients with multiple sclerosis and walking impairment. Gait Posture 61, 393–397. doi: 10.1016/j.gaitpost.2018.01.033
Bigy, A. A. M., Banitsas, K., Badii, A., and Cosmas, J. (2015). “Recognition of postures and freezing of gait in Parkinson's disease patients using Microsoft Kinect sensor,” in 2015 7th International IEEE/EMBS Conference on Neural Engineering (NER) (Montpellier: IEEE), 731–734.
Blin, O., Ferrandez, A., and Serratrice, G. (1990). Quantitative analysis of gait in Parkinson patients: increased variability of stride length. J. Neurol. Sci. 98, 91–97. doi: 10.1016/0022-510X(90)90184-O
Bloem, B. R., Hausdorff, J. M., Visser, J. E., and Giladi, N. (2004). Falls and freezing of gait in Parkinson's disease: a review of two interconnected, episodic phenomena. Mov. Disord. 19, 871–884. doi: 10.1002/mds.20115
Boppana, A., and Anderson, A. P. (2019). DynaMo: dynamic body shape and motion capture with Intel RealSense cameras. J. Open Source Softw. 4:1466. doi: 10.21105/joss.01466
Bot, B., Suver, C., Neto, E., Kellen, M., Klein, A., Bare, C., et al. (2016). The mPower study, Parkinson disease mobile data collected using ResearchKit. Scientific Data 3:160011. doi: 10.1038/sdata.2016.11
Buckley, C., Alcock, L., McArdle, R., Rehman, R. Z. U., Din, S. D., Mazza, C., et al. (2019). The role of movement analysis in diagnosing and monitoring neurodegenerative conditions: Insights form gait and postural control. Brain Sci. 9:24. doi: 10.3390/brainsci9020034
Caldas, R., Mundt, M., Potthast, W., de Lima Neto, F. B., and Markert, B. (2017). A systematic review of gait analysis methods based on inertial sensors and adaptive algorithms. Gait Posture 57, 204–210. doi: 10.1016/j.gaitpost.2017.06.019
Cao, Z., Hidalgo, G., Simon, T., Wei, S.-E., and Sheikh, Y. (2019). OpenPose: realtime multi-person 2D pose estimation using part affinity fields. IEEE Trans. Pattern Anal. Mach. Intell. 43, 172–186. doi: 10.1109/TPAMI.2019.2929257
Casamassima, F., Ferrari, A., Milosevic, B., Ginis, P., Farella, E., and Rocchi, L. (2014). A wearable system for gait training in subjects with Parkinson's disease. Sensors 14, 6229–6246. doi: 10.3390/s140406229
Cebi, I., Scholten, M., Gharabaghi, A., and Weiss, D. (2020). Clinical and Kinematic correlates of favorable gait outcomes from subthalamic stimulation. Front. Neurol. 11:212. doi: 10.3389/fneur.2020.00212
Celik, Y., Stuart, S., Woo, W. L., and Godfrey, A. (2021). Gait analysis in neurological populations: progression in the use of wearables. Med. Eng. Phys. 87, 9–29. doi: 10.1016/j.medengphy.2020.11.005
Cerrito, A., Bichsel, L., Radlinger, L., and Schmid, S. (2015). Reliability and validity of a smartphone-based application for the quantification of the sit-to-stand movement in healthy seniors. Gait Posture 41, 409–413. doi: 10.1016/j.gaitpost.2014.11.001
Chatzaki, C., Skaramagkas, V., Tachos, N., Christodoulakis, G., Maniadi, E., Kefalopoulou, Z., et al. (2021). The smart-insole dataset: Gait analysis using wearable sensors with a focus on elderly and Parkinson's patients. Sensors 21:2821. doi: 10.3390/s21082821
Chen, C.-H., and Ramanan, D. (2017). “3D human pose estimation = 2D pose estimation + matching,” in Proceedings of the IEEE Conference on Computer Vision and Pattern Recognition (Honolulu, HI: IEEE), 7035–7043.
Chen, T., Fan, Y., Zhuang, X., Feng, D., Chen, Y., Chan, P., et al. (2018). Postural sway in patients with early parkinson's disease performing cognitive tasks while standing. Neurol Res. 40, 491–498. doi: 10.1080/01616412.2018.1451017
Chen, W., and Yan, X. (2020). Progress in achieving high-performance piezoresistive and capacitive flexible pressure sensors: a review. J. Mater. Sci. Technol. 43, 175–188. doi: 10.1016/j.jmst.2019.11.010
Chomiak, T., Xian, W., Pei, Z., and Hu, B. (2019). A novel single-sensor-based method for the detection of gait-cycle breakdown and freezing of gait in Parkinson's disease. J. Neural Transm. 126, 1029–1036. doi: 10.1007/s00702-019-02020-0
Clark, R. A., Mentiplay, B. F., Hough, E., and Pua, Y. H. (2019). Three-dimensional cameras and skeleton pose tracking for physical function assessment: a review of uses, validity, current developments and kinect alternatives. Gait Posture 68, 193–200. doi: 10.1016/j.gaitpost.2018.11.029
Clark, R. A., Pua, Y.-H., Fortin, K., Ritchie, C., Webster, K. E., Denehy, L., et al. (2012). Validity of the Microsoft Kinect for assessment of postural control. Gait Posture 36, 372–377. doi: 10.1016/j.gaitpost.2012.03.033
Clarke, T. E. (1980). The Pressure Distribution Under the Foot During Barefoot Walking. State College, PA: The Pennsylvania State University.
Cofré Lizama, L. E., Bastani, A., van der Walt, A., Kilpatrick, T., Khan, F., and Galea, M. P. (2020). Increased ankle muscle coactivation in the early stages of multiple sclerosis. Mult. Scler. J. Exp. Transl. Clin. 6:2055217320905870. doi: 10.1177/2055217320905870
Colyer, S. L., Evans, M., Cosker, D. P., and Salo, A. I. (2018). A review of the evolution of vision-based motion analysis and the integration of advanced computer vision methods towards developing a markerless system. Sports Med. Open 4, 1–15. doi: 10.1186/s40798-018-0139-y
Comber, L., Galvin, R., and Coote, S. (2017). Gait deficits in people with Multiple sclerosis: a systematic review and meta-analysis. Gait Posture 51, 25–35. doi: 10.1016/j.gaitpost.2016.09.026
Craig, J. J., Bruetsch, A. P., Lynch, S. G., Horak, F. B., and Huisinga, J. M. (2017). Instrumented balance and walking assessments in persons with Multiple Sclerosis show strong test-retest reliability. J. Neuroeng. Rehabil. 14, 1–9. doi: 10.1186/s12984-017-0251-0
Cronin, N. J., Rantalainen, T., Ahtiainen, J. P., Hynynen, E., and Waller, B. (2019). Markerless 2D kinematic analysis of underwater running: a deep learning approach. J Biomech. 87, 75–82. doi: 10.1016/j.jbiomech.2019.02.021
Curtze, C., Nutt, J. G., Carlson-Kuhta, P., Mancini, M., and Horak, F. B. (2015). Levodopa is a double-edged sword for balance and gait in people with Parkinson's disease. Mov. Disord. 30, 1361–1370. doi: 10.1002/mds.26269
D'Antonio, E., Taborri, J., Mileti, I., Rossi, S., and Patané, F. (2021). Validation of a 3D markerless system for gait analysis based on OpenPose and two RGB webcams. IEEE Sens. J. 21, 17064–17075. doi: 10.1109/JSEN.2021.3081188
D'Antonio, E., Taborri, J., Palermo, E., Rossi, S., and Patané, F. (2020). “A markerless system for gait analysis based on OpenPose library,” in 2020 IEEE International Instrumentation and Measurement Technology Conference (I2MTC) (Dubrovnik: IEEE), 1–6.
de Vries, W., Veeger, H., Baten, C., and van der Helm, F. (2009). Magnetic distortion in motion labs, implications for validating inertial magnetic sensors. Gait Posture 29, 535–541. doi: 10.1016/j.gaitpost.2008.12.004
de-la Herran, A. M., Garcia-Zapirain, B., and Mendez-Zorrilla, A. (2014). Gait analysis methods: an overview of wearable and non-wearable systems, highlighting clinical applications. Sensors 14, 3362–3394. doi: 10.3390/s140203362
Deuschl, G., Beghi, E., Fazekas, F., Varga, T., Christoforidi, K. A., Sipido, E., et al. (2020). The burden of neurological diseases in Europe: an analysis for the Global Burden of Disease Study 2017. Lancet Public Health 5, e551–e567. doi: 10.1016/S2468-2667(20)30190-0
Dewey, D. C., Miocinovic, S., Bernstein, I., Khemani, P., Dewey, I. I. I. R. B., et al. (2014). Automated gait and balance parameters diagnose and correlate with severity in Parkinson disease. J. Neurol. Sci. 345, 131–138. doi: 10.1016/j.jns.2014.07.026
Diaz, S., Stephenson, J. B., and Labrador, M. A. (2020). Use of wearable sensor technology in gait, balance, and range of motion analysis. Appl. Sci. 10:234. doi: 10.3390/app10010234
Domínguez, A. G., Hochsprung, A., Duarte, S. P., Camino, C. P., Rodríguez, A. A., Durán, C., et al. (2020). Study for the validation of the FeetMe® integrated sensor insole system compared to GAITRite® system to assess the characteristics of the gait in patients with Multiple Sclerosis (4038). Neurology 94(15 Suppl.), 4038. Retrieved from: https://n.neurology.org/content/94/15_Supplement/4038
Donath, L., Faude, O., Lichtenstein, E., Pagenstert, G., Nesch, C., and Mndermann, A. (2016). Mobile inertial sensor based gait analysis: validity and reliability of spatiotemporal gait characteristics in healthy seniors. Gait Posture 49, 371–374. doi: 10.1016/j.gaitpost.2016.07.269
Dorsey, E., Omberg, L., Waddell, E., Adams, J. L., Adams, R., Ali, M. R., et al. (2020). Deep phenotyping of Parkinson's disease. J. Parkinsons Dis. 10, 855–873. doi: 10.3233/JPD-202006
Elkurdi, A., Soufian, M., and Nefti-Meziani, S. (2018). Gait speeds classifications by supervised modulation based machine-learning using kinect camera. Med. Res. Innovat. 2, 1–6. doi: 10.15761/MRI.1000147
Ellis, R. J., Ng, Y. S., Zhu, S., Tan, D. M., Anderson, B., Schlaug, G., et al. (2015). A validated smartphone-based assessment of gait and gait variability in Parkinson's disease. PLoS ONE 10:e0141694. doi: 10.1371/journal.pone.0141694
Eltoukhy, M., Kuenze, C., Oh, J., Jacopetti, M., Wooten, S., and Signorile, J. (2017). Microsoft Kinect can distinguish differences in over-ground gait between older persons with and without Parkinson's disease. Med. Eng. Phys. 44, 1–7. doi: 10.1016/j.medengphy.2017.03.007
Espay, A. J., Hausdorff, J. M., Sánchez-Ferro, Á., Klucken, J., Merola, A., Bonato, P., et al. (2019). A roadmap for implementation of patient-centered digital outcome measures in Parkinson's disease obtained using mobile health technologies. Mov. Disord. 34, 657–663. doi: 10.1002/mds.27671
Fang, H.-S., Xie, S., Tai, Y.-W., and Lu, C. (2017). “RMPE: regional multi-person pose estimation,” in Proceedings of the IEEE International Conference on Computer Vision (Venice: IEEE), 2334–2343.
Farid, L., Jacobs, D., Do Santos, J., Simon, O., Gracies, J.-M., and Hutin, E. (2021). FeetMe®monitor-connected insoles are a valid and reliable alternative for the evaluation of gait speed after stroke. Top. Stroke Rehabil. 28, 127–134. doi: 10.1080/10749357.2020.1792717
Feichtenhofer, C., Pinz, A., and Zisserman, A. (2017). “Detect to track and track to detect,” in Proceedings of the IEEE International Conference on Computer Vision (ICCV) (Venice: IEEE), 3038–3046.
Ferrari, A., Ginis, P., Nieuwboer, A., Greenlaw, R., Muddiman, A., and Chiari, L. (2016). “Handling gait impairments of persons with Parkinson's disease by means of real-time biofeedback in a daily life environment,” in Inclusive Smart Cities and Digital Health, Vol. 9677, eds C. K. Chang, L. Chiari, Y. Cao, H. Jin, M. Mokhtari, and H. Aloulou (Cham: Springer International Publishing), 250–261.
Filli, L., Sutter, T., Easthope, C. S., Killeen, T., Meyer, C., Reuter, K., et al. (2018). Profiling walking dysfunction in multiple sclerosis: characterisation, classification and progression over time. Sci. Rep. 8, 1–13. doi: 10.1038/s41598-018-22676-0
Fino, P. C., and Mancini, M. (2020). Phase-dependent effects of closed-loop tactile feedback on gait stability in Parkinson's disease. IEEE Trans. Neural Syst. Rehabil. Eng. 28, 1636–1641. doi: 10.1109/TNSRE.2020.2997283
Franco, C., Fleury, A., Guméry, P.-Y., Diot, B., Demongeot, J., and Vuillerme, N. (2012). ibalance-abf: a smartphone-based audio-biofeedback balance system. IEEE Trans. Biomed. Eng. 60, 211–215. doi: 10.1109/TBME.2012.2222640
Gabel, M., Gilad-Bachrach, R., Renshaw, E., and Schuster, A. (2012). “Full body gait analysis with kinect,” in 2012 Annual International Conference of the IEEE Engineering in Medicine and Biology Society (EMBC) (San Diego, CA: IEEE), 1964–1967.
Galea, M. P., Cofre Lizama, L. E., Butzkueven, H., and Kilpatrick, T. J. (2017). Gait and balance deterioration over a 12-month period in multiple sclerosis patients with EDSS scores < /= 3.0. Neurorehabilitation 40, 277–284. doi: 10.3233/NRE-161413
Galna, B., Lord, S., Burn, D. J., and Rochester, L. (2015). Progression of gait dysfunction in incident Parkinson's disease: impact of medication and phenotype. Mov. Disord. 30, 359–367. doi: 10.1002/mds.26110
Gao, Z., Yu, Y., Zhou, Y., and Du, S. (2015). Leveraging two Kinect sensors for accurate full-body motion capture. Sensors 15, 24297–24317. doi: 10.3390/s150924297
Geng, J. (2011). Structured-light 3D surface imaging: a tutorial. Adv. Optics Photonics 3, 128–160. doi: 10.1364/AOP.3.000128
Gera, G., Fling, B. W., and Horak, F. B. (2020). Cerebellar white matter damage is associated with postural sway deficits in people with multiple sclerosis. Arch. Phys. Med. Rehabil. 101, 258–264. doi: 10.1016/j.apmr.2019.07.011
Ghislieri, M., Agostini, V., Rizzi, L., Knaflitz, M., and Lanotte, M. (2021). Atypical gait cycles in Parkinson's disease. Sensors 21:5079. doi: 10.3390/s21155079
Gholami, F., Trojan, D. A., Kövecses, J., Haddad, W. M., and Gholami, B. (2016). A microsoft kinect-based point-of-care gait assessment framework for multiple sclerosis patients. IEEE J. Biomed. Health Inform. 21, 1376–1385. doi: 10.1109/JBHI.2016.2593692
Giladi, N., Shabtai, H., Simon, E. S., Biran, S., Tal, J., and Korczyn, A. D. (2000). Construction of freezing of gait questionnaire for patients with Parkinsonism. Parkinsonism Relat. Disord. 6, 165–170. doi: 10.1016/S1353-8020(99)00062-0
Ginis, P., Heremans, E., Ferrari, A., Dockx, K., Canning, C. G., and Nieuwboer, A. (2017). Prolonged walking with a wearable system providing intelligent auditory input in people with Parkinson's disease. Front. Neurol. 8:128. doi: 10.3389/fneur.2017.00128
Ginis, P., Nieuwboer, A., Dorfman, M., Ferrari, A., Gazit, E., Canning, C. G., et al. (2016). Feasibility and effects of home-based smartphone-delivered automated feedback training for gait in people with Parkinson's disease: a pilot randomized controlled trial. Parkinsonism Relat. Disord. 22, 28–34. doi: 10.1016/j.parkreldis.2015.11.004
Gnther, M., Bartsch, R. P., Miron-Shahar, Y., Hassin-Baer, S., Inzelberg, R., Kurths, J., et al. (2019). Coupling between leg muscle activation and EEG during normal walking, intentional stops, and freezing of gait in Parkinson's disease. Front. Physiol. 10:870. doi: 10.3389/fphys.2019.00870
Gonzalez-Jorge, H., Rodríguez-Gonzálvez, P., Martínez-Sánchez, J., González-Aguilera, D., Arias, P., Gesto, M., et al. (2015). Metrological comparison between Kinect I and Kinect II sensors. Measurement 70, 21–26. doi: 10.1016/j.measurement.2015.03.042
Gouelle, A., Mégrot, F., Presedo, A., Husson, I., Yelnik, A., and Penneçot, G.-F. (2013). The gait variability index: a new way to quantify fluctuation magnitude of spatiotemporal parameters during gait. Gait Posture 38, 461–465. doi: 10.1016/j.gaitpost.2013.01.013
Graham, J. E., Ostir, G. V., Fisher, S. R., and Ottenbacher, K. J. (2008). Assessing walking speed in clinical research: a systematic review. J. Eval. Clin. Pract. 14, 552–562. doi: 10.1111/j.1365-2753.2007.00917.x
Graving, J. M., Chae, D., Naik, H., Li, L., Koger, B., Costelloe, B. R., et al. (2019). DeepPoseKit, a software toolkit for fast and robust animal pose estimation using deep learning. Elife 8:e47994. doi: 10.7554/eLife.47994
Gray, P., and Hildebrand, K. (2000). Fall risk factors in Parkinson's disease. J. Neurosci. Nurs. 32, 222–228. doi: 10.1097/01376517-200008000-00006
Gu, X., Deligianni, F., Lo, B., Chen, W., and Yang, G.-Z. (2018). “Markerless gait analysis based on a single RGB camera,” in 2018 IEEE 15th International Conference on Wearable and Implantable Body Sensor Networks (BSN) (Las Vegas, NV: IEEE), 42–45.
Guess, T. M., Razu, S., Jahandar, A., Skubic, M., and Huo, Z. (2017). Comparison of 3D joint angles measured with the Kinect 2.0 skeletal tracker versus a marker-based motion capture system. J. Appl. Biomech. 33, 176–181. doi: 10.1123/jab.2016-0107
Gurchiek, R. D., Choquette, R. H., Beynnon, B. D., Slauterbeck, J. R., Tourville, T. W., Toth, M. J., et al. (2019). Open-source remote gait analysis: A post-surgery patient monitoring application. Sci. Rep. 9, 1–10. doi: 10.1038/s41598-019-54399-1
Gutta, V., Fallavollita, P., Baddour, N., and Lemaire, E. D. (2021). Development of a smart hallway for marker-less human foot tracking and stride analysis. IEEE J. Transl. Eng. Health Med. 9, 1–12. doi: 10.1109/JTEHM.2021.3069353
Harrington, W., Greenberg, A., King, E., McNames, J., Holmstrom, L., Horak, F. B., et al. (2016). Alleviating freezing of gait using phase-dependent tactile biofeedback. Conf. Proc. IEEE Eng. Med. Biol. Soc. 2016, 5841–5844. doi: 10.1109/EMBC.2016.7592056
Hausamann, P., Sinnott, C. B., Daumer, M., and MacNeilage, P. R. (2021). Evaluation of the Intel RealSense T265 for tracking natural human head motion. Sci. Rep. 11:12486. doi: 10.1038/s41598-021-91861-5
He, K., Zhang, X., Ren, S., and Sun, J. (2016). “Deep residual learning for image recognition,” in Proceedings of the IEEE Conference on Computer Vision and Pattern Recognition (Las Vegas, NV: IEEE), 770–778
Herman, T., Weiss, A., Brozgol, M., Giladi, N., and Hausdorff, J. M. (2014). Gait and balance in Parkinson's disease subtypes: objective measures and classification considerations. J. Neurol. 261, 2401–2410. doi: 10.1007/s00415-014-7513-6
Horak, F., King, L., and Mancini, M. (2015). Role of body-worn movement monitor technology for balance and gait rehabilitation. Phys. Ther. 95, 461–470. doi: 10.2522/ptj.20140253
Horak, F. B., and Mancini, M. (2013). Objective biomarkers of balance and gait for Parkinson's disease using body-worn sensors. Mov. Disord. 28, 1544–1551. doi: 10.1002/mds.25684
Horak, F. B., Mancini, M., Carlson-Kuhta, P., Nutt, J. G., and Salarian, A. (2016). Balance and gait represent independent domains of mobility in Parkinson disease. Phys. Ther. 96, 1364–1371. doi: 10.2522/ptj.20150580
Horn, L. B., Rice, T., Stoskus, J. L., Lambert, K. H., Dannenbaum, E., and Scherer, M. R. (2015). Measurement characteristics and clinical utility of the clinical test of sensory interaction on balance (ctsib) and modified ctsib in individuals with vestibular dysfunction. Arch. Phys. Med. Rehabil. 96, 1747–1748. doi: 10.1016/j.apmr.2015.04.003
Hsieh, K. L., Roach, K. L., Wajda, D. A., and Sosnoff, J. J. (2019). Smartphone technology can measure postural stability and discriminate fall risk in older adults. Gait Posture 67, 160–165. doi: 10.1016/j.gaitpost.2018.10.005
Hsieh, K. L., and Sosnoff, J. J. (2021). Smartphone accelerometry to assess postural control in individuals with Multiple Sclerosis. Gait Posture 84:114–119. doi: 10.1016/j.gaitpost.2020.11.011
Hu, K., Wang, Z., Mei, S., Martens, K. A. E., Yao, T., Lewis, S. J., et al. (2019). Vision-based freezing of gait detection with anatomic directed graph representation. IEEE J. Biomed. Health Inform. 24, 1215–1225. doi: 10.1109/JBHI.2019.2923209
Ibrahim, A. A., Küderle, A., Gassner, H., Klucken, J., Eskofier, B. M., and Kluge, F. (2020). Inertial sensor-based gait parameters reflect patient-reported fatigue in Multiple Sclerosis. J. Neuroeng. Rehabil. 17:165. doi: 10.1186/s12984-020-00798-9
Iijima, M., Mitoma, H., Uchiyama, S., and Kitagawa, K. (2017). Long-term monitoring gait analysis using a wearable device in daily lives of patients with Parkinson's disease: the efficacy of selegiline hydrochloride for gait disturbance. Front. Neurol. 8:542. doi: 10.3389/fneur.2017.00542
Insafutdinov, E., Pishchulin, L., Andres, B., Andriluka, M., and Schiele, B. (2016). “DeeperCut: a deeper, stronger, and faster multi-person pose estimation model,” in European Conference on Computer Vision (Amsterdam: Springer), 34–50.
Ionescu, C., Papava, D., Olaru, V., and Sminchisescu, C. (2013). Human3.6M: Large scale datasets and predictive methods for 3D human sensing in natural environments. IEEE Trans. Pattern Anal. Mach. Intell. 36, 1325–1339. doi: 10.1109/TPAMI.2013.248
Iskakov, K., Burkov, E., Lempitsky, V., and Malkov, Y. (2019). “Learnable triangulation of human pose,” in Proceedings of the IEEE/CVF International Conference on Computer Vision (Seoul), 7718–7727.
Kalron, A., Aloni, R., Dolev, M., Frid, L., Givon, U., and Menascu, S. (2018). The relationship between gait variability and cognitive functions differs between fallers and non-fallers in ms. J. Neural Transm. 125, 945–952. doi: 10.1007/s00702-018-1843-y
Karashchuk, P., Rupp, K. L., Dickinson, E. S., Sanders, E., Azim, E., Brunton, B. W., et al. (2020). Anipose: a toolkit for robust markerless 3D pose estimation. BioRxiv. doi: 10.1101/2020.05.26.117325
Karatsidis, A., Bellusci, G., Schepers, H. M., De Zee, M., Andersen, M. S., and Veltink, P. H. (2017). Estimation of ground reaction forces and moments during gait using only inertial motion capture. Sensors 17:75. doi: 10.3390/s17010075
Kelleher, K. J., Spence, W., Solomonidis, S., and Apatsidis, D. (2010). The characterisation of gait patterns of people with Multiple Sclerosis. Disabil Rehabil. 32, 1242–1250. doi: 10.3109/09638280903464497
Keselman, L., Iselin Woodfill, J., Grunnet-Jepsen, A., and Bhowmik, A. (2017). “Intel RealSense stereoscopic depth cameras,” in Proceedings of the IEEE Conference on Computer Vision and Pattern Recognition Workshops (Honolulu), 1–10.
Kieseier, B. C., and Pozzilli, C. (2012). Assessing walking disability in Multiple Sclerosis. Multiple Sclerosis J. 18, 914–924. doi: 10.1177/1352458512444498
Kolb, A., Barth, E., Koch, R., and Larsen, R. (2010). Time-of-flight cameras in computer graphics. Comput. Graph. Forum 29, 141–159. doi: 10.1111/j.1467-8659.2009.01583.x
Kosse, N. M., Caljouw, S., Vervoort, D., Vuillerme, N., and Lamoth, C. J. (2015). Validity and reliability of gait and postural control analysis using the tri-axial accelerometer of the ipod touch. Ann. Biomed. Eng. 43, 1935–1946. doi: 10.1007/s10439-014-1232-0
Laidig, D., Jocham, A. J., Guggenberger, B., Adamer, K., Fischer, M., and Seel, T. (2021). Calibration-free gait assessment by foot-worn inertial sensors. Front. Digit. Health 3:147. doi: 10.3389/fdgth.2021.736418
Lakshminarayana, R., Wang, D., Burn, D., Chaudhuri, K. R., Galtrey, C., Guzman, N. V., et al. (2017). Using a smartphone-based self-management platform to support medication adherence and clinical consultation in Parkinson's disease. npj Parkinsons Dis. 3, 1–10. doi: 10.1038/s41531-016-0003-z
Leone, C., Kalron, A., Smedal, T., Normann, B., Wens, I., Eijnde, B. O., et al. (2018). Effects of rehabilitation on gait pattern at usual and fast speeds depend on walking impairment level in multiple sclerosis. Int. J. MS Care 20, 199–209. doi: 10.7224/1537-2073.2015-078
Li, J., Wang, C., Zhu, H., Mao, Y., Fang, H.-S., and Lu, C. (2019). “CrowdPose: Efficient crowded scenes pose estimation and a new benchmark,” in Proceedings of the IEEE/CVF Conference on Computer Vision and Pattern Recognition (Long Beach, CA: IEEE), 10863–10872.
Li, M. H., Mestre, T. A., Fox, S. H., and Taati, B. (2018). Vision-based assessment of Parkinsonism and levodopa-induced dyskinesia with pose estimation. J. Neuroeng. Rehabil. 15:97. doi: 10.1186/s12984-018-0446-z
Lipsmeier, F., Taylor, K. I., Kilchenmann, T., Wolf, D., Scotland, A., Schjodt-Eriksen, J., et al. (2018). Evaluation of smartphone-based testing to generate exploratory outcome measures in a phase 1 Parkinson's disease clinical trial. Mov. Disord. 33, 1287–1297. doi: 10.1002/mds.27376
Loper, M., Mahmood, N., Romero, J., Pons-Moll, G., and Black, M. J. (2015). SMPL: a skinned multi-person linear model. ACM Trans. Graph. 34, 1–16. doi: 10.1145/2816795.2818013
Lord, S., Galna, B., and Rochester, L. (2013). Moving forward on gait measurement: toward a more refined approach. Mov. Disord. 28, 1534–1543. doi: 10.1002/mds.25545
Ma, C. Z.-H., Wong, D. W.-C., Lam, W. K., Wan, A. H.-P., and Lee, W. C.-C. (2016). Balance improvement effects of biofeedback systems with state-of-the-art wearable sensors: a systematic review. Sensors 16:434. doi: 10.3390/s16040434
Ma, L., Mi, T.-M., Jia, Q., Han, C., Chhetri, J. K., and Chan, P. (2020a). Gait variability is sensitive to detect Parkinson's disease patients at high fall risk. Int. J. Neurosci. 1–13. doi: 10.1080/00207454.2020.1849189. [Epub ahead of print].
Ma, Y., Sheng, B., Hart, R., and Zhang, Y. (2020b). “The validity of a dual Azure Kinect-based motion capture system for gait analysis: a preliminary study,” in 2020 Asia-Pacific Signal and Information Processing Association Annual Summit and Conference (APSIPA ASC) (Auckland: IEEE), 1201–1206.
Macpherson, T. W., Taylor, J., McBain, T., Weston, M., and Spears, I. R. (2016). Real-time measurement of pelvis and trunk kinematics during treadmill locomotion using a low-cost depth-sensing camera: a concurrent validity study. J. Biomech. 49, 474–478. doi: 10.1016/j.jbiomech.2015.12.008
Maetzler, W., Liepelt, I., and Berg, D. (2009). Progression of Parkinson's disease in the clinical phase: potential markers. Lancet Neurol. 8, 1158–1171. doi: 10.1016/S1474-4422(09)70291-1
Mancini, M., Carlson-Kuhta, P., Zampieri, C., Nutt, J. G., Chiari, L., and Horak, F. B. (2012a). Postural sway as a marker of progression in Parkinson's disease: a pilot longitudinal study. Gait Posture 36, 471–476. doi: 10.1016/j.gaitpost.2012.04.010
Mancini, M., and Horak, F. B. (2016). Potential of APDM mobility lab for the monitoring of the progression of Parkinson's disease. Expert Rev. Med. Devices 13, 455–462. doi: 10.1586/17434440.2016.1153421
Mancini, M., Horak, F. B., Zampieri, C., Carlson-Kuhta, P., Nutt, J. G., and Chiari, L. (2011). Trunk accelerometry reveals postural instability in untreated Parkinson's disease. Parkinsonism Relat. Disord. 17, 557–562. doi: 10.1016/j.parkreldis.2011.05.010
Mancini, M., Salarian, A., Carlson-Kuhta, P., Zampieri, C., King, L., Chiari, L., et al. (2012b). ISway: a sensitive, valid and reliable measure of postural control. J. Neuroeng. Rehabil. 9:59. doi: 10.1186/1743-0003-9-59
Mancini, M., Shah, V. V., Stuart, S., Curtze, C., Horak, F. B., Safarpour, D., et al. (2021). Measuring freezing of gait during daily-life: an open-source, wearable sensors approach. J. Neuroeng. Rehabil. 18:1. doi: 10.1186/s12984-020-00774-3
Mancini, M., Smulders, K., Harker, G., Stuart, S., and Nutt, J. G. (2018). Assessment of the ability of open- and closed-loop cueing to improve turning and freezing in people with Parkinson's disease. Scientific Rep. 8:12773. doi: 10.1038/s41598-018-31156-4
Manor, B., Yu, W., Zhu, H., Harrison, R., Lo, O.-Y., Lipsitz, L., et al. (2018). Smartphone app-based assessment of gait during normal and dual-task walking: demonstration of validity and reliability. JMIR Mhealth Uhealth 6:e8815. doi: 10.2196/mhealth.8815
Mariani, B., Jimnez, M. C., Vingerhoets, F. J. G., and Aminian, K. (2013). On-shoe wearable sensors for gait and turning assessment of patients with Parkinson's disease. IEEE Trans. Biomed. Eng. 60, 155–158. doi: 10.1109/TBME.2012.2227317
Marín, J., Blanco, T., de la Torre, J., and Marín, J. J. (2020). Gait analysis in a box: a system based on magnetometer-free IMUs or clusters of optical markers with automatic event detection. Sensors 20:3338. doi: 10.3390/s20123338
Marques, D. L., Neiva, H. P., Pires, I. M., Zdravevski, E., Mihajlov, M., Garcia, N. M., et al. (2021). An experimental study on the validity and reliability of a smartphone application to acquire temporal variables during the single sit-to-stand test with older adults. Sensors 21:2050. doi: 10.3390/s21062050
Martin, C. L., Phillips, B. A., Kilpatrick, T., Butzkueven, H., Tubridy, N., McDonald, E., et al. (2006). Gait and balance impairment in early Multiple Sclerosis in the absence of clinical disability. Multiple Sclerosis J. 12, 620–628. doi: 10.1177/1352458506070658
Martinez-Mendez, R., Sekine, M., and Tamura, T. (2012). Postural sway parameters using a triaxial accelerometer: comparing elderly and young healthy adults. Comput. Methods Biomech. Biomed. Eng. 15, 899–910. doi: 10.1080/10255842.2011.565753
Mathis, A., Mamidanna, P., Cury, K. M., Abe, T., Murthy, V. N., Mathis, M. W., et al. (2018). DeepLabCut: markerless pose estimation of user-defined body parts with deep learning. Nat. Neurosci. 21, 1281–1289. doi: 10.1038/s41593-018-0209-y
Mathis, A., and Warren, R. (2018). On the inference speed and video-compression robustness of DeepLabCut. BioRxiv 457242. doi: 10.1101/457242
Mazilu, S., Blanke, U., Dorfman, M., Gazit, E., Mirelman, A. M., et al. (2015). A wearable assistant for gait training for Parkinson's disease with freezing of gait in out-of-the-lab environments. ACM Trans. Interact. Intell. Syst. 5, 1–31. doi: 10.1145/2701431
Mazumder, O., Khandelwal, P., Gavas, R., and Sinha, A. (2018). “Assessment of insole based gait feature variation with progression of Parkinson's disease,” in IEEE Sensors Journal (New Delhi: IEEE), 1–4.
McLoughlin, J. V., Barr, C. J., Crotty, M., Sturnieks, D. L., and Lord, S. R. (2014). Six minutes of walking leads to reduced lower limb strength and increased postural sway in people with multiple sclerosis. Neurorehabilitation 35, 503–508. doi: 10.3233/NRE-141143
McLoughlin, J. V., Barr, C. J., Patritti, B., Crotty, M., Lord, S. R., and Sturnieks, D. L. (2016). Fatigue induced changes to kinematic and kinetic gait parameters following six minutes of walking in people with Multiple Sclerosis. Disabil. Rehabil. 38, 535–543. doi: 10.3109/09638288.2015.1047969
Mejia-Trujillo, J. D., Casta no-Pino, Y. J., Navarro, A., Arango-Paredes, J. D., Rincón, D., Valderrama, J., et al. (2019). “Kinect and Intel RealSense D435 comparison: a preliminary study for motion analysis,” in 2019 IEEE International Conference on E-health Networking, Application &Services (HealthCom) (Bogota: IEEE), 1–4.
Mellone, S., Tacconi, C., and Chiari, L. (2012). Validity of a smartphone-based instrumented timed up and go. Gait Posture 36, 163–165. doi: 10.1016/j.gaitpost.2012.02.006
Mentiplay, B. F., Perraton, L. G., Bower, K. J., Pua, Y.-H., McGaw, R., Heywood, S., et al. (2015). Gait assessment using the Microsoft Xbox One kinect: concurrent validity and inter-day reliability of spatiotemporal and kinematic variables. J. Biomech. 48, 2166–2170. doi: 10.1016/j.jbiomech.2015.05.021
Mickelborough, J., van der Linden, M., Tallis, R., and Ennos, A. (2004). Muscle activity during gait initiation in normal elderly people. Gait Posture 19, 50–57. doi: 10.1016/S0966-6362(03)00016-X
Monje, M. H., Foffani, G., Obeso, J., and Sánchez-Ferro, Á. (2019). New sensor and wearable technologies to aid in the diagnosis and treatment monitoring of Parkinson's disease. Ann. Rev. Biomed. Eng. 21, 111–143. doi: 10.1146/annurev-bioeng-062117-121036
Moon, G., Chang, J. Y., and Lee, K. M. (2019). “Camera distance-aware top-down approach for 3D multi-person pose estimation from a single RGB image,” in Proceedings of the IEEE/CVF International Conference on Computer Vision (Seoul: IEEE), 10133–10142.
Moon, Y., Wajda, D. A., Motl, R. W., and Sosnoff, J. J. (2015). Stride-time variability and fall risk in persons with multiple sclerosis. Mult. Scler. Int. 2015:964790. doi: 10.1155/2015/964790
Moore, S. T., MacDougall, H. G., and Ondo, W. G. (2008). Ambulatory monitoring of freezing of gait in Parkinson's disease. J. Neurosci. Methods 167, 340–348. doi: 10.1016/j.jneumeth.2007.08.023
Morita, P., Rocha, A., Shaker, G., Lee, D., Wei, J., Fong, B., et al. (2020). Comparative analysis of gait speed estimation using wideband and narrowband radars, thermal camera, and motion tracking suit technologies. J. Healthcare Inform. Res. 4, 215–237. doi: 10.1007/s41666-020-00071-7
Morris, R., Hickey, A., Del Din, S., Godfrey, A., Lord, S., and Rochester, L. (2017). A model of free-living gait: a factor analysis in Parkinson's disease. Gait Posture 52, 68–71. doi: 10.1016/j.gaitpost.2016.11.024
Morris, R., Stuart, S., McBarron, G., Fino, P. C., Mancini, M., and Curtze, C. (2019). Validity of mobility lab (version 2) for gait assessment in young adults, older adults and Parkinson's disease. Physiol. Meas. 40:095003. doi: 10.1088/1361-6579/ab4023
Motta, C., Palermo, E., Studer, V., Germanotta, M., Germani, G., Centonze, D., et al. (2016). Disability and fatigue can be objectively measured in multiple sclerosis. PLoS ONE 11:e0148997. doi: 10.1371/journal.pone.0148997
Mu noz, B., Casta no-Pino, Y. J., Paredes, J. D. A., and Navarro, A. (2018). “Automated gait analysis using a Kinect camera and wavelets,” in 2018 IEEE 20th International Conference on e-Health Networking, Applications and Services (Healthcom) (Ostrava: IEEE), 1–5.
Müller, R., Hamacher, D., Hansen, S., Oschmann, P., and Keune, P. M. (2021). Wearable inertial sensors are highly sensitive in the detection of gait disturbances and fatigue at early stages of multiple sclerosis. BMC Neurol. 21:337. doi: 10.1186/s12883-021-02361-y
Muthukrishnan, N., Abbas, J. J., Shill, H. A., and Krishnamurthi, N. (2019). Cueing paradigms to improve gait and posture in parkinson's disease: a narrative review. Sensors 19:5468. doi: 10.3390/s19245468
Nakano, N., Sakura, T., Ueda, K., Omura, L., Kimura, A., Iino, Y., et al. (2020). Evaluation of 3D markerless motion capture accuracy using OpenPose with multiple video cameras. Front. Sports Active Living 2:50. doi: 10.3389/fspor.2020.00050
Nantel, J., McDonald, J. C., and Bronte-Stewart, H. (2012). Effect of medication and STN-DBS on postural control in subjects with Parkinson's disease. Parkinsonism Relat. Disord. 18, 285–289. doi: 10.1016/j.parkreldis.2011.11.005
Napoli, A., Glass, S., Ward, C., Tucker, C., and Obeid, I. (2017). Performance analysis of a generalized motion capture system using Microsoft Kinect 2.0. Biomed. Signal Process. Control 38:265–280. doi: 10.1016/j.bspc.2017.06.006
Nath, T., Mathis, A., Chen, A. C., Patel, A., Bethge, M., and Mathis, M. W. (2019). Using DeepLabCut for 3D markerless pose estimation across species and behaviors. Nat. Protocols 14, 2152–2176. doi: 10.1038/s41596-019-0176-0
Needham, L., Evans, M., Cosker, D. P., Wade, L., McGuigan, P. M., Bilzon, J. L., et al. (2021). Human movement science in the wild: Can current deep-learning based pose estimation free us from the lab? BioRxiv. doi: 10.1101/2021.04.22.440909
Nguyen, A., Roth, N., Ghassemi, N. H., Hannink, J., Seel, T., Klucken, J., et al. (2019). Development and clinical validation of inertial sensor-based gait-clustering methods in Parkinson's disease. J. Neuroeng. Rehabil. 16, 1–14. doi: 10.1186/s12984-019-0548-2
Nutt, J. G., Bloem, B. R., Giladi, N., Hallett, M., Horak, F. B., and Nieuwboer, A. (2011). Freezing of gait: moving forward on a mysterious clinical phenomenon. Lancet Neurol. 10, 734–744. doi: 10.1016/S1474-4422(11)70143-0
Otte, K., Kayser, B., Mansow-Model, S., Verrel, J., Paul, F., Brandt, A. U., et al. (2016). Accuracy and reliability of the Kinect version 2 for clinical measurement of motor function. PLoS ONE 11:e0166532. doi: 10.1371/journal.pone.0166532
Ouyang, W., Chu, X., and Wang, X. (2014). “Multi-source deep learning for human pose estimation,” in Proceedings of the IEEE Conference on Computer Vision and Pattern Recognition (Columbus, OH: IEEE), 2329–2336.
Palmerini, L., Mellone, S., Avanzolini, G., Valzania, F., and Chiari, L. (2013). Quantification of motor impairment in Parkinson's disease using an instrumented timed up and go test. IEEE Trans. Neural Syst. Rehabil. Eng. 21, 664–673. doi: 10.1109/TNSRE.2012.2236577
Palmerini, L., Rocchi, L., Mazilu, S., Gazit, E., Hausdorff, J. M., and Chiari, L. (2017). Identification of characteristic motor patterns preceding freezing of gait in Parkinson's disease using wearable sensors. Front. Neurol. 8:394. doi: 10.3389/fneur.2017.00394
Pardoel, S., Kofman, J., Nantel, J., and Lemaire, E. D. (2019). Wearable-sensor-based detection and prediction of freezing of gait in Parkinson's disease: a review. Sensors 19:5141. doi: 10.3390/s19235141
Pardoel, S., Shalin, G., Nantel, J., Lemaire, E. D., and Kofman, J. (2021). Early detection of freezing of gait during walking using inertial measurement unit and plantar pressure distribution data. Sensors 21:2246. doi: 10.3390/s21062246
Pavlakos, G., Zhu, L., Zhou, X., and Daniilidis, K. (2018). “Learning to estimate 3D human pose and shape from a single color image,” in Proceedings of the IEEE Conference on Computer Vision and Pattern Recognition (Rustenburg), 459–468.
Pavllo, D., Feichtenhofer, C., Grangier, D., and Auli, M. (2019). “3D human pose estimation in video with temporal convolutions and semi-supervised training,” in Proceedings of the IEEE/CVF Conference on Computer Vision and Pattern Recognition, (Long Beach, CA: IEEE), 7753–7762.
Pfister, A., West, A. M., Bronner, S., and Noah, J. A. (2014). Comparative abilities of Microsoft Kinect and Vicon 3D motion capture for gait analysis. J. Med. Eng. Technol. 38, 274–280. doi: 10.3109/03091902.2014.909540
Pham, M. H., Elshehabi, M., Haertner, L., Del Din, S., Srulijes, K., Heger, T., et al. (2017). Validation of a step detection algorithm during straight walking and turning in patients with Parkinson's disease and older adults using an inertial measurement unit at the lower back. Front. Neurol. 8:457. doi: 10.3389/fneur.2017.00457
Pirker, W., and Katzenschlager, R. (2017). Gait disorders in adults and the elderly. Wiener Klinische Wochenschrift 129, 81–95. doi: 10.1007/s00508-016-1096-4
Pishchulin, L., Insafutdinov, E., Tang, S., Andres, B., Andriluka, M., Gehler, P. V., et al. (2016). “DeepCut: joint subset partition and labeling for multi person pose estimation,” in Proceedings of the IEEE Conference on Computer Vision and Pattern Recognition (Las Vegas, NV: IEEE), 4929–4937.
Podsiadlo, D., and Richardson, S. (1991). The timed up &go: a test of basic functional mobility for frail elderly persons. J. Am. Geriatr. Soc. 39, 142–148. doi: 10.1111/j.1532-5415.1991.tb01616.x
Ponciano, V., Pires, I. M., Ribeiro, F. R., and Spinsante, S. (2020). Sensors are capable to help in the measurement of the results of the timed-up and go test? a systematic review. J. Med. Syst. 44, 1–16. doi: 10.1007/s10916-020-01666-8
Popovic, M., Djuric-Jovicic, M., Radovanovic, S., Petrovic, I., and Kostic, V. (2010). A simple method to assess freezing of gait in Parkinson's disease patients. Brazilian J. Med. Biol. Res. 43, 883–889. doi: 10.1590/S0100-879X2010007500077
Pratap, A., Grant, D., Vegesna, A., Tummalacherla, M., Cohan, S., Deshpande, C., et al. (2020). Evaluating the utility of smartphone-based sensor assessments in persons with Multiple Sclerosis in the real-world using an app (elevateMS): Observational, prospective pilot digital health study. JMIR Mhealth Uhealth 8:e22108. doi: 10.2196/22108
Reches, T., Dagan, M., Herman, T., Gazit, E., Gouskova, N. A., Giladi, N., et al. (2020). Using wearable sensors and machine learning to automatically detect freezing of gait during a fog-provoking test. Sensors 20:4474. doi: 10.3390/s20164474
Remelius, J. G., Jones, S. L., House, J. D., Busa, M. A., Averill, J. L., Sugumaran, K., et al. (2012). Gait impairments in persons with Multiple Sclerosis across preferred and fixed walking speeds. Arch. Phys. Med. Rehabil. 93, 1637–1642. doi: 10.1016/j.apmr.2012.02.019
Rhodin, H., Spörri, J., Katircioglu, I., Constantin, V., Meyer, F., Müller, E., et al. (2018). “Learning monocular 3D human pose estimation from multi-view images,” in Proceedings of the IEEE Conference on Computer Vision and Pattern Recognition (Salt Lake City: IEEE), 8437–8446.
Rocha, A. P., Choupina, H. M. P., Vilas-Boas, M. d. C., Fernandes, J. M., and Cunha, J. P. S. (2018). System for automatic gait analysis based on a single RGB-D camera. PLoS ONE 13:e0201728. doi: 10.1371/journal.pone.0201728
Rochester, L., Galna, B., Lord, S., and Burn, D. (2014). The nature of dual-task interference during gait in incident Parkinson's disease. Neuroscience 265, 83–94. doi: 10.1016/j.neuroscience.2014.01.041
Rodgers, M. M., Mulcare, J. A., King, D. L., Mathews, T., Gupta, S. C., Glaser, R. M., et al. (1999). Gait characteristics of individuals with multiple sclerosis before and after a 6-month aerobic training program. J. Rehabil. Res. Dev. 36, 183–188.
Rodrguez-Martn, D., Sam, A., Prez-Lpez, C., Catal, A., Moreno Arostegui, J. M., Cabestany, J., et al. (2017). Home detection of freezing of gait using support vector machines through a single waist-worn triaxial accelerometer. PLoS ONE 12:e0171764. doi: 10.1371/journal.pone.0171764
Roeing, K. L., Hsieh, K. L., and Sosnoff, J. J. (2017). A systematic review of balance and fall risk assessments with mobile phone technology. Arch. Gerontol. Geriatr. 73, 222–226. doi: 10.1016/j.archger.2017.08.002
Roth, N., Küderle, A., Ullrich, M., Gladow, T., Marxreiter, F., Klucken, J., et al. (2021). Hidden Markov model based stride segmentation on unsupervised free-living gait data in Parkinson's disease patients. J. Neuroeng. Rehabil. 18:93. doi: 10.1186/s12984-021-00883-7
Roy, B., Nowak, R. J., Roda, R., Khokhar, B., Patwa, H. S., Lloyd, T., et al. (2020). Teleneurology during the covid-19 pandemic: a step forward in modernizing medical care. J. Neurol. Sci. 414:116930. doi: 10.1016/j.jns.2020.116930
Sabatini, A. M. (2006). Quaternion-based extended Kalman filter for determining orientation by inertial and magnetic sensing. IEEE Trans. Biomed. Eng. 53, 1346–1356. doi: 10.1109/TBME.2006.875664
Sabo, A., Mehdizadeh, S., Ng, K.-D., Iaboni, A., and Taati, B. (2020). Assessment of Parkinsonian gait in older adults with dementia via human pose tracking in video data. J. Neuroeng. Rehabil. 17, 1–10. doi: 10.1186/s12984-020-00728-9
Santinelli, F. B., van Emmerik, R. E., Silva, F. A., Imaizumi, L. F. I., Penedo, T., Canzonieri, A. M., et al. (2019). Saccadic eye movements are able to reduce body sway in mildly-affected people with multiple sclerosis. Mult. Scler. Relat. Disord. 30, 63–68. doi: 10.1016/j.msard.2019.02.005
Sato, K., Nagashima, Y., Mano, T., Iwata, A., and Toda, T. (2019). Quantifying normal and Parkinsonian gait features from home movies: practical application of a deep learning-based 2D pose estimator. PLoS ONE 14:e0223549. doi: 10.1371/journal.pone.0223549
Schauer, T. (2017). Sensing motion and muscle activity for feedback control of functional electrical stimulation: ten years of experience in Berlin. Ann. Rev. Control 44, 355–374. doi: 10.1016/j.arcontrol.2017.09.014
Schepers, M., Giuberti, M., and Bellusci, G. (2018). Xsens mvn: consistent tracking of human motion using inertial sensing. Xsens Technol, 1–8. doi: 10.13140/RG.2.2.22099.07205
Schlachetzki, J. C., Barth, J., Marxreiter, F., Gossler, J., Kohl, Z., Reinfelder, S., et al. (2017). Wearable sensors objectively measure gait parameters in Parkinson's disease. PLoS ONE 12:e0183989. doi: 10.1371/journal.pone.0183989
Schlenstedt, C., Muthuraman, M., Witt, K., Weisser, B., Fasano, A., and Deuschl, G. (2016). Postural control and freezing of gait in Parkinson's disease. Parkinsonism Relat. Disord. 24, 107–112. doi: 10.1016/j.parkreldis.2015.12.011
Schlenstedt, C., Peterson, D. S., and Mancini, M. (2020). The effect of tactile feedback on gait initiation in people with Parkinson's disease: a pilot study. Gait Posture 80, 240–245. doi: 10.1016/j.gaitpost.2020.06.001
Schmitz, A., Ye, M., Shapiro, R., Yang, R., and Noehren, B. (2014). Accuracy and repeatability of joint angles measured using a single camera markerless motion capture system. J. Biomech. 47, 587–591. doi: 10.1016/j.jbiomech.2013.11.031
Seel, T., Kok, M., and McGinnis, R. S. (2020). Inertial sensors applications and challenges in a nutshell. Sensors 20:6221. doi: 10.3390/s20216221
Seel, T., Landgraf, L., and Schauer, T. (2014a). Online gait phase detection with automatic adaption to gait velocity changes using accelerometers and gyroscopes. Biomedizinische Technik 59, S795–S798. doi: 10.1515/bmt-2014-5011
Seel, T., Raisch, J., and Schauer, T. (2014b). IMU-based joint angle measurement for gait analysis. Sensors 14, 6891–6909. doi: 10.3390/s140406891
Shah, V. V., McNames, J., Mancini, M., Carlson-Kuhta, P., Spain, R. I., Nutt, J. G., et al. (2020). Laboratory versus daily life gait characteristics in patients with Multiple Sclerosis, Parkinson's disease, and matched controls. J. Neuroeng. Rehabil. 17, 1–12. doi: 10.1186/s12984-020-00781-4
Shalin, G., Pardoel, S., Nantel, J., Lemaire, E. D., and Kofman, J. (2020). “Prediction of freezing of gait in Parkinson's disease from foot plantar-pressure arrays using a convolutional neural network,” in 2020 42nd Annual International Conference of the IEEE Engineering in Medicine &Biology Society (EMBC) (Montreal, QC,: IEEE), 244–247.
Shanahan, C. J., Boonstra, F., Cofré Lizama, L. E., Strik, M., Moffat, B. A., Khan, F., et al. (2018). Technologies for advanced gait and balance assessments in people with Multiple Sclerosis. Front. Neurol. 8:708. doi: 10.3389/fneur.2017.00708
Shin, J. H., Yu, R., Ong, J. N., Lee, C. Y., Jeon, S. H., Park, H., et al. (2021). Quantitative gait analysis using a pose-estimation algorithm with a single 2D-video of Parkinson's disease patients. J. Parkinsons Dis. 11, 1271–1283. doi: 10.3233/JPD-212544
Shotton, J., Fitzgibbon, A., Cook, M., Sharp, T., Finocchio, M., Moore, R., et al. (2011). “Real-time human pose recognition in parts from single depth images” in Conference on Computer Vision and Pattern Recognition (CVPR) (Colorado Springs: IEEE), 1297–1304.
Sibley, K. G., Girges, C., Hoque, E., and Foltynie, T. (2021). Video-based analyses of Parkinson's disease severity: a brief review. J. Parkinsons Dis., 11, S83–S93. doi: 10.3233/JPD-202402
Sigal, L., Balan, A. O., and Black, M. J. (2010). HumanEva: Synchronized video and motion capture dataset and baseline algorithm for evaluation of articulated human motion. Int. J. Comput. Vis. 87:4. doi: 10.1007/s11263-009-0273-6
Sijobert, B., Azevedo-Coste, C., Andreu, D., Verna, C., and Geny, C. (2016). Effects of sensitive electrical stimulation based cueing in Parkinson's disease: a preliminary study. Eur. J. Transl. Myol. 26:6018. doi: 10.4081/ejtm.2016.6018
Silva de Lima, A. L., Evers, L. J. W., Hahn, T., Bataille, L., Hamilton, J. L., Little, M. A., et al. (2017). Freezing of gait and fall detection in Parkinson's disease using wearable sensors: a systematic review. J. Neurol. 264, 1642–1654. doi: 10.1007/s00415-017-8424-0
Siragy, T., and Nantel, J. (2018). Quantifying dynamic balance in young, elderly and Parkinson's individuals: a systematic review. Front. Aging Neurosci. 10:387. doi: 10.3389/fnagi.2018.00387
Snijders, A. H., Van De Warrenburg, B. P., Giladi, N., and Bloem, B. R. (2007). Neurological gait disorders in elderly people: clinical approach and classification. Lancet Neurol. 6, 63–74. doi: 10.1016/S1474-4422(06)70678-0
Solomon, A. J., Jacobs, J. V., Lomond, K. V., and Henry, S. M. (2015). Detection of postural sway abnormalities by wireless inertial sensors in minimally disabled patients with Multiple Sclerosis: a case-control study. J. Neuroeng. Rehabil. 12, 1–9. doi: 10.1186/s12984-015-0066-9
Spain, R., St. George, R., Salarian, A., Mancini, M., Wagner, J., Horak, F., et al. (2012). Body-worn motion sensors detect balance and gait deficits in people with Multiple Sclerosis who have normal walking speed. Gait Posture 35, 573–578. doi: 10.1016/j.gaitpost.2011.11.026
Spain, R. I., Mancini, M., Horak, F. B., and Bourdette, D. (2014). Body-worn sensors capture variability, but not decline, of gait and balance measures in Multiple Sclerosis over 18 months. Gait Posture 39, 958–964. doi: 10.1016/j.gaitpost.2013.12.010
Stenum, J., Rossi, C., and Roemmich, R. T. (2021). Two-dimensional video-based analysis of human gait using pose estimation. PLoS Comput. Biol. 17:e1008935. doi: 10.1371/journal.pcbi.1008935
Straudi, S., Benedetti, M., Venturini, E., Manca, M., Foti, C., and Basaglia, N. (2013). Does robot-assisted gait training ameliorate gait abnormalities in multiple sclerosis? a pilot randomized-control trial. Neurorehabilitation 33, 555–563. doi: 10.3233/NRE-130990
Sun, R., Moon, Y., McGinnis, R. S., Seagers, K., Motl, R. W., Sheth, N., et al. (2018). Assessment of postural sway in individuals with multiple sclerosis using a novel wearable inertial sensor. Digit. Biomarkers 2, 1–10. doi: 10.1159/000485958
Suppa, A., Kita, A., Leodori, G., Zampogna, A., Nicolini, E., Lorenzi, P., et al. (2017). L-DOPA and freezing of gait in Parkinson's disease: objective assessment through a wearable wireless system. Front. Neurol. 8:406. doi: 10.3389/fneur.2017.00406
Szegedy, C., Liu, W., Jia, Y., Sermanet, P., Reed, S., Anguelov, D., et al. (2015). “Going deeper with convolutions,” in Proceedings of the IEEE Conference on Computer Vision and Pattern Recognition (Boston, MA: IEEE), 1–9.
Taborri, J., Palermo, E., Rossi, S., and Cappa, P. (2016). Gait partitioning methods: a systematic review. Sensors 16:66. doi: 10.3390/s16010066
Teufl, W., Lorenz, M., Miezal, M., Taetz, B., Fröhlich, M., and Bleser, G. (2019). Towards inertial sensor based mobile gait analysis: Event-detection and spatio-temporal parameters. Sensors 19:38. doi: 10.3390/s19010038
Tölgyessy, M., Dekan, M., Chovanec, L., and Hubinskỳ, P. (2021). Evaluation of the Azure Kinect and its comparison to Kinect v1 and Kinect v2. Sensors 21:413. doi: 10.3390/s21020413
Tome, D., Toso, M., Agapito, L., and Russell, C. (2018). “Rethinking pose in 3D: Multi-stage refinement and recovery for markerless motion capture,” in 2018 International Conference on 3D Vision (Verona: IEEE), 474–483.
Tompson, J., Goroshin, R., Jain, A., LeCun, Y., and Bregler, C. (2015). “Efficient object localization using convolutional networks,” in Proceedings of the IEEE Conference on Computer Vision and Pattern Recognition (Boston, MA: IEEE), 648–656.
Toshev, A., and Szegedy, C. (2014). “DeepPose: human pose estimation via deep neural networks,” in Proceedings of the IEEE Conference on Computer Vision and Pattern Recognition (Columbus, OH: IEEE), 1653–1660.
Ťupa, O., Procházka, A., Vyšata, O., Schätz, M., Mareš, J., Vališ, M., et al. (2015). Motion tracking and gait feature estimation for recognising Parkinson's disease using MS Kinect. Biomed. Eng. Online 14, 1–20. doi: 10.1186/s12938-015-0092-7
Tzallas, A. T., Tsipouras, M. G., Rigas, G., Tsalikakis, D. G., Karvounis, E. C., Chondrogiorgi, M., et al. (2014). PERFORM: a system for monitoring, assessment and management of patients with Parkinson's disease. Sensors 14, 21329–21357. doi: 10.3390/s141121329
Vaith, A., Taetz, B., and Bleser, G. (2020). “Uncertainty based active learning with deep neural networks for inertial gait analysis,” in 2020 IEEE 23rd International Conference on Information Fusion (FUSION) (Salt Lake City: IEEE), 1–8.
Valet, M., El Sankari, S., Van Pesch, V., Detrembleur, C., Lejeune, T., and Stoquart, G. (2021). Effects of prolonged-release fampridine on multiple sclerosis-related gait impairments. a crossover, double-blinded, placebo-controlled study. Clin. Biomech. 86:105382. doi: 10.1016/j.clinbiomech.2021.105382
Veeraragavan, S., Gopalai, A. A., Gouwanda, D., and Ahmad, S. A. (2020). Parkinson's disease diagnosis and severity assessment using ground reaction forces and neural networks. Front. Physiol. 11:1409. doi: 10.3389/fphys.2020.587057
Vienne-Jumeau, A., Quijoux, F., Vidal, P.-P., and Ricard, D. (2020). Wearable inertial sensors provide reliable biomarkers of disease severity in Multiple Sclerosis: a systematic review and meta-analysis. Ann. Phys. Rehabil. Med. 63, 138–147. doi: 10.1016/j.rehab.2019.07.004
Viqueira Villarejo, M., Maeso Garcia, J., Garcia Zapirain, B., and Mendez Zorrilla, A. (2014). Technological solution for determining gait parameters using pressure sensors: a case study of Multiple Sclerosis patients. Biomed. Mater. Eng. 24, 3511–3522. doi: 10.3233/BME-141177
Viswakumar, A., Rajagopalan, V., Ray, T., and Parimi, C. (2019). “Human gait analysis using OpenPose,” in 2019 Fifth International Conference on Image Information Processing (ICIIP) (Shimla: IEEE), 310–314.
Vohralik, S., Bowen, A., Burns, J., Hiller, C., and Nightingale, E. (2015). Reliability and validity of a smartphone app to measure joint range. Am. J. Phys. Med. Rehabil. 94, 325–330. doi: 10.1097/PHM.0000000000000221
Wang, Q., Kurillo, G., Ofli, F., and Bajcsy, R. (2015). “Evaluation of pose tracking accuracy in the first and second generations of Microsoft Kinect,” in 2015 International Conference on Healthcare Informatics (Dallas, TX: IEEE), 380–389.
Wang, Z., Ramamoorthy, V., Gal, U., and Guez, A. (2020). Possible life saver: a review on human fall detection technology. Robotics 9:55. doi: 10.3390/robotics9030055
Wei, S.-E., Ramakrishna, V., Kanade, T., and Sheikh, Y. (2016). “Convolutional pose machines,” in Proceedings of the IEEE Conference on Computer Vision and Pattern Recognition (Las Vegas, NV: IEEE), 4724–4732.
Wei, X., Zhang, P., and Chai, J. (2012). Accurate realtime full-body motion capture using a single depth camera. ACM Trans. Graph. 31, 1–12. doi: 10.1145/2366145.2366207
Weiss, A., Herman, T., Giladi, N., and Hausdorff, J. (2015). New evidence for gait abnormalities among Parkinson's disease patients who suffer from freezing of gait: insights using a body-fixed sensor worn for 3 days. J. Neural Transmiss. 122, 403–410. doi: 10.1007/s00702-014-1279-y
Winter, D. A. (1989). Biomechanics of normal and pathological gait. J. Motor Behav. 21, 337–355. doi: 10.1080/00222895.1989.10735488
Woodman, O. J. (2007). An introduction to inertial navigation. Technical report, University of Cambridge, Computer Laboratory.
Xiu, Y., Li, J., Wang, H., Fang, Y., and Lu, C. (2018). Pose flow: Efficient online pose tracking. arXiv e-prints, page arXiv:1802.00977.
Xu, H., Yu, Y., Zhou, Y., Li, Y., and Du, S. (2013). Measuring accurate body parameters of dressed humans with large-scale motion using a Kinect sensor. Sensors 13, 11362–11384. doi: 10.3390/s130911362
Xu, X., McGorry, R. W., Chou, L.-S., Lin, J.-H., and Chang, C.-C. (2015). Accuracy of the Microsoft Kinect for measuring gait parameters during treadmill walking. Gait Posture 42, 145–151. doi: 10.1016/j.gaitpost.2015.05.002
Xue, D., Sayana, A., Darke, E., Shen, K., Hsieh, J.-T., Luo, Z., et al. (2018). Vision-based gait analysis for senior care. arXiv e-prints, arXiv:1812.00169.
Yang, L., Yang, B., Dong, H., and El Saddik, A. (2016). 3-D markerless tracking of human gait by geometric trilateration of multiple Kinects. IEEE Syst. J. 12, 1393–1403. doi: 10.1109/JSYST.2016.2553518
Yang, S., and Li, Q. (2012). Inertial sensor-based methods in walking speed estimation: a systematic review. Sensors 12, 6102–6116. doi: 10.3390/s120506102
Yeung, L.-F., Yang, Z., Cheng, K. C.-C., Du, D., and Tong, R. K.-Y. (2021). Effects of camera viewing angles on tracking kinematic gait patterns using Azure Kinect, Kinect v2 and Orbbec Astra Pro v2. Gait Posture 87, 19–26. doi: 10.1016/j.gaitpost.2021.04.005
Zabatani, A., Surazhsky, V., Sperling, E., Moshe, S. B., Menashe, O., Silver, D. H., et al. (2019). Intel®RealSense sr300 coded light depth camera. IEEE Trans. Pattern Anal. Mach. Intell. 42, 2333–2345. doi: 10.1109/TPAMI.2019.2915841
Zago, M., Luzzago, M., Marangoni, T., De Cecco, M., Tarabini, M., and Galli, M. (2020). 3D Tracking of Human Motion Using Visual Skeletonization and Stereoscopic Vision. Front Bioeng Biotechnol. 8, 181. doi: 10.3389/fbioe.2020.00181
Zhang, Z. (2012). Microsoft Kinect sensor and its effect. IEEE Mult. Media 19, 4–10. doi: 10.1109/MMUL.2012.24
Keywords: motion tracking, human kinematics, locomotion, postural control, wearables, digital image processing, Parkinson's disease, multiple sclerosis
Citation: Salchow-Hömmen C, Skrobot M, Jochner MCE, Schauer T, Kühn AA and Wenger N (2022) Review—Emerging Portable Technologies for Gait Analysis in Neurological Disorders. Front. Hum. Neurosci. 16:768575. doi: 10.3389/fnhum.2022.768575
Received: 31 August 2021; Accepted: 07 January 2022;
Published: 03 February 2022.
Edited by:
Ioannis Ugo Isaias, Julius Maximilian University of Wrzburg, GermanyReviewed by:
Camila Pinto, Federal University of Health Sciences of Porto Alegre, BrazilMarco Ghislieri, Politecnico di Torino, Italy
Francesco Bolzoni, University of Milan, Italy
Copyright © 2022 Salchow-Hömmen, Skrobot, Jochner, Schauer, Kühn and Wenger. This is an open-access article distributed under the terms of the Creative Commons Attribution License (CC BY). The use, distribution or reproduction in other forums is permitted, provided the original author(s) and the copyright owner(s) are credited and that the original publication in this journal is cited, in accordance with accepted academic practice. No use, distribution or reproduction is permitted which does not comply with these terms.
*Correspondence: Christina Salchow-Hömmen, Y2hyaXN0aW5hLnNhbGNob3dAY2hhcml0ZS5kZQ==