- 1Research Institute of Foreign Languages, Beijing Foreign Studies University, Beijing, China
- 2National Research Centre for Foreign Language Education, Beijing Foreign Studies University, Beijing, China
- 3Artificial Intelligence and Human Languages Lab, Beijing Foreign Studies University, Beijing, China
In discourse comprehension, we need to draw inferences to make sense of discourse. Previous neuroimaging studies have investigated the neural correlates of causal inferences in discourse understanding. However, these findings have been divergent, and how these types of inferences are related to causal inferences in logical problem-solving remains unclear. Using the activation likelihood estimation (ALE) approach, the current meta-analysis analyzed 19 experiments on causal inferences in discourse understanding and 20 experiments on those in logical problem-solving to identify the neural correlates of these two cognitive processes and their shared and distinct neural correlates. We found that causal inferences in discourse comprehension recruited a left-lateralized frontotemporal brain system, including the left inferior frontal gyrus, the left middle temporal gyrus (MTG), and the bilateral medial prefrontal cortex (MPFC), while causal inferences in logical problem-solving engaged a nonoverlapping brain system in the frontal and parietal cortex, including the left inferior frontal gyrus, the bilateral middle frontal gyri, the dorsal MPFC, and the left inferior parietal lobule (IPL). Furthermore, the pattern similarity analyses showed that causal inferences in discourse understanding were primarily related to the terms about language processing and theory-of-mind processing. Both types of inferences were found to be related to the terms about memory and executive function. These findings suggest that causal inferences in discourse understanding recruit distinct neural bases from those in logical problem-solving and rely more on semantic knowledge and social interaction experiences.
Introduction
Discourse is a unit of language larger than a single sentence, which is mainly used for communication in speech or writing. In discourse understanding, people often need to draw inferences to build semantic coherence and to make sense of discourse (Van Dijk and Kintsch, 1983; Kintsch, 1988). Although the internal relations between the sentences or clauses within a discourse could be different, discourse comprehension has been found to mainly rely on inferring causality (Schank, 1975; Warren et al., 1979; Graesser and Clark, 1985; Keenan et al., 1990; Singer, 1994). Such causally relevant inferences, so-called causal inferences, allow the hearers and readers to supply the causes or effects that are not overt in the discourse and to establish the links of events or information between one utterance and another (van den Broek, 1990, 1994). Thus, making causal inferences is essential for daily communication in both spoken and written language.
In the past few decades, previous studies have investigated the cognitive and neural mechanisms of causal inferences across sentences during discourse comprehension using behavioral methods and neuroscience tools such as functional MRI (fMRI) and PET. Behavioral methods were adopted to explore various types of inference processes during real-time discourse comprehension, including predictive inferences (Calvo and Castillo, 2001; Calvo et al., 2001), bridging inferences (Sansosti et al., 2013), and elaborative inferences (Calvo et al., 2003). The results revealed that individuals could generate inferences online by activating and integrating textual information and background knowledge. In addition, substantial research examined the individual factors affecting inferential processing during text reading, such as comprehension skills (e.g., Hawelka et al., 2015), vocabulary knowledge (Calvo et al., 2003; Calvo, 2005), and working memory capacity (Calvo, 2005; Yeari, 2017).
Neuroimaging studies employing fMRI and PET techniques investigated the neural activities of causal inferences during discourse comprehension. One line of studies has explored inferential processing by comparing the stories with implicit causality to the stories with explicit causality (e.g., Kuperberg et al., 2006; Virtue et al., 2006, 2008; Siebörger et al., 2007; Mason and Just, 2011; Kim et al., 2012; Prado et al., 2015). For example, Kuperberg et al. (2006) conducted an fMRI experiment to measure the localization of neural activity-mediating causal inferences when a sentence was highly causally related, intermediately related, or unrelated to its preceding contexts. When compared to a highly related condition, the sentences intermediately related to the preceding contexts elicited increasing neural activities within the bilateral inferior frontal gyri (IFG), bilateral inferior parietal lobules (IPLs), left middle frontal gyrus (MFG), left middle temporal gyrus (MTG), and medial prefrontal cortex (MPFC). These results suggested that causal inferential processing may engage a semantic activation, retrieval, selection, and integration from the long-term memory during discourse comprehension. Virtue et al. (2006, 2008) aurally presented explicitly or implicitly stated inference scenarios to the participants and compared the differences of neural activity in the earlier (i.e., the verb that implied the inference) and later (the coherence break) time points. They confirmed the critical roles of the bilateral temporal cortex and found that the right superior temporal gyrus (STG) is particularly involved in early inferential processing while the left STG is particularly recruited in later inferential processing. Mason and Just (2011) distinguished different inference contents and revealed that a set of brain regions, including the MPFC, bilateral IFG, left posterior STG, and bilateral anterior temporal lobes, form a general inference network, while the right temporoparietal junction, as a theory-of-mind brain region, is involved in the inferences concerning others' intentions but not in physical inferences. Another line of studies on causal inferences has investigated elaborative or bridging inferential processing by directly comparing coherent discourse with incoherent discourse (e.g., Robertson et al., 2000; Ferstl and von Cramon, 2001, 2002; Kuperberg et al., 2006; Siebörger et al., 2007; Yarkoni et al., 2008; Prat et al., 2011). These studies also got results that were inconsistent and hard to collate. Concerning unrelated sentences, a coherent discourse may elicit activations in MPFC and the posterior cingulate cortex (Ferstl and von Cramon, 2001, 2002), activations in the frontoparietal areas related to executive functions (Siebörger et al., 2007), or activations in frontotemporal regions for situation model maintenance (Yarkoni et al., 2008). Different from the above two lines of studies, Chow et al. (2008) looked into the neural correlates of causal inferences by manipulating the reading goal (i.e., reading-with-prediction condition or reading-without-prediction condition). Comparing the reading-with-prediction condition to the reading-without-prediction condition, they found that explicitly predictive inferential processing elicited increased hemodynamic activity in the left anterior PFC and left anterior ventral IFG, reflecting the phase of coherence evaluation and the process of drawing strategic inferences. To sum up, previous studies found that the brain regions in both left and right hemispheres may be involved in the inference generation during discourse comprehension, especially the regions in the frontal and temporal lobes, but results for which region is involved in the process have been divergent.
The causally relevant inferences during discourse comprehension were often viewed as a problem-solving process (van den Broek, 1990) and were conceived by using an analogy to logical thinking (Suvorova and Polyakova, 2018). Meanwhile, considering the interplay between thought and language, an important view is that thinking is deeply rooted in the language (Polk and Newell, 1995). Previous studies have demonstrated that logical inferences relied heavily on language processing (Goel et al., 1998; Goel and Dolan, 2004). Thus, being one type of inferences outside the domain of discourse comprehension, causal inferences in logical problem-solving (hereafter “logical inferences”) seem to share some component processes with causal inferences in language understanding (hereafter “discourse inferences”). In this regard, we wondered whether the two types of inferences share some or even all cognitive processes.
Logical inferences are considered as a cognitive activity of evaluating arguments (Goel et al., 2004). On the basis of human rationality, the neural correlates of logical inferences have been investigated by using many neuroimaging studies. In these studies, participants were generally required to read sets of arguments rather than coherent texts and to evaluate whether the arguments are valid. Prado et al. (2011) performed a quantitative meta-analysis of 28 fMRI and PET studies on deductive reasoning. The results revealed that the studies consistently reported activations in the left frontoparietal network and in the left basal ganglia. Most recently, the meta-analysis of the conditional and syllogistic inferences including 32 original neuroimaging experiments, also showed that such causal inferences are associated with a left-lateralized widespread pattern of activations, which included the left (and right) IFG, left (and right) MFG, left IPL, left superior frontal gyrus, and MPFC (Wertheim and Ragni, 2020). Although the evidence from both abovementioned groups of studies has been accumulated, few studies have directly compared the neural correlates of discourse inferences and logical inferences. Hence, it is still an open question whether the neural bases of these two cognitive processes are similar or distinct.
In the current study, we aimed to investigate the shared and distinct neural bases of discourse inferences and logical inferences. We used meta-analytic methods to investigate the neural correlates of discourse inferences and logical inferences because meta-analyses could increase the statistical power and help to detect robust effects across the experiments. To address the aim, we first computed the map of each cognitive process using the activation likelihood estimation (ALE) method. In order to make data more comparable across those two lines of studies, we excluded the studies that used non-verbal stimuli as materials for logical inferences. Then, we compared them to investigate their conjunction and their respective specific activations. In addition, we conducted neural pattern similarity analyses to further identify the neurocognitive subprocesses underlying discourse inferences and logical inferences using a large-scale meta-analytic database. Based on the previous studies, we hypothesized that the discourse inferences may recruit the left frontotemporal network (e.g., Ferstl and von Cramon, 2001, 2002; Siebörger et al., 2007) and might even activate the regions in the right frontotemporal network (e.g., Kuperberg et al., 2006; Virtue et al., 2006, 2008; Mason and Just, 2011) while the logical inferences may engage the activations of a left-lateralized frontoparietal network (Prado et al., 2011; Wertheim and Ragni, 2020). Additionally, these two types of inferential processing may share the neural activity in IFG and MPFC.
Materials and Methods
Study Selection
The study selection process is presented in a flow diagram in Figure 1 and the definitions of key terms are provided in Table 1. We conducted an online literature search in the Web of Science and PubMed databases to acquire the studies published between January 1980 and March 2020 (last search on March 27, 2020). The function of the advanced search was used to detect the target literature. The primary literature was collected by using the following strategies: Topic = [(“causal*” OR “predict*” OR “bridg*”) AND (“inferen*” OR “reasoning”) AND (“fMRI” OR “neuroimaging” OR “functional magnetic imaging” OR “functional MRI” OR “functional MRI” OR “functional imaging”)]. This search generated 986 articles in the Web of Science database and 734 articles in the PubMed database. In order to avoid omission, we additionally considered the studies in the relevant meta-analyses (Prado et al., 2011; Yang et al., 2019; Wertheim and Ragni, 2020) and reviews (e.g., Barbey and Patterson, 2011; Virtue and Sundermeier, 2016; Brascamp et al., 2018; Wang et al., 2018), and the studies that cited those meta-analyses and reviews.
After deduplication, we removed the articles that were not written in English and the articles that were obviously irrelevant according to their title and abstract. Then, three of the present authors (JL, WW, and ZW) gathered relevant information from the remaining 196 studies, including the number of participants, research techniques, the type of tasks, the type of stimuli, the type of contrasts, and the standard space in which coordinates were reported. They checked each other's work, and they discussed and resolved a few disagreements. The studies included in the current meta-analysis must meet the following requirements: (1) the technology used was task-induced fMRI or PET; (2) the experiment recruited at least one group of healthy adult participants; (3) the stimulus materials were in the participants' first languages; and (4) peak coordinates were presented in the standard Talairach space or the standard Montreal Neurological Institute (MNI) space. We further excluded studies for their lack of univariate analysis at the whole-brain level (i.e., Mason and Just, 2004), their low-level baseline conditions (i.e., Knauff et al., 2003; Brunetti et al., 2014; Porcaro et al., 2014; Smith et al., 2014, 2015), or their usage of pseudoword or nonword (i.e., Prado and Noveck, 2007; Reverberi et al., 2010, 2012; Hearne et al., 2015). Moreover, studies on nonliteral language comprehension, such as conversational implicature or indirect speech, were not included in this meta-analysis (e.g., van Ackeren et al., 2012; Jang et al., 2013; Feng et al., 2017) because the processing involved in these complex pragmatic phenomena goes beyond inferring the causal structure of the literal meaning (see Bohrn et al., 2012; Rapp et al., 2012 for reviews). In addition, we focused on the comparison between an inference and a non-/weak-inference across the sentences; therefore, the studies that did not adopt sentence-level stimuli (i.e., Satpute et al., 2005; Wende et al., 2012) or did not include an appropriate contrast (i.e., Parsons and Osherson, 2001; Ferstl et al., 2005; Reverberi et al., 2007; Egidi and Caramazza, 2014, 2016) were excluded. Finally, the selected studies were classified as discourse inferences and logical inferences according to their verbal materials. Specifically, a study will fall into the logical inference group if the status of its stimuli depends on explicit logical connectors studied in elementary logic (e.g., if…then, or, not) while a study will fall into the discourse inference group if the inferential processing does not necessarily rely on the sentential connectives but mainly requires to build a semantic representation.
Overall, the current meta-analysis included 19 published neuroimaging studies on the neural substrates of discourse inferences (see Table 2) and 20 experiments reported in the 19 published articles on the neural substrates of logical problem-solving (see Table 3). The studies of the discourse comprehension group suggest various types of inferences, such as bridging inferences (e.g., Kuperberg et al., 2006; Virtue et al., 2008; Mason and Just, 2011; Kim et al., 2012), predictive inferences (e.g., Chow et al., 2008; Jin et al., 2009), and elaborative inferences (e.g., Yarkoni et al., 2008). Similarly, the 20 experiments of the logical reasoning group also covered various types of reasoning, such as inductive reasoning (e.g., Goel et al., 1997; Goel and Dolan, 2004), conditional reasoning (e.g., Canessa et al., 2005, 2014; Monti et al., 2007; Coetzee and Monti, 2018), and syllogistic reasoning (e.g., Goel et al., 1998; Jia et al., 2009; Rodriguez-Moreno and Hirsch, 2009). The studies also differed in many aspects, including the ways of stimulus presentation (i.e., visual or auditory) and response (i.e., reading or listening without an immediate response, or a response by pressing keys or buttons), neuroimaging methods (i.e., PET or fMRI), templates (i.e., MNI or Talairach), and software packages used for preprocessing and statistical analysis.
Meta-Analytic Approach
The GingerALE 3.0.2 software (http://www.brainmap.org) was used for conducting the meta-analysis of functional neuroimaging data (Eickhoff et al., 2009, 2012; Turkeltaub et al., 2012). The ALE approach is suitable for carrying out meta-analyses, for it treats the reported foci not as single points, but as centers for the three-dimensional (3D) Gaussian probability distributions that reflect the reliability of neuroimaging results (Eickhoff et al., 2009). For each experiment, we calculated a modeled activation (MA) map using the ALE method, which contained the Gaussian probability distributions of all reported foci of all contrasts-of-interest in this particular experiment. In the current meta-analysis, all included experiments were obtained from the different samples. The full-width half-maximum (FWHM) values of Gaussian distributions were determined by using the number of participants. Next, separate MA maps for each group of studies were combined into a single ALE map, and the ALE values were computed on a voxel-by-voxel basis (Eickhoff et al., 2012). The value of p of a particular ALE value was calculated by using the random-effects inference.
Meta-Analysis Procedure
Reported foci in the contrasts-of-interest of each included experiment were extracted by two of the current authors independently (WW and WF). They re-examined and resolved rare disagreements. In total, there were 217 foci extracted from the studies on discourse understanding and 360 foci from the studies on logical problem-solving. All activation coordinates reported in the Talairach space were first converted into MNI space by using the GingerALE converter tool (Lancaster et al., 2007; Laird et al., 2010). Next, we separately performed an ALE analysis for each group of studies (discourse understanding vs. logical problem-solving) and obtained their respective ALE map. Based on the recommendations of Eickhoff et al. (2012, 2016), we evaluated the results of these single data set analyses using an uncorrected voxel-level threshold at p < 0.001 and a cluster-level threshold at q < 0.05 family-wise error (FWE) corrected. We applied 5,000 threshold permutations to derive cluster-level thresholds.
To determine the shared and separated neural correlates of discourse inferences and logical inferences, we conducted contrast analyses between the two groups of studies. Contrast analyses computed the voxel-wise difference between two thresholded ALE images calculated from the single data set analyses (Laird et al., 2005; Eickhoff et al., 2011). The conjunction was created by using the voxel-wise minimum value of these ALE images. We subtracted one input image from the other to generate two ALE contrast images. Considering the balance between sensitivity and specificity (Eickhoff et al., 2012), in the contrast data set analyses, we conducted a threshold of uncorrected p < 0.01 after 10,000 permutations and excluded the results with a volume size smaller than 200 mm3.
Pattern Similarity Analyses
We used the Neurosynth Image Decoder (http://neurosynth.org/decode; Yarkoni et al., 2011) to further quantify the neural pattern similarity between the ALE maps and the patterns associated with the cognitive terms obtained from previous neuroimaging studies. More than 14,000 published studies have been included in the Neurosynth database by the time we conducted the decoding (December 2020). The reverse inference z map for each of the 1,307 terms has been automatically generated by using the Neurosynth database. The Pearson correlation coefficients (r) between the unthresholded ALE map and the reverse inference map for each term were calculated. The r values reflect the spatial correlation across voxels between the two maps. All maps were restricted by using a gray matter mask.
Results
The current study analyzed 19 experiments on discourse inferences and 20 experiments on logical inferences to identify the neural correlates of these two cognitive processes, as well as the shared and distinct neural correlates of them. For the discourse comprehension group, participants were 285 healthy adults (~51% females) while for the logical problem group, participants were 264 healthy adults (41% females). All participants were right-handed with the exception of one study (Mason and Just, 2011), which did not report participants' handedness.
Single Data Set Analyses
The single data set analysis of discourse inferences revealed that six clusters of activations achieved significant convergence across the experiments (Figure 2A and Table 4). The pattern of activations showed left-hemisphere laterality. The largest cluster was observed in the pars triangularis and opercularis of the left IFG extending to the left precentral gyrus [Brodmann area (BA) 45/44/48]. Another cluster in the left IFG was found in the pars orbitalis extending to the pars triangularis (BA 47/45). Two other clusters were detected in the middle (BA 21) and posterior (BA 37/21) parts of the left MTG, respectively. Finally, two more clusters were in MPFC (BA 10 and 9).
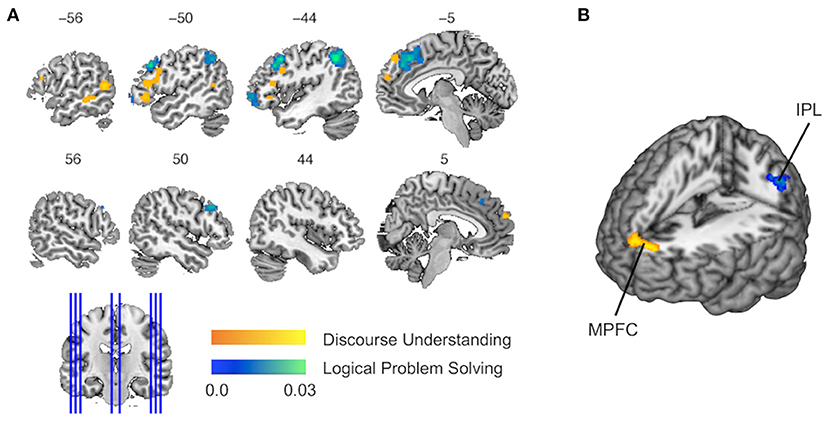
Figure 2. Results of single data set analyses and contrast data sets analysis. (A) The activation likelihood estimation (ALE) maps show the significant activations associated with discourse inferences (in orange) and logical inferences (in blue). (B) The contrast map shows the activation for the contrast of discourse inferences vs. logical inferences (in orange) and the activation for the reverse contrast (in blue). MPFC, medial prefrontal cortex; IPL, inferior parietal lobule.
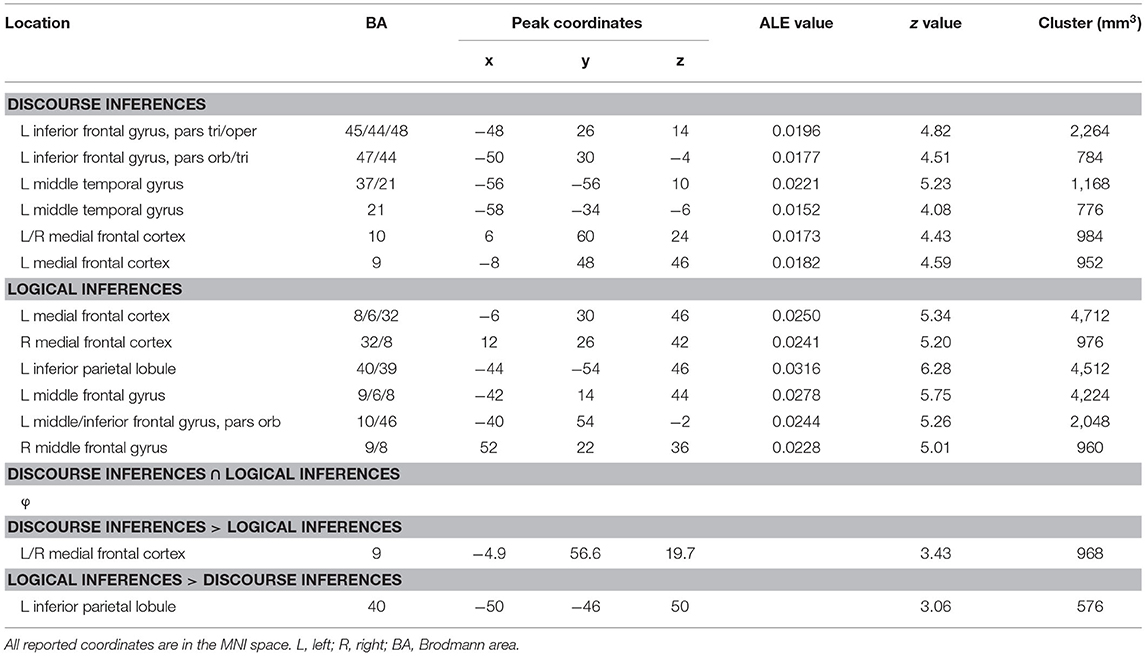
Table 4. Regions are consistently activated across the experiments on discourse inferences and logical inferences.
The single data set analysis of logical inferences revealed six clusters of activations, which achieved significant convergence across the experiments (Figure 2A and Table 4). The largest cluster and another cluster located in MPFC extending to the anterior cingulate cortex (BA 8/32/6). The second largest cluster was found in the left IPL (BA 40/39). Two other clusters were observed in MFG extending to the precentral gyrus (BA 9, 8, and 6) bilaterally. One more cluster covered the pars orbitalis of the left MFG extending to IFG (BA 46/10).
Contrast Data Sets Analysis
The contrast data sets analysis did not reveal any significant activations that was commonly recruited by discourse inferences and logical inferences. When conducting a liberal threshold (p < 0.05 and volume > 50 mm3), we still observed no activation.
Compared to logical inferences, discourse inferences elicited a significant activation in MPFC (BA 9; see Figure 2B in orange, Table 4) whereas, compared to discourse inferences, logical inferences more strongly activated a brain region in the left IPL (BA 40; see Figure 2B in blue, Table 4).
Pattern Similarity Analyses
To further investigate the cognitive processes that supported discourse inferences and logical inferences, we calculated the spatial correlations between the ALE map and the neural pattern for each term generated by using the Neurosynth database (see Figure 3). The mean r value was 0.009 (± SD = 0.061) for discourse inferences and 0.005 (±0.050) for logical inferences. The results showed that the discourse inferences were primarily related to the terms about language processing (e.g., sentence, comprehension, and language) and theory-of-mind processing (e.g., theory mind, mind, and mental states). Meanwhile, this process was also related to the terms associated with memory such as retrieval (r = 0.22), memory (0.143), and working memory (0.142), and executive function such as demand (0.217), task (0.140), and tasks (0.134). In contrast, logical inferences were mainly related to the terms about executive function (e.g., task, tasks, and demands) and memory (e.g., working memory, retrieval, and memory). In addition, this process was also related to the terms associated with language comprehension, like semantics (0.161), word (0.157), and language (0.129). These findings indicated that both discourse inferences and logical inferences need the support of the cognitive processes associated with memory, executive function, and language, but their extent of participation in the two types of inferential processing was different. Furthermore, discourse inferences are additionally associated with theory-of-mind processing.
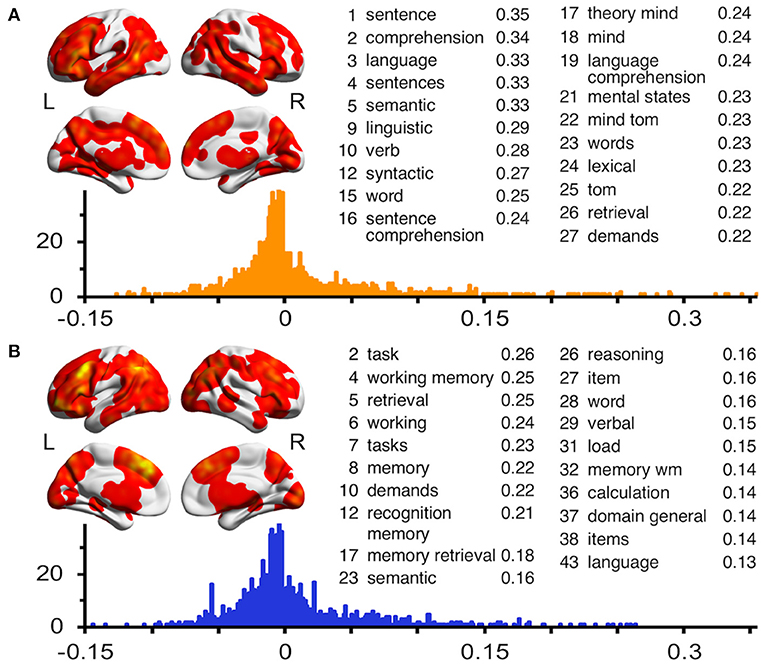
Figure 3. Results of neural pattern similarity analyses using the ALE map of discourse inferences (A) and logical inferences (B). The unthresholded ALE maps are visualized on a surface rendering of the smoothed ICBM152 template. The histograms represent the frequency distributions of the Pearson's r between the ALE map and the z map for each term in the Neurosynth database. For each ALE map, the embedded table lists the most highly correlated cognitive or psychological terms ranked by r values. Terms associated with brain regions are not included in these tables.
Discussion
The aims of the present meta-analysis study were to identify the neural substrates of discourse inferences and to compare it with those neural substrates of logical inferences. Using the ALE approach (Eickhoff et al., 2009, 2012), we analyzed 19 neuroimaging experiments on discourse inferences and 20 experiments on logical inferences and separately identified the commonly activated brain regions across the experiments for each process. Our study demonstrates that discourse inferences mainly engage a left-lateralized frontotemporal brain system whereas logical inferences rely on a non-overlapping brain system in the frontal and parietal cortex. The pattern similarity analyses also revealed that the subprocesses involved in the two inferential processing are different.
Causal Inferences in Discourse Understanding
Previous studies on discourse comprehension have shown various results of neural correlates underlying causal inferences. This meta-analytic study reveals that the left IFG, left middle and posterior MTG, and bilateral MPFC are congruently activated by using discourse inferences across the experiments.
In the stage of study selection, we adopted a relatively strict criterion and excluded the studies using low-level baseline conditions such as fixation presentation. After subtracting adequate baseline conditions, discourse inferences still activated the core brain regions of language processing (i.e., IFG and MTG), which is congruent with a previous meta-analysis based on eight studies (Yang et al., 2019). Specifically, the left IFG was well-known as a core region of syntactic and semantic processing such as grammatical categorization (Ni et al., 2000), syntax parsing (Friederici and Kotz, 2003), semantic information retrieval (Wagner et al., 2001), semantic selection (Thompson-Schill et al., 1997; Kan and Thompson-Schill, 2004), and semantic unification (Hagoort et al., 2004; Zhu et al., 2012) while the left MTG was found to be associated with semantic processing (Xu et al., 2005; Binder et al., 2009; Visser and Lambon Ralph, 2011), especially a fine semantic activation that supports the processing of semantic integration [see Jung-Beeman (2005) for a review]. Based on the construction-integration model, discourse comprehension involves the process of constructing a discourse representation, which mainly relies on a conceptual or propositional net (Kintsch, 1988). When individuals need to draw discourse inferences, associative information will be elaborated and added to the existing discourse representation. Meanwhile, plenty of studies have demonstrated that individuals' long-term memory and working memory play important roles in discourse inferences (Keenan et al., 1984; Myers et al., 1987; Myers and Duffy, 1990). Thus, in the current study, the congruently enhanced activations in the left IFG and MTG during discourse inferences may reflect the processing of retrieving and selecting additional information from a semantic memory, and the processing of integrating them together to construct higher-order discourse representations in the working memory. Being consistent with this idea, the pattern similarity analysis revealed that discourse inferences would recruit the processing of language and memory.
Similarly, two clusters of activations within the anterior and dorsal MPFC (BA 10/9) also contribute to discourse inferences. MPFC has been reported in association with various high-order cognitive processes such as theory-of-mind processing (Van Overwalle and Baetens, 2009; Koster-Hale and Saxe, 2013), coherence evaluation (Chow et al., 2008), and inductive reasoning (Ferstl and von Cramon, 2002; Siebörger et al., 2007). Previous studies on discourse comprehension have suggested that the engagement of MPFC reflects controlled, strategic, and higher-order inferential processes (Ferstl and von Cramon, 2001, 2002; Kuperberg et al., 2006). We consider this idea as the most reasonable explanation of the role of MPFC in discourse inferences. However, as shown in the present pattern similarity analysis, discourse inferences may engage the theory-of-mind-related inferential processing. This may be caused by the fact that we did not distinguish the contents of causal inferences; that is, this meta-analysis included the studies involving both physical and intentional inferences (e.g., Fletcher et al., 1995; Mason and Just, 2011).
Causal Inferences in Logical Problem-Solving
This meta-analysis found that a frontoparietal network, including the left IFG, bilateral MFG, MPFC (extending to the anterior cingulate cortex), and left IPL, would be consistently recruited by using logical inferences across the studies. In this study, we included neuroimaging experiments on verbal and content-based causal inferences across inductive and deductive reasoning, conditional and syllogistic inferences. Although the current meta-analysis is different from the previous meta-analyses in study selection, the results are essentially consistent with the previous findings of logical inferences (Prado et al., 2011; Wertheim and Ragni, 2020). Thus, our results further confirmed the association between verbal logical reasoning and activity in the left-lateralized frontoparietal network.
The lateral prefrontal cortex, including IFG and MFG, is related to working memory load (Rypma and D'Esposito, 1999; Fletcher and Henson, 2001; Jia et al., 2009). Furthermore, previous studies have demonstrated that content-based inferences additionally require the retrieval and application of world knowledge (Goel et al., 2000; Wertheim and Ragni, 2020). Thus, in logical problem-solving, the engagement of IFG and MFG reflects the processing of retrieving, maintaining, and refreshing information in memory. Meanwhile, IPL has been known to be linked with domain-general executive functions (Ye and Zhou, 2009a,b; Duncan, 2010). Such explanations are confirmed by using the results of the pattern similarity analysis, which suggested that the activation pattern of logical inferences is spatially correlated to that of memory and executive control.
Dissociation of Causal Inferences in Discourse Understanding and Logical Problem-Solving
In this study, the single-study analyses showed that discourse inferences and logical inferences elicit basically different brain activation patterns. Moreover, the conjunction analysis between the two data sets did not yield any significant overlapping in terms of brain activation, further strengthening this observation. More specifically, although both discourse inferences and logical inferences recruit activations in the left lateral and MPFC (IFG and MPFC), these activations did not overlap.
First, as shown in our results, discourse inferences activated more posterior and ventral parts of the left lateral prefrontal cortex while logical inferences activated more anterior and dorsal parts (see Figure 2A). A similar pattern of results has been found by using a previous meta-analysis on analogical reasoning (Hobeika et al., 2016). In their work, the contrast of semantic vs. visuospatial analogy revealed an activation located in the posterior and ventral part of the left IFG while the contrast of visuospatial vs. semantic analogy revealed clusters in the anterior region of the left IFG and MFG, dorsal to the semantic analogy region. Moreover, Monti et al. (2009) also reported similar results that linguistic inferences recruited an activity in the vicinity of Broca's area (left BA 44/45) while logical inferences recruited an activity in the rostrolateral section of the left middle and IFG (BA 10/47). According to the assumptions of hierarchical models, the organization of the prefrontal cortex along the rostro-caudal axis is based on the content of a working memory representation (Badre, 2008; Badre and D'Esposito, 2009; Christoff et al., 2009). More specifically, more anterior and dorsal parts of the lateral prefrontal cortex support progressively more abstract representations in working memory while more posterior and ventral parts support more concrete representations. Thus, we believed that the activity in the left lateral frontal cortex is responsible for constructing concrete representations in working memory during discourse inferences, but for constructing abstract representations during logical inferences. The essentiality of representations has been stressed by using both classic models of discourse inferences [e.g., the construction-integration model (Kintsch, 1988), the constructionist theory (Singer, 1994)], and models of logical inferences [e.g., the mental model theory of reasoning (Johnson-Laird, 1999)].
Second, our study showed that both discourse inferences and logical inferences activated the clusters in the dorsal MPFC, but the activation cluster of discourse understanding is anterior to a larger activation cluster of logical problem-solving. Previous findings of the neural bases of conflict control demonstrated that distinct brain regions in dorsal MPFC respond to conflicts arising at different information inputs (Jost et al., 2012). Hence, the activity in the dorsal MPFC may reflect the selection and coordination of multiple task demands in discourse inferences and logical inferences. In discourse comprehension, this region may be central to the establishment of coherence under uncertainty (Ferstl and von Cramon, 2001; 2002). Meanwhile, in logical problem-solving, this region may enable individuals to convert premises into conclusions when multiple rules are available (Monti et al., 2009; Rodriguez-Moreno and Hirsch, 2009). In addition, the bilateral anterior portions of MPFC are exclusively recruited by using discourse inferences. This region may be involved in the connection between conceptual information and their affective or social meaning (see Roy et al., 2012 for a review). These results suggested that the affective and social information might be more involved in discourse inferences than in logical inferences.
Overall, in the current study, we found that the cognitive processes of memory and executive control were involved in both discourse inferences and logical inferences whereas the neural bases underlying the two types of causal inferences were completely separate: the discourse inferences relied more on language processing and theory-of-mind-related inferential processing. In combination with these findings, we speculated that discourse inferences and logical inferences may recruit different subcomponents of the cognitive processes associated with memory and executive control. The dissociation of the two types of causal inferences originates from different inference contents. In discourse inferences, the construction of a discourse representation in the working memory mainly depends on one's semantic knowledge and social interaction experience. Relatively, although logical inferences are also based on verbal stimuli in the current meta-analysis, such inferences rely more on abstract representations in the working memory.
Notably, by directly comparing linguistic inferences and logical inferences in the same population, Monti et al. (2009) revealed that the activations of linguistic inferences and logical inferences overlapped in inferior/middle frontal gyri and superior/IPLs, which has been reported to be associated with the working memory and executive functions (Rypma and D'Esposito, 1999). Although we found that the cognitive processes of memory and executive control were involved in both discourse inferences and logical inferences, our meta-analysis did not identify any co-activation between both types of inferences. The inconformity between our results and the results of Monti et al. (2009) could be caused by several reasons. First, this meta-analysis incorporates a number of experiments, which exhibit heterogeneity in various aspects. With the exception of Monti et al. (2009), the studies included in the current meta-analysis do not investigate both discourse inferences and logical inferences, and each group of studies included several types of inferences as we mentioned in the Section “Method.” Given that, it is hard for the current meta-analysis to detect the conjunction that only appears when a certain set of conditions are satisfied. Likewise, since meta-analyses are limited to the studies available, we could not rule out the possibility that the lack of significant co-activations is due to the differences in the aspects of the subject, task, etc., rather than differences between discourse inferences and logical inferences. In addition, with the limited number of available studies and the hard-to-control variation within each group of studies, it should also be considered that this absence of co-activations may be caused by the fact that the statistical power is insufficient. Thus, more data points need to be accumulated in order to obtain objective and accurate results in the future. Future research is expected to investigate what factors would influence the activity pattern in the inferior/middle frontal cortex and superior/IPLs during discourse inferences and logical inferences.
Conclusion
Our meta-analysis identified the neural correlates of both discourse inferences and logical inferences. Discourse inferences recruit a left-lateralized frontotemporal brain system, including the left IFG, left middle and posterior MTG, and bilateral MPFC while logical inferences engage a frontoparietal brain system, consisting of the left IFG, bilateral MFG, dorsal MPFC, and left IPL. Furthermore, this meta-analysis contributes to the question of whether the two types of inferential processing have shared neural bases by revealing that they rely on the activities in separate brain regions. Considering that the cognitive processes of memory and executive control are involved in both inferential processes, the dissociation suggests that discourse inferences and logical inferences use the information from different sources to construct mental representations in the working memory. The current meta-analysis identifies the neural correlates that are consistently activated by using discourse inferences and logical inferences across the studies. These findings provide reliable quantitative evidence and extend current knowledge for the neural correlates underlying the two types of causal inferences. The dissociation of discourse inferences and logical inferences may remind that the analogy between the two should be carefully considered. In addition, this meta-analysis confirms the important roles of the working memory and executive function in both discourse inferences and logical inferences, which may be of assistance to build and extend cognitive models to account for causal inferences.
Data Availability Statement
The raw data that support the conclusions of this study are available on request from the corresponding authors, without undue reservation.
Author Contributions
WF: conceptualization, methodology, formal analysis, writing—original draft, and visualization. WW: formal analysis, investigation, and writing—original draft. JL and ZW: investigation and writing—original draft. LT: writing—original draft and visualization. LF: writing—review and editing. All authors contributed to the article and approved the submitted version.
Funding
This study was supported by the fellowship of China Postdoctoral Science Foundation (2020M670212) and the Fundamental Research Funds for the Central Universities (2020JJ017) to Dr. WF, and the Social Science Foundation of China (18BYY088) and the First-Class Disciplines Project of Beijing Foreign Studies University (2020SYLZDXM040) to LF, and the Social Science Planning Fund Project of Shandong Province (12CQSJ03) to LS.
Conflict of Interest
The authors declare that the research was conducted in the absence of any commercial or financial relationships that could be construed as a potential conflict of interest.
Acknowledgments
We thank Dr. Jiaxing Jiang for his assistance and suggestions. The authors also thank the two anonymous reviewers for their constructive comments on an earlier version of the manuscript.
References
Badre, D. (2008). Cognitive control, hierarchy, and the rostro-caudal organization of the frontal lobes. Trends Cogn. Sci. 12, 193–200. doi: 10.1016/j.tics.2008.02.004
Badre, D., and D'Esposito, M. (2009). Is the rostro-caudal axis of the frontal lobe hierarchical?. Nat. Rev. Neurosci. 10, 659–669. doi: 10.1038/nrn2667
Barbey, A. K., and Patterson, R. (2011). Architecture of explanatory inference in the human prefrontal cortex. Front. Psychol. 2:162. doi: 10.3389/fpsyg.2011.00162
Binder, J. R., Desai, R. H., Graves, W. W., and Conant, L. L. (2009). Where is the semantic system? A critical review and meta-analysis of 120 functional neuroimaging studies. Cereb. Cortex 19, 2767–2796. doi: 10.1093/cercor/bhp055
Bohrn, I. C., Altmann, U., and Jacobs, A. M. (2012). Looking at the brains behind figurative language—a quantitative meta-analysis of neuroimaging studies on metaphor, idiom, and irony processing. Neuropsychologia 50, 2669–2683. doi: 10.1016/j.neuropsychologia.2012.07.021
Brascamp, J., Sterzer, P., Blake, R., and Knapen, T. (2018). Multistable perception and the role of frontoparietal cortex in perceptual inference. Annu. Rev. Psychol. 69, 77–103. doi: 10.1146/annurev-psych-010417-085944
Brunetti, M., Perrucci, M. G., Di Naccio, M. R., Ferretti, A., Del Gratta, C., Casadio, C., et al. (2014). Framing deductive reasoning with emotional content: An fMRI study. Brain Cogn. 87, 153–160. doi: 10.1016/j.bandc.2014.03.017
Calvo, M. G. (2005). Relative contribution of vocabulary knowledge and working memory span to elaborative inferences in reading. Learn. Individ. Differ. 15, 53–65. doi: 10.1016/j.lindif.2004.07.002
Calvo, M. G., and Castillo, M. D. (2001). Bias in predictive inferences during reading. Discourse Process. 32, 43–71. doi: 10.1207/S15326950DP3201_03
Calvo, M. G., Estévez, A., and Dowens, M. G. (2003). Time course of elaborative inferences in reading as a function of prior vocabulary knowledge. Learn. Instr. 13, 611–631. doi: 10.1016/S0959-4752(02)00055-5
Calvo, M. G., Meseguer, E., and Carreiras, M. (2001). Inferences about predictable events: Eye movements during reading. Psychol. Res. 65, 158–169. doi: 10.1007/s004260000050
*Canessa, N., Gorini, A., Cappa, S. F., Piattelli-Palmarini, M., Danna, M., Fazio, F., et al. (2005). The effect of social content on deductive reasoning: An fMRI study. Hum. Brain Mapp. 26, 30–43. doi: 10.1002/hbm.20114
*Canessa, N., Pantaleo, G., Crespi, C., Gorini, A., and Cappa, S. F. (2014). The impact of egocentric vs. Allocentric agency attributions on the neural bases of reasoning about social rules. Brain Res. 1581, 40–50. doi: 10.1016/j.brainres.2014.06.001
*Chow, H. M., Kaup, B., Raabe, M., and Greenlee, M. W. (2008). Evidence of fronto-temporal interactions for strategic inference processes during language comprehension. Neuroimage 40, 940–954. doi: 10.1016/j.neuroimage.2007.11.044
Christoff, K., Gordon, A. M., Smallwood, J., Smith, R., and Schooler, J. W. (2009). Experience sampling during fMRI reveals default network and executive system contributions to mind wandering. Proc. Natl. Acad. Sci. U.S.A. 106, 8719–8724. doi: 10.1073/pnas.0900234106
*Coetzee, J. P., and Monti, M. M. (2018). At the core of reasoning: Dissociating deductive and non–deductive load. Hum. Brain Mapp. 39, 1850–1861. doi: 10.1002/hbm.23979
Duncan, J. (2010). The multiple-demand (MD) system of the primate brain: Mental programs for intelligent behaviour. Trends Cogn. Sci. 14, 172–179. doi: 10.1016/j.tics.2010.01.004
Egidi, G., and Caramazza, A. (2014). Mood-dependent integration in discourse comprehension: Happy and sad moods affect consistency processing via different brain networks. Neuroimage 103, 20–32. doi: 10.1016/j.neuroimage.2014.09.008
Egidi, G., and Caramazza, A. (2016). Integration processes compared: Cortical differences for consistency evaluation and passive comprehension in local and global coherence. J. Cogn. Neurosci. 28, 1568–1583. doi: 10.1162/jocn_a_00982
Eickhoff, S. B., Bzdok, D., Laird, A. R., Kurth, F., and Fox, P. T. (2012). Activation likelihood estimation meta-analysis revisited. Neuroimage 59, 2349–2361. doi: 10.1016/j.neuroimage.2011.09.017
Eickhoff, S. B., Bzdok, D., Laird, A. R., Roski, C., Caspers, S., Zilles, K., et al. (2011). Co-activation patterns distinguish cortical modules, their connectivity and functional differentiation. Neuroimage 57, 938–949. doi: 10.1016/j.neuroimage.2011.05.021
Eickhoff, S. B., Laird, A. R., Grefkes, C., Wang, L. E., Zilles, K., and Fox, P. T. (2009). Coordinate-based activation likelihood estimation meta–analysis of neuroimaging data: A random-effects approach based on empirical estimates of spatial uncertainty. Hum. Brain Mapp. 30, 2907–2926. doi: 10.1002/hbm.20718
Eickhoff, S. B., Nichols, T. E., Laird, A. R., Hoffstaedter, F., Amunts, K., Fox, P. T., et al. (2016). Behavior, sensitivity, and power of activation likelihood estimation characterized by massive empirical simulation. Neuroimage 137, 70–85. doi: 10.1016/j.neuroimage.2016.04.072
Feng, W., Wu, Y., Jan, C., Yu, H., Jiang, X., and Zhou, X. (2017). Effects of contextual relevance on pragmatic inference during conversation: an fMRI study. Brain Lang. 171, 52–61. doi: 10.1016/j.bandl.2017.04.005
Ferstl, E. C., Rinck, M., and von Cramon, D. Y. (2005). Emotional and temporal aspects of situation model processing during text comprehension: an event-related fMRI study. J. Cogn. Neurosci. 17, 724–739. doi: 10.1162/0898929053747658
*Ferstl, E. C., and von Cramon, D. Y. (2001). The role of coherence and cohesion in text comprehension: an event-related fMRI study. Cogn. Brain Res. 11, 325–340. doi: 10.1016/S0926-6410(01)00007-6
*Ferstl, E. C., and von Cramon, D. Y. (2002). What does the frontomedian cortex contribute to language processing: coherence or theory of mind? Neuroimage 17, 1599–1612. doi: 10.1006/nimg.2002.1247
*Fletcher, P. C., Happe, F., Frith, U., Baker, S. C., Dolan, R. J., Frackowiak, R. S. J., et al. (1995). Other minds in the brain: a functional imaging study of “theory of mind” in story comprehension. Cognition 57, 109–128. doi: 10.1016/0010-0277(95)00692-R
Fletcher, P. C., and Henson, R. N. A. (2001). Frontal lobes and human memory: insights from functional neuroimaging. Brain 124, 849–881. doi: 10.1126/science.308.5718.36b
Friederici, A. D., and Kotz, S. A. (2003). The brain basis of syntactic processes: functional imaging and lesion studies. Neuroimage 20, S8–S17. doi: 10.1016/j.neuroimage.2003.09.003
*Friese, U., Rutschmann, R., Raabe, M., and Schmalhofer, F. (2008). Neural indicators of inference processes in text comprehension: an event-related functional magnetic resonance imaging study. J. Cogn. Neurosci. 20, 2110–2124. doi: 10.1162/jocn.2008.20141
*Goel, V., Buchel, C., Frith, C., and Dolan, R. J. (2000). Dissociation of mechanisms underlying syllogistic reasoning. Neuroimage 12, 504–514. doi: 10.1006/nimg.2000.0636
*Goel, V., and Dolan, R. J. (2001). Functional neuroanatomy of three-term relational reasoning. Neuropsychologia 39, 901–909. doi: 10.1016/S0028-3932(01)00024-0
*Goel, V., and Dolan, R. J. (2004). Differential involvement of left prefrontal cortex in inductive and deductive reasoning. Cognition 93, B109–B121. doi: 10.1016/j.cognition.2004.03.001
*Goel, V., Gold, B., Kapur, S., and Houle, S. (1997). The seats of reason? An imaging study of deductive and inductive reasoning. NeuroReport 8, 1305–1310 doi: 10.1097/00001756-199703240-00049
*Goel, V., Gold, B., Kapur, S., and Houle, S. (1998). Neuroanatomical correlates of human reasoning. J. Cogn. Neurosci. 10, 293–302. doi: 10.1162/089892998562744
*Goel, V., Makale, M., and Grafman, J. (2004). The hippocampal system mediates logical reasoning about familiar spatial environments. J. Cogn. Neurosci. 16, 654–664. doi: 10.1162/089892904323057362
*Goel, V., Stollstorff, M., Nakic, M., Knutson, K., and Grafman, J. (2009). A role for right ventrolateral prefrontal cortex in reasoning about indeterminate relations. Neuropsychologia 47, 2790–2797. doi: 10.1016/j.neuropsychologia.2009.06.002
Graesser, A. C., and Clark, L. F. (1985). Structures and Procedures of Implicit Knowledge. Norwood, NJ: Ablex Publishing Corp.
Hagoort, P., Hald, L., Bastiaansen, M., and Petersson, K. M. (2004). Integration of word meaning and world knowledge in language comprehension. Science 304, 438–441. doi: 10.1126/science.1095455
Hawelka, S., Schuster, S., Gagl, B., and Hutzler, F. (2015). On forward inferences of fast and slow readers. An eye movement study. Sci. Rep. 5:8432. doi: 10.1038/srep08432
Hearne, L., Cocchi, L., Zalesky, A., and Mattingley, J. B. (2015). Interactions between default mode and control networks as a function of increasing cognitive reasoning complexity. Hum. Brain Mapp. 36, 2719–2731. doi: 10.1002/hbm.22802
Hobeika, L., Diard–Detoeuf, C., Garcin, B., Levy, R., and Volle, E. (2016). General and specialized brain correlates for analogical reasoning: a meta-analysis of functional imaging studies. Hum. Brain Mapp. 37, 1953–1969. doi: 10.1002/hbm.23149
Jang, G., Yoon, S. A., Lee, S. E., Park, H., Kim, J., and Ko, J. H. (2013). Everyday conversation requires cognitive inference: neural bases of comprehending implicated meanings in conversations. Neuroimage 81, 61–72. doi: 10.1016/j.neuroimage.2013.05.027
*Jia, X., Lu, S., Zhong, N., Yao, Y., Li, K., and Yang, Y. (2009). “Common and distinct neural substrates of forward-chaining and backward-chaining syllogistic reasoning,” in 2009 ICME International Conference on Complex Medical Engineering (Tempe, AZ: IEEE), 1–6.
*Jin, H., Liu, H. L., Mo, L., Fang, S. Y., Zhang, J. X., and Lin, C. D. (2009). Involvement of the left inferior frontal gyrus in predictive inference making. Int. J. Psychophysiol. 71, 142–148. doi: 10.1016/j.ijpsycho.2008.08.009
Johnson-Laird, P. N. (1999). Deductive reasoning. Annu. Rev. Psychol. 50, 109–135. doi: 10.1146/annurev.psych.50.1.109
Jost, K., Khader, P. H., Düsel, P., Richter, F. R., Rohde, K. B., Bien, S., et al. (2012). Controlling conflict from interfering long-term memory representations. J. Cogn. Neurosci. 24, 1173–1190. doi: 10.1162/jocn_a_00199
Jung-Beeman, M. (2005). Bilateral brain processes for comprehending natural language. Trends Cogn. Sci. 9, 512–518. doi: 10.1016/j.tics.2005.09.009
Kan, I. P., and Thompson-Schill, S. L. (2004). Selection from perceptual and conceptual representations. Cogn. Affect. Behav. Neurosci. 4, 466–482. doi: 10.3758/cabn.4.4.466
Keenan, J. M., Baillet, S. D., and Brown, P. (1984). The effects of causal cohesion on comprehension and memory. J. Verbal Learn. Verbal Behav. 23, 115–126. doi: 10.1016/S0022-5371(84)90082-3
Keenan, J. M., Potts, G. R., Golding, J. M., and Jennings, T. M. (1990). “Which elaborative inferences are drawn during reading? A question of methodologies,” in Comprehension Processes in Reading, eds. D. A. Balota, G. B. F. d'Arcais and K. Rayner (Hillsdale, NJ: Erlbaum), 377–402.
*Kim, S. I., Yoon, M., Kim, W., Lee, S., and Kang, E. (2012). Neural correlates of bridging inferences and coherence processing. J. Psycholinguist Res. 41, 311–321. doi: 10.1007/s10936-011-9185-z
Kintsch, W. (1988). The role of knowledge in discourse comprehension: a construction-integration model. Psychol. Rev. 95, 163–182. doi: 10.1037//0033-295X.95.2.163
Knauff, M., Fangmeier, T., Ruff, C. C., and Johnson-Laird, R. N. (2003). Reasoning, models, and images: behavioral measures and cortical activity. J. Cogn. Neurosci. 15, 559–573. doi: 10.1162/089892903321662949
*Knauff, M., Mulack, T., Kassubek, J., Salih, H. R., and Greenlee, M. W. (2002). Spatial imagery in deductive reasoning: a functional MRI study. Cogn. Brain Res. 13, 203–212. doi: 10.1016/S0926-6410(01)00116-1
Koster-Hale, J., and Saxe, R. (2013). Theory of mind: a neural prediction problem. Neuron 79, 836–848. doi: 10.1016/j.neuron.2013.08.020
*Kranjec, A., Cardillo, E. R., Schmidt, G. L., Lehet, M., and Chatterjee, A. (2012). Deconstructing events: the neural bases for space, time, and causality. J. Cogn. Neurosci. 24, 1–16. doi: 10.1162/jocn_a_00124
*Kuperberg, G. R., Lakshmanan, B. M., Caplan, D. N., and Holcomb, P. J. (2006). Making sense of discourse: an fMRI study of causal inferencing across sentences. Neuroimage 33, 343–361. doi: 10.1016/j.neuroimage.2006.06.001
Laird, A. R., Fox, P. M., Price, C. J., Glahn, D. C., Uecker, A. M., Lancaster, J. L., et al. (2005). ALE meta-analysis: controlling the false discovery rate and performing statistical contrasts. Hum. Brain Mapp. 25, 155–164. doi: 10.1002/hbm.20136
Laird, A. R., Robinson, J. L., McMillan, K. M., Tordesillas-Gutiérrez, D., Moran, S. T., Gonzales, S. M., et al. (2010). Comparison of the disparity between Talairach and MNI coordinates in functional neuroimaging data: validation of the Lancaster transform. Neuroimage 51, 677–683. doi: 10.1016/j.neuroimage.2010.02.048
Lancaster, J. L., Tordesillas-Gutiérrez, D., Martinez, M., Salinas, F., Evans, A., Zilles, K., et al. (2007). Bias between MNI and Talairach coordinates analyzed using the ICBM-152 brain template. Hum. Brain Mapp. 28, 1194–1205. doi: 10.1002/hbm.20345
*Liu, J., Zhang, M., Jou, J., Wu, X., Li, W., and Qiu, J. (2012). Neural bases of falsification in conditional proposition testing: Evidence from an fMRI study. Int. J. Psychophysiol. 85, 249–256. doi: 10.1016/j.ijpsycho.2012.02.011
Mason, R. A., and Just, M. A. (2004). How the brain processes causal inferences in text. Psychol. Sci. 15, 1–7. doi: 10.1111/j.0963-7214.2004.01501001.x
*Mason, R. A., and Just, M. A. (2011). Differentiable cortical networks for inferences concerning people's intentions versus physical causality. Hum. Brain Mapp. 32, 313–329. doi: 10.1002/hbm.21021
*Monti, M. M., Osherson, D. N., Martinez, M. J., and Parsons, L. M. (2007). Functional neuroanatomy of deductive inference: a language-independent distributed network. Neuroimage 37, 1005–1016. doi: 10.1016/j.neuroimage.2007.04.069
*Monti, M. M., Parsons, L. M., and Osherson, D. N. (2009). The boundaries of language and thought in deductive inference. Proc. Natl. Acad. Sci. U.S.A. 106, 12554–12559. doi: 10.1073/pnas.0902422106
Myers, J. L., and Duffy, S. A. (1990). Causal inferences and text memory. Psychol. Learn. Motiv. 25, 159–173. doi: 10.1016/S0079-7421(08)60254-6
Myers, J. L., Shinjo, M., and Duffy, S. A. (1987). Degree of causal relatedness and memory. J. Mem. Lang. 26, 453–465. doi: 10.1016/0749-596X(87)90101-X
Ni, W., Constable, R. T., Mencl, W. E., Pugh, K. R., Fulbright, R. K., Shaywitz, S. E., et al. (2000). An event-related neuroimaging study distinguishing form and content in sentence processing. J. Cogn. Neurosci. 12, 120–133. doi: 10.1162/08989290051137648
*Noveck, I. A., Goel, V., and Smith, K. W. (2004). The neural basis of conditional reasoning with arbitrary conten. Cortex 40, 613–622. doi: 10.1016/S0010-9452(08)70157-6
*Osherson, D., Perani, D., Cappa, S., Schnur, T., Grassi, F., and Fazio, F. (1995). Distinct brain loci in deductive versus probabilistic reasoning. Neuropsychologia 36, 369–376. doi: 10.1016/S0028-3932(97)00099-7
Parsons, L. M., and Osherson, D. N. (2001). New evidence for distinct right and left brain systems for deductive vs. probabilistic reasoning. Cereb. Cortex 11, 954–965. doi: 10.1093/cercor/11.10.954
Polk, T. A., and Newell, A. (1995). Deduction as verbal reasoning. Psychol. Rev. 102, 533–566. doi: 10.1037/0033-295X.102.3.533
Porcaro, C., Medaglia, M. T., Thai, N. J., Seri, S., Rotshtein, P., and Tecchio, F. (2014). Contradictory reasoning network: an EEG and fMRI study. PLoS ONE 9:e92835. doi: 10.1371/journal.pone.0092835
Prado, J., Chadha, A., and Booth, J. R. (2011). The brain network for deductive reasoning: a quantitative meta-analysis of 28 neuroimaging studies. J. Cogn. Neurosci. 23, 3483–3497. doi: 10.1162/jocn_a_00063
Prado, J., and Noveck, I. A. (2007). Overcoming perceptual features in logical reasoning: a parametric functional magnetic resonance imaging study. J. Cogn. Neurosci. 19, 642–657. doi: 10.1162/jocn.2007.19.4.642
*Prado, J., Spotorno, N., Koun, E., Hewitt, E., Van der Henst, J. B., Sperber, D., et al. (2015). Neural interaction between logical reasoning and pragmatic processing in narrative discourse. J. Cogn. Neurosci. 27, 692–704. doi: 10.1162/jocn_a_00744
*Prado, J., Van Der Henst, J. B., and Noveck, I. A. (2010). Recomposing a fragmented literature: how conditional and relational arguments engage different neural systems for deductive reasoning. Neuroimage 51, 1213–1221. doi: 10.1016/j.neuroimage.2010.03.026
*Prat, C. S., Mason, R. A., and Just, M. A. (2011). Individual differences in the neural basis of causal inferencing. Brain Lang. 116, 1–13. doi: 10.1016/j.bandl.2010.08.004
Rapp, A. M., Mutschler, D. E., and Erb, M. (2012). Where in the brain is nonliteral language? A coordinate-based meta-analysis of functional magnetic resonance imaging studies. Neuroimage 63, 600–610. doi: 10.1016/j.neuroimage.2012.06.022
Reverberi, C., Bonatti, L. L., Frackowiak, R. S. J., Paulesu, E., Cherubini, P., and Macaluso, E. (2012). Large scale brain activations predict reasoning profiles. Neuroimage 59, 1752–1764. doi: 10.1016/j.neuroimage.2011.08.027
Reverberi, C., Cherubini, P., Frackowiak, R. S., Caltagirone, C., Paulesu, E., and Macaluso, E. (2010). Conditional and syllogistic deductive tasks dissociate functionally during premise integration. Hum. Brain Mapp. 31, 1430–1445. doi: 10.1002/hbm.20947
Reverberi, C., Cherubini, P., Rapisarda, A., Rigamonti, E., Caltagirone, C., and Frackowiak, R. S. (2007). Neural basis of generation of conclusions in elementary deduction. Neuroimage 38, 752–762. doi: 10.1016/j.neuroimage.2007.07.060
*Robertson, D. A., Gernsbacher, M. A., Guidotti, S. J., Robertson, R. R. W., Irwin, W., Mock, B. J., et al. (2000). Functional neuroanatomy of the cognitive process of mapping during discourse comprehension. Psychol. Sci. 11, 255–260. doi: 10.1111/1467-9280.00251
*Rodriguez-Moreno, D., and Hirsch, J. (2009). The dynamics of deductive reasoning: an fMRI investigation. Neuropsychologia 47, 949–961. doi: 10.1016/j.neuropsychologia.2008.08.030
Roy, M., Shohamy, D., and Wager, T. D. (2012). Ventromedial prefrontal-subcortical systems and the generation of affective meaning. Trends Cogn. Sci. 16, 147–156. doi: 10.1016/j.tics.2012.01.005
Rypma, B., and D'Esposito, M. (1999). The roles of prefrontal brain regions in components of working memory: effects of memory load and individual differences. Proc. Natl. Acad. Sci. U.S.A. 96, 6558–6563. doi: 10.1073/pnas.96.11.6558
Sansosti, F. J., Was, C., Rawson, K. A., and Remaklus, B. L. (2013). Eye movements during processing of text requiring bridging inferences in adolescents with higher functioning autism spectrum disorders: a preliminary investigation. Res. Autism Spectr. Disord. 7, 1535–1542. doi: 10.1016/j.rasd.2013.09.001
Satpute, A. B., Fenker, D. B., Waldmann, M. R., Tabibnia, G., Holyoak, K. J., and Lieberman, M. D. (2005). An fMRI study of causal judgments. Eur. J. Neurosci. 22, 1233–1238. doi: 10.1111/j.1460-9568.2005.04292.x
Schank, R. (1975). “The structure of episodes in memory,” in Representation and Understanding, ed. D. B. A. A. Collins (New York: Academic Press), 237–272.
*Siebörger, F. T., Ferstl, E. C., and von Cramon, D. Y. (2007). Making sense of nonsense: An fMRI study of task induced inference processes during discourse comprehension. Brain Res. 1166, 77–91. doi: 10.1016/j.brainres.2007.05.079
Singer, M. (1994). “Discourse inference processes,” in Handbook of Psycholinguistics ed. M. A. Gernsbacher (New York: Academic Press), 479–516.
Smith, K. W., Balkwill, L. L., Vartanian, O., and Goel, V. (2015). Syllogisms delivered in an angry voice lead to improved performance and engagement of a different neural system compared to neutral voice. Front. Hum. Neurosci. 9:273. doi: 10.3389/fnhum.2015.00273
Smith, K. W., Vartanian, O., and Goel, V. (2014). Dissociable neural systems underwrite logical reasoning in the context of induced emotions with positive and negative valence. Front. Hum. Neurosci. 8:736. doi: 10.3389/fnhum.2014.00736
Suvorova, E. V., and Polyakova, L. S. (2018). Types of inferences in discourse. Arab World English J. (AWEJ) 9, 294–306. doi: 10.2139/ssrn.3150915
Thompson-Schill, S. L., D'Esposito, M., Aguirre, G. K., and Farah, M. J. (1997). Role of left inferior prefrontal cortex in retrieval of semantic knowledge: a reevaluation. Proc. Natl. Acad. Sci. India Sect. B. Biol. Sci. 94, 14792–14797. doi: 10.1073/pnas.94.26.14792
Turkeltaub, P. E., Eickhoff, S. B., Laird, A. R., Fox, M., Wiener, M., and Fox, P. (2012). Minimizing within-experiment and within-group effects in activation likelihood estimation meta-analyses. Hum. Brain Mapp. 33, 1–13. doi: 10.1002/hbm.21186
van Ackeren, M. J., Casasanto, D., Bekkering, H., Hagoort, P., and Rueschemeyer, S. A. (2012). Pragmatics in action: Indirect requests engage theory of mind areas and the cortical motor network. J. Cogn. Neurosci. 24, 2237–2247. doi: 10.1162/jocn_a_00274
van den Broek, P. (1990). “The causal inference maker: Towards a process model of inference generation in text comprehension,” in Comprehension Processes in Reading, eds. D. A. Balota, G. B. F. d'Arcais and K. Rayner (New York: Lawrence Erlbaum Associates, Hillsdale), 423–446.
van den Broek, P. (1994). “Comprehension and memory of narrative texts: Inferences and coherence,” in Handbook of Psycholinguistics, ed. M. A. Gernsbacher (New York: Academic Press), 539–588.
Van Dijk, T. A., and Kintsch, W. (1983). Strategies of Discourse Comprehension. New York, NY: Academic Press.
Van Overwalle, F., and Baetens, K. (2009). Understanding others' actions and goals by mirror and mentalizing systems: a meta-analysis. Neuroimage 48, 564–584. doi: 10.1016/j.neuroimage.2009.06.009
*Virtue, S., Haberman, J., Clancy, Z., Parrish, T., and Jung Beeman, M. (2006). Neural activity of inferences during story comprehension. Brain Res. 1084, 104–114. doi: 10.1016/j.brainres.2006.02.053
*Virtue, S., Parrish, T., and Jung-Beeman, M. (2008). Inferences during story comprehension: cortical recruitment affected by predictability of events and working memory capacity. J. Cogn. Neurosci. 20, 2274–2284. doi: 10.1162/jocn.2008.20160
Virtue, S. M., and Sundermeier, B. A. (2016). What can neuroimaging research tell us about inference generation during text comprehension? Neuroimaging and inferences. Linguistics Lang. Compass 10, 257–271. doi: 10.1111/lnc3.12193
Visser, M., and Lambon Ralph, M. A. (2011). Differential contributions of bilateral ventral anterior temporal lobe and left anterior superior temporal gyrus to semantic processes. J. Cogn. Neurosci. 23, 3121–3131. doi: 10.1162/jocn_a_00007
Wagner, A. D., Paré-Blagoev, E. J., Clark, J., and Poldrack, R. A. (2001). Recovering meaning: Left prefrontal cortex guides controlled semantic retrieval. Neuron 31, 329–338. doi: 10.1016/s0896-6273(01)00359-2
Wang, W., Fan, L., and Xu, F. (2018). Hemispheric processing of inferences during discourse reading: a review of the literature. Am. J. Psychol. Behav. Sci. 5, 72–79. doi: 10.3758/BF03193276
Warren, W. H., Nicholas, D. W., and Trabasso, T. (1979). “Event chains and inferences in understanding narratives,” in New Direactions in Discourse Processing, ed. R. Freedle (Hillsdale, NJ: Erlbaum), 23–52.
Wende, K. C., Straube, B., Stratmann, M., Sommer, J., Kircher, T., and Nagels, A. (2012). Neural correlates of continuous causal word generation. Neuroimage 62, 1399–1407. doi: 10.1016/j.neuroimage.2012.06.003
Wertheim, J., and Ragni, M. (2020). The neurocognitive correlates of human reasoning: A meta-analysis of conditional and syllogistic inferences. J. Cogn. Neurosci. 32, 1–18. doi: 10.1162/jocn_a_01531
*Xu, J., Kemeny, S., Park, G., Frattali, C., and Braun, A. (2005). Language in context: Emergent features of word, sentence, and narrative comprehension. Neuroimage 25, 1002–1015. doi: 10.1016/j.neuroimage.2004.12.013
Yang, X., Li, H., Lin, N., Zhang, X., Wang, Y., Zhang, Y., et al. (2019). Uncovering cortical activations of discourse comprehension and their overlaps with common large-scale neural networks. Neuroimage 203:116200. doi: 10.1016/j.neuroimage.2019.116200
Yarkoni, T., Poldrack, R. A., Nichols, T. E., Van Essen, D. C., and Wager, T. D. (2011). Large-scale automated synthesis of human functional neuroimaging data. Nat. Methods 8, 665–670. doi: 10.1038/nmeth.1635
*Yarkoni, T., Speer, N. K., and Zacks, J. M. (2008). Neural substrates of narrative comprehension and memory. Neuroimage 41, 1408–1425. doi: 10.1016/j.neuroimage.2008.03.062
Ye, Z., and Zhou, X. (2009a). Conflict control during sentence comprehension: FMRI evidence. Neuroimage 48, 280–290. doi: 10.1016/j.neuroimage.2009.06.032
Ye, Z., and Zhou, X. (2009b). Executive control in language processing. Neurosci. Biobehav. Rev. 33, 1168–1177. doi: 10.1016/j.neubiorev.2009.03.003
Yeari, M. (2017). The role of working, memory in inference generation during reading comprehension: retention, (re)activation, or suppression of verbal information? Learn. Individ. Differ. 56, 1–12. doi: 10.1016/j.lindif.2017.04.002
Zhu, Z., Hagoort, P., Zhang, J. X., Feng, G., Chen, H. C., Bastiaansen, M., et al. (2012). The anterior left inferior frontal gyrus contributes to semantic unification. Neuroimage 60, 2230–2237. doi: 10.1016/j.neuroimage.2012.02.036
*^References marked with an asterisk indicate studies included in the meta-analysis.
Keywords: causal inferences, meta-analysis, neuroimaging, discourse understanding, logical problem-solving, frontotemporal network
Citation: Feng W, Wang W, Liu J, Wang Z, Tian L and Fan L (2021) Neural Correlates of Causal Inferences in Discourse Understanding and Logical Problem-Solving: A Meta-Analysis Study. Front. Hum. Neurosci. 15:666179. doi: 10.3389/fnhum.2021.666179
Received: 09 February 2021; Accepted: 21 May 2021;
Published: 23 June 2021.
Edited by:
Kirrie J. Ballard, The University of Sydney, AustraliaReviewed by:
Fiona Kumfor, The University of Sydney, AustraliaAmy E. Ramage, University of New Hampshire, United States
Copyright © 2021 Feng, Wang, Liu, Wang, Tian and Fan. This is an open-access article distributed under the terms of the Creative Commons Attribution License (CC BY). The use, distribution or reproduction in other forums is permitted, provided the original author(s) and the copyright owner(s) are credited and that the original publication in this journal is cited, in accordance with accepted academic practice. No use, distribution or reproduction is permitted which does not comply with these terms.
*Correspondence: Lin Fan, ZmFubGluQGJmc3UuZWR1LmNu; Wangshu Feng, Zi53YW5nc2h1QGdtYWlsLmNvbQ==