- 1Centre de Recherche Cerveau et Cognition (CerCo), Centre National de la Recherche Scientifique (CNRS), Paul Sabatier University, Toulouse, France
- 2Institute of Noetic Sciences (IONS), Petaluma, CA, United States
- 3Swartz Center for Computational Neuroscience (SCCN), Institute of Neural Computation (INC), University of California, San Diego, San Diego, CA, United States
Electroencephalography (EEG) alpha asymmetry is thought to reflect crucial brain processes underlying executive control, motivation, and affect. It has been widely used in psychopathology and, more recently, in novel neuromodulation studies. However, inconsistencies remain in the field due to the lack of consensus in methodological approaches employed and the recurrent use of small samples. Wearable technologies ease the collection of large and diversified EEG datasets that better reflect the general population, allow longitudinal monitoring of individuals, and facilitate real-world experience sampling. We tested the feasibility of using a low-cost wearable headset to collect a relatively large EEG database (N = 230, 22–80 years old, 64.3% female), and an open-source automatic method to preprocess it. We then examined associations between well-being levels and the alpha center of gravity (CoG) as well as trait EEG asymmetries, in the frontal and temporoparietal (TP) areas. Robust linear regression models did not reveal an association between well-being and alpha (8–13 Hz) asymmetry in the frontal regions, nor with the CoG. However, well-being was associated with alpha asymmetry in the TP areas (i.e., corresponding to relatively less left than right TP cortical activity as well-being levels increased). This effect was driven by oscillatory activity in lower alpha frequencies (8–10.5 Hz), reinforcing the importance of dissociating sub-components of the alpha band when investigating alpha asymmetries. Age was correlated with both well-being and alpha asymmetry scores, but gender was not. Finally, EEG asymmetries in the other frequency bands were not associated with well-being, supporting the specific role of alpha asymmetries with the brain mechanisms underlying well-being levels. Interpretations, limitations, and recommendations for future studies are discussed. This paper presents novel methodological, experimental, and theoretical findings that help advance human neurophysiological monitoring techniques using wearable neurotechnologies and increase the feasibility of their implementation into real-world applications.
Introduction
Well-Being
The question of what determines well-being has intrigued humans throughout recorded history and to this day remains a topic of significant interest and debate (Dodge et al., 2012; Alexandrova, 2015). The hedonic view of well-being focuses on the emotional dimension (i.e., positive and negative affect) to address this question. The eudaimonic perspective focuses on the sense of striving toward realizing one’s potential or goals, a life purpose, and seeking personal growth (Ryan et al., 2008). Well-being is now understood as a multidimensional and dynamic construct encompassing both views and other new essential components such as mental and physical health, autonomy, social relationships, spirituality, self-acceptance (Keyes and Waterman, 2003; Ryff and Singer, 2013). Well-being can be mediated by numerous factors such as demographics (Keyes and Waterman, 2003; Carstensen et al., 2011), genetic predisposition (Keyes et al., 2010), personality traits (Lucas and Diener, 2008), income (Luhmann et al., 2011), exercise (Hassmén et al., 2000; Svensson et al., 2021), mindfulness meditation practice (Carmody and Baer, 2008), or connectedness with nature (Howell et al., 2011; Russell et al., 2013). These factors also shape the structure and function of our brains throughout the lifespan, with important implications for well-being levels. While progress has been made recently regarding our understanding of the relationships between well-being and the brain, much is still unknown (Dolcos et al., 2018). By identifying the neural correlates of well-being, we may better understand the mechanisms that underly higher levels of well-being, and in turn, develop promising interventions aiming at helping people live happier and more successful lives (Dolcos et al., 2018).
Frontal Electroencephalographic Asymmetry
Definition and Calculation
For decades, frontal electroencephalographic (EEG) asymmetry has been a useful tool to study emotion-related states and traits, motivation, temperament, cognitive control, and psychopathologies (Coan and Allen, 2003, 2004; Allen et al., 2004; Harmon-Jones et al., 2010; Scherer and Ekman, 2014; Allen and Reznik, 2015; Smith et al., 2017). Frontal EEG asymmetry refers to a relative difference in alpha power spectral activity (8–13 Hz) between the left and right frontal regions of the brain. Because alpha oscillations are known to functionally inhibit regional cortical activity (Laufs et al., 2003, 2006; Oakes, 2004; Mathewson et al., 2011; Scheeringa et al., 2012; Grimshaw and Carmel, 2014), authors have associated an increased alpha activity with a decrease in brain activity or a decrease in allocation of cortical resources in the same region (Davidson, 1988; Davidson et al., 1990; Gevins et al., 1997; Cook et al., 1998; Allen et al., 2004). Thus, positive asymmetry scores (i.e., greater alpha power in the right frontal area relative to the left) are thought to reflect relatively lower right than left frontal cortical activity, and vice versa for a negative asymmetry score.
The Main Models
Decades of work using the alpha asymmetry metric have led to emotional valence and motivation models (Allen et al., 2004; Harmon-Jones et al., 2010). These models highlight that approach motivation emotional processes are associated with relatively greater cortical activity in the left frontal area compared to the right, which in turn, is inversely correlated with alpha power (i.e., greater right than left alpha power in these areas). Inversely, emotional processes related to avoidance motivation and a negative valence are associated with relatively greater right than left frontal cortical activity (corresponding to greater left than right frontal alpha power). Extreme approach-oriented traits and behaviors include for example positive urgency (i.e., the tendency toward rash action in response to extreme positive emotional states (Tomarken and Davidson, 1994), sensation-seeking (Santesso et al., 2008), and high reward sensitivity (Pizzagalli et al., 2005), whereas avoidance-related traits and behaviors include depression and anxiety (Thibodeau et al., 2006), shy temperament (Fox et al., 1995), negative dispositional affect (Tomarken and Davidson, 1994), and poor regulation of negative emotions (Jackson et al., 2003). These models align with the clinical literature showing that lesions in the left frontal area are associated with depression symptoms (Robinson and Price, 1982; Harmon-Jones et al., 2010).
The Underlying Brain Networks and Systems
Going one step further beyond these descriptive models, investigators using EEG source-localization techniques (Laufs et al., 2003; Pizzagalli et al., 2005; Mantini et al., 2007; Koslov et al., 2011; Gable et al., 2015; Smith et al., 2018) found that frontal asymmetries originate from the dorsal frontoparietal network (dFPN), the inferior frontal gyrus, and the right dorsolateral prefrontal cortex (dlPFC; which is part of the dFPN). These results led them to suspect that frontal asymmetries reflect the integrity of the supervisory system, which is theorized to generate effortful constraint and self-control (Sutton and Davidson, 1997; Cacioppo et al., 2007; Gable et al., 2015). Gable et al. (2015) suspected that the alpha asymmetry is driven by the activity of this supervisory control system, supposedly located in the right frontal area (Gable et al., 2015). Frontal asymmetries may also reflect other associated executive control mechanisms, which play an essential role in allocating attention toward a goal and inhibiting interference from distractors (Corbetta et al., 2008; Vossel et al., 2013; Grimshaw and Carmel, 2014; Gable et al., 2015). In this view, termed the asymmetric inhibition model, mechanisms in the left frontal cortex would inhibit negative distractors, whereas mechanisms in the right frontal cortex would inhibit positive distractors. Consequently, asymmetric aberrations in these systems result in bottom-up and top-down dysfunction, such as the difficulty in disengaging attention from negative/avoidance-motivation information AS in depression and anxiety (Eysenck et al., 2007; Shackman et al., 2009; Cisler and Koster, 2010; De Raedt and Koster, 2010; Engels et al., 2010; Gotlib and Joormann, 2010; Kim et al., 2012; Gable et al., 2015), whereas difficulty in inhibiting positive/approach-motivation distractors results in addiction and positive urgency behaviors (Bechara, 2005; Garavan and Hester, 2007; Goldstein and Volkow, 2011). Thus, multiple lines of research demonstrate that the mechanisms underlying alpha asymmetry measurements are highly implicated in processes that contribute to well-being (positive/negative affect, capacity to fulfill one’s potential and life goals, etc.).
Limitations in Electroencephalographic Asymmetry Research
While the literature on EEG asymmetry is sizable and robust, it is important to note that there have also been failed replications and contradictory results (Gotlib, 1998; Reid et al., 1998; Hagemann et al., 1999; Müller et al., 1999; Coan et al., 2001; Gale et al., 2001; Papousek and Schulter, 2002; Dennis and Solomon, 2010; Stewart et al., 2010; Kop et al., 2011; Koslov et al., 2011; Quinn et al., 2014; Meyer et al., 2015; Arns et al., 2016; Palmiero and Piccardi, 2017; van der Vinne et al., 2017). These inconsistencies can be explained by the heterogeneity in the experimental designs, EEG preprocessing techniques, and statistical approaches employed across investigators over the years (Allen et al., 2004; Smith et al., 2017). A summary of the main limitations of EEG asymmetry research and proposed solutions that were implemented in this study are now described.
Trait Versus State
One limitation is that EEG asymmetry can reflect trait or state aspects and thus, designing experiments to highlight one over the other depending on the research question is essential. When measured during rest, EEG asymmetry is considered a trait variable related to various psychological constructs and predictive of future emotional behavior or psychopathology (Wheeler et al., 1993; Davidson, 1994; Sutton and Davidson, 1997; Stewart et al., 2010; Nusslock et al., 2011; Papousek et al., 2012). When measured as an event-related response, it is considered a state variable reflecting the person’s current emotional state (Coan et al., 2001; Harmon-Jones and Sigelman, 2001; Harmon-Jones, 2004). Some authors estimate that 60% of the variance in asymmetry measure within a resting session is due to trait influence, and the 40% to state influences (Hagemann et al., 2002). Hence, the first approach aims to reduce the state influence during rest, whereas the second one aims to increase it using emotion-elicitation perturbations (Coan et al., 2006). In this study, we focus on the trait variable and hypothesize that trait frontal alpha asymmetry will be associated with multidimensional well-being (since well-being is driven by both emotional valence and motivational components).
Sample Characteristics
The second limitation to EEG asymmetry research is that sample-specific characteristics (e.g., age, gender) have been shown to significantly influence EEG findings because of functional and anatomical differences (Klimesch, 1999; Sowell et al., 2007; Hagemann et al., 2008; Finley et al., 2020). Many EEG asymmetry studies include participants of one gender to reduce this bias (Tomarken et al., 1990; Wheeler et al., 1993; Jacobs and Snyder, 1996; Reid et al., 1998; Gale et al., 2001; Dennis and Solomon, 2010; Mikolajczak et al., 2010; Koslov et al., 2011). However, this prevents investigators from examining gender as a potential mediator or moderator of asymmetry findings (MacKinnon et al., 2013). There is a lack of consensus regarding the role gender plays in EEG asymmetry in the limited studies that have addressed this question (Veldhuizen et al., 1993; Carrier et al., 2001; Miller et al., 2002; Otero et al., 2003; Morgan et al., 2005; Gasbarri et al., 2006, 2007; Stewart et al., 2010; Kovacevic et al., 2015; Müller et al., 2015; Hashemi et al., 2016). Similarly, the role age plays in EEG asymmetry is also not very well known. One solution to the lack of understanding of if and how demographic variables influence EEG asymmetry and well-being is to collect large and diversified datasets that better reflect the general population. A few studies with large samples found that age and gender mediate frontal asymmetry but that ethnicity or socioeconomic status did not (Stewart et al., 2010; Gable et al., 2015; Arns et al., 2016). However, these studies are rare and hard to replicate because of the time and cost involved in recording EEG data on a large number of subjects with conventional systems (equipment cost, EEG preparations time, participants compensation for their time, equipment cleaning, etc.).
Wearable EEG technologies make the collection of large datasets of diversified and under-represented populations more feasible and offer promising new applications for both clinicians and researchers in the long term (Cannard et al., 2020). These applications include brain monitoring in naturalistic settings and in real-time (Hu et al., 2015; Jebelli et al., 2017), brain-computer interfaces (BCI; Park et al., 2020), neurofeedback interventions (Angelakis et al., 2007; Quaedflieg et al., 2016; Brandmeyer and Delorme, 2020a), neuromarketing (Cartocci et al., 2018; Ramsøy et al., 2018), or neuroaesthetics research (i.e., the science studying the biological underpinnings of aesthetic experience; Cheung et al., 2019; Cartocci et al., 2021). While these EEG systems can have inferior hardware capacities than conventional ones, recent technological and algorithmic advancements make the detection and measurement of mental states increasingly reliable (Wu et al., 2017), with as few as a single EEG channel (Umar Saeed et al., 2018; Arpaia et al., 2020; Mahmoodi et al., 2021). Additionally, these systems can easily combine other physiological measures such as electrocardiography (ECG) or galvanic skin response (GSR) to improve the efficacy of mental states detection (e.g., Ahn et al., 2019). Wearable EEG systems have been used extensively over the past few years to measure frontal asymmetry (Peng et al., 2011; Hu et al., 2015; Hashemi et al., 2016; Jebelli et al., 2017, 2018; Wu et al., 2017; Zhao et al., 2017; Hwang et al., 2018; Umar Saeed et al., 2018; Cao et al., 2019; Arpaia et al., 2020; Park et al., 2020; Saeed et al., 2020) and were used in this present study to enable the collection of a large dataset. Hence, in this study, we aim to evaluate the potential relationship between well-being, alpha asymmetry, and individual characteristics (namely age and gender) in a large sample, collected using a low-cost wearable EEG headset.
Alpha Frequencies and Bounds
The third main limitation in EEG asymmetry research is the handling of alpha-band frequencies and bounds. The alpha band is dominantly considered as a single phenomenon in EEG asymmetry studies. However, previous evidence suggested that it should not. For instance, measuring alpha power spectral density (PSD) on the traditionally a priori-defined bandwidth 8-13 Hz does not account well for interindividual differences because parts of the alpha power distribution fall outside this range for some individuals (Klimesch et al., 1990; Klimesch, 1997). Furthermore, differential changes in opposing directions within the same dataset have been observed between lower (8–10.5 Hz) and upper (11–13 Hz) alpha oscillations, as well as between local and global properties (Klimesch, 1999; Nunez et al., 2001; Nunez and Srinivasan, 2006).
The individual alpha frequency (IAF) refers to the dominant frequency within the alpha power distribution and is thought to reflect the dominant neural circuits that generate alpha oscillations. Because it varies within and across individuals, measuring alpha power on each individual’s IAF better accounts for inter-individual variability (Klimesch, 1999; Haegens et al., 2014; Mierau et al., 2017). Individual alpha frequency estimates are considered a trait-like characteristic of the human EEG (Grandy et al., 2013), have high heritability (Smit et al., 2006), decrease with age (Klimesch, 1997; Corcoran et al., 2017; Finley et al., 2020), and have good test-retest reliability (Näpflin et al., 2007). Few studies have investigated EEG asymmetry using IAF estimates to our knowledge (Klimesch et al., 1998; Angelakis et al., 2004a; Vecchiato et al., 2012; Quaedflieg et al., 2015, 2016; Di Flumeri et al., 2016).
The first approach to estimate IAF is to use the peak alpha frequency (PAF; frequency within the alpha band with the highest power). While this technique has been extensively used for the study of cognition (Klimesch, 1999; Angelakis et al., 2004b; Rathee et al., 2020), it does not perform well with a portion of the population that have ambiguous alpha peaks, “split peaks” (i.e., several peaks within the alpha band), or no peak at all (Anokhin and Vogel, 1996; Chiang et al., 2008, 2011). A second approach called the alpha center of gravity (CoG) considers the shape of the alpha PSD distribution and is thought to provide a more accurate summary of the underlying alpha activity. Initial techniques to estimate IAFs relied on visual and manual inspection (Klimesch et al., 1990) or cross-frequency assumptions (Doppelmayr et al., 1998; Klimesch, 1999; Posthuma et al., 2001; Goljahani et al., 2012). These methods were very time-consuming and prone to subjective judgment error. Novel automated methods have now been developed to avoid these limitations. While the channel-based method (CRB; Goljahani et al., 2012, 2014) is better suited for event-related EEG asymmetry, other statistical curve-fitting and clustering techniques are particularly promising for IAF-estimation of resting EEG data (Chiang et al., 2008, 2011; Lodder and van Putten, 2011, 2013; Van Albada and Robinson, 2013; Corcoran et al., 2017). Corcoran et al. (2017) have implemented these algorithms into a fast, reliable, open-source toolbox operating in MATLAB and Python (Corcoran et al., 2017). This method seems suitable for large datasets with a relatively low signal-to-noise ratio (SNR) acquired with a wearable dry EEG system.
Hence, calculating alpha asymmetry scores on PSD estimated on the predefined alpha band (8–13 Hz), the lower (8–10.5 Hz) and upper (11–13 Hz) alpha sub-bands, and the CoG may help us understand more about the underlying mechanisms of alpha asymmetry. The present study incorporates these metrics to evaluate differences in these measures and their relationship to well-being. We expect well-being to be positively correlated with CoG values, differently correlated with lower and upper alpha (no specific direction is hypothesized), and positively correlated with CoG-asymmetry (and we expect this association to be stronger than that with the traditional whole alpha band asymmetry, by better accounting for interindividual differences).
Limiting Electroencephalographic Asymmetry to the Frontal Areas
The fourth limitation is the reduction of the study of EEG asymmetry phenomenon to only the frontal areas. It has been expressed for a long time that both anterior and posterior cortical regions show asymmetric activity patterns (Davidson, 1988, 1992). This is also reflected by studies showing that FAA obtained on data referenced with the current-source density (CSD) transformation (i.e., reflective of alpha power from local frontal sources only) correspond to a marker for depression risk, whereas FAA obtained on data referenced to mastoids or average (i.e., containing alpha power from distal, posterior cortical regions) correspond to a better marker of current depression severity (Stewart et al., 2010). Furthermore, expanding the analysis of alpha asymmetry to the temporoparietal (TP) regions seems particularly relevant since alpha asymmetries were source-localized to the frontoparietal network (FPN), which includes brain structures in both the frontal and the TP regions (see above; Vossel et al., 2013). Furthermore, different subtypes of anxiety disorders are differently associated with asymmetric activity in frontal and TP regions (Heller et al., 1997; Engels et al., 2007; Mathersul et al., 2008; Müller et al., 2015). Together, these findings suggest that anxious arousal (physiological arousal and hyper-reactivity under conditions of panic) is associated with relatively greater right than left frontal activation, whereas anxious apprehension (involving worry and verbal ruminations; i.e., trait anxiety and generalized anxiety disorder) is linked to the opposite asymmetry in frontal area and asymmetry in the same direction in the TP area. However, other findings suggested that TP asymmetry was less stable over time compared to frontal asymmetry (Müller et al., 2015) and sometimes not associated with self-reported measures of affect and motivation (Davidson et al., 1990). In this study, we examine the relationship between well-being and asymmetry in both frontal and TP regions and hypothesize that alpha asymmetry in both regions will be associated with well-being (with potentially a different direction).
Limiting Electroencephalographic Asymmetry to the Alpha Oscillations
The Fifth and last main limitation in EEG asymmetry research is the need to expand analyses to other frequency bands. Coherence in both alpha and theta oscillations has been highlighted during both relaxation and mental calculation (Nunez and Srinivasan, 2006). This widespread (global) phase coherence phenomenon increases in the upper frequencies of both alpha and theta bands while it simultaneously decreases in the lower frequencies (Wingeier, 2000; Nunez and Srinivasan, 2006). These findings go along with other findings indicating that global alpha and theta rhythms functionally interact during both relaxation and attentional tasks (Klimesch, 1999; Buzsáki, 2006; Laufs et al., 2006). Furthermore, theta power has been used to predict response to depression treatment in several studies (Knott et al., 1996, 2000; Cook and Leuchter, 2001; Cook et al., 2002; Bares et al., 2008; Iosifescu et al., 2009; Spronk et al., 2011; Baskaran et al., 2012; Olbrich and Arns, 2013). Furthermore, theta power decreases while upper alpha power increases in several conditions (i.e., the early part of life until adulthood, in neurological disorders, and the transition phase from awake to sleeping), whereas the direction of their relationship is opposite for the late part of the lifespan (Klimesch, 1999).
Similarly, alpha and beta spectral power have been found to interact (Laufs et al., 2006; Hamid et al., 2010), and both are associated with high levels of mental stress and depression (Hayashi et al., 2009; Alonso et al., 2015; Jena, 2015; Al-shargie et al., 2016; Jun and Smitha, 2016; Díaz et al., 2019; Al-Dabass, 2020; de Hemptinne et al., 2021). More specifically, prefrontal beta power in lateral areas was found to be positively associated with depression and anxiety, whereas lateral beta power was negatively associated with mood (de Hemptinne et al., 2021). The authors interpreted these results to be in line with the organization of the reward networks in the prefrontal cortex (PFC).
However, no robust literature is available to make specific interpretations about how alpha asymmetry interacts with other frequency bands, and whether asymmetries in other frequency bands could be associated with psychological constructs such as well-being. Thus, we aim to bring light to this matter in this study and hypothesize that well-being will be associated with asymmetries in other frequency bands. This study includes asymmetry scores estimated on the delta (1–3 Hz), theta (4–7 Hz), and beta (14–30 Hz) frequency bands, for both frontal and TP sites. Since no previous research exists on this matter, we have no specific hypothesis concerning the direction of these potential associations.
Summary of the Study Goals and Hypotheses
Considering the potential importance of alpha asymmetry as a physiological correlate in general, and for well-being specifically, the overall objective of this study was to determine whether a low-cost wearable EEG headset (the Muse by Interaxon) could be used to measure EEG correlates (CoG, EEG asymmetry) of well-being on a relatively large sample (N = 353). The analyses were designed to address the main limitations of EEG asymmetry research addressed above. The hypotheses for the study were as follows:
1. Well-being will be positively associated with approach-motivation processes and positive valence of emotion, as reflected by relatively greater right than left alpha power. We hypothesize that this will be the case for both frontal and temporoparietal (TP) areas (although the direction might be different, based on the literature discussed).
2. Age and gender will be associated with both well-being and mean alpha asymmetry (predefined 8–13 Hz band).
3. The CoG will be positively correlated with well-being levels.
4. Asymmetry scores estimated on sub-components of alpha oscillations (namely lower/upper alpha and CoG) will provide stronger correlations regarding the relationship between well-being and alpha asymmetry than those estimated on the predefined alpha aband (8–13 Hz), by better accounting for alpha source differences (lower/upper alpha) and interindividual differences (CoG).
5. Well-being levels will be associated with asymmetries in other frequency bands (namely delta, theta, and beta), although we do not have specific hypotheses regarding which bands and their directions.
Materials and Methods
Participants
353 participants were recruited from groups attending workshops focusing on well-being and personal development at the Earthrise Campus. Exclusion criteria: people younger than 18 years of age, inability to read or understand the consent form, acute or chronic illness precluding completion of measurements. Upon arrival at the research laboratory, participants were briefly interviewed by the research assistants to ensure they met the inclusion/exclusion criteria and were then allocated to a carrel where the following equipment was available for their participation: a wearable EEG headset, a Chromebook, and a pair of headphones. The settings allowed the recording of up to 9 participants simultaneously. Participants volunteered and were not compensated for participation. The study and the consent form were approved by the Institute of Noetic Sciences’ institutional review board (IRB). All questionnaires were optional and anonymous.
Multidimensional Well-Being
Participants’ multi-dimensional well-being was assessed on-site using the Arizona Integrative Outcomes Scale (AIOS; Bell et al., 2004) in SurveyMonkey1. The AIOS is a horizontally displayed scale that provides a quick and accurate assessment of the participants’ self-rated global sense of physical, social, psychological, affective, and spiritual well-being over the past 24 h (Bell et al., 2004). The low anchor is “Worst you have ever been” (AIOS score = 0) and the high anchor is “Best you have ever been.” (AIOS score = 100). The 24-h AIOS score was found to significantly reflect psychological well-being, global health, psychological distress, the positive and negative affect, and the positive states of mind, and was significantly correlated with the 1-month AIOS scores (Bell et al., 2004; Otto et al., 2010; Tuason et al., 2021). Furthermore, AIOS-24 h was found to be associated with personality traits (Wahbeh et al., 2021). While these findings suggest the AIOS-24 h reflects trait components of well-being, validation of this hypothesis requires further testing. The online survey included additional questionnaires that are not included in this study and are reported elsewhere (Wahbeh et al., 2021).
EEG
Data Collection
Once participants completed the survey, continuous EEG was recorded using InteraXon’s Muse wearable EEG headband (version 2016). Electroencephalography data were recorded while participants were instructed to focus their attention on their breath and count inhalation/exhalation cycles. They were instructed to bring their attention back to their breath and start counting again if they lost track of their count or noticed their minds wandered. This task reduces EEG artifacts occurring naturally with eye movements. Most importantly, this task can later be implemented into practical translational and therapeutical applications aimed at increasing well-being levels through the modulation of alpha asymmetry and the underlying brain processes (Angelakis et al., 2007; Sessa, 2007; Moynihan et al., 2013; Doll et al., 2016; Schmalzl et al., 2018; Prpa et al., 2020). Electroencephalography data were with a sampling rate of 256 Hz and 12-bits of data resolution. This system has five active dry electrodes: two frontal silver (AF7 and AF8), two temporoparietal (TP) silicone electrodes (TP9 and TP10), and a reference electrode (FPz). Before positioning the headband on the subjects’ heads, their skin was cleaned with alcohol swipes at electrode sites, and a thin layer of water was applied with a sponge to the electrodes to improve signal quality. EEG data were acquired on Chromebooks using the Muse Monitor App and were uploaded onto Dropbox at the end of the recording. Random unique identifiers were used to link survey and EEG data. Impedance check was provided by the App (horseshoe symbol) and visually confirmed by the raw signal displayed on the screen in real-time.
As shown in previous publications, good internal consistency reliability of frontal EEG asymmetry can be obtained with as few as 100 epochs, corresponding to one to 3 min of artifact-free recorded data [depending on window size; (Allen et al., 2004; Towers and Allen, 2009; Smith et al., 2017)]. Allen et al. (2004) found that the number of epochs used to estimate the asymmetry scores matters more than the number of minutes of data (Allen et al., 2004), with asymmetry scores estimated on 2 min of data showing similar consistency reliability than those obtained on 8 min of data. Furthermore, a recent publication showed that individuals can robustly be differentiated using spectral EEG data obtained on segments as short as 30 s (and this was stable weeks later; da Silva Castanheira et al., 2021). Thus, 2 min of EEG data were recorded for each participant. When less than 8 min of data is available, Allen et al. (2004) recommend reporting the internal consistency reliability and how many blocks were treated through the calculation of Cronbach’s alpha (see below).
Data Preprocessing
Data preprocessing was done in EEGLAB version 2020.0 (Delorme and Makeig, 2004) in MATLAB v2020a. EEG data were imported with the muse_monitor plugin v3.2, low-pass filtered at 30 Hz (transition bandwidth 12.5 Hz; passband edge 50 Hz; cutoff frequency -6 dB 56.25 Hz; linear non-causal filter) to remove high-frequency artifacts, and high-passed filtered at 1 Hz (transition bandwidth 1 Hz; passband edge 1 Hz; cutoff frequency -6 dB 0.5 Hz; linear non-causal filter) to remove low-frequency drifts. 10–20 channel template locations from BESA spherical coordinates were used in EEGLAB. Artifactual channels (with ∼50% of data being noisy or artifactual) were manually tagged and removed with a custom-made single-page figure displaying each channel’s overall raw data, standard deviation, and power spectra. Files with at least one bad channel were removed for analyses.
An existing automatic method to clean EEG artifacts over this large sample was cross-validated: 150 files were randomly selected from the database to be cleaned manually and automatically with EEGLAB’s clean_rawdata plugin v2.2 (Euclidean method). Performance was calculated on each channel by comparing each sample as either true positive (TP, bad sample correctly rejected), true negative (TN, good sample correctly kept), false positive (FP, good sample incorrectly rejected), or false negative (FN, bad sample incorrectly kept). “Positive” and “negative” refer to presence or absence. Then, the true positive rate (TPR, i.e., sensitivity) and the true negative rate (TNR, i.e., specificity or selectivity) were calculated for each channel with: TPR = TP/(TP + FN) and TNR = TN/(TN + FP). The average sensitivity and specificity were then calculated over all channels to obtain the overall performance of the automatic method compared to manual rejection. After testing different parameters, the best performance obtained showed 81% sensitivity and 83% specificity [settings: “burst_criteria” = 6, “window_criteria” = 0.3, “window_tolerance” = “(-Inf 7)”]. 50 additional datasets were randomly selected for cross-validation, showing 84% sensitivity and 89% specificity. Since further increasing the sensitivity scores (i.e., removing more subtle artifacts) corresponded to a decrease in specificity (i.e., removing more non-artifactual data), these thresholds were considered most suited for this analysis. On average, this method removed an additional 11.4 s of data (± 23.0). Thus, bad channels were manually tagged and data were cleaned using this automated method and parameters. Files with less than 60 s of remaining artifact-free data were removed for analysis.
Note that this was done on duplicated data that were averaged-referenced to a fifth zero-filled channel as it increased performance by homogenizing raw signal amplitude across channels. But because this average re-referencing method was not validated for this specific montage and is not recommended with less than 30 channels (Smith et al., 2017), artifactual sections were removed from the original raw files and then re-processed as above. The issue of the electrode reference and its impact on asymmetry scores has been detailed and is of high importance (Allen et al., 2004; Smith et al., 2017). The recommended referencing methods (i.e., average-referencing, current-source density transformation) or the “residualization procedure” are not feasible with the low density and sparse montage of the Muse headset. The frontal channels are located close to the Fpz reference, potentially providing invalid asymmetry scores for the frontal channels by not reflecting the same underlying cortical activity as in the literature. Since frontal asymmetry estimated on linked-mastoid data is associated with the severity of current depression (Stewart et al., 2010), frontal channels were re-referenced to TP9/TP10. Temporoparietal channels were kept with the default Fpz reference.
Power Spectral Density and Asymmetry Estimates
Power Spectral Density (PSD) was calculated using MATLAB’s pwelch function on 1-s hamming tapered windows (42.5 dB sidelobe attenuation) with 50% overlap [per guidelines (Allen et al., 2004; Smith et al., 2017)], since the pwelch method smooths over non-systematic noise and is more robust compared to the more popular fft method that is more sensitive to noise and non-stationarities. Power spectra were then converted to 10*log10 deciBels (dB) as untransformed power values tend to be positively skewed due to individual differences in skull thickness that influence the signal amplitude (Allen et al., 2004).
The CoG was estimated for each channel using the automated, open-source method developed by Corcoran et al. (2017) which uses curve-fitting algorithms and a smoothing Savitzky-Golay Filter (SGF). This technique is thought to better account for interindividual variance and to be more reliable under low SNR conditions.
Asymmetry scores were obtained on the alpha PSD averaged over the predefined band (8–13 Hz), averaged over the predefined lower (8–10.5 Hz) and upper (11–13 Hz) sub-bands, and the individualized CoG.
They were calculated following standard procedures by subtracting the alpha power of interest of the left frontal channel from the right frontal channel (alpha_power_dB_AF8 – alpha_power_dB_AF7). Positive scores, therefore, indicate greater alpha power in the right relative to the left electrode. Asymmetry scores were also obtained from the temporoparietal (TP) channels. Finally, asymmetry scores were also computed on the delta (1–3 Hz), theta (4–7 Hz), and beta (14–30) frequency bands. Gamma was not included due to the Muse’s vulnerability to line noise in the high frequencies.
Statistical Analyses
Robust linear regression models were generated in MATLAB 2021a using MATLAB’s fitlm package. Because of small portions of artifacts remaining in some EEG data after automatic preprocessing, robust least-squares regressions (Tukey’s bisquare function; default tuning constant = 4.685) were used for statistical analysis to down-weight the residuals’ influence on the model, using iterative reweighted least-squares (IRLS; Huber and Ronchetti, 2009). All models were tested for lack of fit first using a degenerate model consisting of only a constant term. Reported F-statistics with a p-value, therefore, indicate a valid fit for the model but do not inform on the relationship between the dependent and independent variables. The Beta (β) coefficient estimates and their standard error (SE) are reported in the first column and indicate a significant linear relationship between the predictor and the outcome variables when p-values are present. Summary statistics of the models include the number of observations, the error degrees of freedom, the root mean squared error (RMSE), R2 (for models with one predictor), adjusted R2 (for models with multiple predictors). Note that the descriptions below each table reporting the statistical results indicate whether the models were simple or multiple linear regressions (i.e., one or more predictor variables). In sum, all models were simple linear models and one was a multiple linear model (the two variables being lower and upper alpha asymmetry). Finally, following recommendations (Allen et al., 2004), asymmetry scores were also calculated on eleven 4-s blocks (as opposed to the average alpha power over all blocks for the asymmetry measures) to validate the internal reliability consistency of alpha asymmetry scores obtained on these short file lengths, using Cronbach’s alpha method, where a value below 0.2 indicates poor internal reliability consistency and greater than 0.8 a high internal reliability consistency (Cronbach, 1951).
Results
230 participants remained for analyses after preprocessing. 83 files contained at least one bad channel and 36 had less than 60 s of artifact-free data and were excluded from the analyses (the data loss due to signal quality is discussed in the Discussion). They were aged from 22 to 80 years old (mean age was 55 ± 13.4) and were 64.3% female, 28.7% males, and 7% “Other” or missing. Cronbach’s alpha scores indicated a high internal reliability consistency of the asymmetry scores estimated on both frontal (Cronbach α = 0.95) and temporoparietal (Cronbach α = 0.82) channels.
Well-Being and Alpha Asymmetry (Predefined Frequency Bands)
No association between subjective well-being levels and frontal alpha (predefined 8–13 Hz band) asymmetry was found (Figure 1 and Table 1). However, well-being was negatively correlated with TP alpha asymmetry scores (predefined 8–13 Hz band), reflecting greater cortical activity in the right TP area relative to the left is associated (assuming the inhibitory role of alpha oscillations on regional cortical activity; see Introduction). Detailed statistics are reported in Table 1 and an illustration of the results in the frequency and the scalp topography domain can be found in Figure 1, using the 20 participants with the highest well-being levels. The relationship between well-being and TP total alpha asymmetry scores appear to be driven more specifically by neural activity in the lower frequencies of the alpha band (8–10.5 Hz) because well-being was significantly correlated with lower alpha asymmetry but not with upper alpha asymmetry (see Table 2).
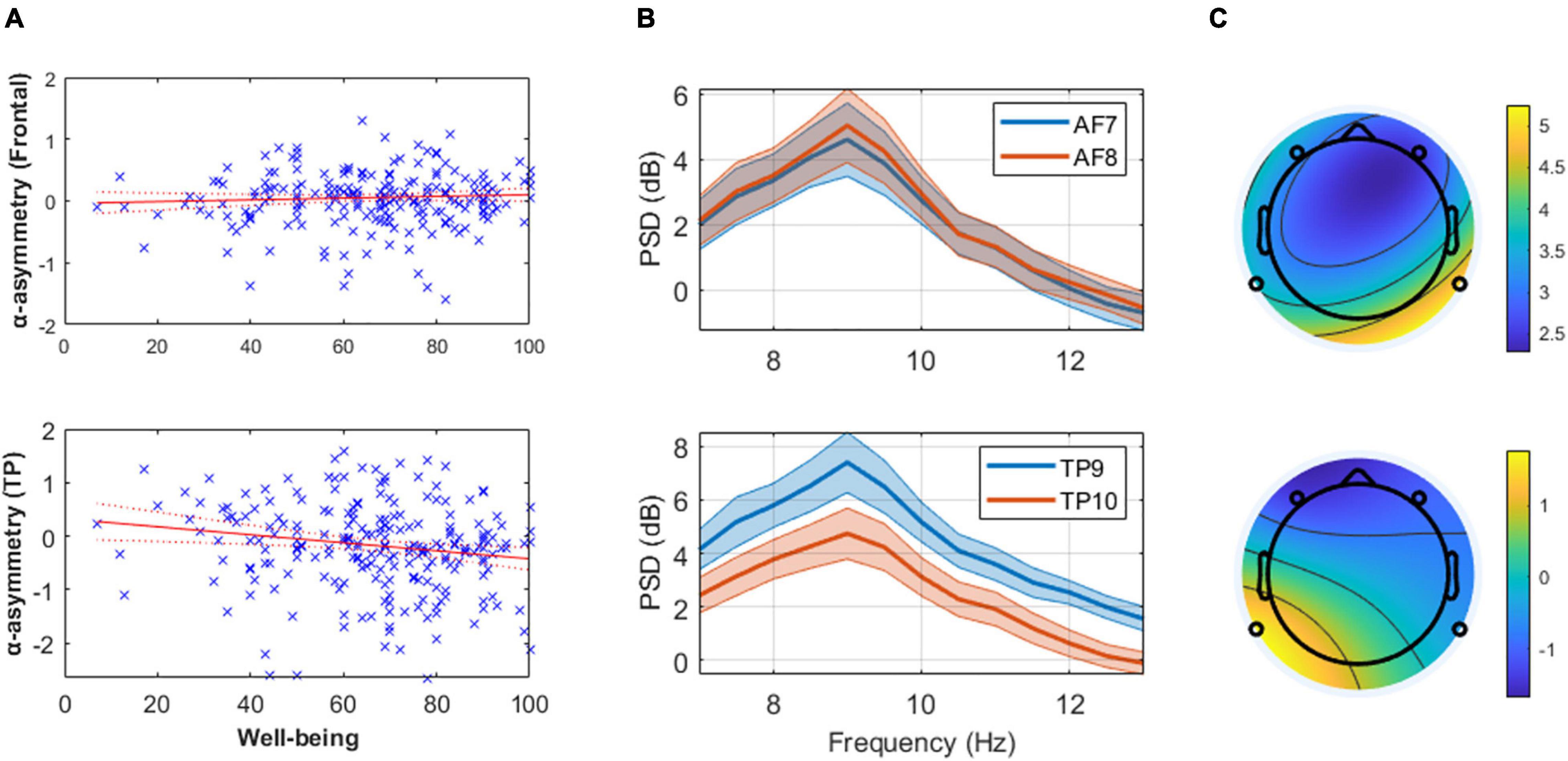
Figure 1. (Panel A) These linear regression models of well-being and mean alpha asymmetry (predefined 8–13 Hz band) show the absence of relationship at frontal channels (top) and the presence of one at temporoparietal (TP, bottom) channels. Higher well-being levels are associated with greater cortical activity in the right TP area relative to the left (assuming alpha inhibits regional cortical activity). (Panel B) Mean and standard error of the alpha power spectral density (PSD) from the 20 participants with highest reported well-being level at frontal (top) and TP (bottom) channels, illustrating the results reported in (Panel A). (Panel C) Scalp topography of mean alpha PSD on a typical subject with low self-reported well-being (AIOS = 17; top) and high self-reported well-being (AIOS = 100; bottom), as an illustration of the effect reported in (Panel A).
Well-Being, Alpha Asymmetry (Predefined 8–13 Hz Band), and Covariates
Age was negatively correlated with alpha asymmetry calculated on the predefined 8–13 Hz band (meaning the older the individual, the greater cortical activity is in the right frontal and TP areas relative to the left ones) and positively correlated with subjective well-being levels (i.e., older age reflecting greater well-being score). However, gender was not associated with well-being or alpha asymmetry (Figure 2 and Table 3).
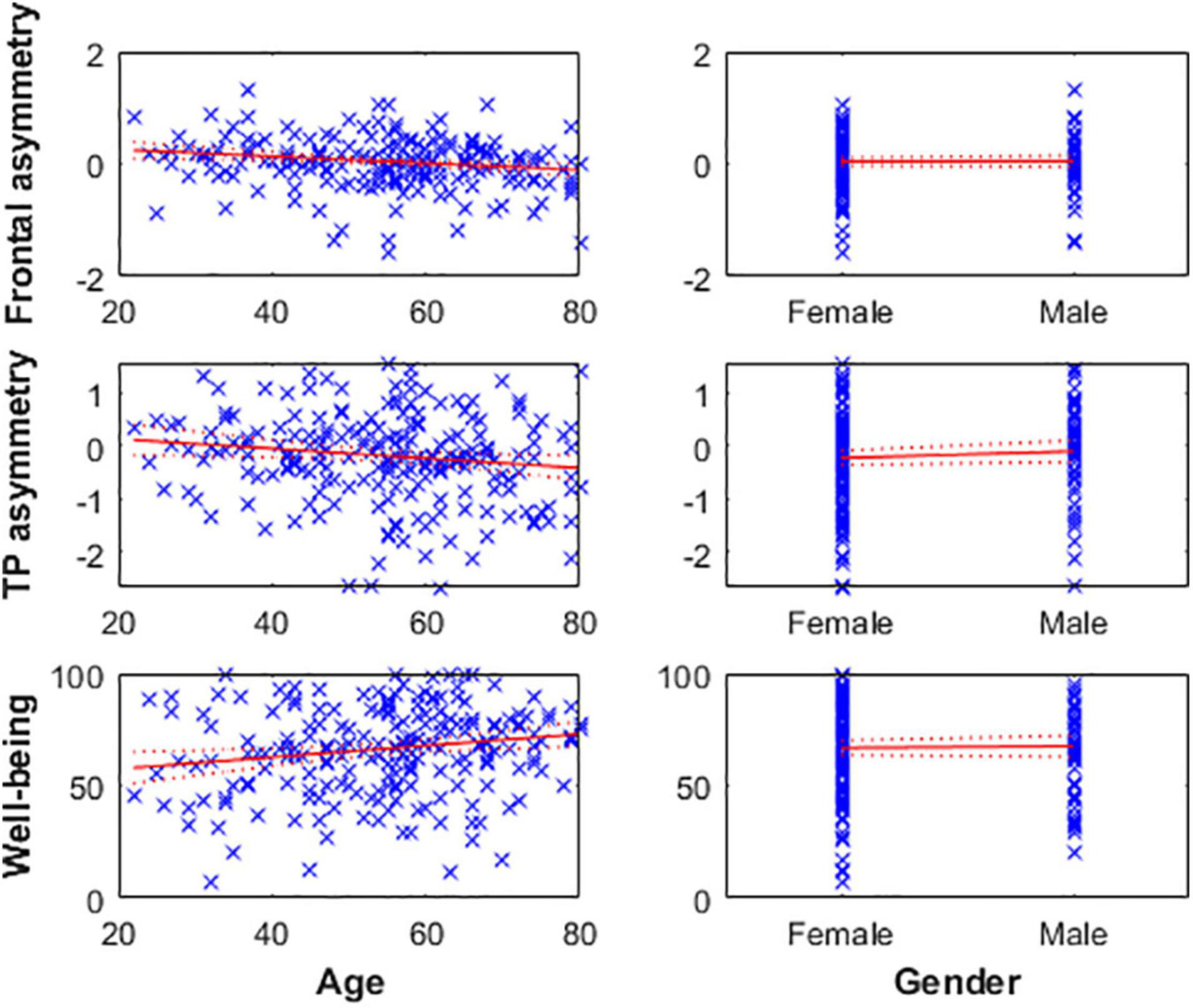
Figure 2. Left: Age is negatively associated with frontal (top) and TP (middle) alpha asymmetry scores, reflecting greater cortical activity in the right hemisphere relative to the left in older individuals. Age is positively associated with well-being levels (bottom). Right: Gender was not associated with any of the three variables.
Well-Being, Alpha Center of Gravity, and Center of Gravity-Asymmetry
No linear relationships were observed between well-being and the CoG (Supplementary Table 2), and between well-being and asymmetry scores calculated on the CoG (Supplementary Table 3), for both frontal and TP channels.
Well-Being and EEG Asymmetry in the Other Frequency Bands
No associations were observed between well-being and EEG asymmetry in the delta (1–3 Hz), theta (3–7 Hz), or beta (14–30 Hz) frequency bands (Supplementary Figure 1 and Supplementary Table 1).
Discussion
Results Summary
Contrary to the existing literature on the emotional valence and the motivational models of frontal EEG asymmetry, we found an absence of association between multidimensional well-being levels and frontal alpha asymmetry (predefined 8–13 Hz band, 8–10.5 Hz, and CoG-asymmetry). However, well-being was negatively correlated with alpha asymmetry at the TP sites (predefined 8–13 Hz and 8–10.5 Hz bands, but not for CoG-asymmetry), reflecting greater cortical activity in the right TP area relative to the left (assuming the inhibitory role of alpha oscillations on regional cortical activity; see Introduction). Interestingly, the direction of the asymmetry is opposite to the one in the frontal areas in the literature of frontal alpha asymmetry. Hence, while approach motivation and the related emotional processes are associated with relatively greater left than right frontal cortical activation, multidimensional well-being seems to be associated with asymmetric activation in the opposite direction in the TP areas.
This effect appears to be driven more specifically by oscillatory activity in the lower frequencies of the alpha band (8–10.5 Hz), aligning with studies highlighting the inhibitory function of these lower frequencies (Oakes, 2004). Making the distinction between lower and upper frequencies of the alpha band seems therefore especially relevant for neurophysiological studies using source-localization or simultaneous EEG-fMRI techniques to identify the intricate mechanisms involved in EEG asymmetry.
Contrary to our expectations, the CoG did not show associations with well-being levels. While CoG is associated with cognitive processes in the literature on the individual alpha frequency (IAF), we hypothesized that it would also be associated with self-reported well-being levels. However, the CoG may reflect other brain processes associated with cognition that are different than those involved with multidimensional well-being. Future studies using advanced source localization methods and high-density EEG systems should elucidate the different sources and networks associated with the different sub-components of alpha oscillations, and their associations with cognitive systems (i.e., PAF, CoG, lower/upper alpha).
While some researchers suspected that gender was the main driver of frontal alpha asymmetry levels (Gale et al., 2001; Dennis and Solomon, 2010; Mikolajczak et al., 2010), it was not associated with well-being or alpha asymmetry measures (for both frontal and temporoparietal sites) in this sample. However, age was negatively correlated with alpha asymmetry scores of both regions (meaning that cortical activity is greater in the right areas relative to the left ones as age increases) and positively correlated with subjective well-being levels. This finding aligns with the well-being literature (e.g., Carstensen et al., 2011), and supports a strong mediator role of age on the relationship between well-being and TP alpha asymmetry. Hence, the absence of a relationship between well-being and CoG-asymmetry might further indicate that there is a strong relationship between well-being, age, and alpha asymmetry in the TP area. Age is likely not the mechanism of change itself but may represent many underlying factors associated with brain changes and well-being (Kazdin, 2007). Thus, future studies using larger samples and higher density EEG data are necessary to confirm the accuracy of the asymmetry estimates obtained with this automated method, as well as to confirm or disprove the relationship between age, well-being, and alpha asymmetry in the TP area. If confirmed, the IAF-estimation method can be used to homogenize EEG asymmetry estimation procedures across investigators, and the specific interactions between these three variables should be further elucidated to determine the underlying mechanisms.
No associations were observed between subjective well-being and PSD asymmetry in the delta (1–3 Hz), theta (3–7 Hz), or beta (14–30 Hz) frequency bands (Supplementary Figure 1 and Supplementary Table 1), supporting the specific role of alpha oscillations in the brain processes underlying well-being.
Interpretations of the Results and Potential Mechanisms
Studies using source-localization methods found the alpha asymmetry to originate mainly from brain activity in the dorsal system of the frontoparietal network (FPN; 13). Functional magnetic resonance imagery (fMRI) showed that this system is organized bilaterally and comprises the intraparietal sulcus (IPS) and the frontal eye fields (FEF) of each hemisphere, and is thought to mediate top-down guided voluntary allocation of attention to locations or features (Vossel et al., 2013). Both IPS and FEF are active when attention is overtly or covertly oriented in space and are suspected to be the regions for the maintenance of spatial priority maps, saccade planning, and visual working memory. In contrast, the ventral system comprises the temporoparietal junction (TPJ) and the ventral frontal cortex (VFC) and is associated with detecting unattended or unexpected stimuli and triggering shifts of attention (Vossel et al., 2013). It has been proposed that the ventral system is lateralized to the right hemisphere of the brain and exhibits asymmetric activity during attentional reorientation, the processing of rare deviant stimuli, and the response to valid vs. invalid cued targets (Corbetta and Shulman, 2002; Corbetta et al., 2008; Doricchi et al., 2010). The functional role of the TPJ also includes filtering irrelevant distractors during focused states of attention, modulating neural activity between various networks, and it has been implicated in social cognition and theory of mind (Vossel et al., 2013).
Hence, since our experimental task consisted of focusing attention on the breath, detecting mind-wandering thoughts (i.e., mental distractions), and reallocating attention to the goal, the TP alpha asymmetry may reflect these attentional processes and the underlying activity of the TPJ. Whereas, frontal alpha asymmetry may better reflect the dorsal system, as most studies use traditionally a cross-fixation task or resting-state condition with no focus of attention on any object. In line with these systems, one might speculate that participants with lower subjective well-being were more likely to ruminate on negative thoughts or memories (associated with negative valence and a withdrawal motivation; Mason et al., 2013; Smallwood and Andrews-Hanna, 2013) and less able to redirect their attention to their breath. This would decrease their capacity to detect negative thoughts and redirect their attention to their breath, corresponding to relatively greater left than right cortical activity in the TP area (positive TP asymmetry score). On the other hand, participants with higher well-being would be more likely to engage in mind wandering with positive valence and more likely to redirect their attention to their breath, which would correspond to greater cortical activity in the right TP area (negative TP asymmetry score). Another possibility is that alpha asymmetry in the TP regions might simply occur in opposite direction compared to the alpha asymmetry in the frontal areas (Davidson et al., 1990). Future studies using high-density systems and advanced source-localization methods are necessary to confirm or disprove this hypothesis.
Limits and Recommendations
There are several limitations of this study that should be considered when reviewing the results.
While the AIOS-24h was found to be associated with longer-term well-being levels (i.e., reported well-being levels reflective of the past month and personality trait; see Methods), further validation is required to fully validate it as a measure of trait well-being.
While the asymmetry scores showed a relatively high internal reliability consistency and the Muse was validated for ERP research (Krigolson et al., 2017), 83 files had at least one bad channel and 36 had less than 60 s of remaining artifact-free data after preprocessing. This is a significant loss of data. The largest loss of data came from the presence of bad channels (considered bad when at least 50% of the channel was artifactual), likely due to the headband’s flexibility that is prone to moving and disconnecting electrodes. Thus, future investigators could consider using the more recent Muse S that was developed for sleep studies. The Muse S is made of a flexible fabric that can stretch and keep stronger pressure on the electrodes, preventing them from disconnecting as much. Furthermore, we recorded the data when participants already started the task with their eyes closed to reduce data cleaning over the large sample. Automatic cleaning performance would have likely been increased by adding a period before the task that includes obvious artifacts (e.g., asking participants to produce eye blinks and jaw clenching) to help the automatic method algorithms create a more robust baseline and therefore reject artifacts more efficiently. Thus, higher-grade and -density wearable EEG systems and longer recordings (at least 4 min of continuous data to ensure having at least 2 min of artifact-free data on a larger portion of the sample) are recommended for future studies to keep the advantages of wearable technologies to acquire large datasets without compromising data quantity and quality.
The Muse has only four channels. There are obvious benefits to having more EEG channels in terms of scalp distribution and data quality, which allow the use of advanced methods such as independent component analysis (ICA) which can be used to remove subtle artifacts such as muscle activity, subtle eye movements, or channel noise (Makeig et al., 1996; Delorme and Makeig, 2004). Furthermore, while we controlled for the potential reference issue using this system, a wearable headset with at least 30 channels would allow multiple referencing methods (e.g., average or CSD) and ensure highly accurate asymmetry estimates. However, this study showed that it is feasible to use a low-cost, low-density wearable system to examine the relationships between well-being and alpha asymmetry in a relatively large and diverse population.
Alpha center of gravity (CoG) and therefore CoG-asymmetry is expected to better account for interindividual differences. The automated IAF-estimation toolbox used in this study was not able to detect the CoG for 8 subjects (see Supplementary Tables 2, 3). We wanted to ensure that the absence of association between well-being and TP asymmetry calculated on the CoG was not due to this small sample difference (8 subjects missing compared to models on predefined alpha bands). Thus, we removed these 8 subjects from the model assessing the association between well-being and TP-asymmetry (predefined 8–13 Hz band) to see if the effect disappeared as a consequence of these 8 subjects being removed. Results showed that the significant association was still present (see Supplementary Table 4). Hence, this absence of association between well-being and CoG-asymmetry is either due to:
1) poorer estimation of alpha activity by the automatic method compared to the predefined band since the method performs best with more neighboring EEG channels (and the Muse has only four sparse channels). Here, we fed the algorithm with 2 channels at a time to avoid alpha contamination from distal channels (to keep alpha activity from frontal and TP channels separate).
2) this method better accounting for interindividual differences, which would indicate that the main effect (TP asymmetry calculated on the predefined 8–13 Hz band) might be a consequence of the relationship between age, well-being, and related brain activity.
Lastly, cross-sectional designs are always a limitation to consider. More sessions would be beneficial for the field to confirm the results and assess changes in both well-being and EEG asymmetry to evaluate the stability of this relationship over time.
Long Term Applications and Goals
Attentional and inhibitory impairments are thought to be crucially associated with an increased vulnerability to depressive episodes and cognitive vulnerability (De Raedt and Koster, 2010). Alpha asymmetry (both frontal and TP) seems to play an essential role in understanding the neural networks underlying executive functions, attention, emotion regulation, and well-being. A better understanding of these processes is crucial to improving general well-being levels via targeted interventions. For example, Xu et al. (2018) found that positive psychological interventions (PPIs) increased not only subjective well-being and relief in depression but also left frontal asymmetry scores (Xu et al., 2018). Kim et al. (2012) found that positive reappraisals (i.e., techniques to recognize the negative pattern that one’s thoughts have taken using meta-awareness to cognitively reframe an event as more positive and therefore increase the sense of well-being) showed an increase in metabolic activity in the left dlPFC, caudate, and cingulate regions (Kim et al., 2012). Moynihan et al. (2013) found that mindfulness-based stress reduction produced significant changes in executive and immune functions, as well as in left frontal alpha asymmetry scores.
Neuroscientific tools such as neurofeedback (Linden, 2014; Brandmeyer and Delorme, 2020b) might increase these interventions’ efficacy by targeting brain networks on the same occasion. For instance, Angelakis et al. (2007) improved cognitive processing speed and executive function of elderly individuals using PAF as a neurofeedback index (Angelakis et al., 2007). Allen et al. (2001) found that increasing right frontal activity relative to the left using frontal asymmetry neurofeedback led to decreased positive affect (Allen et al., 2001).
Furthermore, neuromodulation techniques may be used to directly modulate specific networks such as the FPN. For example, some clinical studies have shown that exciting the left dlPFC with transcranial magnetic stimulation (TMS) or transcranial direct current stimulation (tDCS) improved depression symptoms (Kalu et al., 2012). Conversely, excitation of the right dlPFC led to reductions in craving (Boggio et al., 2008; Fregni et al., 2008) and risky decision-making (Fecteau et al., 2007), i.e., behaviors associated with difficulty in inhibiting extreme rewards with positive valence. Additionally, Sanguinetti et al. (2020) recently used novel transcranial focused ultrasound stimulation to target the right prefrontal cortex with higher resolution and depth than TMS or tDCS and successfully modulated mood and emotion regulation. By modulating both bottom-up and top-down systems, long-term solutions without side effects and at lower costs will emerge by helping patients self-control negative biases (Moser et al., 2002; Hanslmayr et al., 2011).
Understanding the role of third variables on these mechanisms will help adapt these therapies to meet each individual’s anatomy, physiology, and medical history, for more efficiency and safety. Once these intricacies are better understood, neuromodulation therapies might positively affect both the executive control and perceptive systems to decrease the propensity of depressive patients to focus on negative information and ruminative thought.
Finally, advancements in wearable technologies may allow care providers to monitor patients and apply neurofeedback or neuromodulation protocols at a low cost and remotely while patients are in the comfort of their homes (Cannard et al., 2020; Biondi et al., 2021).
Conclusion
Overall, this study brings practical methodological information, challenges, and guidelines for conducting EEG research on large samples on well-being or related neuropsychological constructs, using wearable EEG technologies. Our findings bring novel knowledge that will help deepen our understanding of EEG asymmetries and their relations with well-being, the potential underlying neural networks and mechanisms, and the foreseeable long-term applications.
Data Availability Statement
The datasets presented in this study can be found in online repositories. The names of the repository/repositories and accession number(s) can be found below: https://osf.io/nq7ga/.
Ethics Statement
The studies involving human participants were reviewed and approved by Institute of Noetic Sciences’ Institutional Review Board. The patients/participants provided their written informed consent to participate in this study.
Author Contributions
CC, HW, and AD made substantial contributions to the conception and design of the work, made substantial contributions to revising it critically for important intellectual content, and agreed to be accountable for all aspects of the work in ensuring that questions related to the accuracy or integrity of any part of the work are appropriately investigated and resolved. CC made a substantial contribution to the acquisition, analysis, interpretation of data, and writing the work. HW and AD provided approval for the publication of the content. All authors contributed to the article and approved the submitted version.
Funding
This work was funded by the Occitanie Region (France) and the Institute of Noetic Sciences (non-profit research organization, CA, United States). Grant number: contrat LSP n° 183258, BIGDAT, UMR5549.
Conflict of Interest
The authors declare that the research was conducted in the absence of any commercial or financial relationships that could be construed as a potential conflict of interest.
Publisher’s Note
All claims expressed in this article are solely those of the authors and do not necessarily represent those of their affiliated organizations, or those of the publisher, the editors and the reviewers. Any product that may be evaluated in this article, or claim that may be made by its manufacturer, is not guaranteed or endorsed by the publisher.
Supplementary Material
The Supplementary Material for this article can be found online at: https://www.frontiersin.org/articles/10.3389/fnhum.2021.745135/full#supplementary-material
Footnotes
References
Ahn, J. W., Ku, Y., and Kim, H. C. (2019). A novel wearable EEG and ECG recording system for stress assessment. Sensors 19:1991. doi: 10.3390/s19091991
Al-Dabass, D. (2020). Novel methods for stress features identification using EEG signals. Int. J. Simul. Syst. Sci. Technol. 12, 27–33. doi: 10.5013/IJSSST.a.12.01.04
Alexandrova, A. (2015). Well-being and philosophy of science. Philos. Compass 10, 219–231. doi: 10.1111/phc3.12203
Allen, C., Coan, J. A., and Nazarian, M. (2004). Issues and assumptions on the road from raw signals to metrics of frontal EEG asymmetry in emotion. Biol. Psychol. 67, 183–218. doi: 10.1016/j.biopsycho.2004.03.007
Allen, J. J. B., and Reznik, S. J. (2015). Frontal EEG asymmetry as a promising marker of depression vulnerability: summary and methodological considerations. Curr. Opin. Psychol. 4, 93–97. doi: 10.1016/j.copsyc.2014.12.017
Allen, J. J., Harmon-Jones, E., and Cavender, J. H. (2001). Manipulation of frontal EEG asymmetry through biofeedback alters self-reported emotional responses and facial EMG. Psychophysiology 38, 685–693.
Alonso, J. F., Romero, S., Ballester, M. R., Antonijoan, R. M., and Mañanas, M. A. (2015). Stress assessment based on EEG univariate features and functional connectivity measures. Physiol. Meas. 36, 1351–1365. doi: 10.1088/0967-3334/36/7/1351
Al-shargie, F. M., Tang, T. B., Badruddin, N., and Kiguchi, M. (2016). “Mental stress quantification using EEG signals,” in International Conference for Innovation in Biomedical Engineering and Life Sciences, eds F. Ibrahim, J. Usman, M. S. Mohktar, and M. Y. Ahmad (Cham: Springer), 15–19. doi: 10.1007/978-981-10-0266-3_4
Angelakis, E., Lubar, J. F., and Stathopoulou, S. (2004a). Electroencephalographic peak alpha frequency correlates of cognitive traits. Neurosci. Lett. 371, 60–63. doi: 10.1016/j.neulet.2004.08.041
Angelakis, E., Lubar, J. F., Stathopoulou, S., and Kounios, J. (2004b). Peak alpha frequency: an electroencephalographic measure of cognitive preparedness. Clin. Neurophysiol. 115, 887–897. doi: 10.1016/j.clinph.2003.11.034
Angelakis, E., Stathopoulou, S., Frymiare, J. L., Green, D. L., Lubar, J. F., and Kounios, J. (2007). EEG neurofeedback: a brief overview and an example of peak alpha frequency training for cognitive enhancement in the elderly. Clin. Neuropsychol. 21, 110–129. doi: 10.1080/13854040600744839
Anokhin, A., and Vogel, F. (1996). EEG Alpha rhythm frequency and intelligence in normal adults. Intelligence 23, 1–14. doi: 10.1016/S0160-2896(96)80002-X
Arns, M., Bruder, G., Hegerl, U., Spooner, C., Palmer, D. M., Etkin, A., et al. (2016). EEG alpha asymmetry as a gender-specific predictor of outcome to acute treatment with different antidepressant medications in the randomized iSPOT-D study. Clin. Neurophysiol. 127, 509–519. doi: 10.1016/j.clinph.2015.05.032
Arpaia, P., Moccaldi, N., Prevete, R., Sannino, I., and Tedesco, A. (2020). A wearable EEG instrument for real-time frontal asymmetry monitoring in worker stress analysis. IEEE Trans. Instr. Meas. 69, 8335–8343. doi: 10.1109/TIM.2020.2988744
Bares, M., Brunovsky, M., Kopecek, M., Novak, T., Stopkova, P., Kozeny, J., et al. (2008). Early reduction in prefrontal theta QEEG cordance value predicts response to venlafaxine treatment in patients with resistant depressive disorder. Eur. Psychiatry 23, 350–355. doi: 10.1016/j.eurpsy.2008.03.001
Baskaran, A., Milev, R., and McIntyre, R. S. (2012). The neurobiology of the EEG biomarker as a predictor of treatment response in depression. Neuropharmacology 63, 507–513. doi: 10.1016/j.neuropharm.2012.04.021
Bechara, A. (2005). Decision making, impulse control and loss of willpower to resist drugs: a neurocognitive perspective. Nat. Neurosci. 8, 1458–1463. doi: 10.1038/nn1584
Bell, I. R., Cunningham, V., Caspi, O., Meek, P., and Ferro, L. (2004). Development and validation of a new global well-being outcomes rating scale for integrative medicine research. BMC Complement. Altern. Med. 4:1. doi: 10.1186/1472-6882-4-1
Biondi, A., Laiou, P., Bruno, E., Viana, P. F., Schreuder, M., Hart, W., et al. (2021). Remote and long-term self-monitoring of electroencephalographic and noninvasive measurable variables at home in patients with epilepsy (EEG@HOME): protocol for an observational study. JMIR Res. Protoc. 10:e25309. doi: 10.2196/25309
Boggio, P. S., Sultani, N., Fecteau, S., Merabet, L., Mecca, T., Pascual-Leone, A., et al. (2008). Prefrontal cortex modulation using transcranial DC stimulation reduces alcohol craving: a double-blind, sham-controlled study. Drug Alcohol Depend. 92, 55–60. doi: 10.1016/j.drugalcdep.2007.06.011
Brandmeyer, T., and Delorme, A. (2020a). Closed-loop frontal midlineθ neurofeedback: a novel approach for training focused-attention meditation. Front. Hum. Neurosci. 14:246. doi: 10.3389/fnhum.2020.00246
Brandmeyer, T., and Delorme, A. (2020b). Meditation and the wandering mind: a theoretical account of underlying and converging neural mechanisms. Perspect. Psychol. Sci. 16, 39–66.
Buzsáki, G. (2006). Rhythms of the Brain. Oxford: . Oxford University Press, doi: 10.1093/acprof:oso/9780195301069.001.0001
Cacioppo, J. T., Tassinary, L. G., and Berntson, G. (2007). Handbook of Psychophysiology. Cambridge: Cambridge University Press.
Cannard, C., Brandmeyer, T., Wahbeh, H., and Delorme, A. (2020). Self-health monitoring and wearable neurotechnologies. Handb. Clin. Neurol. 168, 207–232. doi: 10.1016/B978-0-444-63934-9.00016-0
Cao, Z., Lin, C.-T., Ding, W., Chen, M.-H., Li, C.-T., and Su, T.-P. (2019). Identifying ketamine responses in treatment-resistant depression using a wearable forehead EEG. IEEE Trans. Biomed. Eng. 66, 1668–1679. doi: 10.1109/TBME.2018.2877651
Carmody, J., and Baer, R. A. (2008). Relationships between mindfulness practice and levels of mindfulness, medical and psychological symptoms and well-being in a mindfulness-based stress reduction program. J. Behav. Med. 31, 23–33. doi: 10.1002/jclp.20555
Carrier, J., Land, S., Buysse, D. J., Kupfer, D. J., and Monk, T. H. (2001). The effects of age and gender on sleep EEG power spectral density in the middle years of life (ages 20-60 years old). Psychophysiology 38, 232–242.
Carstensen, L. L., Turan, B., Scheibe, S., Ram, N., Ersner-Hershfield, H., Samanez-Larkin, G. R., et al. (2011). Emotional experience improves with age: evidence based on over 10 years of experience sampling. Psychol. Aging 26, 21–33. doi: 10.1037/a0021285
Cartocci, G., Modica, E., Rossi, D., Cherubino, P., Maglione, A. G., Colosimo, A., et al. (2018). Neurophysiological measures of the perception of antismoking public service announcements among young population. Front. Hum. Neurosci. 12:231. doi: 10.3389/fnhum.2018.00231
Cartocci, G., Rossi, D., Modica, E., Maglione, A. G., Martinez Levy, A. C., Cherubino, P., et al. (2021). NeuroDante: poetry mentally engages more experts but moves more non-experts, and for both the cerebral approach tendency goes hand in hand with the cerebral effort. Brain Sci. 11:281. doi: 10.3390/brainsci11030281
Cheung, M.-C., Law, D., Yip, J., and Wong, C. W. Y. (2019). Emotional responses to visual art and commercial stimuli: implications for creativity and aesthetics. Front. Psychol. 10:14. doi: 10.3389/fpsyg.2019.00014
Chiang, A. K. I., Rennie, C. J., Robinson, P. A., Roberts, J. A., Rigozzi, M. K., Whitehouse, R. W., et al. (2008). Automated characterization of multiple alpha peaks in multi-site electroencephalograms. J. Neurosci. Methods 168, 396–411. doi: 10.1016/j.jneumeth.2007.11.001
Chiang, A. K. I., Rennie, C. J., Robinson, P. A., van Albada, S. J., and Kerr, C. C. (2011). Age trends and sex differences of alpha rhythms including split alpha peaks. Clin. Neurophysiol. 122, 1505–1517. doi: 10.1016/j.clinph.2011.01.040
Cisler, J. M., and Koster, E. H. W. (2010). Mechanisms of attentional biases towards threat in anxiety disorders: an integrative review. Clin. Psychol. Rev. 30, 203–216. doi: 10.1016/j.cpr.2009.11.003
Coan, J. A., Allen, J. J. B., and McKnight, P. E. (2006). A capability model of individual differences in frontal EEG asymmetry. Biol. Psychol. 72, 198–207. doi: 10.1016/j.biopsycho.2005.10.003
Coan, J. A., Allen, J. J., and Harmon-Jones, E. (2001). Voluntary facial expression and hemispheric asymmetry over the frontal cortex. Psychophysiology 38, 912–925. doi: 10.1111/1469-8986.3860912
Coan, J. A., and Allen, J. J. (2004). Frontal EEG asymmetry as a moderator and mediator of emotion. Biol. Psychol. 67, 7–49. doi: 10.1016/j.biopsycho.2004.03.002
Coan, J. A., and Allen, J. J. B. (2003). “The state and trait nature of frontal EEG asymmetry in emotion,” in The Asymmetrical Brain, eds K. Hugdahl and R. J. Davidson (Cambridge, MA: MIT Press), 565–615. doi: 10.1017/S0954579420001558
Cook, I. A., O’Hara, R., Uijtdehaage, S. H. J., Mandelkern, M., and Leuchter, A. F. (1998). Assessing the accuracy of topographic EEG mapping for determining local brain function. Electroencephalogr. Clin. Neurophysiol. 107, 408–414. doi: 10.1016/S0013-4694(98)00092-3
Cook, I., and Leuchter, A. (2001). Prefrontal changes and treatment response prediction in depression. Semin. Clin. Neuropsychiatry 6, 113–120. doi: 10.1053/scnp.2001.21844
Cook, I., Leuchter, A., Morgan, M., Witte, E., Stubbeman, W., Abrams, M., et al. (2002). Early changes in prefrontal activity characterize clinical responders to antidepressants. Neuropsychopharmacology 27, 120–131. doi: 10.1016/S0893-133X(02)00294-4
Corbetta, M., and Shulman, G. L. (2002). Control of goal-directed and stimulus-driven attention in the brain. Nat. Rev. Neurosci. 3, 201–215. doi: 10.1038/nrn755
Corbetta, M., Patel, G., and Shulman, G. L. (2008). The reorienting system of the human brain: from environment to theory of mind. Neuron 58, 306–324. doi: 10.1016/j.neuron.2008.04.017
Corcoran, A. W., Alday, P. M., Schlesewsky, M., and Bornkessel-Schlesewsky, I. (2017). Towards a reliable, automated method of individual alpha frequency (IAF) quantification. Psychophysiology 55:e13064. doi: 10.1101/176792
Cronbach, L. J. (1951). Coefficient alpha and the internal structure of tests. Psychometrika 16, 297–334. doi: 10.1007/BF02310555
da Silva Castanheira, J., Orozco Perez, H. D., Misic, B., and Baillet, S. (2021). Brief segments of neurophysiological activity enable individual differentiation. Nat. Commun. 12:5713. doi: 10.1038/s41467-021-25895-8
Davidson, R. J. (1988). EEG measures of cerebral asymmetry: conceptual and methodological issues. Int. J. Neurosci. 39, 71–89. doi: 10.3109/00207458808985694
Davidson, R. J. (1992). Anterior cerebral asymmetry and the nature of emotion. Brain Cogn. 20, 125–151. doi: 10.1016/0278-2626(92)90065-T
Davidson, R. J. (1994). Asymmetric brain function, affective style, and psychopathology: the role of early experience and plasticity. Dev. Psychopathol. 6, 741–758. doi: 10.1017/S0954579400004764
Davidson, R. J., Chapman, J. P., Chapman, L. J., and Henriques, J. B. (1990). Asymmetrical brain electrical activity discriminates between psychometrically-matched verbal and spatial cognitive tasks. Psychophysiology 27, 528–543. doi: 10.1111/j.1469-8986.1990.tb01970.x
de Hemptinne, C., Chen, W., Racine, C. A., Seritan, A. L., Miller, A. M., Yaroshinsky, M. S., et al. (2021). Prefrontal physiomarkers of anxiety and depression in Parkinson’s disease. Front. Neurosci. 15:1337. doi: 10.3389/fnins.2021.748165
De Raedt, R., and Koster, E. H. W. (2010). Understanding vulnerability for depression from a cognitive neuroscience perspective: a reappraisal of attentional factors and a new conceptual framework. Cogn. Affect. Behav. Neurosci. 10, 50–70. doi: 10.3758/CABN.10.1.50
Delorme, A., and Makeig, S. (2004). EEGLAB: an open source toolbox for analysis of single-trial EEG dynamics. J. Neurosci. Methods 134, 9–21. doi: 10.1016/j.jneumeth.2003.10.009
Dennis, T. A., and Solomon, B. (2010). Frontal EEG and emotion regulation: electrocortical activity in response to emotional film clips is associated with reduced mood induction and attention interference effects. Biol. Psychol. 85, 456–464. doi: 10.1016/j.biopsycho.2010.09.008
Di Flumeri, G., Herrero, M. T., Trettel, A., Cherubino, P., Maglione, A. G., Colosimo, A., et al. (2016). “EEG Frontal asymmetry related to pleasantness of olfactory stimuli in young subjects,” in Selected Issues in Experimental Economics, eds K. Nermend and M. Łatuszyńska (New York, NY: Springer International Publishing), 373–381. doi: 10.1007/978-3-319-28419-4_23
Díaz, H., Cid, F. M., Otárola, J., Rojas, R., Alarcón, O., and Cañete, L. (2019). EEG Beta band frequency domain evaluation for assessing stress and anxiety in resting, eyes closed, basal conditions. Proc. Comput. Sci. 162, 974–981. doi: 10.1016/j.procs.2019.12.075
Dodge, R., Daly, A. P., Huyton, J., and Sanders, L. D. (2012). The challenge of defining wellbeing. Int. J. Wellbeing 2:3.
Dolcos, S., Moore, M., and Katsumi, Y. (2018). “Neuroscience and well-being,” in Handbook of Well-being, eds E. Diener, S. Oishi, and L. Tay (Salt Lake City, UT: DEF Publishers), 26.
Doll, A., Hölzel, B. K., Mulej Bratec, S., Boucard, C. C., Xie, X., Wohlschläger, A. M., et al. (2016). Mindful attention to breath regulates emotions via increased amygdala–prefrontal cortex connectivity. NeuroImage 134, 305–313. doi: 10.1016/j.neuroimage.2016.03.041
Doppelmayr, M., Klimesch, W., Pachinger, T., and Ripper, B. (1998). Individual differences in brain dynamics: important implications for the calculation of event-related band power. Biol. Cybernet. 79, 49–57. doi: 10.1007/s004220050457
Doricchi, F., Macci, E., Silvetti, M., and Macaluso, E. (2010). Neural correlates of the spatial and expectancy components of endogenous and stimulus-driven orienting of attention in the posner task. Cereb. Cortex 20, 1574–1585. doi: 10.1093/cercor/bhp215
Engels, A. S., Heller, W., Mohanty, A., Herrington, J. D., Banich, M. T., Webb, A. G., et al. (2007). Specificity of regional brain activity in anxiety types during emotion processing. Psychophysiology 44, 352–363. doi: 10.1111/j.1469-8986.2007.00518.x
Engels, A. S., Heller, W., Spielberg, J. M., Warren, S. L., Sutton, B. P., Banich, M. T., et al. (2010). Co-occurring anxiety influences patterns of brain activity in depression. Cogn. Affect. Behav. Neurosci. 10, 141–156. doi: 10.3758/CABN.10.1.141
Eysenck, M. W., Derakshan, N., Santos, R., and Calvo, M. G. (2007). Anxiety and cognitive performance: attentional control theory. Emotion (Washington, D.C.) 7, 336–353. doi: 10.1037/1528-3542.7.2.336
Fecteau, S., Knoch, D., Fregni, F., Sultani, N., Boggio, P., and Pascual-Leone, A. (2007). Diminishing risk-taking behavior by modulating activity in the prefrontal cortex: a direct current stimulation study. J. Neurosci. 27, 12500–12505. doi: 10.1523/JNEUROSCI.3283-07.2007
Finley, A., Angus, D., van Reekum, C., Davidson, R., and Schaefer, S. (2020). Age, theta/beta ratios, and individual peak alpha frequency in older adults: virtual annual meeting of the society-for-psychophysiological-research (SPR). Psychophysiology 57:S58. doi: 10.1111/psyp.13670
Fox, N. A., Rubin, K. H., Calkins, S. D., Marshall, T. R., Coplan, R. J., Porges, S. W., et al. (1995). Frontal activation asymmetry and social competence at four years of age. Child Dev. 66, 1770–1784. doi: 10.1111/j.1467-8624.1995.tb00964.x
Fregni, F., Orsati, F., Pedrosa, W., Fecteau, S., Tome, F. A. M., Nitsche, M. A., et al. (2008). Transcranial direct current stimulation of the prefrontal cortex modulates the desire for specific foods. Appetite 51, 34–41. doi: 10.1016/j.appet.2007.09.016
Gable, P. A., Mechin, N. C., Hicks, J. A., and Adams, D. L. (2015). Supervisory control system and frontal asymmetry: neurophysiological traits of emotion-based impulsivity. Soc. Cogn. Affect. Neurosci. 10, 1310–1315. doi: 10.1093/scan/nsv017
Gale, A., Edwards, J., Morris, P., Moore, R., and Forrester, D. (2001). Extraversion–introversion, neuroticism–stability, and EEG indicators of positive and negative empathic mood. Pers. Individ. Differ. 30, 449–461. doi: 10.1016/S0191-8869(00)00036-2
Garavan, H., and Hester, R. (2007). The role of cognitive control in cocaine dependence. Neuropsychol. Rev. 17, 337–345. doi: 10.1007/s11065-007-9034-x
Gasbarri, A., Arnone, B., Pompili, A., Marchetti, A., Pacitti, F., Calil, S. S., et al. (2006). Sex-related lateralized effect of emotional content on declarative memory: an event related potential study. Behav. Brain Res. 168, 177–184. doi: 10.1016/j.bbr.2005.07.034
Gasbarri, A., Arnone, B., Pompili, A., Pacitti, F., Pacitti, C., and Cahill, L. (2007). Sex-related hemispheric lateralization of electrical potentials evoked by arousing negative stimuli. Brain Res. 1138, 178–186. doi: 10.1016/j.brainres.2006.12.073
Gevins, A., Smith, M. E., McEvoy, L., and Yu, D. (1997). High-resolution EEG mapping of cortical activation related to working memory: effects of task difficulty, type of processing, and practice. Cereb. Cortex 7, 374–385. doi: 10.1093/cercor/7.4.374
Goldstein, R. Z., and Volkow, N. D. (2011). Dysfunction of the prefrontal cortex in addiction: neuroimaging findings and clinical implications. Nat. Rev. Neurosci. 12, 652–669. doi: 10.1038/nrn3119
Goljahani, A., Bisiacchi, P., and Sparacino, G. (2014). An EEGLAB plugin to analyze individual EEG alpha rhythms using the “channel reactivity-based method”. Comput. Methods Progr. Biomed. 113, 853–861. doi: 10.1016/j.cmpb.2013.12.010
Goljahani, A., D’Avanzo, C., Schiff, S., Amodio, P., Bisiacchi, P., and Sparacino, G. (2012). A novel method for the determination of the EEG individual alpha frequency. NeuroImage 60, 774–786. doi: 10.1016/j.neuroimage.2011.12.001
Gotlib, I. H. (1998). EEG alpha asymmetry, depression, and cognitive functioning. Cogn. Emot. 12, 449–478. doi: 10.1080/026999398379673
Gotlib, I. H., and Joormann, J. (2010). Cognition and depression: current status and future directions. Annu. Rev. Clin. Psychol. 6, 285–312. doi: 10.1146/annurev.clinpsy.121208.131305
Grandy, T. H., Werkle-Bergner, M., Chicherio, C., Schmiedek, F., Lövdén, M., and Lindenberger, U. (2013). Peak individual alpha frequency qualifies as a stable neurophysiological trait marker in healthy younger and older adults: alpha stability. Psychophysiology 50, 570–582. doi: 10.1111/psyp.12043
Grimshaw, G. M., and Carmel, D. (2014). An asymmetric inhibition model of hemispheric differences in emotional processing. Front. Psychol. 5:489. doi: 10.3389/fpsyg.2014.00489
Haegens, S., Cousijn, H., Wallis, G., Harrison, P. J., and Nobre, A. C. (2014). Inter- and intra-individual variability in alpha peak frequency. NeuroImage 92, 46–55. doi: 10.1016/j.neuroimage.2014.01.049
Hagemann, D., Hewig, J., Walter, C., and Naumann, E. (2008). Skull thickness and magnitude of EEG alpha activity. Clin. Neurophysiol. 119, 1271–1280. doi: 10.1016/j.clinph.2008.02.010
Hagemann, D., Naumann, E., Lürken, A., Becker, G., Maier, S., and Bartussek, D. (1999). EEG asymmetry, dispositional mood and personality. Pers. Individ. Differ. 27, 541–568. doi: 10.1016/S0191-8869(98)00263-3
Hagemann, D., Naumann, E., Thayer, J. F., and Bartussek, D. (2002). Does resting electroencephalograph asymmetry reflect a trait? An application of latent state-trait theory. J. Pers. Soc. Psychol. 82, 619–641. doi: 10.1037/0022-3514.82.4.619
Hamid, N. H. A., Sulaiman, N., Aris, S. A. M., Murat, Z. H., and Taib, M. N. (2010). “Evaluation of human stress using EEG Power Spectrum,” in Proceedings of the 2010 6th International Colloquium on Signal Processing its Applications, Malacca, 1–4. doi: 10.1109/CSPA.2010.5545282
Hanslmayr, S., Gross, J., Klimesch, W., and Shapiro, K. L. (2011). The role of alpha oscillations in temporal attention. Brain Res. Rev. 67, 331–343. doi: 10.1016/j.brainresrev.2011.04.002
Harmon-Jones, E. (2004). Contributions from research on anger and cognitive dissonance to understanding the motivational functions of asymmetrical frontal brain activity. Biol. Psychol. 67, 51–76. doi: 10.1016/j.biopsycho.2004.03.003
Harmon-Jones, E., and Sigelman, J. (2001). State anger and prefrontal brain activity: evidence that insult-related relative left-prefrontal activation is associated with experienced anger and aggression. J. Pers. Soc. Psychol. 80, 797–803. doi: 10.1037/0022-3514.80.5.797
Harmon-Jones, E., Gable, P. A., and Peterson, C. K. (2010). The role of asymmetric frontal cortical activity in emotion-related phenomena: a review and update. Biol. Psychol. 84, 451–462. doi: 10.1016/j.biopsycho.2009.08.010
Hashemi, A., Pino, L. J., Moffat, G., Mathewson, K. J., Aimone, C., Bennett, P. J., et al. (2016). Characterizing population EEG dynamics throughout adulthood. ENeuro 3:ENEURO.275-ENEURO.216. doi: 10.1523/ENEURO.0275-16.2016
Hassmén, P., Koivula, N., and Uutela, A. (2000). Physical exercise and psychological well-being: a population study in finland. Prevent. Med. 30, 17–25. doi: 10.1006/pmed.1999.0597
Hayashi, T., Okamoto, E., Nishimura, H., Mizuno-Matsumoto, Y., Ishii, R., and Ukai, S. (2009). Beta activities in EEG associated with emotional stress. Int. J. Intell. Comput. Med. Sci. Image Proces. 3, 57–68. doi: 10.1080/1931308X.2009.10644171
Heller, W., Nitschke, J. B., Etienne, M. A., and Miller, G. A. (1997). Patterns of regional brain activity differentiate types of anxiety. J. Abnorm. Psychol. 106, 376–385. doi: 10.1037//0021-843x.106.3.376
Howell, A. J., Dopko, R. L., Passmore, H.-A., and Buro, K. (2011). Nature connectedness: associations with well-being and mindfulness. Pers. Individ. Differ. 51, 166–171. doi: 10.1016/j.paid.2011.03.037
Hu, B., Peng, H., Zhao, Q., Hu, B., Majoe, D., Zheng, F., et al. (2015). Signal quality assessment model for wearable EEG sensor on prediction of mental stress. IEEE Trans. NanoBiosci. 14, 553–561. doi: 10.1109/TNB.2015.2420576
Hwang, S., Jebelli, H., Choi, B., Choi, M., and Lee, S. (2018). Measuring workers’ emotional state during construction tasks using wearable EEG. J. Construct. Eng. Manag. 144:04018050. doi: 10.1061/(ASCE)CO.1943-7862.0001506
Iosifescu, D., Greenwald, S., Devlin, P., Mischoulon, D., Denninger, J., Alpert, J., et al. (2009). Frontal EEG predictors of treatment outcome in major depressive disorder. Eur. Neuropsychopharmacol. 19, 772–777. doi: 10.1016/j.euroneuro.2009.06.001
Jackson, D. C., Mueller, C. J., Dolski, I., Dalton, K. M., Nitschke, J. B., Urry, H. L., et al. (2003). Now you feel it, now you don’t: frontal brain electrical asymmetry and individual differences in emotion regulation. Psychol. Sci. 14, 612–617. doi: 10.1046/j.0956-7976.2003.psci_1473.x
Jacobs, G. D., and Snyder, D. (1996). Frontal brain asymmetry predicts affective style in men. Behav. Neurosci. 110, 3–6. doi: 10.1037//0735-7044.110.1.3
Jebelli, H., Hwang, S., and Lee, S. (2017). “Feasibility of field measurement of construction workers’ valence using a wearable EEG device,” in Proceedings of the 2017 ASCE International Workshop on Computing in Civil Engineering, IWCCE, Seattle, 99–106. doi: 10.1061/9780784480830.013
Jebelli, H., Khalili, M. M., Hwang, S., and Lee, S. (2018). A supervised learning-based construction workers’ stress recognition using a wearable electroencephalography (EEG) Device. Construct. Res. Congr. 2018, 40–50. doi: 10.1061/9780784481288.005
Jena, S. (2015). Examination stress and its effect on EEG. Int. J. Med. Sci. Public Health 4:1493. doi: 10.5455/ijmsph.2015.23042015308
Jun, G., and Smitha, K. G. (2016). “EEG based stress level identification,” in Proceedings of the 2016 IEEE International Conference on Systems, Man, and Cybernetics (SMC), 003270–003274, Budapest. doi: 10.1109/SMC.2016.7844738
Kalu, U. G., Sexton, C. E., Loo, C. K., and Ebmeier, K. P. (2012). Transcranial direct current stimulation in the treatment of major depression: a meta-analysis. Psychol. Med. 42, 1791–1800. doi: 10.1017/S0033291711003059
Kazdin, A. E. (2007). Mediators and mechanisms of change in psychotherapy research. Annu. Rev. Clin. Psychol. 3, 1–27. doi: 10.1146/annurev.clinpsy.3.022806.091432
Keyes, C. L. M., and Waterman, M. B. (2003). “Dimensions of well-being and mental health in adulthood,” in Well-Being: Positive Development Across the Life Course, eds M. H. Bornstein, L. Davidson, C. L. M. Keyes, and K. A. Moore (Mahwah, NJ: Lawrence Erlbaum Associates Publishers), 477–497.
Keyes, C. L. M., Myers, J. M., and Kendler, K. S. (2010). The structure of the genetic and environmental influences on mental well-being. Am. J. Public Health 100, 2379–2384. doi: 10.2105/AJPH.2010.193615
Kim, J., Chae, J. H., Ko, H. K., Latchoumane, C. F., Banerjee, A., Mandell, D. J., et al. (2012). Hemispheric asymmetry in non-linear interdependence of EEG in post-traumatic stress disorder. Psychiatry Clin. Neurosci. 66, 87–96. doi: 10.1111/j.1440-1819.2011.02300.x
Klimesch, W. (1997). EEG-alpha rhythms and memory processes. Int. J. Psychophysiol. 26, 319–340. doi: 10.1016/s0167-8760(97)00773-3
Klimesch, W. (1999). EEG alpha and theta oscillations reflect cognitive and memory performance: a review and analysis. Brain Res. 29, 169–195. doi: 10.1016/s0165-0173(98)00056-3
Klimesch, W., Doppelmayr, M., Russegger, H., Pachinger, T., and Schwaiger, J. (1998). Induced alpha band power changes in the human EEG and attention. Neurosci. Lett. 244, 73–76. doi: 10.1016/S0304-3940(98)00122-0
Klimesch, W., Schimke, H., Ladurner, G., and Pfurtscheller, G. (1990). Alpha frequency and memory performance. J. Psychophysiol. 4, 381–390.
Knott, V., Mohr, E., Mahoney, C., and Ilivitsky, V. (2000). Electroencephalographic coherence in Alzheimer’s disease: comparisons with a control group and population norms. J. Geriatr. Psychiatry Neurol. 13, 1–8. doi: 10.1177/089198870001300101
Knott, V., Telner, J., Lapierre, Y., Browne, M., and Horn, E. (1996). Quantitative EEG in the prediction of antidepressant response to imipramine. J. Affect. Disord. 39, 175–184. doi: 10.1016/0165-0327(96)00003-1
Kop, W. J., Synowski, S. J., Newell, M. E., Schmidt, L. A., Waldstein, S. R., and Fox, N. A. (2011). Autonomic nervous system reactivity to positive and negative mood induction: the role of acute psychological responses and frontal electrocortical activity. Biol. Psychol. 86, 230–238. doi: 10.1016/j.biopsycho.2010.12.003
Koslov, K., Mendes, W. B., Pajtas, P. E., and Pizzagalli, D. A. (2011). Greater left resting intracortical activity as a buffer to social threat. Psychol. Sci. 22, 641–649. doi: 10.1177/0956797611403156
Kovacevic, N., Ritter, P., Tays, W., Moreno, S., and McIntosh, A. R. (2015). ‘My Virtual Dream’: collective neurofeedback in an immersive art environment. PLoS One 10:e0130129. doi: 10.1371/journal.pone.0130129
Krigolson, O. E., Williams, C. C., Norton, A., Hassall, C. D., and Colino, F. L. (2017). Choosing MUSE: validation of a low-cost, portable EEG system for ERP research. Front. Neurosci. 11:109. doi: 10.3389/fnins.2017.00109
Laufs, H., Holt, J. L., Elfont, R., Krams, M., Paul, J. S., Krakow, K., et al. (2006). Where the BOLD signal goes when alpha EEG leaves. Neuroimage 31, 1408–1418. doi: 10.1016/j.neuroimage.2006.02.002
Laufs, H., Kleinschmidt, A., Beyerle, A., Eger, E., Salek-Haddadi, A., Preibisch, C., et al. (2003). EEG-correlated fMRI of human alpha activity. NeuroImage 19, 1463–1476. doi: 10.1016/S1053-8119(03)00286-6
Linden, D. E. J. (2014). Neurofeedback and networks of depression. Dialog. Clin. Neurosci. 16, 103–112. doi: 10.31887/DCNS.2014.16.1/dlinden
Lodder, S. S., and van Putten, M. J. A. M. (2011). Automated EEG analysis: characterizing the posterior dominant rhythm. J. Neurosci. Methods 200, 86–93. doi: 10.1016/j.jneumeth.2011.06.008
Lodder, S. S., and van Putten, M. J. A. M. (2013). Quantification of the adult EEG background pattern. Clin. Neurophysiol. 124, 228–237. doi: 10.1016/j.clinph.2012.07.007
Lucas, R. E., and Diener, E. (2008). “Subjective well-being,” in Handbook of Emotions, 3rd Edn, ed. E. Diener (New York, NY: The Guilford Press), 471–484.
Luhmann, M., Schimmack, U., and Eid, M. (2011). Stability and variability in the relationship between subjective well-being and income. J. Res. Pers. 45, 186–197. doi: 10.1016/j.jrp.2011.01.004
MacKinnon, D. P., Lockhart, G., Baraldi, A. N., and Gelfand, L. A. (2013). “Evaluating treatment mediators and moderators,” in The Oxford Handbook of Research Strategies for Clinical Psychology, eds J. S. Comer and P. C. Kendall (Oxford: Oxford University Press), 262–286.
Mahmoodi, M., Makkiabadi, B., Mahmoudi, M., and Sanei, S. (2021). A new method for accurate detection of movement intention from single channel EEG for online BCI. Comput. Methods Progr. Biomed. Update 1:100027. doi: 10.1016/j.cmpbup.2021.100027
Makeig, S., Bell, A. J., Jung, T.-P., and Sejnowski, T. J. (1996). “Independent component analysis of electroencephalographic data,” in Advances in Neural Information Processing Systems, Vol. 8, eds D. Touretzky, M. Mozer, and M. Hasselmo (Boston, MA: Springer), 145–151.
Mantini, D., Perrucci, M. G., Del Gratta, C., Romani, G. L., and Corbetta, M. (2007). Electrophysiological signatures of resting state networks in the human brain. Proc. Natl. Acad. Sci. U.S.A. 104, 13170–13175. doi: 10.1073/pnas.0700668104
Mason, M. F., Brown, K., Mar, R. A., and Smallwood, J. (2013). Driver of discontent or escape vehicle: the affective consequences of mindwandering. Front. Psychol. 4:477. doi: 10.3389/fpsyg.2013.00477
Mathersul, D., Williams, L. M., Hopkinson, P. J., and Kemp, A. H. (2008). Investigating models of affect: relationships among EEG alpha asymmetry, depression, and anxiety. Emotion (Washington, D.C.) 8, 560–572. doi: 10.1037/a0012811
Mathewson, K. E., Lleras, A., Beck, D. M., Fabiani, M., Ro, T., and Gratton, G. (2011). Pulsed Out of Awareness: EEG alpha oscillations represent a pulsed-inhibition of ongoing cortical processing. Front. Psychol. 2:99. doi: 10.3389/fpsyg.2011.00099
Meyer, T., Smeets, T., Giesbrecht, T., Quaedflieg, C. W. E. M., Smulders, F. T. Y., Meijer, E. H., et al. (2015). The role of frontal EEG asymmetry in post-traumatic stress disorder. Biol. Psychol. 108, 62–77. doi: 10.1016/j.biopsycho.2015.03.018
Mierau, A., Klimesch, W., and Lefebvre, J. (2017). State-dependent alpha peak frequency shifts: experimental evidence, potential mechanisms and functional implications. Neuroscience 360, 146–154. doi: 10.1016/j.neuroscience.2017.07.037
Mikolajczak, M., Bodarwé, K., Laloyaux, O., Hansenne, M., and Nelis, D. (2010). Association between frontal EEG asymmetries and emotional intelligence among adults. Pers. Individ. Differ. 48, 177–181. doi: 10.1016/j.paid.2009.10.001
Miller, A., Fox, N. A., Cohn, J. F., Forbes, E. E., Sherrill, J. T., and Kovacs, M. (2002). Regional patterns of brain activity in adults with a history of childhood-onset depression: gender differences and clinical variability. Am. J. Psychiatry 159, 934–940. doi: 10.1176/appi.ajp.159.6.934
Morgan, M. L., Witte, E. A., Cook, I. A., Leuchter, A. F., Abrams, M., and Siegman, B. (2005). Influence of age, gender, health status, and depression on quantitative EEG. Neuropsychobiology 52, 71–76. doi: 10.1159/000086608
Moser, D. J., Jorge, R. E., Manes, F., Paradiso, S., Benjamin, M. L., and Robinson, R. G. (2002). Improved executive functioning following repetitive transcranial magnetic stimulation. Neurology 58, 1288–1290. doi: 10.1212/wnl.58.8.1288
Moynihan, J. A., Chapman, B. P., Klorman, R., Krasner, M. S., Duberstein, P. R., Brown, K. W., et al. (2013). Mindfulness-based stress reduction for older adults: effects on executive function, frontal alpha asymmetry and immune function. Neuropsychobiology 68, 34–43. doi: 10.1159/000350949
Müller, B. C., Kühn-Popp, N., Meinhardt, J., Sodian, B., and Paulus, M. (2015). Long-term stability in children’s frontal EEG alpha asymmetry between 14-months and 83-months. Int. J. Dev. Neurosci. 41, 110–114. doi: 10.1016/j.ijdevneu.2015.01.002
Müller, M. M., Keil, A., Gruber, T., and Elbert, T. (1999). Processing of affective pictures modulates right-hemispheric gamma band EEG activity. Clin. Neurophysiol. 110, 1913–1920. doi: 10.1016/S1388-2457(99)00151-0
Näpflin, M., Wildi, M., and Sarnthein, J. (2007). Test–retest reliability of resting EEG spectra validates a statistical signature of persons. Clin. Neurophysiol. 118, 2519–2524. doi: 10.1016/j.clinph.2007.07.022
Nunez, P. L., and Srinivasan, R. (2006). Electric Fields of the Brain: The Neurophysics of EEG, 2nd Edn. Oxford: Oxford University Press. doi: 10.1093/acprof:oso/9780195050387.001.0001
Nunez, P. L., Wingeier, B. M., and Silberstein, R. B. (2001). Spatial-temporal structures of human alpha rhythms: theory, microcurrent sources, multiscale measurements, and global binding of local networks. Hum. Brain Mapp. 13, 125–164. doi: 10.1002/hbm.1030
Nusslock, R., Shackman, A. J., Harmon-Jones, E., Alloy, L. B., Coan, J. A., and Abramson, L. Y. (2011). Cognitive vulnerability and frontal brain asymmetry: common predictors of first prospective depressive episode. J. Abnorm. Psychol. 120, 497–503. doi: 10.1037/a0022940
Oakes, T. R. (2004). Functional coupling of simultaneous electrical and metabolic activity in the human brain. Hum. Brain Mapp. 21, 257–270. doi: 10.1002/hbm.20004
Olbrich, S., and Arns, M. (2013). EEG biomarkers in major depressive disorder: discriminative power and prediction of treatment response. Int. Rev. Psychiatry 25, 604–618. doi: 10.3109/09540261.2013.816269
Otero, G. A., Pliego-Rivero, F. B., Fernández, T., and Ricardo, J. (2003). EEG development in children with sociocultural disadvantages: a follow-up study. Clin. Neurophysiol. 114, 1918–1925. doi: 10.1016/s1388-2457(03)00173-1
Otto, L. M., Howerter, A., Bell, I. R., and Jackson, N. (2010). Exploring measures of whole person wellness: integrative well-being and psychological flourishing. EXPLORE 6, 364–370. doi: 10.1016/j.explore.2010.08.001
Palmiero, M., and Piccardi, L. (2017). Frontal EEG asymmetry of mood: a mini-review. Front. Behav. Neurosci. 11:224. doi: 10.3389/fnbeh.2017.00224
Papousek, I., and Schulter, G. (2002). Covariations of EEG asymmetries and emotional states indicate that activity at frontopolar locations is particularly affected by state factors. Psychophysiology 39, 350–360. doi: 10.1017/S0048577201393083
Papousek, I., Reiser, E. M., Weber, B., Freudenthaler, H. H., and Schulter, G. (2012). Frontal brain asymmetry and affective flexibility in an emotional contagion paradigm. Psychophysiology 49, 489–498. doi: 10.1111/j.1469-8986.2011.01324.x
Park, S., Han, C.-H., and Im, C.-H. (2020). Design of wearable EEG devices specialized for passive brain–computer interface applications. Sensors 20:4572. doi: 10.3390/s20164572
Peng, H., Majoe, D., and Kaegi-Trachsel, T. (2011). “Design and application of a novel wearable EEG system for e-healthcare,” in Proceedings of the 2011 International Workshop on Ubiquitous Affective Awareness and Intelligent Interaction, Zurich, 1–8. doi: 10.1145/2030092.2030094
Pizzagalli, D. A., Sherwood, R. J., Henriques, J. B., and Davidson, R. J. (2005). Frontal brain asymmetry and reward responsiveness: a source-localization study. Psychol. Sci. 16, 805–813. doi: 10.1111/j.1467-9280.2005.01618.x
Posthuma, D., Neale, M. C., Boomsma, D. I., and de Geus, E. J. (2001). Are smarter brains running faster? Heritability of alpha peak frequency, IQ, and their interrelation. Behav. Genet. 31, 567–579. doi: 10.1023/a:1013345411774
Prpa, M., Stepanova, E. R., Schiphorst, T., Riecke, B. E., and Pasquier, P. (2020). “Inhaling and exhaling: how technologies can perceptually extend our breath awareness,” in Proceedings of the 2020 CHI Conference on Human Factors in Computing Systems, Vancouver, BC, 1–15. doi: 10.1145/3313831.3376183
Quaedflieg, C. W., Meyer, T., Smulders, F. T. Y., and Smeets, T. (2015). The functional role of individual-alpha based frontal asymmetry in stress responding. Biol. Psychol. 104, 75–81. doi: 10.1016/j.biopsycho.2014.11.014
Quaedflieg, C. W., Smulders, F. T., Meyer, T., Peeters, F., Merckelbach, H., and Smeets, T. (2016). The validity of individual frontal alpha asymmetry EEG neurofeedback. Soc. Cogn. Affect. Neurosci. 11, 33–43. doi: 10.1093/scan/nsv090
Quinn, C. R., Rennie, C. J., Harris, A. W. F., and Kemp, A. H. (2014). The impact of melancholia versus non-melancholia on resting-state, EEG alpha asymmetry: electrophysiological evidence for depression heterogeneity. Psychiatry Res. 215, 614–617. doi: 10.1016/j.psychres.2013.12.049
Ramsøy, T. Z., Skov, M., Christensen, M. K., and Stahlhut, C. (2018). Frontal brain asymmetry and willingness to pay. Front. Neurosci. 12:138. doi: 10.3389/fnins.2018.00138
Rathee, S., Bhatia, D., Punia, V., and Singh, R. (2020). Peak alpha frequency in relation to cognitive performance. J. Neurosci. Rural Pract. 11, 416–419. doi: 10.1055/s-0040-1712585
Reid, S. A., Duke, L. M., and Allen, J. J. B. (1998). Resting frontal electroencephalographic asymmetry in depression: inconsistencies suggest the need to identify mediating factors. Psychophysiology 35, 389–404. doi: 10.1111/1469-8986.3540389
Robinson, R. G., and Price, T. R. (1982). Post-stroke depressive disorders: a follow-up study of 103 patients. Stroke 13, 635–641. doi: 10.1161/01.STR.13.5.635
Russell, R., Guerry, A., Balvanera, P., Gould, R., Basurto, X., Chan, K., et al. (2013). Humans and nature: how knowing and experiencing nature affect well-being. Annu. Rev. Environ. Resour. 38, 473–502. doi: 10.1146/annurev-environ-012312-110838
Ryan, R. M., Huta, V., and Deci, E. L. (2008). Living well: a self-determination theory perspective on eudaimonia. J. Happ. Stud. 9, 139–170. doi: 10.1007/s10902-006-9023-4
Ryff, C. D., and Singer, B. H. (2013). Know Thyself and Become What You are: A Eudaimonic Approach to Psychological Well-Being. New York, NY: Springer Science + Business Media, 116. doi: 10.1007/978-94-007-5702-8_6
Saeed, S. M. U., Anwar, S. M., Khalid, H., Majid, M., and Bagci, U. (2020). EEG based classification of long-term stress using psychological labeling. Sensors 20:1886. doi: 10.3390/s20071886
Sanguinetti, J. L., Hameroff, S., Smith, E. E., Sato, T., Daft, C. M. W., Tyler, W. J., et al. (2020). Transcranial focused ultrasound to the right prefrontal cortex improves mood and alters functional connectivity in humans. Front. Hum. Neurosci. 14:52. doi: 10.3389/fnhum.2020.00052
Santesso, D. L., Segalowitz, S. J., Ashbaugh, A. R., Antony, M. M., McCabe, R. E., and Schmidt, L. A. (2008). Frontal EEG asymmetry and sensation seeking in young adults. Biol. Psychol. 78, 164–172. doi: 10.1016/j.biopsycho.2008.02.003
Scheeringa, R., Petersson, K. M., Kleinschmidt, A., Jensen, O., and Bastiaansen, M. C. M. (2012). EEG alpha power modulation of fMRI resting-state connectivity. Brain Connect. 2, 254–264. doi: 10.1089/brain.2012.0088
Schmalzl, L., Powers, C., Zanesco, A. P., Yetz, N., Groessl, E. J., and Saron, C. D. (2018). The effect of movement-focused and breath-focused yoga practice on stress parameters and sustained attention: a randomized controlled pilot study. Conscious. Cogn. 65, 109–125. doi: 10.1016/j.concog.2018.07.012
Sessa, S. A. (2007). Meditation, breath work, and focus training for teachers and students—The five minutes a day that can really make a difference. J. Col. Teach. Learn. (TLC) 4, 10. doi: 10.19030/tlc.v4i10.1536
Shackman, A. J., McMenamin, B. W., Maxwell, J. S., Greischar, L. L., and Davidson, R. J. (2009). Right dorsolateral prefrontal cortical activity and behavioral inhibition. Psychol. Sci. 20, 1500–1506. doi: 10.1111/j.1467-9280.2009.02476.x
Smallwood, J., and Andrews-Hanna, J. (2013). Not all minds that wander are lost: the importance of a balanced perspective on the mind-wandering state. Front. Psychol. 4:441. doi: 10.3389/fpsyg.2013.00441
Smit, C. M., Wright, M. J., Hansell, N. K., Geffen, G. M., and Martin, N. G. (2006). Genetic variation of individual alpha frequency (IAF) and alpha power in a large adolescent twin sample. Int. J. Psychophysiol. 61, 235–243. doi: 10.1016/j.ijpsycho.2005.10.004
Smith, E. E., Cavanagh, J. F., and Allen, J. J. B. (2018). Intracranial source activity (eLORETA) related to scalp-level asymmetry scores and depression status. Psychophysiology 55:e13019. doi: 10.1111/psyp.13019
Smith, E. E., Reznik, S. J., Stewart, J. L., and Allen, J. J. B. (2017). Assessing and conceptualizing frontal EEG Asymmetry: an updated primer on recording, processing, analyzing, and interpreting frontal alpha asymmetry. Int. J. Psychophysiol. 111, 98–114. doi: 10.1016/j.ijpsycho.2016.11.005
Sowell, E. R., Peterson, B. S., Kan, E., Woods, R. P., Yoshii, J., Bansal, R., et al. (2007). Sex differences in cortical thickness mapped in 176 healthy individuals between 7 and 87 years of age. Cereb. Cortex 17, 1550–1560. doi: 10.1093/cercor/bhl066
Spronk, D., Arns, M., Barnett, K. J., Cooper, N., and Gordon, E. (2011). An investigation of EEG, genetic and cognitive markers of treatment response to antidepressant medication in patients with major depressive disorder: a pilot study. J. Affect. Disord. 128, 41–48. doi: 10.1016/j.jad.2010.06.021
Stewart, J. L., Bismark, A. W., Towers, D. N., Coan, J. A., and Allen, J. J. B. (2010). Resting frontal EEG asymmetry as an endophenotype for depression risk: sex-specific patterns of frontal brain asymmetry. J. Abnorm. Psychol. 119, 502–512. doi: 10.1037/a0019196
Sutton, S. K., and Davidson, R. J. (1997). Prefrontal brain asymmetry: a biological substrate of the behavioral approach and inhibition systems. Psychol. Sci. 8, 204–210. doi: 10.1111/j.1467-9280.1997.tb00413.x
Svensson, M., Brundin, L., Erhardt, S., Hållmarker, U., James, S., and Deierborg, T. (2021). Physical activity is associated with lower long-term incidence of anxiety in a population-based, large-scale study. Front. Psychiatry 12:1489. doi: 10.3389/fpsyt.2021.714014
Thibodeau, R., Jorgensen, R. S., and Kim, S. (2006). Depression, anxiety, and resting frontal EEG asymmetry: a meta-analytic review. J. Abnorm. Psychol. 115, 715–729. doi: 10.1037/0021-843X.115.4.715
Tomarken, A. J., and Davidson, R. J. (1994). Frontal brain activation in repressors and nonrepressors. J. Abnorm. Psychol. 103, 339–349. doi: 10.1037//0021-843x.103.2.339
Tomarken, A. J., Davidson, R. J., and Henriques, J. B. (1990). Resting frontal brain asymmetry predicts affective responses to films. J. Pers. Soc. Psychol. 59, 791–801. doi: 10.1037//0022-3514.59.4.791
Towers, D. N., and Allen, J. J. B. (2009). A better estimate of the internal consistency reliability of frontal EEG Asymmetry scores. Psychophysiology 46, 132–142. doi: 10.1111/j.1469-8986.2008.00759.x
Tuason, M. T., Güss, C. D., and Boyd, L. (2021). Thriving during COVID-19: predictors of psychological well-being and ways of coping. PLoS One 16:e0248591. doi: 10.1371/journal.pone.0248591
Umar Saeed, S. M., Anwar, S. M., Majid, M., Awais, M., and Alnowami, M. (2018). Selection of neural oscillatory features for human stress classification with single channel EEG headset. BioMed. Res. Int. 2018:e1049257. doi: 10.1155/2018/1049257
Van Albada, S., and Robinson, P. (2013). Relationships between electroencephalographic spectral peaks across frequency bands. Front. Hum. Neurosci. 7:56. doi: 10.3389/fnhum.2013.00056
van der Vinne, N., Vollebregt, M. A., van Putten, M. J. A. M., and Arns, M. (2017). Frontal alpha asymmetry as a diagnostic marker in depression: fact or fiction? A meta-analysis. NeuroImage Clin. 16, 79–87. doi: 10.1016/j.nicl.2017.07.006
Vecchiato, G., Maglione, A. G., Scorpecci, A., Malerba, P., Marsella, P., Di Francesco, G., et al. (2012). “EEG frontal asymmetry related to pleasantness of music perception in healthy children and cochlear implanted users,” in Proceedings of the 2012 Annual International Conference of the IEEE Engineering in Medicine and Biology Society, San Diego, CA, 4740–4743. doi: 10.1109/EMBC.2012.6347026
Veldhuizen, R. J., Jonkman, E. J., and Poortvliet, D. C. (1993). Sex differences in age regression parameters of healthy adults—Normative data and practical implications. Electroencephalogr. Clin. Neurophysiol. 86, 377–384. doi: 10.1016/0013-4694(93)90133-g
Vossel, S., Geng, J. J., and Fink, G. R. (2013). Dorsal and ventral attention systems: distinct neural circuits but collaborative roles. Neuroscientist 20, 150–159. doi: 10.1177/1073858413494269
Wahbeh, H., Yount, G., Vieten, C., Radin, D., and Delorme, A. (2021). Exploring personal development Workshops’ effect on well-being and interconnectedness: a pilot study. J. Altern. Complement. Med. doi: 10.3928/01484834-20101029-01
Wheeler, R. E., Davidson, R. J., and Tomarken, A. J. (1993). Frontal brain asymmetry and emotional reactivity: a biological substrate of affective style. Psychophysiology 30, 82–89. doi: 10.1111/j.1469-8986.1993.tb03207.x
Wingeier, B. M. (2000). A High-Resolution Study of Large-Scale Dynamic Properties of Human EEG. Ph.D. dissertation. New Orleans: Tulane University.
Wu, S., Xu, X., Shu, L., and Hu, B. (2017). “Estimation of valence of emotion using two frontal EEG channels,” in Proceedings of the 2017 IEEE International Conference on Bioinformatics and Biomedicine (BIBM), Kansas City, MO, 1127–1130. doi: 10.1109/BIBM.2017.8217815
Xu, Y.-Y., Feng, Z.-Q., Xie, Y.-J., Zhang, J., Peng, S.-H., Yu, Y.-J., et al. (2018). Frontal alpha EEG asymmetry before and after positive psychological interventions for medical students. Front. Psychiatry 9:432. doi: 10.3389/fpsyt.2018.00432
Zhao, S., Zhao, Q., Zhang, X., Peng, H., Yao, Z., Shen, J., et al. (2017). “Wearable EEG-based real-time system for depression monitoring,” in Brain Informatics, eds Y. Zeng, Y. He, J. H. Kotaleski, M. Martone, B. Xu, H. Peng, et al. (Cham: Springer International Publishing), 190–201. doi: 10.1007/978-3-319-70772-3_18
Keywords: wearable EEG, alpha asymmetry, frontal, temporoparietal, executive control, well-being, large sample analysis
Citation: Cannard C, Wahbeh H and Delorme A (2021) Electroencephalography Correlates of Well-Being Using a Low-Cost Wearable System. Front. Hum. Neurosci. 15:745135. doi: 10.3389/fnhum.2021.745135
Received: 16 August 2021; Accepted: 15 November 2021;
Published: 24 December 2021.
Edited by:
Gianluca Di Flumeri, Sapienza University of Rome, ItalyReviewed by:
Giulia Cartocci, Sapienza University of Rome, ItalyDario Rossi, Guido Carli Free International University for Social Studies, Italy
Copyright © 2021 Cannard, Wahbeh and Delorme. This is an open-access article distributed under the terms of the Creative Commons Attribution License (CC BY). The use, distribution or reproduction in other forums is permitted, provided the original author(s) and the copyright owner(s) are credited and that the original publication in this journal is cited, in accordance with accepted academic practice. No use, distribution or reproduction is permitted which does not comply with these terms.
*Correspondence: Cédric Cannard, Y2Nhbm5hcmRAcHJvdG9ubWFpbC5jb20=