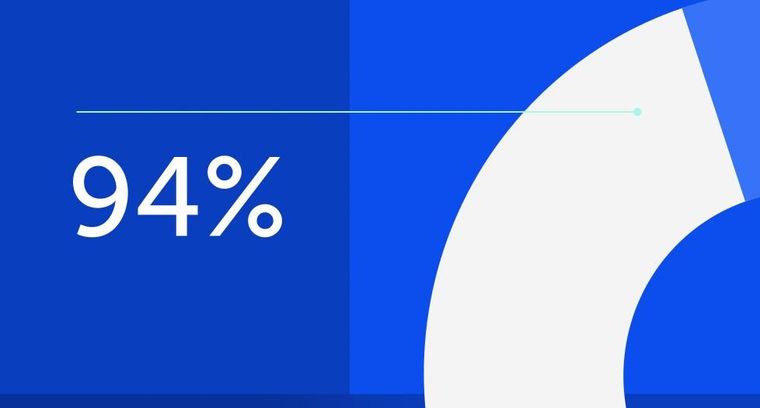
94% of researchers rate our articles as excellent or good
Learn more about the work of our research integrity team to safeguard the quality of each article we publish.
Find out more
MINI REVIEW article
Front. Hum. Neurosci., 11 November 2022
Sec. Motor Neuroscience
Volume 16 - 2022 | https://doi.org/10.3389/fnhum.2022.1037410
This article is part of the Research TopicThe Brain Meets the Body: Neural Basis of Cognitive Contribution in Movement for Healthy and Neurological PopulationsView all 10 articles
Motor imagery is increasingly being used in clinical settings, such as in neurorehabilitation and brain computer interface (BCI). In stroke, patients lose upper limb function and must re-learn bimanual coordination skills necessary for the activities of daily living. Physiotherapists integrate motor imagery with physical rehabilitation to accelerate recovery. In BCIs, users are often asked to imagine a movement, often with sparse instructions. The EEG pattern that coincides with this cognitive task is captured, then used to execute an external command, such as operating a neuroprosthetic device. As such, BCIs are dependent on the efficient and reliable interpretation of motor imagery. While motor imagery improves patient outcome and informs BCI research, the cognitive and neurophysiological mechanisms which underlie it are not clear. Certain types of motor imagery techniques are more effective than others. For instance, focusing on kinesthetic cues and adopting a first-person perspective are more effective than focusing on visual cues and adopting a third-person perspective. As motor imagery becomes more dominant in neurorehabilitation and BCIs, it is important to elucidate what makes these techniques effective. The purpose of this review is to examine the research to date that focuses on both motor imagery and bimanual coordination. An assessment of current research on these two themes may serve as a useful platform for scientists and clinicians seeking to use motor imagery to help improve bimanual coordination, either through augmenting physical therapy or developing more effective BCIs.
The rationale for this brief review was borne out of two observations: (1) brain computer interface (BCI) applications depend largely on motor imagery (Wolpaw and Wolpaw, 2012; McFarland and Wolpaw, 2018) (2) patient outcome can be improved when physical therapy is augmented with motor imagery (Mulder, 2007; Deutsch et al., 2012). Motor imagery is defined as the mental simulation of a movement in the absence of any overt movement (Decety, 1996; Jeannerod, 1999; Neuper et al., 2005). While the benefits of motor imagery have been recognized by athletes for decades, its advantages in clinical and rehabilitative settings, as well as its integration with BCI, is much more recent (Warner and McNeill, 1988; de Vries and Mulder, 2007). In BCI, individuals learn to control an external device, such as a neuroprosthetic device or more commonly, a computer cursor, by regulating their brain activity. Early demonstrations of this type of control were observed when individuals modulated their mu rhythm in the sensorimotor cortex (Wolpaw et al., 1991; McFarland et al., 2000; Neuper et al., 2009). More recent studies have demonstrated that BCI control is not limited to the mu rhythm, comprised of alpha and beta frequencies, but also occurs in several other ranges, including delta, theta, (Babiloni et al., 2017) and gamma rhythms (Babiloni et al., 2016). When a user learns to replicate this pattern, and conversely, the BCI accurately extracts and classifies the signature wave properties, then the individual acquires BCI control (McFarland and Wolpaw, 2018). This phenomenon requires multiple practice sessions to develop and is not present in all users (Vidaurre and Blankertz, 2010; Lotte and Jeunet, 2015; Thompson, 2019). It is important to acknowledge that movement execution and observation, which are closely related to motor imagery, have a complex underpinning. The neural dynamics that underlie movement execution and action observation overlap, and while they are highly relevant, they are beyond the scope of this brief review.
The majority of research in BCI has grown out of the machine learning perspective, i.e., developing computational algorithms that reliably interpret EEG signals (Aricò et al., 2018; McFarland and Wolpaw, 2018), while much less research has been dedicated to the nature of the user’s cognitive state. Further, stroke, and other neurodegenerative diseases such as multiple sclerosis, typically result in the loss of bimanual coordination. Many of the activities of daily living depend on successful bimanual coordination, such as buttoning a shirt or opening a tube of toothpaste. Therefore, a greater understanding of the use of motor imagery in bimanual coordination skills may help accelerate patient recovery and inform future research. The aim of this review is to bring together two themes, motor imagery and bimanual coordination, that are not often considered together, and to examine them in the context of their capacity to enhance neurorehabilitation and BCI development.
This review was conducted using two scientific databases, PubMed and Scopus, with the keywords, motor imagery AND bimanual coordination. We adopted ‘motor imagery’ in favor or ‘mental imagery’ because the former is specific to movement and motor skills (Collet and Guillot, 2010), whereas the latter is a broader term that includes general cognitive tasks and does not necessarily require any movement. For example, mental rotation of a three-dimensional object, memory retrieval, and mental arithmetic would not be categorized as motor imagery. Bimanual coordination includes a diverse range of skills which depend upon simultaneous use of the left and right hands (Ivry et al., 2004; Swinnen and Wenderoth, 2004). The PubMed database retrieved 11 results and Scopus retrieved 17. All of PubMed’s results were duplicates of Scopus. Studies that focused on gait abnormalities in diseased populations (Vercruysse et al., 2012) or included TMS (Levin et al., 2004; Wang et al., 2015, 2016) were excluded.
Bimanual coordination tasks range in complexity from those as simple as clapping, which an infant performs almost reflexively, to others that require a lifetime of practice, such as a concert pianist performing a difficult sonata. In the 16 research articles, the types of bimanual movements tested ranged from simple, laboratory tasks, such as bimanual sequence learning (Debarnot et al., 2012) and finger-thumb opposition task (Nair et al., 2003) to those high in ecological validity, such as tying shoelaces, buttoning a shirt (Szameitat et al., 2012) or mimicking piano playing (Riquelme-Ros et al., 2020). The simultaneous circle and line drawing task was used across a large range of ages and developmental stages (Piedimonte et al., 2014) in autistic spectrum conditions (Piedimonte et al., 2018) and in stroke patients (Morioka et al., 2019). The simultaneous circle-line drawing task is used as a method for investigating the bimanual coupling effect (Franz et al., 1991). That is, when a person attempts to draw a circle with one hand and a line with the other, the line starts to resemble a circle. The extent to which the linear trajectory takes the shape of a circle can be quantified using the ‘ovalization index’ (OI) (Garbarini et al., 2012; Piedimonte et al., 2014). This OI is thereby a quantitative measure of the degree of neural cross-talk. In other words, as the line being drawn with one hand, starts to resemble the circle being drawn by the other (or vice cersa), interference across the two hemispheres can be inferred (Franz et al., 1991; Garbarini et al., 2012; Piedimonte et al., 2014). This model was used in healthy (Piedimonte et al., 2014), autistic (Piedimonte et al., 2018), and stroke patients (Morioka et al., 2019). Morioka et al. (2019) noted that use-dependent plasticity is an important factor to consider in stroke patients. When movement execution becomes difficult or impossible due to a brain lesion, usually a contralateral one, the patient may stop even thinking about the movement. This type of motor neglect could contribute to a decline in motor representation. By asking patients to draw a line or circle with a paralyzed limb, some degree of motor planning was occurring. Therefore, even if movement execution was not observed, the motor representation was presumably being reactivated (Morioka et al., 2019). In their study, King et al. (2022) used a desktop pedal exerciser designed for the hands. They noted that bilateral arm training for the use of motor imagery in BCI is receiving increased attention because unlike unimanual motion, it enables (a) motor cortex disinhibition of the injured hemisphere, (b) enhanced paths from the contralesional region to recruitment, and (c) upregulation of descending motor neuron commands to propriospinal neurons (Whitall et al., 2011; Lee et al., 2016).
It is important to consider seminal research on bimanual coordination constraints. Haken et al. (1985) compared left and right hand movements to a coupled non-linear oscillator. In so doing, they developed what came to be known as the HKB model, which could make robust predictions concerning a range of bimanual coordination patterns (for full review, see Kelso, 2021). One of the implications of this model was that it brought to light the importance of movement topology (McGinnis and Newell, 1982; Schmidt and Lee, 2018). Briefly, when the left and right hands move in a symmetric or mirror-like pattern along the midline, they are easier to perform than when they are required to move in the same direction, i.e., in a parallel, asymmetric pattern (Swinnen, 2002). Further, the model accurately predicted that if individuals were asked to perform the less favorable, asymmetric movements at faster and faster rates, then bimanual coordination would be compromised; movements would start to transition to the intrinsically favorable mirror symmetric patterns (Swinnen and Wenderoth, 2004). Notably, bimanual coordination movements that were reliably predicted using discrete bimanual skills, such as finger tapping, could not be uniformly applied to those that required continuous bimanual skills, such as dial rotation (Sisti et al., 2011). For instance, in finger-tapping, some combinations can be learned at a faster rate others (Summers, 2002); integer ratios, where two taps of the hand occur for every one tap of the other (2:1) are easier to learn compared with non-integer ratios, three taps of one hand for every two taps of the other hand (3:2). However, when subjects are asked to perform a similar combination but with a continuous motor skill instead of a discrete one, this difference disappears and the relative velocity between the two hands becomes the predictive parameter (Sisti et al., 2011). When shifting from behavioral and musculoskeletal perspectives to one that is neurophysiological, the importance of movement topology is especially clear. That is, movement direction is uniquely encoded by neuronal populations (Georgopoulos et al., 1986). Therefore, there is both behavioral and neuronal evidence for the importance of considering movement topology in the context of developing BCI systems and neurorehabilitation strategies that enhance bimanual coordination.
Dahm and Rieger (2016a) point out that a variety of motor coordination constraints, such as Fitts’ Law, are also present in motor imagery (Fitts, 1954; Cerritelli et al., 2011; Dahm and Rieger, 2016a). They included three motor imagery conditions of a bimanual coordination task in order to determine the extent of cognitive constraints (Dahm and Rieger, 2016a). The study built on accumulating evidence for the role of cognitive and perceptual constraints in bimanual coordination (Mechsner et al., 2001). Briefly, participants learned target reaching tasks in which congruent and incongruent targets (X to O, vs. O to O) were included and required both symmetric and parallel movements to perform successfully. Both real and imagined movements were timed, and the three experimental conditions included were based on the phase of movement, i.e., initiation, termination, and the entire duration of the movement (Dahm and Rieger, 2016a). Participants engaged in kinesthetic imagery. Ease of imagery, vividness of imagery and concentration were all assessed using a Likert scale. It was noted that task-dependent processes occur in which several types of motor imagery may be differentially engaged. For instance, while drawing is a unimanual motor task, it relies heavily on visual cues, however, movements such as rowing or reaching may be more dependent on kinesthetic cues (Dahm and Rieger, 2016a). Further, components of the movement, such as initiation and termination, are important considerations. In another study, they examined whether bimanual coordination constraints are present in motor imagery (Dahm and Rieger, 2016b). They used three tasks to compare different versions of the mental chronometry paradigm, in which the time it takes to complete a motor imagery task is measured. The key finding was that bimanual coordination constraints were also present in motor imagery, i.e., imagined symmetric movements resulted in shorter inter-response intervals and greater accuracy than parallel movements (Dahm and Rieger, 2016b).
Szameitat et al. (2012) also included a kinesthetic first person perspective. Further, they instructed participants to “engage intensely” and with “high frequency” for the duration of a set time trial. Self-reported assessment of how vividly participants could imagine the movement was completed using a 7-point Likert scale. Szameitat et al. (2012) captured potential movement during imagery by having participants hold a force sensitive grip in each hand. Interestingly, the only condition that detected a significant increase from baseline was the most difficult one, i.e., imagination of a complex movement using the non-dominant hand.
The Russian neurophysiologist, Nikolai Bernstein (1967) first described what would come to be known as the degrees of freedom problem (1967). That is, redundant solutions exist for any given motor skill. When Bernstein’s degrees of freedom problem is considered in the context of motor imagery, redundant solutions multiply. In addition, there is the obvious issue that while behavior is directly observable, cognition is only indirectly observed. This highlights the need for specifying type of motor imagery, explicit a priori instructions as well as qualitative and quantitative post hoc assessments. The majority of papers reviewed included the favorable kinesthetic, first-person perspective, which emphasizes internal, proprioceptive sensations (see Table 1).
Table 1. A subset of articles from the literature review that focused on neurologically health adults.
The first demonstration of the neuroanatomical correlates of imagined bimanual coordination skills was reported by Szameitat et al. (2012). Their prediction, and substantiation, that the neural activity of a specific cortical region of interest would not be selectively increased, rather connectivity would change, was built on the previous research of Puttemans et al. (2005). They reported that when skills are learned to the point that they become automatic, they are said to be overlearned, and in this case the neuroanatomical correlates are subcortical, and not cortical. In the early stages when attentional resources and cognitive demands are high, cortical regions are engaged; however during later stages of learning, cortical activation dissipates and subcortical activations become dominant (Puttemans et al., 2005). Szameitat et al. (2012) found changes in functional found changes in functional connectivity between parietal and premotor areas within and between hemispheres. This was in contrast to the lack of effect of any change in neural activation of a specific cortical region. This distinction is noteworthy because EEG captures cortical changes, while activity changes in subcortical structures depend on how well the “source localization” problem is addressed.
Coordinating the left and right hands depends on the primary and premotor cortices (M1 and PMC) and supplementary motor area (SMA) in the cortex, the cerebellum, and subcortically, the cingulate motor area and basal ganglia (Swinnen and Wenderoth, 2004; Jantzen et al., 2008; Rueda-Delgado et al., 2014). These areas are also active in unimanual movements (Cabibel et al., 2020). As task demands increase, the network extends beyond these regions to encompass prefrontal, parietal, and temporal areas (Swinnen and Wenderoth, 2004; Rueda-Delgado et al., 2014). While contralateral motor control has long been established, recent studies have highlighted the important contributions of ipsilateral motor control as well (Bundy and Leuthardt, 2019). Several studies have shown that the sensorimotor areas of the ipsilateral hemisphere are also activated (Bundy and Leuthardt, 2019), though its role is not yet clear. Some sustain that the ipsilateral activation contributes to the planning and execution of contralateral limb movements as the kinematic and movement parameters of the contralateral limb can be decoded from signals recorded in the ipsilateral hemisphere in monkeys (Ames and Churchland, 2019) and in humans (Bundy et al., 2018). Furthermore, the two hemispheres seem to have different roles in terms of praxis, independently from handedness. In fact, lesions of the left hemisphere lead to apraxia, i.e., the impairments in the production of complex movement in the absence of muscle deficits, much more often than right hemisphere damages (Haaland, 2006). Notably, a study showed that the ipsilateral cortical representation of reaching arm movements strikingly differs between the left and right hemispheres (Merrick et al., 2022). Finally, Mancini and Mirabella (2021) found left dominance in reactive inhibitory control. Inhibitory control is a pillar of motor control (Mirabella, 2014), and very likely, implementing inhibitory control in BCI could greatly help in restoring naturalistic behaviors (Mirabella and Lebedev, 2017).
-Developing effective BCI systems and neurorehabilitation strategies may depend on careful consideration of the type of bimanual movements individuals are being asked to learn.
-Skills that have been learned so well that they become automatic rely more heavily on subcortical structures and less so on cortical structures. EEG captures only surface cortical changes, and not subcortical ones. Although activity from deeper brain structures may be inferred as the “source localization” problem is resolved, EEG does not have the spatial resolution of fMRI. Therefore, movement topology and learning factors must be considered. For instance, the inclusion of more complex bimanual patterns may reveal cortical activation patterns that are distinct from simpler bimanual coordination patterns that require little training to learn.
-Assessing the vividness of a person’s motor imagery skills using both qualitative and quantitative measures, such as R-VMIQ and mental chronometry, may account for unexplained variance, thereby leading to more effective classifiers in BCI and better MI intervention strategies in clinical settings.
HS conceived the topic, led the literature search, prepared the manuscript, and final table. AB, MB, and EG conducted the literature searches, retrieved full-text articles, and prepared earlier versions of the table. All authors contributed to the article and approved the submitted version.
This project was supported by an Institutional Development Award (IDeA) from the National Institute of General Medical Sciences of the National Institutes of Health under grant no. P20GM103449. Its contents are solely the responsibility of the authors and do not necessarily represent the official views of NIGMS or NIH. Research was also supported by our collaborators at the National Center for Adaptive Neurotechnologies, under grant no. P41 EB018783 (NIH, NIBIB).
The authors declare that the research was conducted in the absence of any commercial or financial relationships that could be construed as a potential conflict of interest.
All claims expressed in this article are solely those of the authors and do not necessarily represent those of their affiliated organizations, or those of the publisher, the editors and the reviewers. Any product that may be evaluated in this article, or claim that may be made by its manufacturer, is not guaranteed or endorsed by the publisher.
Ames, K. C., and Churchland, M. M. (2019). Motor cortex signals for each arm are mixed across hemispheres and neurons yet partitioned within the population response. Elife 8:e46159. doi: 10.7554/eLife.46159.027
Aricò, P., Borghini, G., Di Flumeri, G., Sciaraffa, N., and Babiloni, F. (2018). Passive BCI beyond the lab: Current trends and future directions. Physiol. Meas. 39:08TR02. doi: 10.1088/1361-6579/aad57e
Babiloni, C., Del Percio, C., Lopez, S., Di Gennaro, G., Quarato, P. P., Pavone, L., et al. (2017). Frontal functional connectivity of electrocorticographic delta and theta rhythms during action execution versus action observation in humans. Front. Behav. Neurosci. 11:20. doi: 10.3389/fnbeh.2017.00020
Babiloni, C., Del Percio, C., Vecchio, F., Sebastiano, F., Di Gennaro, G., Quarato, P. P., et al. (2016). Alpha, beta and gamma electrocorticographic rhythms in somatosensory, motor, premotor and prefrontal cortical areas differ in movement execution and observation in humans. Clin. Neurophysiol. 127, 641–654. doi: 10.1016/j.clinph.2015.04.068
Bundy, D. T., and Leuthardt, E. C. (2019). The cortical physiology of ipsilateral limb movements. Trends Neurosci. 42, 825–839.
Bundy, D. T., Szrama, N., Pahwa, M., and Leuthardt, E. C. (2018). Unilateral, 3D arm movement kinematics are encoded in ipsilateral human cortex. J. Neurosci. 38, 10042–10056. doi: 10.1523/JNEUROSCI.0015-18.2018
Cabibel, V., Hordacre, B., and Perrey, S. (2020). Implication of the ipsilateral motor network in unilateral voluntary muscle contraction: The cross-activation phenomenon. J. Neurophysiol. 123, 2090–2098. doi: 10.1152/jn.00064.2020
Cerritelli, C., Guillot, A., Lebon, F., MacIntyre, T., and Moran, A. (2011). Measuring motor imagery using psychometric, behavioral, and psychophysiological tools. Exerc. Sport Sci. Rev. 39, 85–92. doi: 10.1097/JES.0b013e31820ac5e0
Collet, C., and Guillot, A. (2010). “Autonomic nervous system activities during imagined movements,” in The neurophysiological foundations of mental and motor imagery, eds A. Guillot and C. Collet (New York, NY: Oxford University Press), 95–108. doi: 10.1093/acprof:oso/9780199546251.003.0007
Dahm, S. F., and Rieger, M. (2016a). Cognitive constraints on motor imagery. Psychol. Res. 80, 235–247. doi: 10.1007/s00426-015-0656-y
Dahm, S. F., and Rieger, M. (2016b). Is there symmetry in motor imagery? Exploring different versions of the mental chronometry paradigm. Atten. Percept. Psychophys. 78, 1794–1805. doi: 10.3758/s13414-016-1112-9
de Vries, S., and Mulder, T. (2007). Motor imagery and stroke rehabilitation: A critical discussion. J. Rehabil. Med. 39, 5–13. doi: 10.2340/16501977-0020
Debarnot, U., Castellani, E., and Guillot, A. (2012). Selective delayed gains following motor imagery of complex movements. Arch. Ital. Biol. 150, 238–250. doi: 10.4449/aib.v150i4.1394
Decety, J. (1996). The neurophysiological basis of motor imagery. Behav. Brain Res. 77, 45–52. doi: 10.1016/0166-4328(95)00225-1
Deutsch, J., Maidan, I., and Dickstein, R. (2012). Patient-centered integrated motor imagery delivered in the home with telerehabilitation to improve walking after stroke. Phys. Ther. 92, 1065–1077. doi: 10.2522/ptj.20110277
Fitts, P. M. (1954). The information capacity of the human motor system in controlling the amplitude of movement. J. Exp. Psychol. 47, 381–391. doi: 10.1037/h0055392
Franz, E. A., Zelaznik, H. N., and McCabe, G. (1991). Spatial topological constraints in a bimanual task. Acta Psychol. 77, 137–151. doi: 10.1016/0001-6918(91)90028-x
Garbarini, F., Rabuffetti, M., Piedimonte, A., Pia, L., Ferrarin, M., Frassinetti, F., et al. (2012). ‘Moving’ a paralysed hand: Bimanual coupling effect in patients with anosognosia for hemiplegia. Brain 135, 1486–1497. doi: 10.1093/brain/aws015
Georgopoulos, A. P., Schwartz, A. B., and Kettner, R. E. (1986). Neuronal population coding of movement direction. Science 233, 1416–1419. doi: 10.1126/science.3749885
Haaland, K. Y. (2006). Left hemisphere dominance for movement. Clin. Neuropsychol. 20, 609–622. doi: 10.1080/13854040590967577
Haken, H., Kelso, J. A., and Bunz, H. (1985). A theoretical model of phase transitions in human hand movements. Biol. Cybern. 51, 347–356. doi: 10.1007/BF00336922
Ivry, R., Diedrichsen, J., Spencer, R., Hazeltine, E., and Semjen, A. (2004). “A cognitive neuroscience perspective on bimanual coordination and interference,” in Neuro-behavioral determinants of interlimb coordination, eds S. P. Swinnen and J. Duysens (Boston, MA: Springer), 259–295.
Jantzen, K. J., Oullier, O., and Scott Kelso, J. A. (2008). Neuroimaging coordination dynamics in the sport sciences. Methods 45, 325–335. doi: 10.1016/j.ymeth.2008.06.001
Jeannerod, V. (1999). Mental imaging of motor activity in humans. Curr. Opin. Neurobiol. 9, 735–739.
Kelso, J. A. (2021). The Haken–Kelso–Bunz (HKB) model: From matter to movement to mind. Biol. Cybern. 115, 305–322. doi: 10.1007/s00422-021-00890-w
King, J. T., John, A. R., Wang, Y. K., Shih, C. K., Zhang, D., Huang, K. C., et al. (2022). Brain connectivity changes during bimanual and rotated motor imagery. IEEE J. Transl. Eng. Health Med. 10:2100408. doi: 10.1109/jtehm.2022.3167552
Lee, S., Kim, Y., and Lee, B. H. (2016). Effect of virtual reality-based bilateral upper extremity training on upper extremity function after stroke: A randomized controlled clinical trial. Occup. Ther. Int. 23, 357–368. doi: 10.1002/oti.1437
Levin, O., Steyvers, M., Wenderoth, N., Li, Y., and Swinnen, S. P. (2004). Dynamical changes in corticospinal excitability during imagery of unimanual and bimanual wrist movements in humans: A transcranial magnetic stimulation study. Neurosci. Lett. 359, 185–189. doi: 10.1016/j.neulet.2004.01.070
Lotte, F., and Jeunet, C. (2015). “Towards improved BCI based on human learning principles,” in Proceedings of the 3rd international winter conference on brain-computer interface, (Gangwon: IEEE), 1–4. doi: 10.1109/IWW-BCI.2015.7073024
Mancini, C., and Mirabella, G. (2021). Handedness does not impact inhibitory control, but movement execution and reactive inhibition are more under a left-hemisphere control. Symmetry 13:1602.
McFarland, D. J., Miner, L. A., Vaughan, T. M., and Wolpaw, J. R. (2000). Mu and beta rhythm topographies during motor imagery and actual movements. Brain Topogr. 12, 177–186. doi: 10.1023/a:1023437823106
McFarland, D. J., and Wolpaw, J. R. (2018). Brain–computer interface use is a skill that user and system acquire together. PLoS Biol. 16:e2006719. doi: 10.1371/journal.pbio.2006719
McGinnis, P. M., and Newell, K. M. (1982). Topological dynamics: A framework for describing movement and its constraints. Hum. Mov. Sci. 1, 289–305.
Mechsner, F., Kerzel, D., Knoblich, G., and Prinz, W. (2001). Perceptual basis of bimanual coordination. Nature 414, 69–73. doi: 10.1038/35102060
Merrick, C. M., Dixon, T. C., Breska, A., Lin, J., Chang, E. F., King-Stephens, D., et al. (2022). Left hemisphere dominance for bilateral kinematic encoding in the human brain. Elife 11:e69977. doi: 10.7554/eLife.69977
Mirabella, G. (2014). Should I stay or should I go? Conceptual underpinnings of goal-directed actions. Front. Syst. Neurosci. 8:206. doi: 10.3389/fnsys.2014.00206
Mirabella, G., and Lebedev, M. À (2017). Interfacing to the brain’s motor decisions. J. Neurophysiol. 117, 1305–1319. doi: 10.1152/jn.00051.2016
Morioka, S., Osumi, M., Nishi, Y., Ishigaki, T., Ishibashi, R., Sakauchi, T., et al. (2019). Motor-imagery ability and function of hemiplegic upper limb in stroke patients. Ann. Clin. Transl. Neurol. 6, 596–604.
Mulder, T. (2007). Motor imagery and action observation: Cognitive tools for rehabilitation. J. Neural Transm. 114, 1265–1278. doi: 10.1007/s00702-007-0763-z
Nair, D. G., Purcott, K. L., Fuchs, A., Steinberg, F., and Kelso, J. A. (2003). Cortical and cerebellar activity of the human brain during imagined and executed unimanual and bimanual action sequences: A functional MRI study. Brain Res. Cogn. Brain Res. 15, 250–260. doi: 10.1016/s0926-6410(02)00197-0
Neuper, C., Scherer, R., Reiner, M., and Pfurtscheller, G. (2005). Imagery of motor actions: Differential effects of kinesthetic and visual-motor mode of imagery in single-trial EEG. Brain Res. Cogn. Brain Res. 25, 668–677. doi: 10.1016/j.cogbrainres.2005.08.014
Neuper, C., Scherer, R., Wriessnegger, S., and Pfurtscheller, G. (2009). Motor imagery and action observation: Modulation of sensorimotor brain rhythms during mental control of a brain–computer interface. Clin. Neurophysiol. 120, 239–247. doi: 10.1016/j.clinph.2008.11.015
Piedimonte, A., Conson, M., Frolli, A., Bari, S., Della Gatta, F., Rabuffetti, M., et al. (2018). Dissociation between executed and imagined bimanual movements in autism spectrum conditions. Autism Res. 11, 376–384. doi: 10.1002/aur.1902
Piedimonte, A., Garbarini, F., Rabuffetti, M., Pia, L., and Berti, A. (2014). Executed and imagined bimanual movements: A study across different ages. Dev. Psychol. 50, 1073–1080. doi: 10.1037/a0034482
Puttemans, V., Wenderoth, N., and Swinnen, S. P. (2005). Changes in brain activation during the acquisition of a multifrequency bimanual coordination task: From the cognitive stage to advanced levels of automaticity. J. Neurosci. 25, 4270–4278. doi: 10.1523/jneurosci.3866-04.2005
Riquelme-Ros, J. V., Rodriguez-Bermudez, G., Rodriguez-Rodriguez, I., Rodriguez, J. V., and Molina-Garcia-Pardo, J. M. (2020). On the better performance of pianists with motor imagery-based brain-computer interface systems. Sensors 20:4452. doi: 10.3390/s20164452
Rueda-Delgado, L. M., Solesio-Jofre, E., Serrien, D. J., Mantini, D., Daffertshofer, A., and Swinnen, S. P. (2014). Understanding bimanual coordination across small time scales from an electrophysiological perspective. Neurosci. Biobehav. Rev. 47, 614–635. doi: 10.1016/j.neubiorev.2014.10.003
Sallard, E., Spierer, L., Ludwig, C., Deiber, M. P., and Barral, J. (2014). Age-related changes in the bimanual advantage and in brain oscillatory activity during tapping movements suggest a decline in processing sensory reafference. Exper. Brain Res. 232, 469–479. doi: 10.1007/s00221-013-3754-3
Schmidt, R. A., and Lee, T. D. (2018). Motor control and learning: A behavioral emphasis. Champaign, IL: Human Kinetics.
Sisti, H. M., Geurts, M., Clerckx, R., Gooijers, J., Coxon, J. P., Heitger, M. H., et al. (2011). Testing multiple coordination constraints with a novel bimanual visuomotor task. PLoS One 6:e23619. doi: 10.1371/journal.pone.0023619
Summers, J. (2002). Practice and training in bimanual coordination tasks: Strategies and constraints. Brain Cogn. 48, 166–178.
Swinnen, S. P. (2002). Intermanual coordination: From behavioural principles to neural-network interactions. Nat. Rev. Neurosci. 3, 348–359. doi: 10.1038/nrn807
Swinnen, S. P., and Wenderoth, N. (2004). Two hands, one brain: Cognitive neuroscience of bimanual skill. Trends Cogn. Sci. 8, 18–25.
Szameitat, A. J., McNamara, A., Shen, S., and Sterr, A. (2012). Neural activation and functional connectivity during motor imagery of bimanual everyday actions. PLoS One 7:e38506. doi: 10.1371/journal.pone.0038506
Thompson, M. C. (2019). Critiquing the concept of BCI illiteracy. Sci. Eng. Ethics 25, 1217–1233. doi: 10.1007/s11948-018-0061-1
Vercruysse, S., Spildooren, J., Heremans, E., Vandenbossche, J., Wenderoth, N., Swinnen, S. P., et al. (2012). Abnormalities and cue dependence of rhythmical upper-limb movements in Parkinson patients with freezing of gait. Neurorehabil. Neural Repair 26, 636–645. doi: 10.1177/1545968311431964
Vidaurre, C., and Blankertz, B. (2010). Towards a cure for BCI illiteracy. Brain Topogr. 23, 194–198. doi: 10.1007/s10548-009-0121-6
Wang, K., Wang, Z., Zhou, P., Qi, H., He, F., Liu, S., et al. (2015). “MEP analysis of hand motor imagery with bimanual coordination under transcranial magnetic stimulation,” in Proceedings of the IEEE 7th international conference on awareness science and technology (iCAST), Qinhuangdao. 111–114. doi: 10.1109/ICAwST.2015.7314030
Wang, K., Wang, Z., Zhou, P., Qi, H., He, F., Liu, S., et al. (2016). MEP analysis of hand motor imagery with bimanual coordination under transcranial magnetic stimulation. J. Adv. Comput. Intell. Intell. Inform. 20, 462–466.
Warner, L., and McNeill, M. E. (1988). Mental imagery and its potential for physical therapy. Phys. Ther. 68, 516–521. doi: 10.1093/ptj/68.4.516
Whitall, J., Waller, S. M., Sorkin, J. D., Forrester, L. W., Macko, R. F., Hanley, D. F., et al. (2011). Bilateral and unilateral arm training improve motor function through differing neuroplastic mechanisms: A single-blinded randomized controlled trial. Neurorehabil. Neural Repair 25, 118–129. doi: 10.1177/1545968310380685
Wolpaw, J. R., McFarland, D. J., Neat, G. W., and Forneris, C. A. (1991). An EEG-based brain-computer interface for cursor control. Electroencephalogr. Clin. Neurophysiol. 78, 252–259. doi: 10.1016/0013-4694(91)90040-b
Keywords: motor imagery (MI), bimanual coordination, brain computer interface (BCI), neurorehabilitation, motor control, EEG, neuroplasticity, learning
Citation: Sisti HM, Beebe A, Bishop M and Gabrielsson E (2022) A brief review of motor imagery and bimanual coordination. Front. Hum. Neurosci. 16:1037410. doi: 10.3389/fnhum.2022.1037410
Received: 05 September 2022; Accepted: 18 October 2022;
Published: 11 November 2022.
Edited by:
Giovanni Mirabella, University of Brescia, ItalyReviewed by:
Luca Falciati, University of Brescia, ItalyCopyright © 2022 Sisti, Beebe, Bishop and Gabrielsson. This is an open-access article distributed under the terms of the Creative Commons Attribution License (CC BY). The use, distribution or reproduction in other forums is permitted, provided the original author(s) and the copyright owner(s) are credited and that the original publication in this journal is cited, in accordance with accepted academic practice. No use, distribution or reproduction is permitted which does not comply with these terms.
*Correspondence: Helene M. Sisti, aHNpc3RpQG5vcndpY2guZWR1
Disclaimer: All claims expressed in this article are solely those of the authors and do not necessarily represent those of their affiliated organizations, or those of the publisher, the editors and the reviewers. Any product that may be evaluated in this article or claim that may be made by its manufacturer is not guaranteed or endorsed by the publisher.
Research integrity at Frontiers
Learn more about the work of our research integrity team to safeguard the quality of each article we publish.