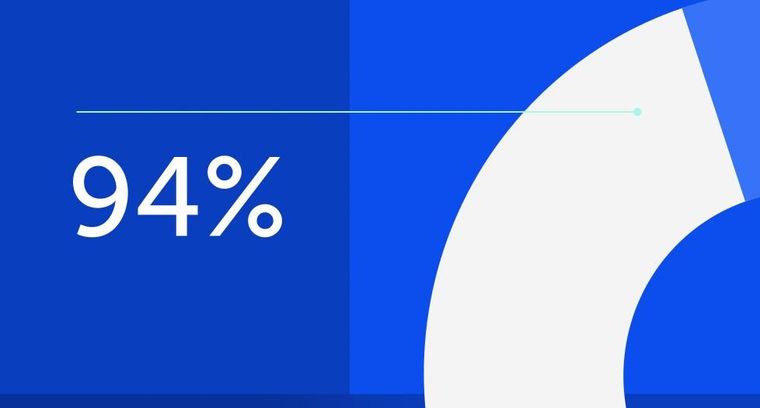
94% of researchers rate our articles as excellent or good
Learn more about the work of our research integrity team to safeguard the quality of each article we publish.
Find out more
MINI REVIEW article
Front. Hum. Neurosci., 13 August 2021
Sec. Brain-Computer Interfaces
Volume 15 - 2021 | https://doi.org/10.3389/fnhum.2021.705064
This article is part of the Research TopicWomen in NeuroscienceView all 35 articles
In the last few decades, Brain-Computer Interface (BCI) research has focused predominantly on clinical applications, notably to enable severely disabled people to interact with the environment. However, recent studies rely mostly on the use of non-invasive electroencephalographic (EEG) devices, suggesting that BCI might be ready to be used outside laboratories. In particular, Industry 4.0 is a rapidly evolving sector that aims to restructure traditional methods by deploying digital tools and cyber-physical systems. BCI-based solutions are attracting increasing attention in this field to support industrial performance by optimizing the cognitive load of industrial operators, facilitating human-robot interactions, and make operations in critical conditions more secure. Although these advancements seem promising, numerous aspects must be considered before developing any operational solutions. Indeed, the development of novel applications outside optimal laboratory conditions raises many challenges. In the current study, we carried out a detailed literature review to investigate the main challenges and present criteria relevant to the future deployment of BCI applications for Industry 4.0.
Recent advances in neuroscience and engineering led to the development of new applications interfacing minds with machines, known as Brain-Computer Interface (BCI) technology. The origins of BCI date back to the 1960s, with Delgado (1969) who notably developed an implantable chip used to both stimulate the brain by radio and send electrical signals of the brain by telemetry, allowing the subject to move about freely. A few years later, Vidal (1973) explored the use of scalp-recorded brain signals in humans to implement a simple non-invasive BCI based on “visually evoked potentials” (see Vidal, 1973). Those experiments paved the way for the development of non-invasive BCI paradigms that made use of neuroimaging techniques as electroencephalography (EEG), magnetoencephalography (MEG), functional magnetic resonance imaging (fMRI) and functional near-infrared spectroscopy (fNIRS) (see Rao, 2013 for a comprehensive review). Indeed, by translating the recorded neural activity into digital commands via mathematical and AI methods (see Wolpaw et al., 2002) (Figure 1), BCI enables controlling external devices with the brain (e.g., Padfield et al., 2019; Khan et al., 2020), such as a computer, a robot, or an exoskeleton (e.g., Nuyujukian et al., 2018; Benabid et al., 2019; Moses et al., 2019). This ability is particularly interesting in specific contexts where voice or motor commands cannot be used (e.g., Lin et al., 2014).
Regarding the user's task and the neural patterns of interest, we can distinguish three main categories of BCI, namely, active, reactive and passive BCI (see Kögel et al., 2019). Firstly, while using an active BCI, the agent must intentionally modulate their brain activity to bring out neural characteristics that will become identifiable after mathematical processing and classification, as Motor Imagery (MI) paradigm (e.g., Salvaris, 2014). Secondly, reactive BCI relies on neural activity that is typically triggered by an external stimulus—mostly visual or auditory—and that evokes brain responses, such as P300 event-related potential (ERP) (e.g., Jin et al., 2012) or Steady State Evoked Potentials (SSEP) (e.g., Chen et al., 2017). Thirdly, passive BCI relies on brain activity which is not voluntarily modulated by the user, in order to evaluate psychological states such as drowsiness (e.g., Hongfei et al., 2011; Dehais et al., 2018), frustration, or even cognitive load (e.g., Roy et al., 2013; Myrden and Chau, 2017). More details concerning the neurophysiological underpinnings, as well as the advantages and limitations of these three BCI methods are provided in section 3.1.
Thus, in the last two decades, many types of BCI techniques and applications have emerged, especially in the clinical field where it represents a promising technology for assisting or rehabilitating neurological patients and contribute to the faster reintegration of brain-injured patients (e.g., Chaudhary et al., 2016; Verplaetse et al., 2016). However, recent advances in neuroscience and technology, especially non-invasive and portable brain imaging techniques related to EEG, have encouraged the development of novel applications outside the medical and scientific areas (e.g., Abdulkader et al., 2015; Rashid et al., 2020). Notably, one might list the following fields of education (e.g., Wegemer, 2019), entertainment (e.g., Bonnet et al., 2013; Kerous et al., 2018; Ramchurn et al., 2018; Vasiljevic and Miranda, 2020), biometrics authentification (e.g., Alariki et al., 2018; Chan et al., 2018), or even civil and military aviation (e.g., Dehais et al., 2018).
Recently, the industrial sector has also shown a growing interest in BCI (e.g., Angrisani et al., 2018), where the societal, economic and commercial impacts of this technology could be important (e.g., Van Erp et al., 2012). Indeed, since modern times, Industry has continuously seized on emerging technologies to improve its efficiency and performance and each industrial revolution has entailed deep socioeconomic changes and challenges (see Morrar et al., 2017). The last industrial revolution, also known as Industry 4.0, specifically harnessed digital technologies such as AI, big data and analytics, or the Internet of things (see Chunguang et al., 2020), which led to a constantly evolving and intelligent automation of industrial processes. However, it also raises important questions regarding environmental, ethical and human factors (see Melnyk et al., 2019). Interestingly, similar technologies are presently required to develop sophisticated BCI, notably, to efficiently process brain signals and emit commands to the connected device. Then, regarding this technological compatibility, BCI applications could theoretically constitute a potential extension of the 4th industrial revolution. Moreover, “Human enhancement” provided by BCI techniques could represent a viable way to conciliate industrial and societal concerns in a near future.
To this day, the use of BCI techniques in Industry 4.0 remains theoretical, being mainly explored in academic articles or exhibition demonstrators. In the following, we investigate the potential benefits of implementing BCI and how it could help to re-introduce humans within the industrial processes, by facilitating the operator's work and limiting potential risks and human errors (e.g., Jinjing et al., 2021). Indeed, technical and ethical limitations inherent in non-invasive BCI necessarily hamper the expansion of this technology within operational contexts (e.g., Rashid et al., 2020). Thus, the question of selecting the most suitable and relevant BCI technique for the industrial sector arises, especially regarding its reliability, its generalizability or its ease of use. In this context, we sought to explore the criteria that will be decisive for the potential integration of BCI in industrial settings regarding the current maturity of BCI techniques. In the current review, we attempt to answer the following question:
• Which BCI techniques are most likely to be deployed in future industrial applications?
To this end, we carried out a detailed literature review of the databases (Science direct, PubMed, IEEE, Springer, ArXiv, ResearchGate, Google Scholar, MDPI, HAL) queried with the following inclusive keywords: BCI, Brain-computer interface, BCI and Industry 4.0, EEG-based BCI, BCI applications, BCI challenges, Assistive technology associated to scientific studies published between 2010 and 2021. Then, we extracted recent and relevant empirical and review articles, conference proceedings, research reports related to the usage of BCI in industrial environments. Note that the exclusion criteria include “Invasive BCI techniques, non-invasive BCI for both clinical applications and non-industrial fields.” In addition, all the references that are provided in the following sections serve as recent examples for the identified BCI applications for Industry 4.0.
Theoretically, the deployment of BCI applications in Industry 4.0 could contribute to put the operator back at the center of industrial processes. The possible industrial applications could be categorized as follows: (1) safety at work, (2) adaptive training and (3) device's control (e.g., Tamburrini, 2014; Balderas et al., 2015; Oztemel and Gursev, 2018).
Recently, there has been a growing interest in using EEG-based BCI as a potential solution allowing to reduce safety risks, while enhancing productivity and improving decisions in operators and managers (e.g., Villalba-Diez et al., 2019). Regarding technical aspects, this application would rely on the use of passive BCI. Notably, the decomposition of EEG signal into frequency bands represents a convenient way to identify the user's neurocognitive condition. For instance, many studies have shown that the spectral power in alpha (8–13 Hz) and theta (4–7 Hz) bands increase when a person feels fatigued (e.g., Craig et al., 2012; Dehais et al., 2018). Such modulation can therefore constitute a first-rate indicator of the user's arousal state (e.g., Zhang et al., 2017). Changes in theta rhythm in frontal sites and alpha rhythm in parietal sites (e.g., Borghini et al., 2013), could also indicate a state of cognitive overload, which has been linked to reduced performances in complex tasks (e.g., Aricò et al., 2018). Thus, by allowing user-state monitoring, passive BCI could notably limit or prevent safety risks and human errors without requiring any particular effort from the user. Theoretically, this passive aspect makes it potentially usable in multitasking contexts and does not induce additional fatigue. However, passive BCI is subject to an important inter-individual variability. Moreover, EEG band frequencies must be carefully analyzed because similar spectral power patterns could be associated to several mental states (e.g., Aricò et al., 2018).
Then, the neurofeedback provided by passive BCIs could reinforce safety at work by preventing agents from committing dangerous errors due to drowsiness or cognitive overload for instance (e.g., Villalba-Diez et al., 2019). In fact, some industrial sectors—including among others, manufacturing, quality control or pharmaceutical industry—require operators to carry out a large number of repetitive and sensible actions that directly depend on the operator's neurocognitive states (e.g., Villalba-Diez et al., 2019). In this context, EEG-based BCI could allow to monitor operators' mental states like fatigue, stress, or loss of vigilance which can be critical during dangerous activities. In particular, fatigue monitoring is more considered as a valuable tool in repetitive and automatic tasks such as driving, piloting or quality control (e.g., Zhang et al., 2017; Huang et al., 2019). For this reason, some solutions that integrate EEG captors within worksite helmets (e.g., Li et al., 2014; Barkallah et al., 2015) or under headwears (e.g., Zhang et al., 2017) have already been proposed to warn users whenever a critical drowsiness threshold is reached.
Another emerging BCI application—also relying on passive BCI technique—concerns adaptive training, which might reinforce the learning process of complex industrial procedures. This neurofeedback approach is already used in clinical settings, to support learning or rehabilitate attention in children with neurodevelopmental disorders (e.g., Papanastasiou et al., 2020). Indeed, such monitored training would allow boosting attentional processes while adapting task difficulty according to cognitive load or vigilance, to optimize learning and prevent frustration. In this context, Huang et al. (2019) proposed to combine BCI with other technologies such as Virtual Reality (VR) and/or Augmented Reality (AR) to make the learning task even more immersive and efficient. Similarly, Nisiforou (2013) proposed the use of eye tracking coupled with EEG to assess and evaluate students' cognitive dimensions.
Besides monitoring applications, another potential industrial use case concerns cobots or machine' s control. Both active and reactive BCI paradigms could be relevant for this kind of application. Regarding active BCI, motor imagery (MI-BCI) is the most commonly used paradigm. During this task, the user is typically required to imagine specific movements (e.g., for limb), that allows controlling an external device in the same way (e.g., a robot, an exoskeleton or an avatar etc.). Indeed, imagining a movement typically produces neural activity that is spatiotemporally similar to the activity generated during actual movement, but smaller in magnitude (see Wolpaw et al., 1991; Pfurtscheller et al., 1997; McFarland et al., 2000). Although this method is particularly promising in the context of motor disability, its main drawbacks stem from some limitations of the EEG method itself. Notably, due to a low spatial resolution, it is not possible to localize accurately activation sources within the same hemispheric sensorimotor cortex, which prevents the reliable identification of fine motor movements (e.g. distinguishing a movement of the whole arm from a movement of the sole hand). This generally limits the number of potential and reliable orders to 4 (e.g., Schlögl et al., 2005). Another disadvantage of the MI paradigm relies on the EEG low signal/noise ratio. Indeed, due to its non-invasive nature, EEG recordings also contain irrelevant, non-brain signals like environmental electromagnetic artifacts or peripheral nervous transmissions. In addition, this technique requires a long training phase to be properly mastered (e.g., Vidaurre et al., 2011) that can last several days or weeks and which is incompatible with many industrial contexts. Then, even after a substantial training phase, more than 30% of individuals would remain BCI illiterate (e.g., Ahn et al., 2014; Lee et al., 2019), by staying unable to control the device. Finally, motor imagery requires an intense concentration from the user and is incompatible with other “real” movements that would interfere with the thought command and ultimately, with the BCI accuracy. Thus, MI paradigms prevents the user from performing other tasks at the same time and necessarily generates fatigue (e.g., Talukdar et al., 2019) which makes its use rather inflexible. Despite all these limitations, MI training session design is of vital importance for clinicians planning to implement interventions adapted to participant health status, age and gender. For that, numerous studies are attempting to overpass these limitations—notably regarding the problematic user-training phase—by proposing some guidelines that could be useful to improve this critical dimension (e.g., Schuster et al., 2011; Jeunet et al., 2016)
Regarding reactive BCI, the two most widely used and reliable EEG markers are the P300 and SSVEP (steady-state evoked potentials). P300 is a positive event-related potential that is apparent whenever the user has noticed an unexpected or a rare visual or auditory event (e.g., Walter et al., 1964; Donchin and Smith, 1970). Although associated to a fast training, this technique remains very sensitive to surrounding noise and motor artifacts (e.g., Chamola et al., 2020), preventing its use in a noisy or multitasking context. In addition, a single command requires the user to focus their attention on several consecutive events, including non-relevant (non-rare) and relevant (rare and unexpected) ones, which necessarily decreases the system's speed (e.g., Lotte et al., 2015) while being costly in terms of attentional processes (fatigability).
As regards SSVEPs, the distinct potential commands are displayed via a visual interface (e.g., screen or AR glasses), icons that flicker at distinct frequencies (e.g., 10 Hz) represent different options. Then, while the user is focusing on one flickering option, visual neurons (i.e., from the primary visual cortex) are synchronously discharging at the same rate, which will ultimately allow the user's choice to be identified with classification algorithms (e.g., Middendorf et al., 2000; Faller et al., 2010). Interestingly, SSVEP-BCI is less prone to inter-individual differences, which enhances its accuracy (e.g., Lotte et al., 2015) and reduces its illiteracy rate (e.g., Lee et al., 2019). Moreover, it does not require a long training phase (e.g., Guger et al., 2012) while the latency between the neural command and the command execution can potentially be lower than in other BCI paradigms. However, similarly to the P300 paradigm, extended use can induce significant fatigue, due to the required active concentration on stimuli. Another disadvantage of reactive BCI is the need to use external stimuli to allow the agent to make a choice. The exerted control is therefore limited to the presented options and is not strictly endogenous. Moreover, it requires an additional interface, such as a screen, which decreases its portability (e.g., Cecotti et al., 2010). Regarding this last limitation, recent works have attempted to create new interface designs and stimuli that reduce fatigue and discomfort, to promote a daily and long-term use of this BCI paradigm (see Baek et al., 2019).
In industrial settings, an SSVEP-BCI combined with AR glasses could facilitate making certain tasks hands-free (and therefore, replace buttons/joysticks) for operators who control machines (e.g., Angrisani et al., 2018, 2020). Looking further ahead, one might also imagine that a MI-BCI could eventually allow to quickly take over control of transport vehicles in case of emergency braking. In this scenario, BCI must be sufficiently advanced to allow a reliable transmission of the “thought” braking command to the mobile device. It should also be faster than our peripheral nervous transmission to become valuable regarding accidents prevention, which is far from being the case at present (e.g., Royer et al., 2010; Kim and Lee, 2017; Georgescu et al., 2020).
Regardless the potential benefits that BCI could bring to Industry and besides the actual weaknesses of EEG-based BCI, it is also necessary to consider its current ethical, ergonomic and technical limitations, before any operational development or usage.
The first limitation relates to ethics and acceptability that must be further questioned and regulated regarding the individual and societal impacts that industrial BCI applications might have. Among other things, industrial BCI must ensure data confidentiality and security given the sensible and personal nature of recorded physiological signals (e.g., Burwell et al., 2017). In addition to the operator consent, individual data must be locally stored and processed. Then, the relevant extracted information must be accessible to the sole concerned operators. To be acceptable to the end users, the BCI system must provide a real improvement of work conditions and/or safety by limiting the risks in dangerous conditions, such as passive BCI. Presently, non-invasive BCI for device control (active and reactive BCI) remains too immature to get easily used and adopted by the agents. According to Burwell et al., 2017, the need for regular and challenging training sessions (e.g, Motor Imagery) may impose physical, emotional, and financial burdens on the user (e.g., Fenton and Alpert, 2008) and it may require more cognitive planning and attention than a user can achieve on a regular basis, leading to frustration (e.g., Glannon, 2014).
Another crucial requirement for an effective adoption by end-users concerns ergonomics (e.g., Li et al., 2014). More precisely, BCI solutions must be non-invasive; comfortable to wear; portable and not bulky to allow mobility in different areas; non-tiring for the user; multitasking-compatible to allow usual tasks without limitations; inexpensive in terms of training time and dedicated resources. On the one hand, passive BCIs are currently more suited to the criteria of portability, non-fatigability and multitasking since they do not require external stimuli and devices (e.g., AR glasses or monitor) and they do not require to perform a particular cognitive task, in comparison with active and reactive paradigms (see Wolpaw et al., 2002; Rao, 2013). On the other hand, the training cost is particularly important in active BCI, while it is less important in reactive and passive BCI (e.g., Cecotti, 2010; Jeunet et al., 2017; Myrden and Chau, 2017).
Moreover, BCI technical specificities must be considered to ensure: (1) reliability—which depends on classifications' accuracy—(2) reactivity in terms of response time and (3) flexibility to adapt to context and individual differences (e.g., Rashid et al., 2020). In other words, an ideal BCI solution must be able to interpret an operator's neural signal, by minimizing classification errors and training time, while increasing the information transfer rate (ITR) and its generalizability of use (e.g., Rashid et al., 2020). According to literature, reliability and flexibility appear to be higher in reactive BCI and particularly in SSVEP-based BCI (e.g., Chen et al., 2014), relative to active and passive paradigms. Flexibility appears lower in active paradigms, with a high illiteracy rate, in comparison with reactive and passive paradigms (e.g., Lee et al., 2019). Reliability depends on the quality of the collected signal and the relevance of AI algorithms applied. Besides, some neurophysiological markers used are more or less resistant to surrounding noise and should be carefully selected with a reduced latency. In addition, a large-scale deployment BCI requires adaptability and flexibility to make it usable and equally reliable for a large number of users.
Based on the advantages and disadvantages of each BCI application described previously (section 3.1), Table 1 summarizes the estimated levels of adequacy between the main industrial criteria (section 3.2) and the actual state-of-the-art EEG-based BCI, in the light of potential applications. More specifically, we have compared the three main non-invasive BCI paradigms and their potential industrial applications considering the most important criteria related to ethics, ergonomics & UX, and technical.
Table 1. Levels of adequacy between industrial and technical BCI requirements based on the EEG, regarding potential industrial applications.
The level of adequacy is ranked as follow: “+” rating represents a low level of match between the industrial requirement and the BCI technique, while “++” and “+++” means an intermediate and a high level of suitability, respectively.
BCI is an emerging technology that enables to decode brain activity and translate it into a set of actions reflecting the user's intention, mental state, and even emotions. Numerous public and private actors are envisioning the deployment of BCI in industrial settings in a near future (e.g., Sujatha Ravindran et al., 2020). In the present paper, we summarized the potential applications, key success factors and the most advanced EEG-based BCI paradigms for Industry 4.0. Currently, none of the EEG-based BCI evaluated fits ideally to all the essential industrial criteria we have established based on ethical, ergonomic and technical factors. However, SSVEP-based BCI represents a highly promising technology for device control, while fatigue monitoring appears particularly interesting and appropriate to prevent damaging errors and safety risks in dangerous contexts, or optimize training upstream of these critical situations. With the dramatic rise of EEG-based BCI studies—regarding material, associated algorithms (machine learning, deep learning etc.) or even psychological aspects of BCI—we believe that the large-scale deployment of BCI applications in the Industry is a matter of years. Thus, the ethics and rules related to BCI applications in industrial settings need to be carefully defined to pave the way to effective use.
The authors are aware that the present results should be interpreted with caution and some important limitations deserve to be mentioned. Firstly, the envisioned industrial applications of BCI are still at the first stages of research and development. Thus, the development of reliable and ethical BCI solutions adapted to end-users in industrial contexts will necessarily take several years. Secondly, research focussing on BCI users' experience remains extremely rare and further studies must explore this important dimension. Though the users' experience is currently strongly related to technical specificities of BCIs solutions, a user-centered approach similar to the one proposed by Kubler et al. (2014) must be systematically used in future research before considering any large-scale deployment of such neurotechnology.
KD conceived the initial idea, conducted the research, and wrote the first draft with AL. GB reviewed the article's structure. SL contributed with BCI paradigms relevant to Industry 4.0, performed the synthesis, and perspectives with KD. RB and LN contributed, with other authors, to the final proofreading and commented on the draft. All authors have read and approved the final paper.
The authors declare that the research was conducted in the absence of any commercial or financial relationships that could be construed as a potential conflict of interest.
All claims expressed in this article are solely those of the authors and do not necessarily represent those of their affiliated organizations, or those of the publisher, the editors and the reviewers. Any product that may be evaluated in this article, or claim that may be made by its manufacturer, is not guaranteed or endorsed by the publisher.
Abdulkader, S. N., Atia, A., and Mostafa, M.-S. M. (2015). Brain computer interfacing: applications and challenges. Egypt. Inform. J. 16, 213–230. doi: 10.1016/j.eij.2015.06.002
Ahn, M., Lee, M., Choi, J., and Jun, S. (2014). A review of brain-computer interface games and an opinion survey from researchers, developers and users. Sensors 14, 14601–14633. doi: 10.3390/s140814601
Alariki, A. A., Ibrahimi, A. W., Wardak, M., and Wall, J. (2018). A review study of brian activity-based biometric authentication. J. Comput. Sci. 14, 173–181. doi: 10.3844/jcssp.2018.173.181
Angrisani, L., Arpaia, P., Esposito, A., and Moccaldi, N. (2020). A wearable brain–computer interface instrument for augmented reality-based inspection in industry 4.0. IEEE Trans. Instrument. Meas. 69, 1530–1539. doi: 10.1109/TIM.2019.2914712
Angrisani, L., Arpaia, P., Moccaldi, N., and Esposito, A. (2018). “Wearable augmented reality and brain computer interface to improve human-robot interactions in smart industry: a feasibility study for SSVEP signals,” in 2018 IEEE 4th International Forum on Research and Technology for Society and Industry (RTSI) (Palermo: IEEE), 1–5. doi: 10.1109/RTSI.2018.8548517
Aricò, P., Borghini, G., Di Flumeri, G., Sciaraffa, N., and Babiloni, F. (2018). Passive BCI beyond the lab: current trends and future directions. Physiol. Meas. 39:08TR02. doi: 10.1088/1361-6579/aad57e
Baek, H. J., Chang, M. H., Heo, J., and Park, K. S. (2019). Enhancing the usability of brain-computer interface systems. Comput. Intell. Neurosci. 2019:5427154. doi: 10.1155/2019/5427154
Balderas, D., Molina, A., and Ponce, P. (2015). Alternative classification techniques for brain-computer interfaces for smart sensor manufacturing environments. IFAC Pap. Online 48, 680–685. doi: 10.1016/j.ifacol.2015.06.161
Barkallah, E., Otis, M. J., Ngomo, S., and Heraud, M. (2015). “Measuring operator's pain: toward evaluating musculoskeletal disorder at work,” in 2015 IEEE International Conference on Systems, Man, and Cybernetics (Kowloon: IEEE), 2366–2371.
Benabid, A. L., Costecalde, T., Eliseyev, A., Charvet, G., Verney, A., Karakas, S., et al. (2019). An exoskeleton controlled by an epidural wireless brain-machine interface in a tetraplegic patient: a proof-of-concept demonstration. Lancet Neurol. 18, 1112–1122. doi: 10.1016/S1474-4422(19)30321-7
Bonnet, L., Lotte, F., and Lecuyer, A. (2013). Two brains, One game: design and evaluation of a multiuser BCI video game based on motor imagery. IEEE Trans. Comput. Intell. AI Games 5, 185–198. doi: 10.1109/TCIAIG.2012.2237173
Borghini, G., Aricò, P., Astolfi, L., Toppi, J., Cincotti, F., Mattia, D., et al. (2013). “Frontal EEG theta changes assess the training improvements of novices in flight simulation tasks,” in 2013 35th Annual International Conference of the IEEE Engineering in Medicine and Biology Society (EMBC) (Osaka: IEEE), 6619–6622.
Burwell, S., Sample, M., and Racine, E. (2017). Ethical aspects of brain computer interfaces: a scoping review. BMC Med. Ethics 18:60. doi: 10.1186/s12910-017-0220-y
Cecotti, H. (2010). A self-paced and calibration-less SSVEP-based brain–computer interface speller. IEEE Trans. Neural Syst. Rehabil. Eng. (Gainesville, FL) 18, 127–133. doi: 10.1109/TNSRE.2009.2039594
Cecotti, H., Volosyak, I., and Gräser, A. (2010). “Reliable visual stimuli on lcd screens for SSVEP based BCI,” in 2010 18th European Signal Processing Conference (Aalborg: IEEE), 919–923.
Chamola, V., Vineet, A., Nayyar, A., and Hossain, E. (2020). Brain-computer interface-based humanoid control: A review. Sensors 20:3620. doi: 10.3390/s20133620
Chan, H.-L., Kuo, P.-C., Cheng, C.-Y., and Chen, Y.-S. (2018). Challenges and future perspectives on electroencephalogram-based biometrics in person recognition. Front. Neuroinform. 12:66. doi: 10.3389/fninf.2018.00066
Chaudhary, U., Birbaumer, N., and Ramos-Murguialday, A. (2016). Brain–computer interfaces for communication and rehabilitation. Nat. Rev. Neurol. 12:513. doi: 10.1038/nrneurol.2016.113
Chen, J., Zhang, D., Engel, A. K., Gong, Q., and Maye, A. (2017). Application of a single-flicker online SSVEP BCI for spatial navigation. PLoS ONE 12:e0178385. doi: 10.1371/journal.pone.0178385
Chen, X., Chen, Z., Gao, S., and Gao, X. (2014). A high-ITR SSVEP-based BCI speller. Brain Comput. Interfaces 1, 181–191. doi: 10.1080/2326263X.2014.944469
Chunguang, B., Patrick, D., Guido, O., and Joseph, S. (2020). Industry 4.0 technologies assessment: a sustainability perspective. Int. J. Product. Econ. 229:107776. doi: 10.1016/j.ijpe.2020.107776
Craig, A., Tran, Y., Wijesuriya, N., and Nguyen, H. (2012). Regional brain wave activity changes associated with fatigue. Psychophysiology 49, 574–582. doi: 10.1111/j.1469-8986.2011.01329.x
Dehais, F., Dupres, A., Di Flumeri, G., Verdiere, K., Borghini, G., Babiloni, F., et al. (2018). “Monitoring pilot's cognitive fatigue with engagement features in simulated and actual flight conditions using an hybrid fNIRS-EEG passive BCI,” in 2018 IEEE International Conference on Systems, Man, and Cybernetics (SMC) (Miyazaki: IEEE), 544–549.
Delgado, J. (1969). Physical Control of the Mind: Toward a Psychocivilized Society. New York, NY: Harper and Row.
Donchin, E., and Smith, D. B. (1970). The contingent negative variation and the late positive wave of the average evoked potential. Electroencephalogr. Clin. Neurophysiol. 29, 201–203. doi: 10.1016/0013-4694(70)90124-0
Faller, J., Müller-Putz, G., Schmalstieg, D., and Pfurtscheller, G. (2010). An application framework for controlling an avatar in a desktop-based virtual environment via a software SSVEP brain–computer interface. Presence Teleoperat. Virt. Environ. 19, 25–34. doi: 10.1162/pres.19.1.25
Fenton, A., and Alpert, S. (2008). Extending our view on using BCIs for locked-in syndrome. Neuroethics 1, 119–132. doi: 10.1007/s12152-008-9014-8
Georgescu, L., Wallace, D., Kyong, D., Chun, A., Chun, K., and Oh, P. (2020). “The future of work: towards service robot control through brain-computer interface,” in 2020 10th Annual Computing and Communication Workshop and Conference (CCWC) (Las Vegas, NV), 932–937. doi: 10.1109/CCWC47524.2020.9031211
Glannon, W. (2014). Neuromodulation, agency and autonomy. Brain Topogr. 27, 46–54. doi: 10.1007/s10548-012-0269-3
Guger, C., Allison, B. Z., Großwindhager, B., Prückl, R., Hintermüller, C., Kapeller, C., et al. (2012). How many people could use an SSVEP BCI? Front. Neurosci. 6:169. doi: 10.3389/fnins.2012.00169
Hongfei, J., Jie, L., Lei, C., and Daming, W. (2011). “A EEG-based brain computer interface system towards applicable vigilance monitoring,” in Foundations of Intelligent Systems eds Z. W. Ras and S. Ohsuga (Springer), 743–749.
Huang, Z., Javaid, A., Devabhaktuni, V. K., Li, Y., and Yang, X. (2019). Development of cognitive training program with EEG headset. IEEE Access 7, 126191–126200. doi: 10.1109/ACCESS.2019.2937866
Jeunet, C., N'Kaoua, B., and Lotte, F. (2016). “Chapter 1: Advances in user-training for mental-imagery-based BCI control: psychological and cognitive factors and their neural correlates,” in Brain-Computer Interfaces: Lab Experiments to Real-World Applications, ed. D. Coyle (Ulster: Elsevier), 3–35. doi: 10.1016/bs.pbr.2016.04.002
Jeunet, C., N'Kaoua, B., and Lotte, F. (2017). “Towards a cognitive model of mi-BCI user training,” in International Graz BCI Conference
Jin, J., Allison, B. Z., Kaufmann, T., Kubler, A., Zhang, Y., Wang, X., et al. (2012). The changing face of p300 BCIs: a comparison of stimulus changes in a p300 BCI involving faces, emotion, and movement. PLoS ONE 7:e49688. doi: 10.1371/journal.pone.0049688
Jinjing, K., Ming, Z., Xiaowei, L., and Jiayu, C. (2021). Monitoring distraction of construction workers caused by noise using a wearable electroencephalography (EEG) device. Automat. Construct. 125:103598. doi: 10.1016/j.autcon.2021.103598
Kerous, B., Skola, F., and Liarokapis, F. (2018). EEG-based BCI and video games: a progress report. Virtual Reality 22, 119–135. doi: 10.1007/s10055-017-0328-x
Khan, M., Das, R., Iversen, H., and Puthusserypady, S. (2020). Review on motor imagery based BCI systems for upper limb post-stroke neurorehabilitation: from designing to application. Comput. Biol. Med. 123:103843. doi: 10.1016/j.compbiomed.2020.103843
Kim, J., and Lee, S. (2017). “Time domain EEG analysis for evaluating the effects of driver's mental work load during simulated driving,” in 2017 5th International Winter Conference on Brain-Computer Interface (BCI) (Seoul), 79–80. doi: 10.1109/IWW-BCI.2017.7858165
Kögel, J., Schmid, J. R., Jox, R. J., and Friedrich, O. (2019). Using brain-computer interfaces: a scoping review of studies employing social research methods. BMC Med. Ethics 20:18. doi: 10.1186/s12910-019-0354-1
Kubler, A., Holz, E. M., Riccio, A., Zickler, C., Kaufmann, T., Kleih, S. C., et al. (2014). The user-centered design as novel perspective for evaluating the usability of BCI-controlled applications. PLOS ONE 9:e112392. doi: 10.1371/journal.pone.0112392
Lee, M.-H., Kwon, O.-Y., Kim, Y.-J., Kim, H.-K., Lee, Y.-E., Williamson, J., et al. (2019). EEG dataset and openbmi toolbox for three BCI paradigms: an investigation into BCI illiteracy. GigaScience 8:giz002. doi: 10.1093/gigascience/giz002
Li, P., Meziane, R., Otis, M. J., Ezzaidi, H., and Cardou, P. (2014). “A smart safety helmet using IMU and EEG sensors for worker fatigue detection,” in 2014 IEEE International Symposium on Robotic and Sensors Environments (ROSE) Proceedings (Timisoara), 55–60. doi: 10.1109/ROSE.2014.6952983
Lin, C.-T., Lin, B.-S., Lin, F.-C., and Chang, C.-J. (2014). Brain computer interface-based smart living environmental auto-adjustment control system in UPnP home networking. IEEE Syst. J. 8, 363–370. doi: 10.1109/JSYST.2012.2192756
Lotte, F., Bougrain, L., and Clerc, M. (2015). “Electroencephalography (EEG)-based brain-computer interfaces,” in Wiley Encyclopedia of Electrical and Electronics Engineering, ed B. Kurzman (Hoboken, NJ: Wiley), 44. doi: 10.1002/047134608X.W8278
McFarland, D. J., Miner, L. A., Vaughan, T. M., and Wolpaw, J. R. (2000). Mu and beta rhythm topographies during motor imagery and actual movements. Brain Topogr. 12, 177–186. doi: 10.1023/a:1023437823106
Melnyk, L. H., Kubatko, O. V., Dehtyarova, I. B., Dehtiarova, I. B., Matsenko, O. M., and Rozhko, O. D. (2019). The effect of industrial revolutions on the transformation of social and economic systems. Probl. Perspect. Manage. 17, 381–391. doi: 10.21511/ppm.17(4).2019.31
Middendorf, M., McMillan, G., Calhoun, G., and Jones, K. (2000). Brain computer interfaces based on the steady-state visual-evoked response. IEEE Trans. Rehab. Eng. 8, 211–214. doi: 10.1109/86.847819
Morrar, R., Arman, H., and Mousa, S. (2017). The fourth industrial revolution (industry 4.0): a social innovation perspective. Technol. Innovat. Manage. Rev. 7, 12–20. doi: 10.22215/TIMREVIEW/1117
Moses, D. A., Leonard, M. K., Makin, J. G., and Chang, E. F. (2019). Real-time decoding of question-and-answer speech dialogue using human cortical activity. Nat. Commun. 10:3096. doi: 10.1038/s41467-019-10994-4
Myrden, A., and Chau, T. (2017). A passive EEG-BCI for single-trial detection of changes in mental state. IEEE Trans. Neural Syst. Rehabil. Eng. 25, 345–356. doi: 10.1109/TNSRE.2016.2641956
Nisiforou, E. (2013). Using eye tracking and electroencephalography to assess and evaluate students' cognitive dimensions. CEUR Workshop Proc. 1093, 79–86. doi: 10.1080/09523987.2013.862363
Nuyujukian, P., Albites Sanabria, J., Saab, J., Pandarinath, C., Jarosiewicz, B., Blabe, C. H., et al. (2018). Cortical control of a tablet computer by people with paralysis. PLoS ONE 13:e0204566. doi: 10.1371/journal.pone.0204566
Oztemel, E., and Gursev, S. (2018). Literature review of industry 4.0 and related technologies. J. Intell. Manufact. 31, 127–182. doi: 10.1007/s10845-018-1433-8
Padfield, N., Zabalza, J., Zhao, H., Masero, V., and Ren, J. (2019). EEG-based brain-computer interfaces using motor-imagery: techniques and challenges. Sensors 19:1423. doi: 10.3390/s19061423
Papanastasiou, G., Drigas, A., Skianis, C., and Lytras, M. (2020). Brain computer interface based applications for training and rehabilitation of students with neurodevelopmental disorders. A literature review. Heliyon 6:e04250. doi: 10.1016/j.heliyon.2020.e04250
Pfurtscheller, G., Neuper, C., Andrew, C., and Edlinger, G. (1997). Foot and hand area mu rhythms. Int. J. Psychophysiol. 26, 121–135.
Ramchurn, R., Wilson, M. L., Martindale, S., and Benford, S. (2018). “#Scanners 2 - the MOMENT: a new brain-controlled movie,” in Extended Abstracts of the 2018 CHI Conference on Human Factors in Computing Systems (Montreal, QC: ACM), 1–4. doi: 10.1145/3170427.3186481
Rashid, M., Sulaiman, N., P. P. Abdul Majeed, A., Musa, R. M., Ab. Nasir, A. F., Bari, B. S., et al. (2020). Current status, challenges, and possible solutions of EEG-based brain-computer interface: a comprehensive review. Front. Neurorobot. 14:25. doi: 10.3389/fnbot.2020.00025
Roy, R. N., Bonnet, S., Charbonnier, S., and Campagne, A. (2013). “Mental fatigue and working memory load estimation: interaction and implications for EEG-based passive BCI,” in 2013 35th Annual International Conference of the IEEE Engineering in Medicine and Biology Society (EMBC) (Osaka: IEEE), 6607–6610.
Royer, A. S., Doud, A. J., Rose, M. L., and Bin He (2010). EEG control of a virtual helicopter in 3-dimensional space using intelligent control strategies. IEEE Trans. Neural Syst. Rehabil. Eng. 18, 581–589. doi: 10.1109/TNSRE.2010.2077654
Salvaris, H. P. M. (2014). Decoding intention at sensorimotor timescales. PLoS ONE 9:e85100. doi: 10.1371/journal.pone.0085100
Schlögl, A., Lee, F., Bischof, H., and Pfurtscheller, G. (2005). Characterization of four-class motor imagery EEG data for the BCI-competition 2005. J. Neural Eng. 2:L14. doi: 10.1088/1741-2560/2/4/L02
Schuster, C., Hilfiker, R., Amft, O., Scheidhauer, A., Andrews, B., Butler, J., et al. (2011). Best practice for motor imagery: a systematic literature review on motor imagery training elements in five different disciplines. BMC Med. 9:75. doi: 10.1186/1741-7015-9-75
Sujatha Ravindran, A., Aleksi, T., Ramos-Murguialday, A., Biasiucci, A., Forsland, A., Paek, A., et al. (2020). Standards Roadmap: Neurotechnologies for Brain-Machine Interfacing. Technical report. IEEE.
Talukdar, U., Hazarika, S. M., and Gan, J. Q. (2019). Motor imagery and mental fatigue: inter-relationship and EEG based estimation. J. Comput. Neurosci. 46, 55–76. doi: 10.1007/s10827-018-0701-0
Tamburrini, G. (2014). “Philosophical reflections on brain-computer interfaces,” in Brain-Computer-Interfaces in Their Ethical, Social and Cultural Contexts, The International Library of Ethics, Law and Technology, Vol. 12. eds G. Grubler, and E. Hildt E. (Dordrecht: Springer). doi: 10.1007/978-94-017-8996-7_13
Van Erp, J., Lotte, F., and Tangermann, M. (2012). Brain-computer interfaces: beyond medical applications. Computer 45, 26–34. doi: 10.1109/MC.2012.107
Vasiljevic, G. A. M., and Miranda, L. (2020). Brain-computer interface games based on consumer-grade EEG devices: a systematic literature review. Int. J. Hum. Comput. Interact. 36:105. doi: 10.1080/10447318.2019.1612213
Verplaetse, T., Sanfilippo, F., Rutle, A., Osen, O. L., and Bye, R. T. (2016). “On usage of EEG brain control for rehabilitation of stroke patients,” in ECMS (Regensburg).
Vidal, J. (1973). Toward direct brain- computer communication. Annu. Rev. Biophys. Bioeng. 2, 157–180.
Vidaurre, C., Sannelli, C., Müller, K.-R., and Blankertz, B. (2011). Machine-learning-based coadaptive calibration for brain-computer interfaces. Neural Comput. 23, 791–816. doi: 10.1162/NECO_a_00089
Villalba-Diez, J., Zheng, X., Schmidt, D., and Molina, M. (2019). Characterization of industry 4.0 lean management problem-solving behavioral patterns using EEG sensors and deep learning. Sensors 19:2841. doi: 10.3390/s19132841
Walter, W., Aldridge, V., McCallum, W., and Winter, A. (1964). Contingent negative variation: an electric sign of sensorimotor association and expectancy in the human brain. Nature 203, 380–384. doi: 10.1038/203380a0
Wegemer, C. (2019). Brain-computer interfaces and education: the state of technology and imperatives for the future. Int. J. Learn. Technol. 14, 141–161. doi: 10.1504/IJLT.2019.101848
Wolpaw, J., McFarland, D., Neat, G., and Forneris, C. (1991). An EEG-based brain-computer interface for cursor control. Electroencephalogr Clin Neurophysiol. 78, 252–259.
Wolpaw, J. R., Birbaumer, N., McFarland, D. J., Pfurtscheller, G., and Vaughan, T. M. (2002). Brain–computer interfaces for communication and control. Clin. Neurophysiol. 113, 767–791. doi: 10.1016/S1388-2457(02)00057-3
Keywords: EEG, BCI applications, BCI challenges, technology transfer, Industry 4.0
Citation: Douibi K, Le Bars S, Lemontey A, Nag L, Balp R and Breda G (2021) Toward EEG-Based BCI Applications for Industry 4.0: Challenges and Possible Applications. Front. Hum. Neurosci. 15:705064. doi: 10.3389/fnhum.2021.705064
Received: 04 May 2021; Accepted: 20 July 2021;
Published: 13 August 2021.
Edited by:
Elizabeth B. Torres, Rutgers, The State University of New Jersey, United StatesReviewed by:
Dania Gutiérrez, National Polytechnic Institute, MexicoCopyright © 2021 Douibi, Le Bars, Lemontey, Nag, Balp and Breda. This is an open-access article distributed under the terms of the Creative Commons Attribution License (CC BY). The use, distribution or reproduction in other forums is permitted, provided the original author(s) and the copyright owner(s) are credited and that the original publication in this journal is cited, in accordance with accepted academic practice. No use, distribution or reproduction is permitted which does not comply with these terms.
*Correspondence: Khalida Douibi, a2hhbG91ZGRvdWliaUBnbWFpbC5jb20=; Gabrièle Breda, Z2FicmllbGUuYnJlZGFAYWx0cmFuLmNvbQ==
Disclaimer: All claims expressed in this article are solely those of the authors and do not necessarily represent those of their affiliated organizations, or those of the publisher, the editors and the reviewers. Any product that may be evaluated in this article or claim that may be made by its manufacturer is not guaranteed or endorsed by the publisher.
Research integrity at Frontiers
Learn more about the work of our research integrity team to safeguard the quality of each article we publish.