- 1Department of Community Medicine and Rehabilitation, Physiotherapy, Umeå University, Umeå, Sweden
- 2Department of Statistics, Umeå School of Business, Economics and Statistics, Umeå University, Umeå, Sweden
- 3Department of Physical Therapy, Sackler Faculty of Medicine, Stanley Steyer School of Health Professions, Tel Aviv University, Tel Aviv, Israel
Background: Instrumented gait analysis post-stroke is becoming increasingly more common in research and clinics. Although overall standardized procedures are proposed, an almost infinite number of potential variables for kinematic analysis is generated and there remains a lack of consensus regarding which are the most important for sufficient evaluation. The current aim was to identify a discriminative core set of kinematic variables for gait post-stroke.
Methods: We applied a three-step process of statistical analysis on commonly used kinematic gait variables comprising the whole body, derived from 3D motion data on 31 persons post-stroke and 41 non-disabled controls. The process of identifying relevant core sets involved: (1) exclusion of variables for which there were no significant group differences; (2) systematic investigation of one, or combinations of either two, three, or four significant variables whereby each core set was evaluated using a leave-one-out cross-validation combined with logistic regression to estimate a misclassification rate (MR).
Results: The best MR for one single variable was shown for the Duration of single-support (MR 0.10) or Duration of 2nd double-support (MR 0.11) phase, corresponding to an 89–90% probability of correctly classifying a person as post-stroke/control. Adding Pelvis sagittal ROM to either of the variables Self-selected gait speed or Stride length, alternatively adding Ankle sagittal ROM to the Duration of single-stance phase, increased the probability of correctly classifying individuals to 93–94% (MR 0.06). Combining three variables decreased the MR further to 0.04, suggesting a probability of 96% for correct classification. These core sets contained: (1) a spatial (Stride/Step length) or a temporal variable (Self-selected gait speed/Stance time/Swing time or Duration of 2nd double-support), (2) Pelvis sagittal ROM or Ankle plantarflexion during push-off, and (3) Arm Posture Score or Cadence or a knee/shoulder joint angle variable. Adding a fourth variable did not further improve the MR.
Conclusion: A core set combining a few crucial kinematic variables may sufficiently evaluate post-stroke gait and should receive more attention in rehabilitation. Our results may contribute toward a consensus on gait evaluation post-stroke, which could substantially facilitate future diagnosis and monitoring of rehabilitation progress.
Introduction
Instrumented motion analysis is increasingly used to evaluate movement patterns during gait post-stroke (Baker et al., 2016). It serves to identify specific gait deviations based on objective information to guide clinical decision-making, individual treatment and rehabilitation. Different forms of instrumented measurement techniques, such as timing devices, pressure-sensitive walkways, sensor systems, and 3D motion capture systems, have become popular and are today often available in clinics. However, the resulting raw data is vast and may yield almost endless biomechanical descriptors. Therefore, the need for a consensus regarding which variables to focus on in evaluation and rehabilitation as the most relevant and informative descriptors of gait post-stroke has thus been pointed out repeatedly (Krasovsky and Levin, 2010; Wikström et al., 2014; Wonsetler and Bowden, 2017; Sharififar et al., 2019; Nedergård et al., 2021).
The focus of gait analysis is commonly on the lower limbs. Recent studies nevertheless emphasize the importance of incorporating also the trunk and upper limbs, particularly in post-stroke gait analyses, since this information adds to the understanding of balance control, energy expenditure and functional ability (Verheyden et al., 2006; Stephenson et al., 2010; Punt et al., 2015; Van Criekinge et al., 2017). In addition, post-stroke gait analyses that include more than the lower limbs can identify deviations in other body parts and their possible underlying causes or consequences (Kahn et al., 2019). That kind of information is important when assessing gait function, tailoring exercises that aim to improve gait post-stroke, and evaluating the effects of treatment. There is however a lack of consensus concerning which standard variables to incorporate in whole-body analyses for evaluation of gait post-stroke. This lack of agreement aggravates comparisons between research studies and may detriment the process of evaluating gait rehabilitation post-stroke (Sharififar et al., 2019; Nedergård et al., 2021). The aim of this study was hence to contribute toward such a consensus by identifying a core set of a few kinematic variables to discriminate post-stroke gait from the gait of non-disabled controls. For this purpose, we used a statistical process including leave-one-out cross-validation combined with logistic regression. Similar methods have previously been applied to other populations, e.g., in proposing a test battery for individuals with rupture of the anterior cruciate ligament (Schelin et al., 2017). In our analysis, we included the most common kinematic gait variables obtained from the literature that have been used to evaluate gait post-stroke, as well as information of the upper limb (Johansson et al., 2014) and the coordination of the upper and lower body (Nedergård et al., 2020).
Materials and Methods
Participants
This cross-sectional study involved 31 persons post-stroke and 41 non-disabled controls. Characteristics of the participants are presented in Table 1. The stroke group was recruited from two clinics in Umeå, Sweden. Inclusion criteria were: 35–85 years of age; > 3 months since stroke onset; unilateral hemiparesis following ischemic or hemorrhagic stroke; ability to walk indoors without aids; comprehension of written and verbal information. Exclusion criteria were impairments or diseases other than stroke which could influence gait. The post-stroke participants were assessed as having an average of moderate motor impairments (Duncan et al., 1992) with a total score of 77 ± 18 (100 maximum) on the Fugl-Meyer Assessment (Fugl-Meyer et al., 1975). The controls were recruited among colleagues, acquaintances and through a local organization for retired persons. Individuals with musculoskeletal or neurological movement impairments were excluded from the control group. Some of the participants were also included in a previous study addressing arm swing during post-stroke gait (Johansson et al., 2014). The study was approved by the Regional Ethical Review Board in Umeå, Sweden (Dnr. 2011-199-31). All participants signed a written informed consent form before participation in accordance with the Declaration of Helsinki.
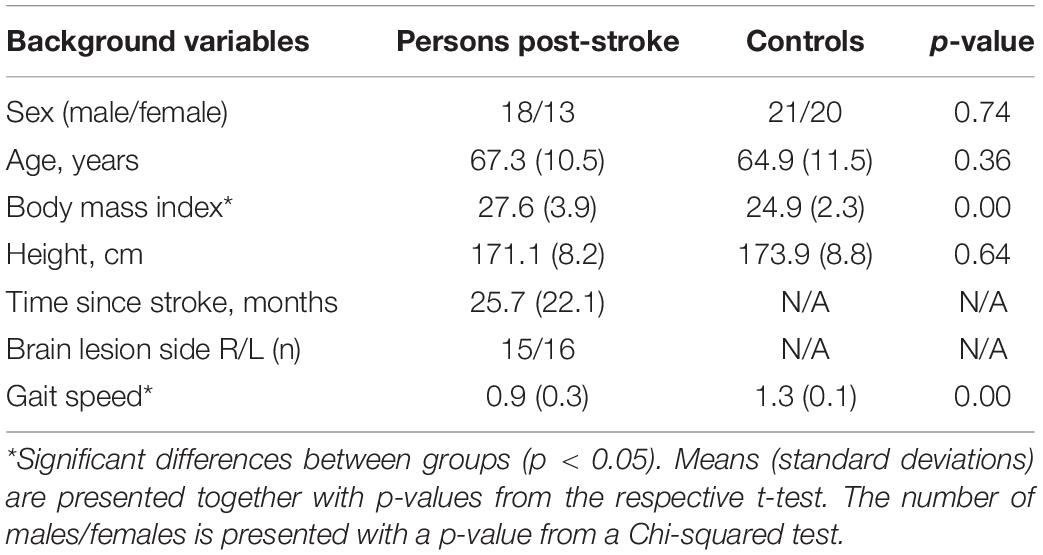
Table 1. Participant characteristics (including summary information for the three explanatory variables for the first step of the analysis).
Procedures
Gait analyses were performed in the U-Motion Laboratory at Umeå University, Sweden. Seventy-two reflective markers were attached to anatomical locations according to a full-body model described in detail previously (Frykberg et al., 2014). Markers were placed according to a strict protocol by three physiotherapists. Participants were instructed to walk barefoot at a self-selected speed on a 10 m walkway for a minimum of six trials. Kinematics were captured by an eight-camera three-dimensional motion capture system (240 Hz; Oqus®, Qualisys Gothenburg, Sweden). Data were recorded from the middle 3 m of the walkway and included 2–4 gait cycles for each trial. The data were analyzed using the Qualisys Track Manager software (QTM; Qualisys), filtered (15 Hz, fourth-order bidirectional low-pass Butterworth digital filter) and processed in Visual 3D (C-motion, Germantown, MD, United States).
Variables and Stepwise Data Analyses
Included kinematic variables are presented in Table 2. Range of motion (ROM) was defined as the difference between the highest and lowest values of the angular joint motion curve, and the maximum (MAX) joint angle was defined as the highest value of the angular joint motion. ROM Index (ROMI) represents the ratio value of ROM, comparing the affected and non-affected side (A/NA) in persons post-stroke, and the non-dominant and dominant side (ND/D) in controls. Upper and lower body inclination angles were defined as the highest value of the inclination angle between the ankle and COM, and the head and COM, respectively (Nedergård et al., 2020). If not specified otherwise, all variables were represented by extracted discrete values based on information from the entire GC. Data from the affected side were depicted for all unilateral outcomes with the one exception of hip abduction during the stance phase. The deviation scores: Gait Profile Score, (Baker et al., 2009), Gait Deviation Index (Schwartz and Rozumalski, 2008) and the Arm Posture Score (Riad et al., 2011; Frykberg et al., 2014), represented the root mean square deviation between the lower and upper limb joint angles of each participant of the post-stroke group and the average of the controls. For further descriptions of the variables, including descriptive statistics (see Supplementary Appendix A: Tables A–C).
The statistical approach consisting of three steps, briefly presented below, followed the main setup in Schelin et al. (2017). For all outcome variables presented in Supplementary Appendix A: Table A, we used a linear regression model to test whether there was a difference between persons post-stroke and non-disabled persons while controlling for age, sex and BMI (Step I). Descriptive statistics for the background variables age, sex and BMI are presented in Table 1. Variables with non-significant group differences were excluded from the subsequent analyses (Table 2).
In order to identify one or several potential core sets of variables, we systematically investigated all combinations consisting of 1–4 variables obtained from the complete set of all those variables that were significantly different between groups. Each core set was evaluated using leave-one-out cross-validation in combination with logistic regression to estimate the different models’ misclassification rate (MR) (Step II). For each core set, a logistic regression model based on the variables in the core set, with no interaction terms, was used to model the probability that the person (that was left out) belonged to the group of persons post-stroke. The default threshold of 0.5 was used for classification, implying that for an estimated probability above 0.5, the person was classified as post-stroke. The MR was estimated as the proportion of wrongly classified individuals. Confusion matrices that identified the rates of true positive, true negative, false positive, and false negative classifications were also considered. To add information about potential relationships between the variables, Spearman’s rank correlation was used to estimate the pair-wise correlations between the variables of interest. All analyses were performed in R (R Core Team, 2014).
Results
The first step in the analysis process identified which variables that were significantly different between persons post-stroke and non-disabled controls (see Table 2, and with further details reported in Supplementary Appendix A: Tables A–C).
In the second step, we calculated the MR for single variables, as well as for the combination of different variables. When using only one variable, the MR ranged from 0.10 to 0.42. The lowest, hence also the best, MR (0.10) was shown for the Duration of single-support phase in the affected side (Table 3). This corresponds to a 90% probability of correctly classifying a person as post-stroke or control based on this specific variable. Duration of 2nd double-support phase classified 89% (MR 0.11), and Stride length 87% (MR 0.13) of the persons correctly. A core set of two variables resulted in MRs ranging from 0.06 to 0.42. The lowest rate (MR 0.06) was shown for Step length/Stride length in combination with Pelvis sagittal ROM (pelvis anterior/posterior tilt). These two combinations of variables classified 94% of persons correctly. Combining three variables decreased the MR further to 0.04 (range: 0.04–0.42). Each core set of three variables correctly classified 96% of persons. A spatial (Stride length/Step length) or temporal variable (Self-selected gait speed/Stance time/Swing time/Duration of 2nd double-support) was combined with either Pelvis sagittal ROM or Ankle plantarflexion, terminal stance (during push-off). In addition, these variables were combined with either Arm Posture Score, Cadence, or a knee or shoulder joint angle variable, to generate the best possible MR based on our populations. Adding yet another variable did not decrease the MR further, nor did it change the types of variables included in the core sets (all core sets still included at least one variable describing joint angle motions, most often Pelvis sagittal ROM). The combination of exclusively spatial and temporal gait variables (i.e., not including joint angle data) that most correctly classified persons post-stroke was Stride width, Cadence, Duration of stance phase and Duration of 2nd double-support phase (Table 3). This combination correctly classified 93% of persons (MR 0.07).
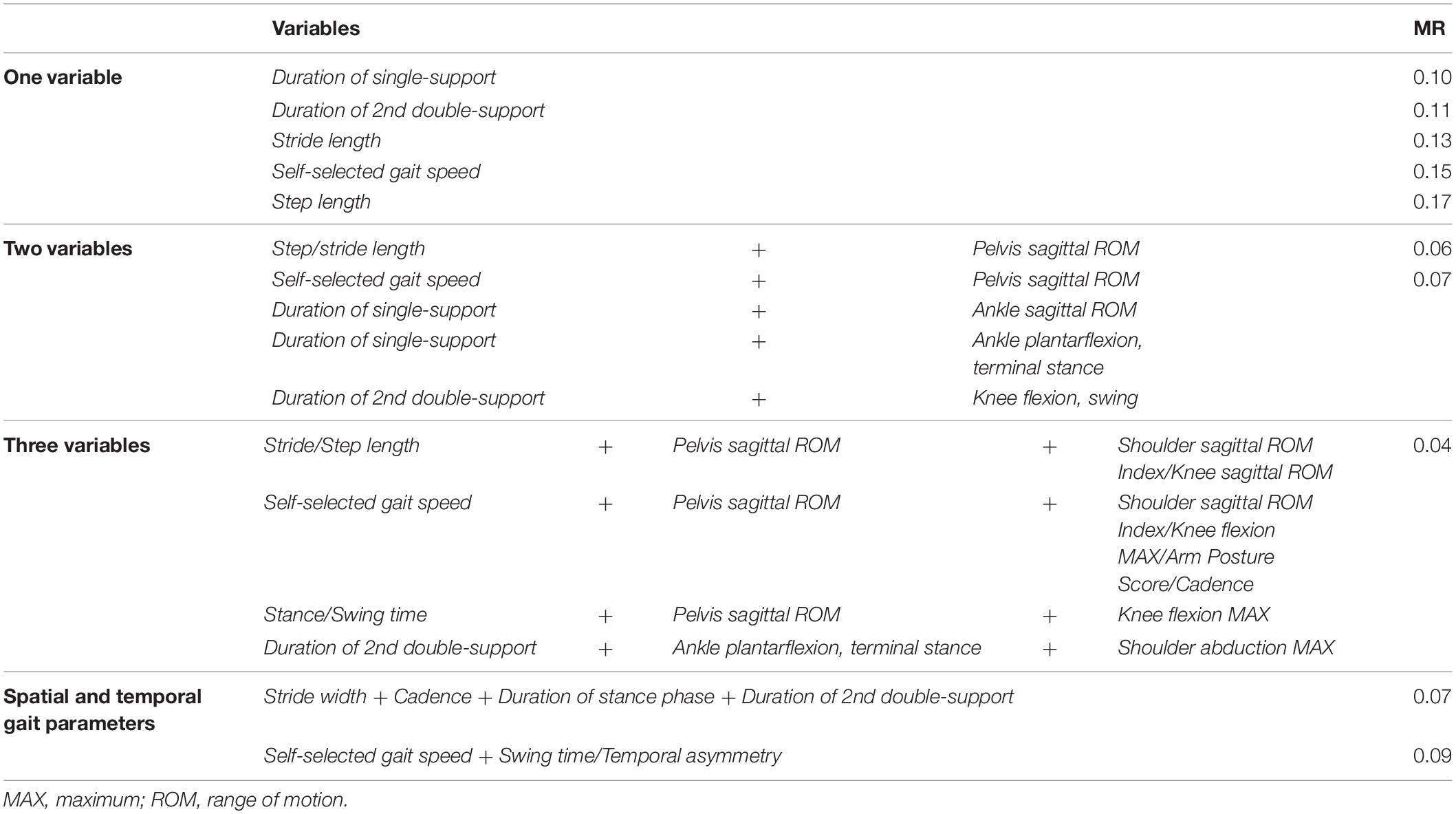
Table 3. The five lowest misclassification rates (MR) for one variable alone, or for core sets of 2–3 variables in combination.
Since the MR does not include information about whether the different models are better at classifying post-stroke persons or controls, this was addressed with confusion matrices to identify the rates for true positive (0.39), true negative (0.57), false positive (0.00), and false negative (0.04) classifications. These values are valid for all core sets of size three with MR equal to 0.04. The results showed that persons post-stroke and controls were classified correctly to a similar extent in the models with low MRs.
When investigating potential relationships between the variables, correlation analyses were performed based on pooled data from both groups but also calculated separately for each group (Supplementary Appendix B: Figures A–C). The correlation matrices revealed both similarities and differences in the correlations between variables when comparing persons post-stroke and controls. In both groups, the spatial and temporal variables were generally significantly correlated, although with some exceptions (see Supplementary Appendix B: Figures B,C). Correlations for joint angle data were fewer among non-disabled controls than in persons post-stroke.
Discussion
The stepwise analysis demonstrated that using only a single variable correctly discriminated persons post-stroke from controls with a 60–90% probability (MR 0.10-0-42) (Table 3). The most sensitive stand-alone variable was the Duration of single-support (MR 0.10) followed by the Duration of 2nd double-support (MR 0.11). Adding Pelvis sagittal ROM to the specific temporal or spatial variables (see Table 3) generated core sets that increased the probability of correctly classifying individuals to as high as 93–94%. Adding yet another kinematic variable to the core sets increased the probability even further to 96%.
Several of the gait variables represented in the core sets, such as the Duration of single- or 2nd double-stance (longer in persons post-stroke), Self-selected gait speed and Stride/Step length (slower and shorter, respectively, in persons post-stroke), are common, well-established variables used for evaluation of gait in persons post-stroke (Balaban and Tok, 2014; Sheffler and Chae, 2015; Baker et al., 2016). As confirmed by our correlation matrices (Supplementary Appendix B: Figures A–C) and in agreement with previous research (Olney and Richards, 1996; Nadeau et al., 2013; Sharififar et al., 2019), these variables were highly interrelated and also generated similar MRs. Note, however, that Cadence alone received the highest MR of the spatial and temporal gait variables and only classified persons correctly with a 68% probability (MR 0.32).
Our results indicate that for improved sensitivity, spatial and temporal variables should be analyzed in combination with variables such as the Pelvis sagittal ROM or knee/shoulder kinematics. When used independently, several variables had a higher probability of correctly differentiating persons post-stroke from controls than Pelvis sagittal ROM. But when Pelvis sagittal ROM was used in combination with other variables, it generated improved MRs and thus seems highly relevant for post-stroke gait analysis. Indeed, two-thirds of the here presented core sets combining 2–3 variables included this specific variable. The pelvis connects the trunk to the lower limbs, and muscular control at the pelvis has been suggested to be crucial for dynamic balance and weight transmission during walking (Karthikbabu et al., 2016). Data from our post-stroke population confirm earlier observed increased ROM regarding pelvis anterior/posterior tilt among persons post-stroke (Verheyden et al., 2014; Karthikbabu et al., 2017). This is presumed to be a consequence of impaired trunk control as well as due to adaptations following lower limb impairments (Van Criekinge et al., 2017). The range of sagittal motion of the pelvis is, however, relatively small especially compared to the range of sagittal motion in the lower limbs (Chen et al., 2003). This makes pelvic tilt more difficult to observe or measure in clinical settings without any technological devices. Furthermore, the constitution and attire of an individual may complicate assessing the pelvic tilt during gait. Nevertheless, the pelvic function should be considered when evaluating and treating gait post-stroke. Selective pelvic exercises, for instance, seems to have a beneficial effect on trunk function, standing balance, and mobility after stroke (Haruyama et al., 2016).
Altered knee movement patterns, such as greater or lesser knee flexion during the early stance phase followed by knee hyperextension in the late stance phase, have been reported post-stroke (Woolley, 2001; Baker et al., 2016). For the swing phase, previous studies have shown that the most common knee joint angle deviation is a generally decreased knee flexion and/or decreased knee extension prior to initial contact (Olney and Richards, 1996; Balaban and Tok, 2014; Baker et al., 2016). In 3D gait analysis, the knee sagittal kinematics are considered as reliable variables that also are suggested to predict gait function post-stroke (Guzik et al., 2020). In agreement with that, our results suggest that sagittal knee angle motions (represented by Knee flexion MAX and Knee sagittal ROM) are of particular importance when classifying gait post-stroke. These two variables were represented in several emerging core sets. For the Knee sagittal ROM, estimates of the minimal detectable change and the minimal clinically important difference have been established for persons post-stroke (Geiger et al., 2019; Guzik et al., 2020) and these estimated values are useful when interpreting changes of knee flexion/extension observed in persons who have undergone gait interventions post-stroke.
In contrast to the focus on the lower limbs, kinematics of the upper limbs during gait post-stroke has been far from as extensively investigated (Carmo et al., 2012). A few recent studies have, however, emphasized the importance of incorporating the trunk (i.e., the thorax) and arm movements in post-stroke gait analyses (Stephenson et al., 2010; Carmo et al., 2012). Stroke affects upper limb function in 50–70% of persons in the subacute phase post-stroke (Krakauer, 2005) and may have a clear influence on gait (Johansson et al., 2014). In the long term, around 40% experience disabilities of the upper limbs (Alt Murphy and Häger, 2015), whereas a lower percentage present with disabilities for functions of the lower limbs. It thus becomes clear that the inclusion of upper limb kinematic variables is of importance for ensuring comprehensive gait analyses. In the current study, upper limb motions (Shoulder abduction MAX, Arm Posture Score and Shoulder sagittal ROM Index) were represented in several suggested core sets of variables, providing further evidence that the upper limbs influence post-stroke gait movement pattern. An increased shoulder abduction angle among persons post-stroke (Shoulder abduction MAX) seems characteristic for post-stroke gait and has been assumed to be part of a compensatory strategy to help improve body weight distribution and balance (Carmo et al., 2012). In addition, an earlier reported variation in shoulder movement deviations in the sagittal plane (Kahn et al., 2019) was also observed among our persons post-stroke. While some persons display excessive movements (large ROM), others employ a more fixed joint posture (small ROM) during walking. Another interesting aspect was the generally greater number of associations between joint position variables among persons post-stroke when compared with controls (see the correlation matrices, Supplementary Appendix A: Tables B,C). Even though the absence of impairments would theoretically allow for greater variation, walking on an even surface without any obstacles and in a controlled fashion seems to generate a stable movement pattern. The fewer correlations between several variables among non-disabled controls when compared to persons post-stroke may hence be explained, at least partly, by lesser variability.
The potential for assessing the core set variables in a clinical context differs. The spatial and temporal variables are most accessible in clinics today since this data can be gathered relatively efficiently with the use of, e.g., pressure-sensitive walkways or timing devices. Self-selected gait speed is one of the most commonly used outcome measures. By combining Self-selected gait speed (MR 0.15) in the present study with another temporal variable, such as Duration of swing or Temporal asymmetry, the MR improved to 0.09. The combination of spatial and temporal variables that most correctly seems to classify persons post-stroke and controls included Stride width, Cadence, Duration of stance and Duration of 2nd double-support. Together these four variables classified persons correctly with a 93% probability.
Gathering joint position data, compared with spatial and temporal variables, generally requires more time, equipment and data processing. For example, calculation of the Arm Posture Score is currently based on comprehensive 3D upper limb joint position data, whereas estimating the Sagittal ROM Shoulder Index requires bilateral shoulder flexion/extension data. Low-cost webcam technology has, however, shown potential for real-time tracking of joint kinematics, particularly in the sagittal plane and if performed following specific recommendations related to data collection setup and camera and analysis software (Michelini et al., 2020). Such technology opens up new, more accessible possibilities for kinematic assessments during gait which may be more easily utilized in a clinical setting compared to laboratory-based data collection with 3D motion capture systems. Data collection of Pelvis sagittal ROM or Knee flexion MAX/Knee sagittal ROM, for instance, can also be made with 2D data-collecting systems. The use of portable inertial sensors to capture kinematic information over an extended space and time and in real-life conditions has also been described by Petraglia et al. (2019) and van Schaik and Dominici (2020).
Methodological Considerations
The purpose of Step II of the current analytical approach was to identify potential core sets of variables for discussion and future research toward a consensus on crucial variables for the kinematic evaluation of gait post-stroke. The focus of the current paper was not on checking model assumptions, interpreting parameter estimates, or comparing models in other ways than their classification ability. If identified core sets are to be used further in similar analyses, these assumptions should be checked. One specific assumption relates to the lack of multicollinearity, i.e., that the variables included in the model are not highly correlated. Note that gait speed and cadence were identified in the same core set and that these variables seem significantly correlated both in our analyses (see correlation matrices, Supplementary Appendix B: Figures A–C) and in previous research (Sharififar et al., 2019). Combining these two variables may therefore not be advisable if they represent the same underlying central nervous networks in the motor control process related to gait. While we have not a priori eliminated collinear variables based on conceptual conjectures, we are aware that this could result in redundancy in the discrimination process (one could potentially use various combinations that provide similar results).
Analyzing individuals walking at a self-selected speed as opposed to at their fastest possible gait speed or at a predetermined pace, will likely influence the results. Earlier research suggests, for instance, that gait speed affects the pelvic motions of non-disabled individuals (Lewis et al., 2017), while knee kinematics in the sagittal plane, on the other hand, seems not to be speed dependent when comparing persons post-stroke with non-disabled persons walking with matched gait speed (Chen et al., 2005). Due to close relationships between gait speed and other temporal and spatial variables, while persons post-stroke walked slower than non-disabled controls in our study, the core sets presented may be influenced either by the pathology per se or by gait speed, or both.
The generalizability of this study is limited to a population in a long-term phase post-stroke and with mainly relatively mild impairments. Previous studies have shown that e.g., pelvic tilt may vary depending on stroke onset (Van Criekinge et al., 2017) and severity level (Chen et al., 2003). Although our stroke sample does not fully represent the whole range of stroke severity, it is an important subpopulation as the individuals included may have treatable gait deficits that are not easily or clearly identified without instrumented motion analysis. Furthermore, the analysis has not considered the possible impact of the localization of injury which may affect functional outcomes and movement strategies post-stroke.
Only significant variables were included in the latter step of the analysis. Some variables could nonetheless still be important but were perhaps not significant due to lack of power. If altering the default threshold of 0.5 that was used for classification, the MR, as well as the rate of true positive/negative, might change. However, since persons post-stroke and controls both were correctly classified, and the MR was low for many models, we see only a limited gain of finding and using an optimal threshold.
Finally, almost an endless number of variables are available from instrumented gait analysis. We chose to initially include those that were considered relevant from a clinical perspective as well as the ones most commonly used, based on the literature in the field. The identified core sets contain spatiotemporal gait variables and joint motion variables in the sagittal plane, several of which are considered highly reliable in persons post-stroke and where previous analyses include estimates of minimal detectable change (Geiger et al., 2019).
Conclusion
Our results contribute toward a consensus on which kinematic variables should be included in the evaluation of gait post-stroke. This may substantially facilitate future diagnostics and treatment planning regarding specific gait deficits post-stroke, as well as the monitoring and evaluation of rehabilitation progress in the clinics. It would also be of great value for comparisons across studies and in meta-analysis contexts if the same variables are to be used in research.
Data Availability Statement
The raw data supporting the conclusions of this article will be made available by the authors, without undue reservation.
Ethics Statement
The studies involving human participants were reviewed and approved by the Regional Ethical Review Board in Umeå, Sweden (Dnr. 2011-199-31). The patients/participants provided their written informed consent to participate in this study.
Author Contributions
HN, DL, CH, and GJ formulated the research question. CH and LS obtained the funding. HN and GJ performed the data collection and data preparations. LS performed the quantitative data analysis. HN drafted the preliminary manuscript. All authors helped in further writing and critical reviewing of the manuscript, read, and approved the final manuscript.
Funding
This study was supported by the Swedish Brain Foundation (FO2016-0289), The Swedish Research Council (2016-02763), the Umeå University Foundation for Medical Research (FS 2.1.6-1870-16), the Umeå University Strategic research Area Health Care Science SFOV, the Region Vasterbotten, the Foundation for Stroke Research in Norrland, and King Gustaf V and Queen Victoria’s Foundation of Freemasons.
Conflict of Interest
The authors declare that the research was conducted in the absence of any commercial or financial relationships that could be construed as a potential conflict of interest.
Publisher’s Note
All claims expressed in this article are solely those of the authors and do not necessarily represent those of their affiliated organizations, or those of the publisher, the editors and the reviewers. Any product that may be evaluated in this article, or claim that may be made by its manufacturer, is not guaranteed or endorsed by the publisher.
Acknowledgments
Jonas Selling, Andrew Strong, and Gunilla E Frykberg are gratefully acknowledged for their assistive role and support of the work. Content of the manuscript in a preliminary version has been included in a doctoral thesis by Nedergård (2021), Umeå university: “Taking the next step: Whole-body biomechanical gait analysis, and user-perspectives on robotic-assisted gait training post-stroke.”
Supplementary Material
The Supplementary Material for this article can be found online at: https://www.frontiersin.org/articles/10.3389/fnhum.2021.820104/full#supplementary-material
References
Alt Murphy, M., and Häger, C. K. (2015). Kinematic analysis of the upper extremity after stroke – how far have we reached and what have we grasped? Phys. Ther. Rev. 20, 137–155. doi: 10.1179/1743288X15Y.0000000002
Baker, R., Esquenazi, A., Benedetti, M. G., and Desloovere, K. (2016). Gait analysis: clinical facts. Eur. J. Phys. Rehabil. Med. 52, 560–574.
Baker, R., McGinley, J. L., Schwartz, M. H., Beynon, S., Rozumalski, A., Graham, H. K., et al. (2009). The gait profile score and movement analysis profile. Gait Posture 30, 265–269. doi: 10.1016/j.gaitpost.2009.05.020
Balaban, B., and Tok, F. (2014). Gait disturbances in patients with stroke. PM R 6, 635–642. doi: 10.1016/j.pmrj.2013.12.017
Carmo, A. A., Kleiner, A. F., Costa, P. H., and Barros, R. M. L. (2012). Three-dimensional kinematic analysis of upper and lower limb motion during gait of post-stroke patients. Braz. J. Med. Biol. Res. 45, 537–545. doi: 10.1590/s0100-879x2012007500051
Chen, C.-L., Chen, H.-C., Tang, S. F.-T., Wu, C. Y., Cheng, P. T., and Hong, W. H. (2003). Gait performance with compensatory adaptations in stroke patients with different degrees of motor recovery. Am. J. Phys. Med. Rehabil. 82, 925–935. doi: 10.1097/01.Phm.0000098040.13355.B5
Chen, G., Patten, C., Kothari, D. H., and Zajac, F. E. (2005). Gait differences between individuals with post-stroke hemiparesis and non-disabled controls at matched speeds. Gait Posture 22, 51–56. doi: 10.1016/j.gaitpost.2004.06.009
Duncan, P. W., Goldstein, L. B., Matchar, D., Divine, G. W., and Feussner, J. (1992). Measurement of motor recovery after stroke. Outcome assessment and sample size requirements. Stroke 23, 1084–1089. doi: 10.1161/01.str.23.8.1084
Frykberg, G. E., Johansson, G. M., Schelin, L., and Häger, C. K. (2014). The Arm Posture Score for assessing arm swing during gait: an evaluation of adding rotational components and the effect of different gait speeds. Gait Posture 40, 64–69. doi: 10.1016/j.gaitpost.2014.02.006
Fugl-Meyer, A. R., Jääskö, L., Leyman, I., Olsson, S., and Steglind, S. (1975). The post-stroke hemiplegic patient. 1. a method for evaluation of physical performance. Scand. J. Rehabil. Med. 7, 13–31.
Geiger, M., Supiot, A., Pradon, D., Do, M. C., Zory, R., and Roche, N. (2019). Minimal detectable change of kinematic and spatiotemporal parameters in patients with chronic stroke across three sessions of gait analysis. Hum. Mov. Sci. 64, 101–107. doi: 10.1016/j.humov.2019.01.011
Guzik, A., Drużbicki, M., Wolan-Nieroda, A., Turolla, A., and Kiper, P. (2020). Estimating minimal clinically important differences for knee range of motion after stroke. J. Clin. Med. 9:3305.
Haruyama, K., Kawakami, M., and Otsuka, T. (2016). Effect of core stability training on trunk function, standing balance, and mobility in stroke patients: a randomized controlled trial. Neurorehabil. Neural Repair. 31, 240–249. doi: 10.1177/1545968316675431
Johansson, G. M., Frykberg, G. E., Grip, H., Broström, E. W., and Häger, C. K. (2014). Assessment of arm movements during gait in stroke - the arm posture score. Gait Posture 40, 549–555. doi: 10.1016/j.gaitpost.2014.06.014
Kahn, M. B., Clark, R. A., Williams, G., Bower, K. J., Banky, M., Olver, J., et al. (2019). The nature and extent of upper limb associated reactions during walking in people with acquired brain injury. J. Neuroeng. Rehabil. 16:160. doi: 10.1186/s12984-019-0637-2
Karthikbabu, S., Chakrapani, M., Ganesan, S., and Ellajosyla, R. (2017). Pelvic alignment in standing, and its relationship with trunk control and motor recovery of lower limb after stroke. Neurol. Clin. Neurosci. 5, 22–28. doi: 10.1111/ncn3.12092
Karthikbabu, S., Chakrapani, M., Ganesan, S., and Ellajosyula, R. (2016). Relationship between pelvic alignment and weight-bearing asymmetry in community-dwelling chronic stroke survivors. J. Neurosci. Rural Pract. 7(Suppl. 1), S37–S40. doi: 10.4103/0976-3147.196460
Krakauer, J. W. (2005). Arm function after stroke: from physiology to recovery. Semin. Neurol. 25, 384–395. doi: 10.1055/s-2005-923533
Krasovsky, T., and Levin, M. F. (2010). Review: toward a better understanding of coordination in healthy and poststroke gait. Neurorehabil. Neural Repair 24, 213–224. doi: 10.1177/1545968309348509
Lewis, C. L., Laudicina, N. M., Khuu, A., and Loverro, K. L. (2017). The human pelvis: variation in structure and function during gait. Anatom. Rec. 300, 633–642. doi: 10.1002/ar.23552
Michelini, A., Eshraghi, A., and Andrysek, J. (2020). Two-dimensional video gait analysis: a systematic review of reliability, validity, and best practice considerations. Prosthet. Orthot. Int. 44, 245–262. doi: 10.1177/0309364620921290
Nadeau, S., Betschart, M., and Bethoux, F. (2013). Gait analysis for poststroke rehabilitation: the relevance of biomechanical analysis and the impact of gait speed. Phys. Med. Rehabil. Clin. N. Am. 24, 265–276. doi: 10.1016/j.pmr.2012.11.007
Nedergård, H. (2021). ‘Taking the Next Step’: Whole-body Biomechanical Gait Analysis, and User-perspectives on Robotic Assisted Gait Training Post-stroke. Ph.D. dissertation, Umeå University, Faculty of Medicine, Umeå. Available online at: http://urn.kb.se/resolve?urn=urn:nbn:se:umu:diva-187069
Nedergård, H., Arumugam, A., Sandlund, M., Bråndal, A., and Häger, C. K. (2021). Effect of robotic-assisted gait training on objective biomechanical measures of gait in persons post-stroke: a systematic review and meta-analysis. J. Neuroeng. Rehabil. 18:64. doi: 10.1186/s12984-021-00857-9
Nedergård, H., Schelin, L., Frykberg, G. E., and Häger, C. K. (2020). Inclination angles of the ankle and head relative to the centre of mass identify gait deviations post-stroke. Gait Posture 82, 181–188. doi: 10.1016/j.gaitpost.2020.08.115
Olney, S. J., and Richards, C. (1996). Hemiparetic gait following stroke. Part I: characteristics. Gait Posture 4, 136–148. doi: 10.1016/0966-6362(96)01063-6
Petraglia, F., Scarcella, L., Pedrazzi, G., Brancato, L., Puers, R., and Costantino, C. (2019). Inertial sensors versus standard systems in gait analysis: a systematic review and meta-analysis. Eur. J. Phys. Rehabil. Med. 55, 265–280. doi: 10.23736/s1973-9087.18.05306-6
Punt, M., Bruijn, S. M., Wittink, H., and van Dieën, J. H. (2015). Effect of arm swing strategy on local dynamic stability of human gait. Gait Posture 41, 504–509. doi: 10.1016/j.gaitpost.2014.12.002
R Core Team (2014). R: A Language and Environment for Statistical Computing. Vienna: R Foundation for Statistical Computing.
Riad, J., Coleman, S., Lundh, D., and Broström, E. (2011). Arm posture score and arm movement during walking: a comprehensive assessment in spastic hemiplegic cerebral palsy. Gait Posture 33, 48–53. doi: 10.1016/j.gaitpost.2010.09.022
Schelin, L., Tengman, E., Ryden, P., and Häger, C. (2017). A statistically compiled test battery for feasible evaluation of knee function after rupture of the Anterior Cruciate Ligament - derived from long-term follow-up data. PLoS One 12:e0176247. doi: 10.1371/journal.pone.0176247
Schwartz, M. H., and Rozumalski, A. (2008). The Gait Deviation Index: a new comprehensive index of gait pathology. Gait Posture 28, 351–357. doi: 10.1016/j.gaitpost.2008.05.001
Sharififar, S., Vincent, H. K., Shuster, J., and Bishop, M. (2019). Quantifying poststroke gait deviations: a meta-analysis of observational and cross-sectional experimental trials. J. Stroke Med. 2, 23–31. doi: 10.1177/2516608519856246
Sheffler, L. R., and Chae, J. (2015). Hemiparetic gait. Phys. Med. Rehabil. Clin. N. Am. 26, 611–623. doi: 10.1016/j.pmr.2015.06.006
Stephenson, J. L., De Serres, S. J., and Lamontagne, A. (2010). The effect of arm movements on the lower limb during gait after a stroke. Gait Posture 31, 109–115. doi: 10.1016/j.gaitpost.2009.09.008
Van Criekinge, T., Saeys, W., Hallemans, A., Velghe, S., Viskens, P. J., Vereeck, L., et al. (2017). Trunk biomechanics during hemiplegic gait after stroke: a systematic review. Gait Posture 54, 133–143. doi: 10.1016/j.gaitpost.2017.03.004
van Schaik, J. E., and Dominici, N. (2020). Motion tracking in developmental research: methods, considerations, and applications. Prog. Brain Res. 254, 89–111. doi: 10.1016/bs.pbr.2020.06.007
Verheyden, G., Ruesen, C., Gorissen, M., Brumby, V., Moran, R., Burnett, M., et al. (2014). Postural alignment is altered in people with chronic stroke and related to motor and functional performance. J. Neurol. Phys. Ther. 38, 239–245. doi: 10.1097/npt.0000000000000054
Verheyden, G., Vereeck, L., Truijen, S., Troch, M., Herregodts, I., Lafosse, C., et al. (2006). Trunk performance after stroke and the relationship with balance, gait and functional ability. Clin. Rehabil. 20, 451–458. doi: 10.1191/0269215505cr955oa
Wikström, J., Georgoulas, G., Moutsopoulos, T., and Seferiadis, A. (2014). Intelligent data analysis of instrumented gait data in stroke patients-a systematic review. Comput. Biol. Med. 51, 61–72. doi: 10.1016/j.compbiomed.2014.04.004
Wonsetler, E. C., and Bowden, M. G. (2017). A systematic review of mechanisms of gait speed change post-stroke. Part 2: exercise capacity, muscle activation, kinetics, and kinematics. Top Stroke Rehabil. 24, 394–403. doi: 10.1080/10749357.2017.1282413
Keywords: gait analysis, walking, stroke, biomechanical evaluation, instrumented gait analysis
Citation: Nedergård H, Schelin L, Liebermann DG, Johansson GM and Häger CK (2022) Core Sets of Kinematic Variables to Consider for Evaluation of Gait Post-stroke. Front. Hum. Neurosci. 15:820104. doi: 10.3389/fnhum.2021.820104
Received: 22 November 2021; Accepted: 28 December 2021;
Published: 24 February 2022.
Edited by:
Marco Iosa, Sapienza University of Rome, ItalyReviewed by:
Stefano Filippo Castiglia, Sapienza University of Rome, ItalyNoureddin Nakhostin Ansari, Tehran University of Medical Sciences, Iran
Copyright © 2022 Nedergård, Schelin, Liebermann, Johansson and Häger. This is an open-access article distributed under the terms of the Creative Commons Attribution License (CC BY). The use, distribution or reproduction in other forums is permitted, provided the original author(s) and the copyright owner(s) are credited and that the original publication in this journal is cited, in accordance with accepted academic practice. No use, distribution or reproduction is permitted which does not comply with these terms.
*Correspondence: Heidi Nedergård, aGVpZGkubmVkZXJnYXJkQHVtdS5zZQ==