- 1Consciousness Lab, Psychology Institute, Jagiellonian University, Krakow, Poland
- 2Center of Functionally Integrative Neuroscience, Aarhus University, Aarhus, Denmark
- 3Cognitive Science, Institute of Philosophy, Jagiellonian University, Krakow, Poland
- 4Department of Cognitive Science and Artificial Intelligence, Tilburg University, Tilburg, Netherlands
- 5Center of Functionally Integrative Neuroscience, Aarhus University Hospital, Aarhus, Denmark
Transcranial magnetic stimulation (TMS) is used to make inferences about relationships between brain areas and their functions because, in contrast to neuroimaging tools, it modulates neuronal activity. The central aim of this article is to critically evaluate to what extent it is possible to draw causal inferences from repetitive TMS (rTMS) data. To that end, we describe the logical limitations of inferences based on rTMS experiments. The presented analysis suggests that rTMS alone does not provide the sort of premises that are sufficient to warrant strong inferences about the direct causal properties of targeted brain structures. Overcoming these limitations demands a close look at the designs of rTMS studies, especially the methodological and theoretical conditions which are necessary for the functional decomposition of the relations between brain areas and cognitive functions. The main points of this article are that TMS-based inferences are limited in that stimulation-related causal effects are not equivalent to structure-related causal effects due to TMS side effects, the electric field distribution, and the sensitivity of neuroimaging and behavioral methods in detecting structure-related effects and disentangling them from confounds. Moreover, the postulated causal effects can be based on indirect (network) effects. A few suggestions on how to manage some of these limitations are presented. We discuss the benefits of combining rTMS with neuroimaging in experimental reasoning and we address the restrictions and requirements of rTMS control conditions. The use of neuroimaging and control conditions allows stronger inferences to be gained, but the strength of the inferences that can be drawn depends on the individual experiment’s designs. Moreover, in some cases, TMS might not be an appropriate method of answering causality-related questions or the hypotheses have to account for the limitations of this technique. We hope this summary and formalization of the reasoning behind rTMS research can be of use not only for scientists and clinicians who intend to interpret rTMS results causally but also for philosophers interested in causal inferences based on brain stimulation research.
Introduction
A fundamental issue in human neuroscience is how to make causal inferences based on research data. Traditional use of neuroimaging methods limits experimental conclusions to correlational inferences (though, the methods of effective connectivity are used to postulate causal inferences; see Valdes-Sosa et al., 2011). Following their introduction, brain stimulation methods, especially TMS, started to be considered as a remedy for this limitation. TMS was developed over thirty years ago and is based on electromagnetic induction (Barker et al., 1985). A TMS coil induces an electric field which might influence the activity of brain tissue. It was originally thought that TMS would make it possible to conclude the causal relations between brain activity, cognitive functions, and behaviors. However, it has since become clearer that the brain cannot simply be parceled into regions responsible for certain functions, and the impact of brain lesions and non-invasive brain stimulation is not necessarily limited to a single area but extends to networks. Currently, TMS is often used to test hypotheses about how short-term changes in the excitability of a stimulated brain area affect cognitive functions. In online TMS paradigms, electromagnetic pulses are applied concurrently with the experimental measurement. The physiological consequences of a single electromagnetic pulse can be detected for over a dozen seconds (Furubayashi et al., 2013). In repetitive (rTMS) paradigms, pulses with a particular frequency pattern are applied during or before experimental measurement because they often lead to neuroplasticity-like changes (Chung et al., 2015). The neuromodulatory rTMS effect can be assessed with standard experimental procedures or neuroimaging techniques (for a review of combined TMS-EEG studies, see Thut and Pascual-Leone, 2010); it can be observed even for up to 45 min after a single protocol application (Huang et al., 2005), or it can last for months after multiple protocol applications over repeated TMS sessions in longitudinal studies (Speer et al., 2000, 2009; Li et al., 2004; Choi et al., 2014, 2019; Kang et al., 2016). Thus, TMS is often considered to be an extension of neuroimaging, which (due to its influence on brain activity) allows causal relations to be tested.
TMS is frequently used to decompose the functional organization of the brain. Multiple scientific articles contain statements that TMS can be used to draw both causal brain-behavior inferences (Sack, 2006; Śliwińska et al., 2014) and causal relationships between brain structure and function (Schutter et al., 2004; Bolognini and Ro, 2010; Hartwigsen, 2015; Veniero et al., 2019). In research practice, this often leads to implicit assumptions that TMS can selectively influence the area of interest, therefore its role can be established. Consequently, multiple studies have presented rTMS-based conclusions on the causal role of certain brain areas in certain cognitive functions (e.g., Carmel et al., 2010; Philiastides et al., 2011; Zanto et al., 2011; Bourgeois et al., 2013; Izuma et al., 2015; Schaal et al., 2015; Siuda-Krzywicka et al., 2016; Montefinese et al., 2017), often without describing alternative explanations or making a distinction between direct and indirect causal effects of an rTMS-induced change in activity in a certain area on a certain behavior or brain process.
Employing chronometry (tracking the time course of functional relevance), online single-pulse, double-pulse, or short-burst TMS protocols (including double-coil approaches) allow investigation of the causal relations between the activity of certain brain areas and behaviors or cognitive functions especially when effective connectivity measures are also employed (e.g., de Graaf et al., 2009). These protocol types might be used to influence cognitive functions or perturb brain activity to track the signal propagation and analyze the topographic pattern of TMS-evoked changes in brain activity. This allows researchers to: (1) identify the brain areas involved in certain behavior; (2) assess the impact of the stimulated brain area upon interconnected areas via direct connections or intermediate areas, including inter-hemispheric interactions (Blankenburg et al., 2008); (3) reveal bottom-up and top-down influences between brain areas; and (4) dissect the specific functional contributions of different cortical areas of an investigated network. Crucially, the propagation of TMS-evoked activity can depend on the degree of wakefulness (Massimini et al., 2005), which in some studies may act as a confound but in others may allow the state-dependence of interactions among remote and interconnected brain regions to be investigated. However, this use of TMS is limited to specific experimental designs, and some TMS effects (as in the case of all active TMS protocols) may be side effects of the stimulation procedure (Holmes and Meteyard, 2018; for a review, see Bestmann et al., 2008a).
The rTMS approach is more limited than single-pulse, double-pulse, and burst-pulse TMS in terms of helping to understand the causal relationships between brain areas and cognitive functions
(however, in certain designs rTMS can be used for chronometry, see Rossi et al., 2011). Online rTMS does not allow concurrent brain activity registration using neuroimaging techniques, while offline rTMS effects depend on neuroplasticity-like changes which might occur at various time points after the start or the end of rTMS. Thus, rTMS does not allow tracking of the direct influence of perturbation to determine the time point at which an area makes a critical contribution to a given behavior or to investigate effective connectivity between brain areas. Although most non-invasive stimulation methods share the same limitations as rTMS, for purposes of clarity we narrow the scope of the discussion below to rTMS. Most of the issues, that are mentioned below, related to the pitfalls of TMS have already been selectively discussed (e.g., Siebner and Rothwell, 2003; Robertson et al., 2003; Thickbroom, 2007; Bestmann et al., 2008a; Siebner et al., 2009). The current article aims to combine, organize, and analyze these insights at the theoretical level and indicate their possible consequences for inferences based on rTMS evidence. Below, we first analyze several known methodological issues that can invalidate inferences about direct causal relations between brain areas, brain processes, and cognitive functions investigated with TMS. Second, we discuss the special role that neuroimaging plays in rTMS-based inferences and approaches to creating TMS control conditions.
Inferences Based on Conditional Statements
Causal inference, and specifically inference based on interventions in the operation of a complex system such as the brain, fall within the theoretical framework of the general theory of causality that was developed by Pearl (2000). We use a small part of Pearl’s Structural Causal Model. This is because unlike causal frameworks such as Bradford Hill’s criteria (Hill, 1965), Pearl’s framework is resistant to counterexamples and makes sense of probabilistic causal inferences about specific mechanisms that are parts of complex systems. In this view, to characterize a relationship between event A and event B as causal is to say that a selective intervention on A might lead to a change in the distribution of B. We assume a causal influence of one event on another is direct if none of the variables included in a given causal model mediates this effect; otherwise, it is indirect. In a setting such as a TMS experiment, where intervention is randomized, we compare the intervention-related distribution of variables with a control distribution and expect to find suitable neuronal candidates that cause the response. For clarity purposes, we address TMS-related inferences with the use of conditional logic.
To consider a simple type of TMS-based inference, assume that a researcher is interested in cognitive function X. To investigate the process (PX) that underlies this function, the researcher aims to determine whether brain area 1 (A1), which is typically associated with PX, is engaged during a task that is assumed to engage cognitive function X (TX). For example, one may investigate the involvement of the dorsolateral prefrontal cortex in decision confidence by measuring the effect of rTMS on confidence ratings. In such a case, the hypothesis (H) often states that PX takes place in A1 and is tested with the application of an active rTMS protocol 1 (rTMS1) to A1. We can formally represent this pattern of reasoning in the following way (the logic symbol ∧ represents the logical conjunction, i.e., “and,” and the → represents implication, i.e., “if <antecedent> then <consequent>”):
H – PX takes place in A1
S1A—rTMS1 is applied to A1
TXD—a difference in TX performance is observed (as compared to a control condition)
Inference 1 (I1) states that the statement that PX takes place in A1 is true if the following two premises are true: (1) if PX takes place in A1 and rTMS1 is applied to A1 then a difference in TX performance is observed; and (2) rTMS1 is applied to A1 and a difference in TX performance is observed.
I1 depicts the basic form of reasoning used in rTMS research. However, like any inductive inference, this form of reasoning does not always lead to true conclusions. For example, the occurrence of the difference in TX performance may be unrelated to rTMS1, in which case, two independent factors contribute to falsely interpreting the consequent of the condition as true. Thus, causal reasoning based on misuse of I1 may lead to false conclusions. Possible overconfidence in I1-based inferences might also stem from overlooking both how TMS and brains work. First, the assumption that TMS selectively influences a targeted area is not always true. The strength of the induced electric field decreases together with the distance from the coil, so the brain areas above or adjacent to the targeted area are likely to be stimulated more than the intended one (Heller and van Hulsteyn, 1992). Second, applying TMS to one area can indirectly influence multiple brain areas that are structurally connected to it and lead to an alteration of the functional state of the targeted network, as pointed out in several reviews (Ruff et al., 2009; Bolognini and Ro, 2010; Ziemann, 2010; Beynel et al., 2020). In sum, TMS applied to a specific brain region can influence other regions directly (e.g., due to stimulation of an area above or adjacent to the area investigated) or indirectly via neural connections (e.g., indirect stimulation of an area that is connected to the investigated area or activity alteration in another area due to excitability alteration in the investigated area). These factors limit the strength of causal conclusions based on I1.
Accordingly, rTMS1 may be responsible for a difference in TX performance via unintended stimulation of an area other than A1. For example, assume that A1 is structurally connected to brain area 2 (A2). Then, there is a possibility that A2 activity is influenced: (1) directly by rTMS1 when A1 is targeted (Figure 1A); or (2) indirectly by rTMS1 via an alteration of A1 activity. At the same time, A2 is responsible or more important than A1 for executing PX (Figure 1B). Unintentional direct stimulation of A2 may occur in several ways. First, the physical spread of an electrical field may reach areas adjacent to the targeted one. Second, since electrical current follows the path of least resistance, the electric field distribution is highly dependent on cerebrospinal fluid distribution and brain folding, thus the peak of the electric field can occur in gray matter regions located some distance from the electric field’s expected peak, which is judged based on the location of the center of the (figure-of-eight) coil. This might result in greater stimulation of area/s other than the targeted one (Bijsterbosch et al., 2012). Third, it is challenging to distinguish whether the rTMS effect stems from excitability alteration in the targeted area or an area above it that possibly has a distinct specialization. These concerns may be raised especially when deeper structures such as the anterior cingulate cortex (Hayward et al., 2007) or insula (Pollatos et al., 2016) are investigated. The vast majority of TMS studies target superficial structures; however, the rule that the strongest electrical field is generated within the outermost areas applies even if the distances (which might be the consequences of brain folding) are small. Because a large part of the cortex lies within sulci, targeted brain coordinates in numerous TMS studies have to be placed within sulci (Busan et al., 2009; Cappelletti et al., 2009; Salillas et al., 2009). Additionally, stimulation of deeper brain structures is obtained at the expense of inducing wider electrical field spread in the brain (Roth et al., 2007; Deng et al., 2013; Downar et al., 2016). For example, metabolic and physiological effects on the primary motor cortex and the primary somatosensory cortex can be observed after rTMS to premotor areas (Siebner et al., 2003). This may compound the difficulty in distinguishing the contribution of direct vs. indirect rTMS effects. The network effects may produce remote activity alteration in cortical areas via cortico-cortical routes and in subcortical structures via cortico-subcortical projections (Strafella et al., 2003; Lefaucheur et al., 2020). The extent of the network effects depends on rTMS protocol parameters (Bestmann et al., 2003). Additionally, the assumption that a difference in TX performance is caused by an rTMS1-induced change in A1 activity may be misleading due to the occurrence of placebo and sensory side effects (Abler et al., 2005). Moreover, rTMS may influence areas related to general cognitive resources (e.g., regions engaged in attentional or working memory processing) or the observed effect may be specific to the TX design (e.g., resulting from rTMS1 influence on brain regions involved in response generation during TX), which is not related to the influence on the investigated cognitive function. In sum, overconfidence in I1 has multiple ways to lead researchers to overinterpret their data as evidence that PX takes place in A1.
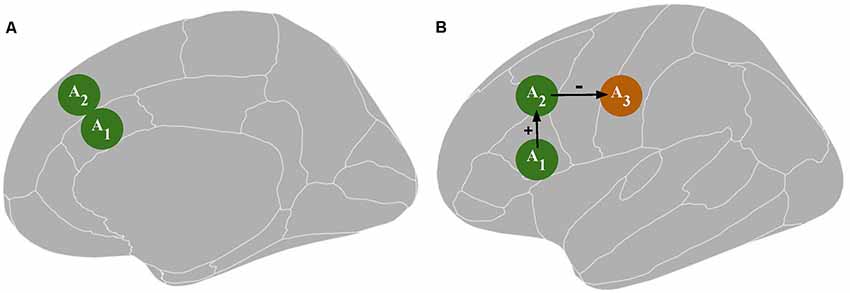
Figure 1. Panel (A) depicts a possible direct influence of transcranial magnetic stimulation [TMS; an excitability alteration in the brain tissue surrounding the targeted area A1, i.e., area 2 (A2)]. Panel (B) depicts a possible indirect TMS influence: an excitability alteration in A2 or area 3 (A3) resulting from an excitability alteration in A1. A1 represents the targeted area; A2 and A3 represent the areas directly and indirectly connected to A1, respectively, which together constitute a functional network. The green color indicates an increase in neuronal excitation while the orange color indicates a decrease in neuronal excitation.
Since statements that follow I1 cannot fully support the conclusion that PX takes place in A1, can some other inference be used to show that PX is not executed in A1? This would provide independent evidence for excluding that region from the area of research interest. This way of reasoning is indeed found in TMS literature: based on the lack of an observed effect, some authors postulate a lack of rTMS influence on investigated cognitive functions (e.g., Ghabra et al., 1999; Poulet et al., 2004; Jung et al., 2010; Bor et al., 2017), which might suggest that an investigated area is not involved in the process underlying the investigated cognitive function. Consider then the inference of the following structure (the logic symbol ⇁ represents negation, i.e., “not”):
H – PX takes place in A1
S1A—rTMS1 is applied to A1
TXD—a difference in TX performance is observed (as compared to a control condition)
Inference 2 (I2) states that the statement that PX is not executed in A1 is true if the following two premises are also true: (1) a difference in TX performance is observed if PX takes place in A1 and rTMS1 is applied to A1; (2) rTMS1 is applied to A1 and a difference in TX performance is not observed.
In research practice, rTMS1 does not always lead to a change in A1 activity and/or a difference in TX performance. rTMS1 may have no factual effect because: (1) the rTMS1 frequency pattern is inadequate for investigating PX (e.g., theta burst stimulation is applied but PX is independent of theta-gamma coupling; De Ridder et al., 2007); (2) rTMS1 parameters are set too low (e.g., intensity or current direction) to influence PX (Valero-Cabré et al., 2017); (3) brain-intrinsic factors such as neurochemical and neurophysiological properties of A1 prevent an alteration in its excitability (e.g., it is impossible to facilitate or inhibit A1 to a greater extent than it is before rTMS1 application; Karabanov et al., 2015); and (4) to influence A1, rTMS1 should be applied with greater precision (e.g., based on individual functional brain images; Hannula and Ilmoniemi, 2017). Altogether, this is enough evidence to assume that I2 is not a stronger form of reasoning than I1. I1 and I2 include a hidden assumption that rTMS1 leads to an alteration in A1 activity but not all active rTMS applications have neural effects. To claim that A1 has changed, the assertion based on the inference presented below has to be true:
S1A—rTMS1 is applied to A1
A1C—a change in A1 activity is present
I3 states that the statement that A1 activity is changed if the following two premises are true: (1) a change in A1 activity is present if rTMS1 is applied to A1; and (2) rTMS1 is applied to A1.
The issue of the impact of rTMS1 on the activity of A1 might be addressed with the use of neuroimaging.
TMS and Neuroimaging
A way of strengthening TMS-based inferences is to combine TMS with neuroimaging, the advantages of which have already been exhaustively described (e.g., Sack, 2006; Bestmann et al., 2008b; Bergmann et al., 2016). Multiple studies have already successfully employed neuroimaging to determine whether a particular rTMS protocol leads to a change in A1 activity (e.g., Bestmann et al., 2008c; Ruff et al., 2008; Capotosto et al., 2012). Despite the advantage of neuroimaging methods in allowing detection of a change in A1 activity, confirmation that the change in A1 activity accompanies TMS1 cannot fully confirm H. Importantly, even if the change in A1 activity can be confirmed with neuroimaging, it does not always lead to a difference in TX performance (Reithler et al., 2011). TMS1 may have no observable effect because: (1) TMS1 could have additional consequences that hinder the original stimulation effect, such as the occurrence of compensatory effects that diminish the TMS-induced alteration in A1 activity or that fulfill the function of A1 (Andoh and Martinot, 2008); and (2) TX may not provide an adequate measure of PX because TX or its performance level is not demanding enough to be influenced by TMS1, or TX is not sensitive enough to capture the impact of TMS1. Nevertheless, this does not imply that null TMS results are not meaningful because they are crucial to proving the functional irrelevance of a brain region to performing a particular function (de Graaf and Sack, 2011).
Next, assume that the influence of TMS1 on A1 can be effectively measured by neuroimaging methods and TX, and both a change in A1 activity and a difference in TX performance is observed. This leads to stronger reasoning than I1 (inference 4; I4):
H – PX takes place in A1
S1A—rTMS1 is applied to A1
TXD—a difference in TX performance is observed (as compared to a control condition)
A1C—a change in A1 activity is present
I4 states that the statement that PX takes place in A1 is true if the following two premises are true: (1) the antecedent of I1; and (2) a difference in TX performance is observed if the antecedent of I3 is true (analogous reasoning including ⇁ T_XD instead of TXD can be used to infer about the lack of A1 involvement in PX).
Again, since the inference is inductive, I4 is not immune to error and H might be false. Even if it is not, I4 merely adds to I1 that whenever rTMS1 is applied to A1, its activity is changed, and if this occurs then a difference in TX performance is observed. However, this reasoning pattern does not guarantee the correctness of the conclusion that the change in A1 activity is a cause of the difference in TX performance, and therefore that PX takes place in A1. It may be the case that TMS1 is a cause of both the change in A1 activity and the difference in TX performance, but the change in A1 activity is not a cause of the difference in TX performance. Thus, the causal inference between rTMS1 to A1 and the difference in TX performance is stronger when the purported cause is brain stimulation but not when the purported cause is the change in brain activity, i.e., TMS causes are not analogs of neural causes. To strengthen I4 inference one might additionally provide evidence that whenever the difference in TX performance is observed the change in A1 activity is present (inference 5; I5):
H – PX takes place in A1
S1A—rTMS1 is applied to A1
TXD—a difference in TX performance is observed (as compared to a control condition)
A1C—a change in A1 activity is present
I5 states that the statement that PX takes place in A1 is true if the following two premises are true: (1) the antecedent of I4; and (2) a change in A1 activity is present if a difference in TX performance is observed.
I4 and I5 are improvements over I1, and I2 and provide more confidence in TMS results. However, the limits of TMS-based conclusions also strongly depend on the complexity of the brain processes/cognitive functions investigated. The assumption that PX takes place in A1 may be simply inadequate because the complexity of PX may require it to be executed by a network rather than a single area (Pessoa, 2014), i.e., a brain area determined with TMS to be “responsible” for a certain cognitive function may be necessary but not sufficient for the realization of this cognitive function. Thus, instead of focusing on the functional properties of a single brain area, often it is necessary to investigate the functional interactions between remote but interconnected brain regions (for a review of different paradigms, see Romei et al., 2016). However, even though H might alternatively state that A1 is partly (not fully) responsible for PX, all the above issues related to the described inferences still hold.
In essence, the employment of neuroimaging may allow the following questions to be answered: (1) Does rTMS1 applied to A1 lead to a detectable change in A1 activity (Siebner et al., 2000)?; (2) How big is the influence of rTMS1 on areas adjacent to A1?; (3) Which areas are functionally connected to A1, and are they involved in PX and/or TX (Bestmann et al., 2005)?; (4) How does rTMS1 affect connectivity between certain brain areas or networks (Gratton et al., 2013)?; (5) What is the relation between the effects of rTMS1 and the other brain activations that occur during TX?; (6) What is the relation between the effects of rTMS1 and the difference in TX performance?; and (7) Which kind of neuroplastic changes arise, and when (Poeppl et al., 2018)? These investigations might be supported by the use of effective connectivity measures (Iwabuchi et al., 2019) based on the application of causal dynamic modeling, Granger causality (Friston et al., 2013), or graph theory (Farahani et al., 2019). Additionally, novel modeling approaches that can localize cortical TMS effects might be employed to determine whether the cortical area is effectively stimulated by TMS (Weise et al., 2020). At the same time, neuroimaging evidence can include confounding activations rather than clearly represent the network responsible for the cognitive function X because: (1) TMS1 may serve as a common cause that has several transcranial and non-transcranial consequences (Conde et al., 2019), thus some of the brain activations (including compensatory mechanisms) may be unrelated to PX; and (2) engagement in TX may activate processes unrelated to PX (which can be addressed with appropriate control conditions). Therefore, determining whether observed changes in brain activity are associated more with activity change in A1 or its adjacent areas and differentiating between network effects related to PX and compensatory effects is both challenging. In sum, the above patterns of reasoning may still lead to false conclusions, especially if no adequate control condition is employed.
rTMS Control Conditions
TMS might result in various psychological, auditory, and somatosensory side effects that might trigger shifts of attention, influence alertness, or interact with elements of the experimental task. Factors like the placement of the TMS coil or the occurrence of a clicking sound can influence task performance. For example, Duecker et al. (2013) showed that lateralized sham TMS pulses caused automatic shifts of spatial attention towards the location of the TMS coil. The use of sham TMS is intended to account for the impact of active TMS’s placebo and sensory side effects. The former is related to behavioral and cognitive changes (including certain expectations) that result from a person’s belief that their brain is being stimulated, while the latter is related to somatosensory effects (e.g., muscle twitches), peripheral nerve stimulation, and auditory effects (perception of a clicking sound). The sham approach might induce placebo effects of different magnitude (Burke et al., 2019). The mismatch between active TMS and the sensory effects of control TMS can form participants’ beliefs about the effectiveness of brain stimulation. The sham approaches can to a certain degree reproduce the sensory effects of active TMS without meaningfully influencing brain activity. They are based on the employment of either regular but tilted TMS coils, in which case, the electric field can still be sufficiently strong to result in somatosensory effects and peripheral nerve stimulation (Loo et al., 2000; Lisanby et al., 2001) or purpose-built sham TMS coils which have a magnetic shield that attenuates the electromagnetic field and prevents stimulation of the brain concurrently limiting somatosensory and peripheral nerve stimulation effects (for a review, see Duecker and Sack, 2015). To mitigate the trade-off between invoking somatosensory effects and not stimulating the brain, Duecker and Sack (2015) recommend the use of surface electrodes for skin stimulation in combination with a sham TMS coil.
However, sham TMS approaches do not demonstrate area specificity. Thus, Duecker and Sack (2015) recommend it might be beneficial to use sham TMS over each brain area where active TMS is applied to ensure that all stimulation sites have a control condition for the sensory side effects of TMS. Proper choice of control condition/s involves taking into account the difference between clinical and experimental research as well as whether and how the investigated process can be influenced by participants’ beliefs. While single-blinding seems to be feasible in between-subject designs, due to distinctive TMS effects, double-blinding is difficult to perform (Broadbent et al., 2011). However, it is practiced to use the sham and active TMS coils that are indistinguishable to the researcher carrying out the stimulation, and/or this researcher is not informed about the hypothesis of the study (Basil et al., 2005). One might also minimize the placebo effect-related issues by the employment of between-subject designs (on the cost of increasing interindividual variability). Despite the chosen design, the researcher might gather from participants information on blinding success or how the TMS was experienced in a form of a short questionnaire which can further inform the study results (Flanagan et al., 2019). An alternative to the control stimulations (including active and sham TMS control strategies) might be an investigation of interindividual differences in the response to TMS measured with neuroimaging techniques and correlating them with the chosen behavioral measure.
The probabilistic strength of inferences based on experimental studies largely depends on the type of control condition used. Below, we discuss how considerations regarding control condition/s apply to TMS research designs. In general, when investigating whether PX underlies cognitive function X, the simplest study designs consist of investigating a difference in TX performance between pre-and post-TMS conditions or between the application of TMS1 and a sham rTMS protocol (rTMS0) to the same area (Duecker and Sack, 2015).
Suppose that TMS1 ab rTMS0 protocols were applied to A1. If a difference in TX performance is observed between rTMS1 and rTMS0 conditions, besides explanations based on sensory and placebo TMS effects (Duecker and Sack, 2015) there are alternative explanations that should be taken into consideration that is related to the direct and indirect influence of TMS on: (1) the areas surrounding A1; (2) excitability of A2, which could be more important for executing PX; (3) processes responsible for general cognitive functions; and (4) processes not specific to cognitive function X but to TX execution. Given this, eliminating these possible alternative explanations should guide the designs of TMS studies.
Protocol Control
Ideally, rTMS0 should account for sensory and placebo effects of rTMS1 but does not cause a change in A1 activity (Duecker and Sack, 2015). Typically used rTMS0 that attempts not to influence brain activity fail to control for all the effects that are not specific to the change in A1 activity because we might assume the ideal control should influence areas which are stimulated when A1 is targeted with TMS to separate the consequence of the change in A1 activity from the consequences of influencing other brain areas. For example, if an area is embedded in brain folds or lies relatively deep in the brain, then distal cortical areas which are situated above that area are affected by the electrical field, most likely more strongly (Heller and van Hulsteyn, 1992). This issue (a direct stimulation influence on the areas surrounding A1) can be partly addressed with a control condition by diminishing the intensity of the used protocol to account for the stimulation of the areas lying above A1, i.e., influencing cerebrospinal fluid distribution or superior areas while not reaching A1 in a significant manner. However, it has to be taken into account that the relationship between TMS protocol intensity and its outcome might not be linear (e.g., Chung et al., 2018). Additionally, active protocols with certain frequency patterns are often classified in TMS literature as “inhibitory” or “excitatory”. Thus, sometimes the protocol patterns of rTMS1 and another active rTMS protocol 2 (rTMS2) differ and might be commonly conceived as being inhibitory and excitatory, respectively; thus, they are used to obtain a difference in TX performance directly (e.g., Gann et al., 2020) or to prime cortex excitability before the application of other protocols (e.g., Todd et al., 2009). It is important to note that inhibitory and excitatory rTMS properties are extrinsic to the protocol pattern and may vary depending on, e.g., protocol length, current direction, intensity, genome, and the targeted area characteristics, including its tissue excitability history and tissue excitability before protocol application (Polanía et al., 2018). Therefore, applying TMS1 and TMS2 separately to A1 cannot inform what change or difference in A1 activity is represented by a difference in TX performance unless it is previously known how the activity of A1 is related to the difference in TX performance, or the change in A1 activity was recorded with neuroimaging methods that can differentiate between an increase or a decrease of A1 activity.
Area Control
The following, previously mentioned, issues can be addressed with a control condition that includes a control area: (1) stimulation of areas next to A1; (2) an indirect network effect on A2 activity that is more important for executing PX; and (3) influence on processes responsible for more general cognitive functions than cognitive function X issue that undermine the strength of TMS-based inferences. In TMS studies, it is often assumed that an adequate control condition employs a stimulation protocol that affects an area that has the lowest possibility of playing a role in PX or does not influence the brain at all.
For a long time, the vertex was conceived to be such a site because it was presumed that its stimulation does not affect the brain at all. Nonetheless, several years ago it was shown that the blood oxygen level-dependent (BOLD) signal decreases in the default mode network after applying 1 Hz rTMS to the vertex, and this is not accompanied by any significant BOLD increases throughout the brain (Jung et al., 2016). The authors concluded that this supports the use of vertex simulation as a control condition. However, such a conclusion is problematic for several reasons. First, it presumes that an increase in the BOLD signal, which determines which parts of the brain are most active, will be observed after the application of a protocol that predominantly acts in an inhibitory manner (Fitzgerald et al., 2002). Second, there is an assumption that a decrease in the BOLD signal cannot indicate a change in neuronal activity (which could represent an increase in the activity of inhibitory neurons). Also, distinctly increasing and decreasing neuronal activity in an area is not equivalent to improving and impairing a cognitive function that depends on this area. Some brain processes require a decrease in local brain activity, e.g., deactivation has often been observed in the hippocampus during encoding and retrieval tasks believed to recruit this brain structure (Axmacher et al., 2009). Third, there is an assumption that the adequate control area is the one with the lowest possibility of affecting PX. Targeting A2 (an area which is not anticipated to carry out PX) does not confirm the specificity of A1 for carrying out PX, i.e., that PX is carried out exclusively in A1. Since the evidence in favor of the specificity of A1 is based on inductive reasoning, in theory, it would be required to effectively stimulate all brain areas to conclude that A1 and only A1 is responsible for PX. Conceivably, an opposite approach should be adopted: adequate control for the site requires the selection of a control site that has a high probability of influencing PX. However, this approach is challenged by consideration of possible indirect network influences on A1 due to the possibility of the control site’s involvement in processes interacting with PX. Furthermore, assume that PX requires activation in areas A1 and A2. When a difference in TX performance between the conditions with rTMS1 to A1 and rTMS1 to A2 is analyzed and rTMS1 in the first condition resulted in impairment of TX performance but in the second condition resulted in improvement of TX performance, one might erroneously conclude that only one area is crucial for X. Similarly, if rTMS1 in both conditions influenced TX performance in the same manner, one might erroneously conclude that rTMS1 was ineffective. Thus, limiting control conditions to area control might be not sufficient to adequately explain the TMS effect.
Task Control
The issues of influencing processes responsible for more general cognitive functions rather than cognitive function X and influencing processes specific to TX but not to cognitive function X, both of which weaken the strength of TMS-based inferences, can be addressed with task control. Dissociations may help reduce the probability of drawing erroneous conclusions on the neural bases of cognitive functions (Machery, 2012). To solve complex issues regarding certain cognitive functions or to include a task control condition in a study, e.g., to demonstrate that a certain brain area is selectively engaged in the execution of PX but not in the execution of the neuronal process that underlies a different cognitive function Y (PY), rTMS can be employed to determine whether the neural underpinnings of cognitive functions X and Y differ. In this case, inferences can be based on a single dissociation that is observed whenever TMS influences TX and influences TY to a lesser extent. This may lead to the conclusion that A1 plays a role in PX but not PY.
However, the results of studies employing task control may still be confounded by the confounds already mentioned. Additionally, the following confounds might be present: (1) a task that taps into one of two processes (TX into PX) might be less sensitive than a task that taps into another one (TY into PY); (2) due to its characteristics, PX might be more difficult to measure than PY; (3) the relative difficulties of TX and TY are likely to require a different amount of available cognitive resources (e.g., memory, attention); (4) when cognitive resources are limited, different brain networks may be engaged in TX or TY execution than when they are available; and (5) a discrepancy between how TX and TY engage A1 and A2 can be observed, even when they recruit the same area or network, e.g., carrying out TX may require a decrease in A1 activity, while carrying out TY may require an increase in A1 activity. In all the above circumstances, it would be erroneous to conclude with certainty that cognitive functions X and Y are based on two distinct brain substrates. The solution may consist of designs that combine different control approaches and allow double dissociation (Dunn and Kirsner, 2003), e.g., TX but not TY performance is impaired when rTMS0 application and rTMS1 application outcomes are compared after stimulation to A1, while TY but not TX performance is impaired when the rTMS0 and rTMS1 outcomes are compared after stimulation to A2. In the case of an uncrossed double dissociation, a difference in TX performance and a difference in TY performance is observed when A1 condition and A2 condition are compared (when pre-and post- rTMS1 or rTMS1 and rTMS0 are compared) but one condition is associated with higher performance in both tasks. A cross-over double dissociation is observed when rTMS1 to A1 influences TX performance more than rTMS1 to A2, and rTMS1 to A2 influences TY performance more than rTMS1 to A1 (for a summary of the solutions that aim to control for TMS confounds, see Figure 2).
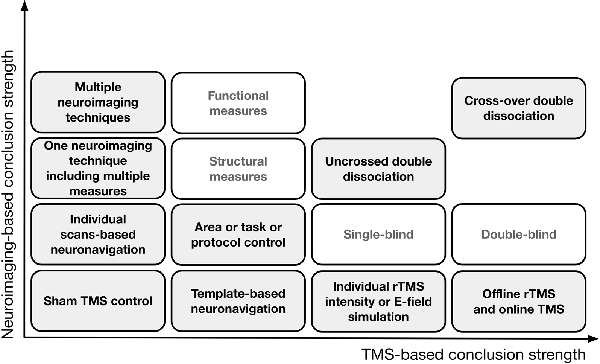
Figure 2. A theoretical representation of the solutions that aim to control for confounds in TMS research and improve the logical strength of the premises behind the conclusions. On the horizontal axis, progress in TMS-based conclusion strength is depicted when only TMS is employed; the vertical axis represents conclusion strength when neuroimaging is involved.
Can it then be concluded that PX takes place in A1 while PY takes place in A2? Unfortunately, most of the mentioned confounds also apply to double dissociations (e.g., rTMS1 to A1 reduces the available cognitive resources to TX, while S2 to A2 reduces them to TY). In the case of uncrossed double dissociations, the additional confound may be that the task demand function for A1 increases monotonically, while the task demand function for A2 is U-shaped: A2 is more active when a task requires fewer or more cognitive resources. In such circumstances, if TX and TY recruit a single process whose neural correlate includes A1 and A2, for A1 the greater task demands may correspond to the increase in its activity, while for A2 the greater task demands can correspond to its inactivation. Such an issue can be avoided when a cross-over double dissociation is observed, but the following confounds may still be present: (1) neuroplasticity-like effects occur at a different rate in A1 and A2 (e.g., depending on the type of brain cells affected by the stimulation); (2) rTMS1 and rTMS2 protocols applied to different areas may differently influence excitability in these areas; (3) an increase in A1 excitability results in a decrease in A2 activity, which is necessary to perform TY, while an increase in A2 excitability results in inactivation of A1, which is the area necessary to perform TX; (4) the execution of PX may correspond to A1 activity increase while the execution of PY may correspond to A1 inactivation; and (5) both A1 and A2 are recruited depending on the available cognitive resources, and the processes recruited when the amount of available resources is greater differ from the processes recruited when fewer resources are available. In all the above circumstances, it would be premature to conclude with certainty that cognitive functions X and Y are based on two distinct brain substrates.
In certain types of research (mostly preclinical and clinical studies), rTMS effects might be studied using longitudinal designs. The effect of longitudinal rTMS studies can be long-lasting, thus they can be used to investigate stable neuroplastic changes and determine whether the observed rTMS effect consistently arises over the time course of a study (Auriat et al., 2015). They also reduce the erroneous identification of side effect-associated changes as the brain stimulation effect, and they enable the employment of multiple testing measures. Similar to single-session rTMS effects, the rTMS effects in longitudinal studies might be related to individual excitability of brain areas, but they are less prone to the influence of day-to-day fluctuations in cortex excitability (Huber et al., 2013). However, there is still a possibility that the long-term effects of neuroplasticity in longitudinal studies might be related to placebo effects or be influenced by confounding factors that occur over the time course of the study.
Conclusions
TMS has traditionally been used to provide evidence for functional brain specialization. Nevertheless—as has been getting clearer over the past two decades—the application of rTMS alone does not allow causal inferences to be drawn on neural causes without additional assumptions. A change in the execution of an experimental task might be a consequence of rTMS but at the same time not a consequence of a change in the excitability of a targeted area. However, this might be avoided when: (1) the research question is grounded in previous research and accounts for the complexity of the investigated cognitive function; (2) neuroimaging/neurophysiological techniques are employed to monitor the direct and indirect influence of rTMS; and (3) more than one control condition is employed in a single experiment to reduce the number of possible interpretations. On one hand, functional neuroimaging could make it possible to determine whether the process responsible for the investigated cognitive function has local or network characteristics and can be used to study the spread of TMS effects throughout the brain networks. On the other hand, confounding factors of neuronal correlates of investigated cognitive processes need to be addressed within each TMS-neuroimaging study. Although TMS has been proven to be a very effective brain stimulation method, its characteristic features have to be considered in reasoning based on its employment. In this article, we have clarified the difference between the causal effects of TMS and structure-related causal effects, and we have pointed out that the latter can be divided into direct and network effects. We have also outlined issues related to TMS-based inferences. Taking them into account requires limiting the extent of TMS-based reasoning but at the same time may support analysis of possible confounds and improve research designs to alleviate these confounds. Although the aforementioned issues are often addressed by experts in the field of non-invasive brain stimulation, we hope that the presented summary and theoretical analysis will help researchers who are developing the field of human-neuroscience based on TMS-based inferences. Even though rTMS without neuroimaging cannot unequivocally prove structure-related causal claims concerning direct relations between brain processes carried out in certain areas and certain behaviors/cognitive functions, it might be used for probabilistic statements about causal influences if its limitations are kept in mind. The fact that combining rTMS with neuroimaging techniques allows stronger inferences to be made does not imply that one should use rTMS only in combination with neuroimaging or/and multiple control conditions. The need for neuroimaging or/and multiple control conditions depends on the research question guiding the study and how its results are intended to be interpreted. There is a trade-off between the inferential limit and experimental feasibility; therefore, when feasible, combining rTMS with neuroimaging, multiple control conditions, and/or perturbational TMS is recommended and might provide further support for conclusions regarding experimental outcomes.
Author Contributions
JH drafted the manuscript. MK, KS, and MW suggested changes and provided comments on the manuscript. JH improved the manuscript. All authors contributed to the article and approved the submitted version.
Funding
This work was supported by the National Science Centre, Poland under grants HARMONIA (2014/14/M/HS6/00911) and OPUS [2017/27/B/HS6/00937] given to MW and grant SONATA (2015/17/D/HS1/01705) given to MK. The publication was funded by the Priority Research Area Society of the Future under the program “Excellence Initiative—Research University” at the Jagiellonian University in Krakow.
Conflict of Interest
The authors declare that the research was conducted in the absence of any commercial or financial relationships that could be construed as a potential conflict of interest.
Acknowledgments
We thank Borysław Paulewicz for providing comments on our manuscript and Michael Timberlake for proofreading. This article is based upon work from COST Action CA18106, supported by COST (European Cooperation in Science and Technology).
Abbreviations
A1C, a change in A1 activity is present; BOLD, blood oxygen level-dependent; H, PX takes place in A1; PX, process underlying cognitive function X; PY, process underlying cognitive function Y; I1, inference 1; I2, inference 2; I3, inference 3; I4, inference 4; I5, inference 5; rTMS, repetitive Transcranial magnetic stimulation (TMS); S0, a sham rTMS protocol; rTMS1, an active rTMS protocol 1; S1A, rTMS1 is applied to A1; rTMS2, an active rTMS protocol 2; TMS, transcranial magnetic stimulation; TX, task X; TXD, an observed difference in TX performance; TY, task Y.
References
Abler, B., Walter, H., Wunderlich, A., Grothe, J., Schönfeldt-Lecuona, C., Spitzer, M., et al. (2005). Side effects of transcranial magnetic stimulation biased task performance in a cognitive neuroscience study. Brain Topogr. 17, 193–196. doi: 10.1007/s10548-005-6028-y
Andoh, J., and Martinot, J.-L. (2008). Interhemispheric compensation: a hypothesis of TMS-induced effects on language-related areas. Eur. Psychiatry 23, 281–288. doi: 10.1016/j.eurpsy.2007.10.012
Auriat, A. M., Neva, J. L., Peters, S., Ferris, J. K., and Boyd, L. A. (2015). A review of transcranial magnetic stimulation and multimodal neuroimaging to characterize post-stroke neuroplasticity. Front. Neurol. 6:226. doi: 10.3389/fneur.2015.00226
Axmacher, N., Elger, C. E., and Fell, J. (2009). The specific contribution of neuroimaging versus neurophysiological data to understanding cognition. Behav. Brain Res. 200, 1–6. doi: 10.1016/j.bbr.2009.01.028
Barker, A. T., Jalinous, R., and Freeston, I. L. (1985). Non-invasive magnetic stimulation of human motor cortex. Lancet 1, 1106–1107. doi: 10.1016/s0140-6736(85)92413-4
Basil, B., Mahmud, J., Mathews, M., Rodriguez, C., and Adetunji, B. (2005). Is there evidence for effectiveness of transcranial magnetic stimulation in the treatment of psychiatric disorders? Psychiatry 2, 64–69.
Bergmann, T. O., Karabanov, A., Hartwigsen, G., Thielscher, A., and Siebner, H. R. (2016). Combining non-invasive transcranial brain stimulation with neuroimaging and electrophysiology: current approaches and future perspectives. NeuroImage 140, 4–19. doi: 10.1016/j.neuroimage.2016.02.012
Bestmann, S., Baudewig, J., Siebner, H. R., Rothwell, J. C., and Frahm, J. (2003). Subthreshold high-frequency TMS of human primary motor cortex modulates interconnected frontal motor areas as detected by interleaved fMRI-TMS. NeuroImage 20, 1685–1696. doi: 10.1016/j.neuroimage.2003.07.028
Bestmann, S., Baudewig, J., Siebner, H. R., Rothwell, J. C., and Frahm, J. (2005). BOLD MRI responses to repetitive TMS over human dorsal premotor cortex. NeuroImage 28, 22–29. doi: 10.1016/j.neuroimage.2005.05.027
Bestmann, S., Ruff, C. C., Blankenburg, F., Weiskopf, N., Driver, J., and Rothwell, J. C. (2008a). Mapping causal interregional influences with concurrent TMS-fMRI. Exp. Brain Res. 191, 383–402. doi: 10.1007/s00221-008-1601-8
Bestmann, S. R., Ruff, C. C., Driver, J., and Blankenburg, F. (2008b). “Concurrent TMS and functional magnetic resonance imaging: methods and current advances,” in Oxford Handbook of Transcranial Stimulation, eds E. Wasserman, C. Epstein and U. Ziemann (Oxford, UK: Oxford University Press), 569–592.
Bestmann, S., Swayne, O., Blankenburg, F., Ruff, C. C., Haggard, P., Weiskopf, N., et al. (2008c). Dorsal premotor cortex exerts state-dependent causal influences on activity in contralateral primary motor and dorsal premotor cortex. Cereb. Cortex 18, 1281–1291. doi: 10.1093/cercor/bhm159
Beynel, L., Powers, J. P., and Appelbaum, L. G. (2020). Effects of repetitive transcranial magnetic stimulation on resting-state connectivity: a systematic review. NeuroImage 211:116596. doi: 10.1016/j.neuroimage.2020.116596
Bijsterbosch, J. D., Barker, A. T., Lee, K.-H., and Woodruff, P. W. R. (2012). Where does transcranial magnetic stimulation (TMS) stimulate? Modelling of induced field maps for some common cortical and cerebellar targets. Med. Biol. Eng. Comput. 50, 671–681. doi: 10.1007/s11517-012-0922-8
Blankenburg, F., Ruff, C. C., Bestmann, S., Bjoertomt, O., Eshel, N., Josephs, O., et al. (2008). Interhemispheric effect of parietal TMS on somatosensory response confirmed directly with concurrent TMS-fMRI. J. Neurosci. 28, 13202–13208. doi: 10.1523/JNEUROSCI.3043-08.2008
Bolognini, N., and Ro, T. (2010). Transcranial magnetic stimulation: disrupting neural activity to alter and assess brain function. J. Neurosci. 30, 9647–9650. doi: 10.1523/JNEUROSCI.1990-10.2010
Bor, D., Schwartzman, D. J., Barrett, A. B., and Seth, A. K. (2017). Theta-burst transcranial magnetic stimulation to the prefrontal or parietal cortex does not impair metacognitive visual awareness. PLoS One 12:e0171793. doi: 10.1371/journal.pone.0171793
Bourgeois, A., Chica, A. B., Valero-Cabré, A., and Bartolomeo, P. (2013). Cortical control of Inhibition of Return: exploring the causal contributions of the left parietal cortex. Cortex 49, 2927–2934. doi: 10.1016/j.cortex.2013.08.004
Broadbent, H. J., van den Eynde, F., Guillaume, S., Hanif, E. L., Stahl, D., David, A. S., et al. (2011). Blinding success of rTMS applied to the dorsolateral prefrontal cortex in randomised sham-controlled trials: a systematic review. The World Journal of Biological Psychiatry: The Official Journal of the World Federation of Societies of Biological Psychiatry 12, 240–248. doi: 10.3109/15622975.2010.541281
Burke, M. J., Kaptchuk, T. J., and Pascual-Leone, A. (2019). Challenges of differential placebo effects in contemporary medicine: the example of brain stimulation. Ann. Neurol. 85, 12–20. doi: 10.1002/ana.25387
Busan, P., Barbera, C., Semenic, M., Monti, F., Pizzolato, G., Pelamatti, G., et al. (2009). Effect of transcranial magnetic stimulation (TMS) on parietal and premotor cortex during planning of reaching movements. PLoS One 4:e4621. doi: 10.1371/journal.pone.0004621
Capotosto, P., Babiloni, C., Romani, G. L., and Corbetta, M. (2012). Differential contribution of right and left parietal cortex to the control of spatial attention: a simultaneous EEG-rTMS study. Cereb. Cortex 22, 446–454. doi: 10.1093/cercor/bhr127
Cappelletti, M., Muggleton, N., and Walsh, V. (2009). Quantity without numbers and numbers without quantity in the parietal cortex. NeuroImage 46, 522–529. doi: 10.1016/j.neuroimage.2009.02.016
Carmel, D., Walsh, V., Lavie, N., and Rees, G. (2010). A causal role for right parietal cortex in binocular rivalry demonstrated with TMS. J. Vision 8:790. doi: 10.1167/8.6.790
Choi, K. M., Choi, S.-H., Lee, S. M., Jang, K.-I., and Chae, J.-H. (2019). Three weeks of rTMS treatment maintains clinical improvement but not electrophysiological changes in patients with depression: a 6-week follow-up pilot study. Front. Psychiatry 10:351. doi: 10.3389/fpsyt.2019.00351
Choi, K. M., Jang, K.-M., Jang, K. I., Um, Y. H., Kim, M.-S., Kim, D.-W., et al. (2014). The effects of 3 weeks of rTMS treatment on P200 amplitude in patients with depression. Neurosci. Lett. 577, 22–27. doi: 10.1016/j.neulet.2014.06.003
Chung, S. W., Rogasch, N. C., Hoy, K. E., and Fitzgerald, P. B. (2015). Measuring brain stimulation induced changes in cortical properties using TMS-EEG. Brain Stimul. 8, 1010–1020. doi: 10.1016/j.brs.2015.07.029
Chung, S. W., Rogasch, N. C., Hoy, K. E., Sullivan, C. M., Cash, R. F. H., and Fitzgerald, P. B. (2018). Impact of different intensities of intermittent theta burst stimulation on the cortical properties during TMS-EEG and working memory performance. Hum. Brain Mapp. 39, 783–802. doi: 10.1002/hbm.23882
Conde, V., Tomasevic, L., Akopian, I., Stanek, K., Saturnino, G. B., Thielscher, A., et al. (2019). The non-transcranial TMS-evoked potential is an inherent source of ambiguity in TMS-EEG studies. NeuroImage 185, 300–312. doi: 10.1016/j.neuroimage.2018.10.052
de Graaf, T. A., Jacobs, C., Roebroeck, A., and Sack, A. T. (2009). FMRI effective connectivity and TMS chronometry: complementary accounts of causality in the visuospatial judgment network. PLoS One 4:e8307. doi: 10.1371/journal.pone.0008307
de Graaf, T. A., and Sack, A. T. (2011). Null results in TMS: from absence of evidence to evidence of absence. Neurosci. Biobehav. Rev. 35, 871–877. doi: 10.1016/j.neubiorev.2010.10.006
Deng, Z.-D., Lisanby, S. H., and Peterchev, A. V. (2013). Electric field depth-focality tradeoff in transcranial magnetic stimulation: simulation comparison of 50 coil designs. Brain Stimul. 6, 1–13. doi: 10.1016/j.brs.2012.02.005
De Ridder, D., De Mulder, G., Verstraeten, E., Sunaert, S., and Moller, A. (2007). Somatosensory cortex stimulation for deafferentation pain. Acta Neurochir. Suppl. 97, 67–74. doi: 10.1007/978-3-211-33081-4_8
Downar, J., Blumberger, D. M., and Daskalakis, Z. J. (2016). The neural crossroads of psychiatric illness: an emerging target for brain stimulation. Trends Cogn. Sci. 20, 107–120. doi: 10.1016/j.tics.2015.10.007
Duecker, F., de Graaf, T. A., Jacobs, C., and Sack, A. T. (2013). Time- and task-dependent non-neural effects of real and sham TMS. PLoS One 8:e73813. doi: 10.1371/journal.pone.0073813
Duecker, F., and Sack, A. T. (2015). Rethinking the role of sham TMS. Front. Psychol. 6:210. doi: 10.3389/fpsyg.2015.00210
Dunn, J. C., and Kirsner, K. (2003). What can we infer from double dissociations? Cortex 39, 1–7. doi: 10.1016/s0010-9452(08)70070-4
Farahani, F. V., Karwowski, W., and Lighthall, N. R. (2019). Application of graph theory for identifying connectivity patterns in human brain networks: a systematic review. Front. Neurosci. 13:585. doi: 10.3389/fnins.2019.00585
Fitzgerald, P. B., Brown, T. L., Daskalakis, Z. J., Chen, R., and Kulkarni, J. (2002). Intensity-dependent effects of 1 Hz rTMS on human corticospinal excitability. Clin. Neurophysiol. 113, 1136–1141. doi: 10.1016/s1388-2457(02)00145-1
Flanagan, S. D., Beethe, A. Z., Eagle, S. R., Proessl, F., Connaboy, C., Dunn-Lewis, C., et al. (2019). Blinding success of sham-controlled motor cortex intermittent theta burst stimulation based on participant perceptions. Brain Stimul. 12, 1058–1060. doi: 10.1016/j.brs.2019.03.004
Friston, K., Moran, R., and Seth, A. K. (2013). Analysing connectivity with Granger causality and dynamic causal modelling. Curr. Opin. Neurobiol. 23, 172–178. doi: 10.1016/j.conb.2012.11.010
Furubayashi, T., Mochizuki, H., Terao, Y., Arai, N., Hanajima, R., Hamada, M., et al. (2013). Cortical hemoglobin concentration changes underneath the coil after single-pulse transcranial magnetic stimulation: a near-infrared spectroscopy study. J. Neurophysiol. 109, 1626–1637. doi: 10.1152/jn.00980.2011
Gann, M. A., King, B. R., Dolfen, N., Veldman, M. P., Chan, K. L., Puts, N. A. J., et al. (2020). Hippocampal and striatal responses during motor learning are modulated by prefrontal cortex stimulation. bioRxiv [Preprint]. doi: 10.1101/2020.06.05.136531
Ghabra, M. B., Hallett, M., and Wassermann, E. M. (1999). Simultaneous repetitive transcranial magnetic stimulation does not speed fine movement in PD. Neurology 52, 768–770. doi: 10.1212/wnl.52.4.768
Gratton, C., Lee, T. G., Nomura, E. M., and D’Esposito, M. (2013). The effect of theta-burst TMS on cognitive control networks measured with resting state fMRI. Front. Syst. Neurosci. 7:124. doi: 10.3389/fnsys.2013.00124
Hannula, H., and Ilmoniemi, R. J. (2017). “Basic principles of navigated TMS,” in Navigated Transcranial Magnetic Stimulation in Neurosurgery, ed S. M. Krieg (Cham: Springer), 3–29. doi: 10.1007/978-3-319-54918-7_1
Hartwigsen, G. (2015). The neurophysiology of language: insights from non-invasive brain stimulation in the healthy human brain. Brain Lang. 148, 81–94. doi: 10.1016/j.bandl.2014.10.007
Hayward, G., Mehta, M. A., Harmer, C., Spinks, T. J., Grasby, P. M., and Goodwin, G. M. (2007). Exploring the physiological effects of double-cone coil TMS over the medial frontal cortex on the anterior cingulate cortex: an H215O PET study. Eur. J. Neurosci. 25, 2224–2233. doi: 10.1111/j.1460-9568.2007.05430.x
Heller, L., and van Hulsteyn, D. B. (1992). Brain stimulation using electromagnetic sources: theoretical aspects. Biophys. J. 63, 129–138. doi: 10.1016/S0006-3495(92)81587-4
Hill, A. B. (1965). The environment and disease: association or causation? Proc. R. Soc. Med. 58, 295–300.
Holmes, N. P., and Meteyard, L. (2018). Subjective discomfort of TMS predicts reaction times differences in published studies. Front. Psychol. 9:1989. doi: 10.3389/fpsyg.2018.01989
Huang, Y.-Z., Edwards, M. J., Rounis, E., Bhatia, K. P., and Rothwell, J. C. (2005). Theta burst stimulation of the human motor cortex. Neuron 45, 201–206. doi: 10.1016/j.neuron.2004.12.033
Huber, R., Mäki, H., Rosanova, M., Casarotto, S., Canali, P., Casali, A. G., et al. (2013). Human cortical excitability increases with time awake. Cereb. Cortex 23, 332–338. doi: 10.1093/cercor/bhs014
Iwabuchi, S. J., Auer, D. P., Lankappa, S. T., and Palaniyappan, L. (2019). Baseline effective connectivity predicts response to repetitive transcranial magnetic stimulation in patients with treatment-resistant depression. Eur. Neuropsychopharmacol. 29, 681–690. doi: 10.1016/j.euroneuro.2019.02.012
Izuma, K., Akula, S., Murayama, K., Wu, D.-A., Iacoboni, M., and Adolphs, R. (2015). A causal role for posterior medial frontal cortex in choice-induced preference change. J. Neurosci. 35, 3598–3606. doi: 10.1523/JNEUROSCI.4591-14.2015
Jung, J., Bungert, A., Bowtell, R., and Jackson, S. R. (2016). Vertex stimulation as a control site for transcranial magnetic stimulation: a concurrent TMS/fMRI study. Brain Stimul. 9, 58–64. doi: 10.1016/j.brs.2015.09.008
Jung, N. H., Delvendahl, I., Kuhnke, N. G., Hauschke, D., Stolle, S., and Mall, V. (2010). Navigated transcranial magnetic stimulation does not decrease the variability of motor-evoked potentials. Brain Stimul. 3, 87–94. doi: 10.1016/j.brs.2009.10.003
Kang, J. I., Lee, H., Jhung, K., Kim, K. R., An, S. K., Yoon, K.-J., et al. (2016). Frontostriatal connectivity changes in major depressive disorder after repetitive transcranial magnetic stimulation: a randomized sham-controlled study. J. Clin. Psychiatry 77, e1137–e1143. doi: 10.4088/JCP.15m10110
Karabanov, A., Ziemann, U., Hamada, M., George, M. S., Quartarone, A., Classen, J., et al. (2015). Consensus paper: probing homeostatic plasticity of human cortex with non-invasive transcranial brain stimulation. Brain Stimul. 8, 442–454. doi: 10.1016/j.brs.2015.01.404
Lefaucheur, J.-P., Aleman, A., Baeken, C., Benninger, D. H., Brunelin, J., Di Lazzaro, V., et al. (2020). Evidence-based guidelines on the therapeutic use of repetitive transcranial magnetic stimulation (rTMS): an update (2014–2018). Clin. Neurophysiol. 131, 474–528. doi: 10.1016/j.clinph.2019.11.002
Li, X., Nahas, Z., Kozel, F. A., Anderson, B., Bohning, D. E., and George, M. S. (2004). Acute left prefrontal transcranial magnetic stimulation in depressed patients is associated with immediately increased activity in prefrontal cortical as well as subcortical regions. Biol. Psychiatry 55, 882–890. doi: 10.1016/j.biopsych.2004.01.017
Lisanby, S. H., Gutman, D., Luber, B., Schroeder, C., and Sackeim, H. A. (2001). Sham TMS: intracerebral measurement of the induced electrical field and the induction of motor-evoked potentials. Biol. Psychiatry 49, 460–463. doi: 10.1016/s0006-3223(00)01110-0
Loo, C. K., Taylor, J. L., Gandevia, S. C., McDarmont, B. N., Mitchell, P. B., and Sachdev, P. S. (2000). Transcranial magnetic stimulation (TMS) in controlled treatment studies: are some “sham” forms active? Biol. Psychiatry 47, 325–331. doi: 10.1016/s0006-3223(99)00285-1
Machery, E. (2012). Dissociations in neuropsychology and cognitive neuroscience. Philos. Sci. 79, 490–518.
Massimini, M., Ferrarelli, F., Huber, R., Esser, S. K., Singh, H., and Tononi, G. (2005). Breakdown of cortical effective connectivity during sleep. Science 309, 2228–2232. doi: 10.1126/science.1117256
Montefinese, M., Turco, C., Piccione, F., and Semenza, C. (2017). Causal role of the posterior parietal cortex for two-digit mental subtraction and addition: a repetitive TMS study. NeuroImage 155, 72–81. doi: 10.1016/j.neuroimage.2017.04.058
Pearl, J. (2000). Causality: Models, Reasoning, and Inference. New York, NY: Cambridge University Press.
Pessoa, L. (2014). Understanding brain networks and brain organization. Phys. Life Rev. 11, 400–435. doi: 10.1016/j.plrev.2014.03.005
Philiastides, M. G., Auksztulewicz, R., Heekeren, H. R., and Blankenburg, F. (2011). Causal role of dorsolateral prefrontal cortex in human perceptual decision making. Curr. Biol. 21, 980–983. doi: 10.1016/j.cub.2011.04.034
Poeppl, T. B., Langguth, B., Lehner, A., Frodl, T., Rupprecht, R., Kreuzer, P. M., et al. (2018). Brain stimulation-induced neuroplasticity underlying therapeutic response in phantom sounds. Hum. Brain Mapp. 39, 554–562. doi: 10.1002/hbm.23864
Polanía, R., Nitsche, M. A., and Ruff, C. C. (2018). Studying and modifying brain function with non-invasive brain stimulation. Nat. Neurosci. 21, 174–187. doi: 10.1038/s41593-017-0054-4
Pollatos, O., Herbert, B. M., Mai, S., and Kammer, T. (2016). Changes in interoceptive processes following brain stimulation. Philos. Trans. R. Soc. B Biol. Sci. 371:20160016. doi: 10.1098/rstb.2016.0016
Poulet, E., Brunelin, J., Boeuve, C., Lerond, J., D’Amato, T., Dalery, J., et al. (2004). Repetitive transcranial magnetic stimulation does not potentiate antidepressant treatment. Eur. Psychiatry 19, 382–383. doi: 10.1016/j.eurpsy.2004.06.021
Reithler, J., Peters, J. C., and Sack, A. T. (2011). Multimodal transcranial magnetic stimulation: using concurrent neuroimaging to reveal the neural network dynamics of noninvasive brain stimulation. Prog. Neurobiol. 94, 149–165. doi: 10.1016/j.pneurobio.2011.04.004
Robertson, E. M., Théoret, H., and Pascual-Leone, A. (2003). Studies in cognition: the problems solved and created by transcranial magnetic stimulation. J. Cogn. Neurosci. 15, 948–960. doi: 10.1162/089892903770007344
Romei, V., Thut, G., and Silvanto, J. (2016). Information-based approaches of noninvasive transcranial brain stimulation. Trends Neurosci. 39, 782–795. doi: 10.1016/j.tins.2016.09.001
Rossi, S., Innocenti, I., Polizzotto, N. R., Feurra, M., De Capua, A., Ulivelli, M., et al. (2011). Temporal dynamics of memory trace formation in the human prefrontal cortex. Cereb. Cortex 21, 368–373. doi: 10.1093/cercor/bhq103
Roth, Y., Amir, A., Levkovitz, Y., and Zangen, A. (2007). Three-dimensional distribution of the electric field induced in the brain by transcranial magnetic stimulation using figure-8 and deep H-coils. J. Clin. Neurophysiol. 24, 31–38. doi: 10.1097/WNP.0b013e31802fa393
Ruff, C. C., Bestmann, S., Blankenburg, F., Bjoertomt, O., Josephs, O., Weiskopf, N., et al. (2008). Distinct causal influences of parietal versus frontal areas on human visual cortex: evidence from concurrent TMS-fMRI. Cereb. Cortex 18, 817–827. doi: 10.1093/cercor/bhm128
Ruff, C. C., Driver, J., and Bestmann, S. (2009). Combining TMS and fMRI: from “virtual lesions” to functional-network accounts of cognition. Cortex 45, 1043–1049. doi: 10.1016/j.cortex.2008.10.012
Sack, A. T. (2006). Transcranial magnetic stimulation, causal structure-function mapping and networks of functional relevance. Curr. Opin. Neurobiol. 16, 593–599. doi: 10.1016/j.conb.2006.06.016
Salillas, E., Basso, D., Baldi, M., Semenza, C., and Vecchi, T. (2009). Motion on numbers: transcranial magnetic stimulation on the ventral intraparietal sulcus alters both numerical and motion processes. J. Cogn. Neurosci. 21, 2129–2138. doi: 10.1162/jocn.2008.21157
Schaal, N. K., Williamson, V. J., Kelly, M., Muggleton, N. G., Pollok, B., Krause, V., et al. (2015). A causal involvement of the left supramarginal gyrus during the retention of musical pitches. Cortex 64, 310–317. doi: 10.1016/j.cortex.2014.11.011
Schutter, D. J. L. G., Van Honk, J., and Panksepp, J. (2004). Introducing transcranial magnetic stimulation (TMS) and its property of causal inference in investigating brain–function relationships. Synthese 141, 155–173. doi: 10.1023/b:synt.0000042951.25087.16
Siebner, H. R., Filipovic, S. R., Rowe, J. B., Cordivari, C., Gerschlager, W., Rothwell, J. C., et al. (2003). Patients with focal arm dystonia have increased sensitivity to slow-frequency repetitive TMS of the dorsal premotor cortex. Brain 126, 2710–2725. doi: 10.1093/brain/awg282
Siebner, H. R., Hartwigsen, G., Kassuba, T., and Rothwell, J. C. (2009). How does transcranial magnetic stimulation modify neuronal activity in the brain? Implications for studies of cognition. Cortex 45, 1035–1042. doi: 10.1016/j.cortex.2009.02.007
Siebner, H. R., Peller, M., Willoch, F., Minoshima, S., Boecker, H., Auer, C., et al. (2000). Lasting cortical activation after repetitive TMS of the motor cortex: a glucose metabolic study. Neurology 54, 956–963. doi: 10.1212/wnl.54.4.956
Siebner, H. R., and Rothwell, J. (2003). Transcranial magnetic stimulation: new insights into representational cortical plasticity. Exp. Brain Res. 148, 1–16. doi: 10.1007/s00221-002-1234-2
Siuda-Krzywicka, K., Bola, Ł., Paplińska, M., Sumera, E., Jednoróg, K., Marchewka, A., et al. (2016). Massive cortical reorganization in sighted Braille readers. eLife 5:e10762. doi: 10.7554/eLife.10762
Śliwińska, M. W., Vitello, S., and Devlin, J. T. (2014). Transcranial magnetic stimulation for investigating causal brain-behavioral relationships and their time course. J. Vis. Exp. 89:51735. doi: 10.3791/51735
Speer, A. M., Benson, B. E., Kimbrell, T. K., Wassermann, E. M., Willis, M. W., Herscovitch, P., et al. (2009). Opposite effects of high and low frequency rTMS on mood in depressed patients: relationship to baseline cerebral activity on PET. J. Affect. Disord. 115, 386–394. doi: 10.1016/j.jad.2008.10.006
Speer, A. M., Kimbrell, T. A., Wassermann, E. M., Repella, J. D., Willis, M. W., Herscovitch, P., et al. (2000). Opposite effects of high and low frequency rTMS on regional brain activity in depressed patients. Biol. Psychiatry 48, 1133–1141. doi: 10.1016/s0006-3223(00)01065-9
Strafella, A. P., Paus, T., Fraraccio, M., and Dagher, A. (2003). Striatal dopamine release induced by repetitive transcranial magnetic stimulation of the human motor cortex. Brain 126, 2609–2615. doi: 10.1093/brain/awg268
Thickbroom, G. W. (2007). Transcranial magnetic stimulation and synaptic plasticity: experimental framework and human models. Exp. Brain Res. 180, 583–593. doi: 10.1007/s00221-007-0991-3
Thut, G., and Pascual-Leone, A. (2010). A review of combined TMS-EEG studies to characterize lasting effects of repetitive TMS and assess their usefulness in cognitive and clinical neuroscience. Brain Topogr. 22, 219–232. doi: 10.1007/s10548-009-0115-4
Todd, G., Flavel, S. C., and Ridding, M. C. (2009). Priming theta-burst repetitive transcranial magnetic stimulation with low- and high-frequency stimulation. Exp. Brain Res. 195, 307–315. doi: 10.1007/s00221-009-1791-8
Valdes-Sosa, P. A., Roebroeck, A., Daunizeau, J., and Friston, K. (2011). Effective connectivity: influence, causality and biophysical modeling. NeuroImage 58, 339–361. doi: 10.1016/j.neuroimage.2011.03.058
Valero-Cabré, A., Amengual, J. L., Stengel, C., Pascual-Leone, A., and Coubard, O. A. (2017). Transcranial magnetic stimulation in basic and clinical neuroscience: a comprehensive review of fundamental principles and novel insights. Neurosci. Biobehav. Rev. 83, 381–404. doi: 10.1016/j.neubiorev.2017.10.006
Veniero, D., Strüber, D., Thut, G., and Herrmann, C. S. (2019). Noninvasive brain stimulation techniques can modulate cognitive processing. Organ. Res. Methods 22, 116–147. doi: 10.1177/1094428116658960
Weise, K., Numssen, O., Thielscher, A., Hartwigsen, G., and Knösche, T. R. (2020). A novel approach to localize cortical TMS effects. NeuroImage 209:116486. doi: 10.1016/j.neuroimage.2019.116486
Zanto, T. P., Rubens, M. T., Thangavel, A., and Gazzaley, A. (2011). Causal role of the prefrontal cortex in top-down modulation of visual processing and working memory. Nat. Neurosci. 14, 656–661. doi: 10.1038/nn.2773
Keywords: causal inferences, brain plasticity, brain excitability, repetitive TMS, TMS-neuroimaging
Citation: Hobot J, Klincewicz M, Sandberg K and Wierzchoń M (2021) Causal Inferences in Repetitive Transcranial Magnetic Stimulation Research: Challenges and Perspectives. Front. Hum. Neurosci. 14:586448. doi: 10.3389/fnhum.2020.586448
Received: 23 July 2020; Accepted: 30 November 2020;
Published: 14 January 2021.
Edited by:
Nivethida Thirugnanasambandam, National Brain Research Centre (NBRC), IndiaReviewed by:
Urvakhsh Meherwan Mehta, National Institutes of Health (NIH), United StatesKyung Mook Choi, Korea University, South Korea
Copyright © 2021 Hobot, Klincewicz, Sandberg and Wierzchoń. This is an open-access article distributed under the terms of the Creative Commons Attribution License (CC BY). The use, distribution or reproduction in other forums is permitted, provided the original author(s) and the copyright owner(s) are credited and that the original publication in this journal is cited, in accordance with accepted academic practice. No use, distribution or reproduction is permitted which does not comply with these terms.
*Correspondence: Justyna Hobot, justyna.hobot@doctoral.uj.edu.pl