- Department of Neurology, Danish Dementia Research Centre, Rigshospitalet, University of Copenhagen, Copenhagen, Denmark
Background: Exercise has been shown to alter brain plasticity and is explored as a therapeutic intervention in a wide variety of neurological diseases. Electroencephalography (EEG) offers an inexpensive method of studying brain electrocortical activity shortly after exercise and thus offers a way of exploring the influence of exercise on the brain. We conducted a systematic review to summarize the current body of evidence regarding methods of EEG analysis and the reported effects of exercise interventions on EEG.
Methods: PubMed, Web of Science and EMBASE were searched for studies investigating resting state EEG in exercise intervention studies carried out in participants >17 years of age and with no history of epilepsy. Further, studies solely investigating event-related potentials as an outcome measure were excluded. Relevant data were extracted, and a risk-of-bias assessment was carried out using the Cochrane risk-of-bias tool. A qualitative synthesis of results was carried out. A protocol for the systematic review was uploaded to https://www.crd.york.ac.uk/PROSPERO/ (ID: CRD42019134570) and the Preferred Reporting Items for Systematic Reviews (PRISMA) statement was followed.
Results: Out of 1,993 records screened, 54 studies were included in a final qualitative synthesis with a total of 1,445 participants. Our synthesis showed that studies were mainly carried out using frequency analysis as an analytical method. Generally, findings across studies were inconsistent and few were adjusted for multiple comparisons. Studies were mainly of low quality and usually carried out in small populations, lowering the significance of results reported.
Conclusions: Changes in the EEG as a result of an exercise intervention are elusive and difficult to replicate. Future studies should provide biologically sound hypotheses underlying assumptions, include larger populations and use standardized EEG methods to increase replicability. EEG remains an interesting methodology to examine the effects of exercise on the brain.
Introduction
There is increasing evidence that exercise may have a profound effect on brain health (Barha et al., 2017). The connection between the brain and exercise is further corroborated by observed changes in brain circuits through altering of the synaptic plasticity after exercise, and possible induction of neurogenesis in the hippocampus by signaling molecules such as brain-derived neurotrophic factor and insulin-like growth factor-1 involved in these processes (Pedersen and Saltin, 2015; Cassilhas et al., 2016; Mellow et al., 2019). Exercise is currently being explored as a therapeutic option in a wide variety of brain diseases, and meta-analyses of findings have showed positive effects on anxiety and depression (Wegner et al., 2014). There is also evidence of a preventive effect of exercise on dementia (Pedersen and Saltin, 2015), while the therapeutic effect on manifest dementia such as Alzheimer's disease is more elusive and will need further research with better study designs (Frederiksen et al., 2018, 2019). One aspect of the influence of exercise on the brain is the idea of a runner's high, which is described as an anxiolytic, euphoric feeling, that is reported by long-distance runners (Hicks et al., 2019). The biochemical pathways underlying this phenomenon is currently being investigated, and reports point to an increase in plasma endocannabinoids that bind to cannabinoid receptors in the brain (Sparling et al., 2003; Fuss et al., 2015). Cannabinoid agonists have recently been shown to alter neural oscillatory activity (Skosnik et al., 2018), thus making it probable that changes in brain oscillations might be a reflection of this effect of exercise.
The sympathetic nervous system is activated during exercise (Christensen and Galbo, 1983). High intensity exercise leads to an increase in circulating cortisol immediately following exercise (Hill et al., 2008), which in resting individuals has been shown to affect brain oscillatory activity (Chapotot et al., 1998). Animal studies have recently shown that adrenergic modulation alters the dendritic excitability in cortical neurons in mice (Labarrera et al., 2018) and strengthens functional connectivity in the pre-frontal cortex in rhesus monkeys (Wang et al., 2007). This coupling between exercise, activation of the sympathetic nervous system and changes in the brain indicates that it might be feasible to measure an effect of exercise by recording the electrocortical activity in humans.
Some researchers link the beneficial effects of exercise to evolutionary features of humans, whereby exercise at a moderate intensity was necessary in hunter-gatherer communities and selection for this sort of activity has been pivotal in the evolution of the modern humans. This can teleologically explain the posited therapeutic effect of exercise in certain neurological disorders (Raichlen and Alexander, 2017).
EEG offers a non-invasive, cheap and easily applied method to study brain electrocortical activity (Rossini et al., 2019). As EEG can be applied shortly after an exercise intervention, it makes it possible to study temporary changes in electrocortical activity. However, it is difficult to capture, without massive influence of movement artifacts, the electrocortical activity during exercise, which represents a challenge for this field, although advances are being made to overcome this issue (Gwin et al., 2010). EEG analysis methods have also been developed to localize the anatomical brain substrate responsible for the signal that can be recorded from the scalp, thus enabling researchers to accurately localize the involved brain regions in e.g. exercise (Pascual-Marqui et al., 1994). Magnetoencephalography (MEG), although more costly to apply, has much of the same properties as EEG, and may be superior in source localization (Cohen and Cuffin, 1983). MEG has a preference for capturing cortical activity in the sulcis of the brain by the recording of tangential electromagnetic fields generated in the sulcal walls (Baillet, 2017). Both EEG and MEG thus offer unique methods for exploring the possible effect of exercise on the brain. Another mentionable method of investigation of brain-exercise dynamics is functional near-infrared spectroscopy (fNIRS) (Herold et al., 2018). Although beyond the scope of this review, this method offers good temporal resolution of brain oxygenation and hemodynamics related to exercise and could be joined with EEG/MEG for assessing hemodynamic associations with phenomena recorded using EEG/MEG (Herold et al., 2018).
To our knowledge, no systematic review exists on the effects of exercise interventions on EEG or MEG. Previous reviews have focused on the affective part of exercise (Lattari et al., 2014) and another review mainly included studies that could be assessed by meta-analytic methods (Crabbe and Dishman, 2004). We thus conducted a comprehensive systematic review to summarize (1) the current body of evidence regarding the effects observed in exercise intervention studies and (2) analytical methods used to quantify these.
Rationale
Since exercise may alter brain plasticity and possibly brain circuits, which might be reflected in EEG or MEG recordings, we wanted to search the literature for studies reporting on EEG or MEG in exercise interventions.
Objectives
To summarize studies reporting on the effects on the EEG/MEG and methods used to analyze resting state EEG/MEG in exercise intervention studies in healthy and diseased individuals.
Research Question
What are the effects of exercise EEG/MEG derived measures of brain activation and by what methods is the EEG/MEG analyzed in exercise intervention studies?
Methods
Study Design
We performed a systematic review in accordance with the guidelines provided by the Preferred Reporting Items in Systematic Reviews (PRISMA) statement (Moher et al., 2009).
Participants, Interventions, Comparators
We included single group, cross-over or parallel group studies with both randomized and non-randomized allocation involving participants who were above the age of 17 years, with no history of epilepsy or sleep disorders. Only full research articles were included. Exercise interventions could be either acute (single bout) or chronic exercise interventions (≥2-weeks of duration). The outcome assessed was changes in the EEG signal or changes in the MEG, which could be analyzed in any way. We excluded studies involving event-related potentials and studies involving sleep EEG.
Systematic Review Protocol
A systematic review protocol was registered 30th of August 2019 in the PROSPERO database (PROSPERO ID: CRD42019134570) (https://www.crd.york.ac.uk/prospero/).
Search Strategy
A search for (“EEG” OR “MEG”) AND “exercise” was carried out. Detailed search strings for the three databases searched are uploaded as Supplementary Material.
Data Sources, Study Selection, and Data Extraction
We searched PubMed (MEDLINE), EMBASE, and Web of Science for records using the previously mentioned search strings. Final searches were conducted 25th of September 2019. Two of the authors, KF and MG, independently screened titles and abstracts and full-text articles. Any disagreements were resolved by discussion and no third party was involved in the selection of studies. Relevant data was extracted by the same authors (MG and KF) using an Excel data extraction sheet that was piloted in three studies before being applied to the rest of the studies. The following items were extracted from the studies:
Study design, comparator (if applicable), age and sex of participants, number of participants, intervention characteristics (type of intervention, length of intervention, intensity of aerobic exercise), EEG/MEG methods, EEG/MEG software, EEG/MEG metrics (e.g., connectivity, modulation of frequency), EEG/MEG paradigm, statistical analysis methods, diagnosis of participants, reported effect of the intervention on EEG/MEG.
A risk-of-bias assessment using version 2 of the revised Cochrane risk-of-bias tool for randomized trials was carried out on included studies (Sterne et al., 2019).
Data Analysis
A qualitative synthesis of results was done as according to protocol. Due to expected large heterogeneity in the studied outcomes, interventions and populations, we did not plan to carry out a meta-analysis nor was this done as a post-hoc analysis due to the aforementioned reasons. Throughout the manuscript, reported results from included studies are only defined as statistically significant if the included study reported a P < 0.05 for the finding. Additionally, we extracted data on whether studies adjusted for multiple comparisons (Benjamini-Hochberg, Bonferroni-Holm, etc.) and denoted studies that specifically reported this.
Results
Study Selection and Characteristics
The study selection process is outlined in Figure 1. A total of 2,250 records were identified through bibliographic searches and hand searches of included studies from which 54 studies with a complete total of 1,445 participants were included. Characteristics of the included studies are shown in Table 1. Of the 54 studies included, 43 studies included subjects who were younger than 50 years of age and 43 studies included participants who were healthy. In total, 40 studies investigated the acute effect of exercise. The study designs of the included studies were as follows: Single group (n = 20), parallel group (n = 5), cross-over (n = 12; of these, n = 4 were sequence randomized), non-randomized, controlled (n = 6), randomized, non-controlled (n = 5) and randomized, controlled (n = 7). In total, 22 studies were done on <20 participants. Four studies performed adequate statistical multiple comparison adjustment (Spring et al., 2017, 2018; Hübner et al., 2018; Devilbiss et al., 2019; Villafaina et al., 2019). It should be noted that these four studies were published recently. No studies reporting on MEG were found in our searches. Table 2 outlines elaborated EEG methods in the included studies.
Exercise Intervention Regimes and Post-intervention Analysis Time
The intervention methods in the included studies are reported in detail in Table 1 and post-intervention analysis intervals are reported in Table 2. A cycle ergometer was the most used exercise intervention instrument in acute interventions (N studies = 25), followed by treadmill (N = 9), track running (N = 3), outdoors running (N = 3), home-trainer (N = 2), arm crank (N = 2), walking (N = 1), wheelchair propulsion (N = 1). Most studies applied an acute intervention ≥30 min (N = 14). Other reported lengths of acute interventions were ≥20 min (N = 6), ≥15 min (N = 5), and one study reported applying the intervention for ≤ 10 min. Generally, studies used a marker for intervention intensity, which was often a percentage of maximal heart rate (MHR) or maximal oxygen consumption (VO2 max), while others reported using a graded intervention until volitional exhaustion or until a critical point (certain percentage of heart rate or VO2 max) was reached (N studies = 15) or used other markers. The reported VO2 max intensities at which the participants were expected to perform in the acute interventions were: 50–60% VO2 max (N = 5), 60–70% VO2 max (N = 2), 70–80% (N = 7), 80–90% (N = 4). The reported MHR intensities at which the participants were expected to perform in the acute interventions were: 60–70% MHR (N = 1), 70–80% MHR (N = 1), 80–90% (N = 3). The post-intervention analysis interval for both acute and chronic interventions was not stated in N = 16 studies. The post-intervention analysis interval was reported as being immediately afterwards in 17 studies, ≤ 5 min (N = 8), ≤ 10 min (N = 6), ≤ 15 min (N = 2), ≤ 20 min (N = 2), ≤ 25 min (N = 1).
The chronic interventions reported on differed to such a large degree that a meaningful summary of the interventions reported is best appreciated by studying Table 1, where chronic intervention studies can be found adjoined.
Analytical Methods of Included Studies
The most frequently reported method of analysis was frequency analysis, whereby the power of frequency bands was studied (N studies = 32, see Tables 1, 3–6 for listings of methods of the included studies). Other derivatives of frequency analysis in the studies included coherence (the correlation between hemispheres for a specific frequency band), laterality (quantifying the lateralization of power) and mean frequency. Another method of analysis frequently reported on was low resolution electromagnetic tomography (LORETA). The studies using LORETA all applied a standard neuroanatomical atlas on a 3D head model. Also studied was microstates (Spring et al., 2017, 2018). Lastly, brain connectivity was considered in one study (Zilidou et al., 2018). Brain connectivity was examined by the authors by applying the LORETA method, and further examining sources of activity as nodes in graph theory based understandings of brain networks (Rossini et al., 2019).
Frequency Analysis
Table 3 summarizes the main findings of studies using frequency analysis, while Table 4 reports the findings of studies from which the outcomes are derived from frequency analysis (asymmetry, coherence, peak shifts, laterality, wave proportion, and mean frequency). Figure 2 summarizes results reported in Table 3, for visual simplification. In total, 32 studies carried out a frequency analysis and within these studies the effects of exercise intervention on alpha and beta band activity were overrepresented (Table 3). Definitions for these bands differed between studies (Table 2). In total, 21 studies reported on derivatives of frequency analysis. Some studies reported on both frequency analysis and derivatives and LORETA, which means that the summed total number of studies reported here for each category is larger than the total number of studies included in the present review.
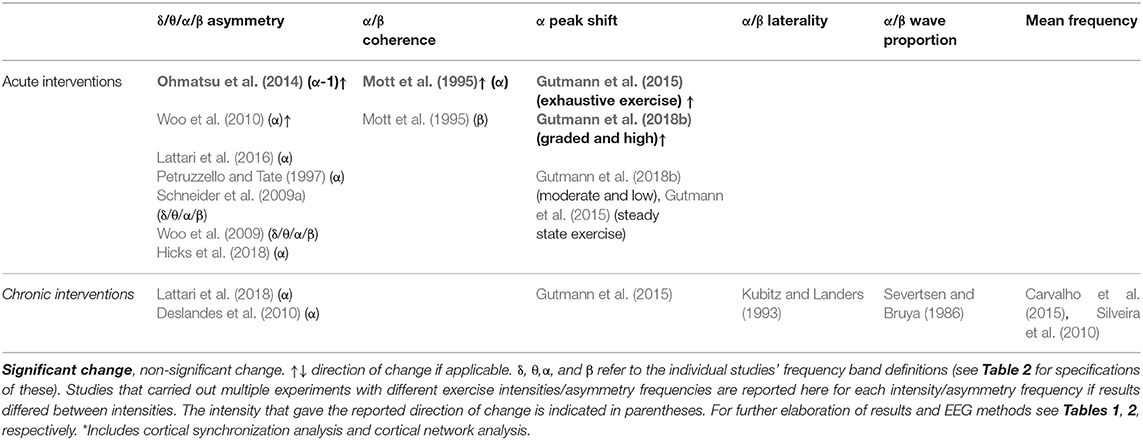
Table 4. A summary of main findings from frequency analysis derivatives of post- vs. pre-exercise measurements.
Acute Interventions
In total, 25 studies investigated frequency analysis in acute intervention studies. Several studies found significant increases in power within the delta, theta, alpha and beta band, and although there was a quantitative overweight of studies reporting increases, there were also studies that reported significant decreases for all the same bands and a high number of studies reported no significant changes (Table 3). No significant changes were reported within the gamma band (Schneider et al., 2009a; Devilbiss et al., 2019). The results by Devilbiss were adjusted for multiple comparisons.
Derivatives of frequency analysis
Table 4 gives the results of studies on derivatives of frequency analysis in acute interventions with hypothesis tests (N = 10). Most of these studies investigated asymmetry (N = 7). Two studies (Woo et al., 2010; Ohmatsu et al., 2014) reported significant increases in alpha asymmetry, while five studies reported no significant changes. Within the studies reporting no significant changes, multiple frequencies were investigated (delta, theta, alpha and beta) in two studies (Schneider et al., 2009a; Woo et al., 2009). An alpha peak shift was observed in two studies by the same group (Gutmann et al., 2015, 2018b), both only showed in high intensity settings.
Chronic Interventions
In total, seven studies reported on frequency analysis results in chronic exercise interventions (Tables 1, 3). Significant increases of alpha-2 (Amjad et al., 2019) and beta-3 bands (Villafaina et al., 2019) were reported, while significant decreases were found for delta (Amjad et al., 2019), and beta-1 (Amjad et al., 2019). Results by Villafaina et al. were adjusted for multiple comparisons. There were reports of no significant changes within all frequencies with an overweight of studies done on alpha and beta bands. No significant findings were reported within the theta band.
Derivatives of frequency analysis
No studies reported significant changes within derivatives of frequency analysis for chronic exercise interventions (Table 4) (N = 7). The investigated derivatives of power spectrum analyses were asymmetry, alpha peak shift, laterality, wave proportion, and mean frequency.
LORETA
In total, 12 studies reported using LORETA as an analytic method for resting state EEG measurements. Table 5 gives the summarized statistically significant results of these studies.
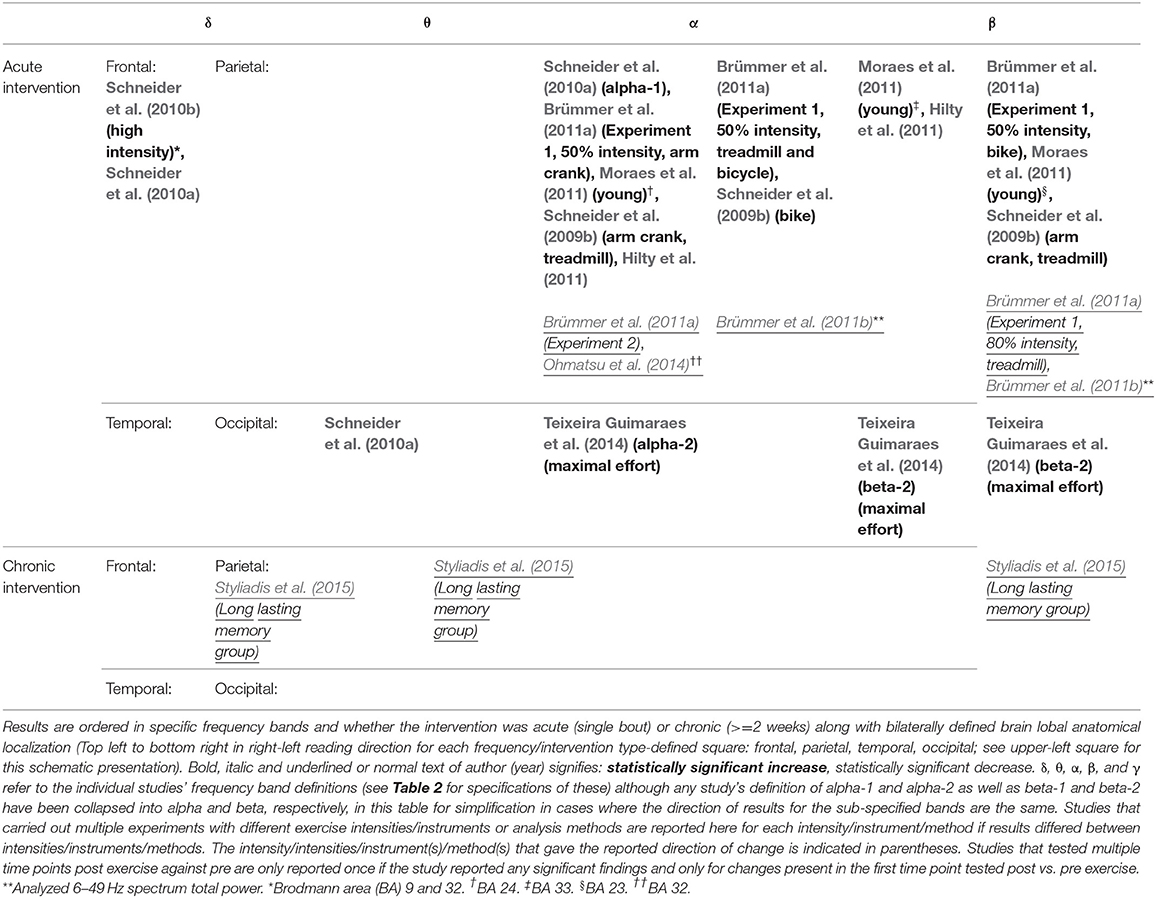
Table 5. A summary of main findings from studies using LORETA analysis of post- vs. pre-exercise measurements.
Acute Interventions
Ten studies investigated LORETA in conjunction with an acute exercise intervention. There were significant findings within all bands with an overweight of significant increases in the alpha and beta bands, which for alpha were found in all but the occipital lobe, whereas beta increases were found in all lobes (see Tables 1, 5). Significant decreases were found within the alpha band (frontal and parietal lobe) (Brümmer et al., 2011a,b; Ohmatsu et al., 2014) and beta band (parietal lobe) (Brümmer et al., 2011a,b). Changes were often localized to small brain areas (see Table 5), with inconsistent findings across studies with regards to anatomical localization of reported changes.
Chronic Interventions
Two studies applied LORETA to their data (Styliadis et al., 2015; Zilidou et al., 2018) in chronic intervention studies. Of these, one study reported significant findings. These were decreases in delta, theta and beta bands (all located to parietal lobe) (Styliadis et al., 2015).
Other Methods
Table 6 gives the results of studies investigating aspects of EEG analysis other than frequency analysis and LORETA.
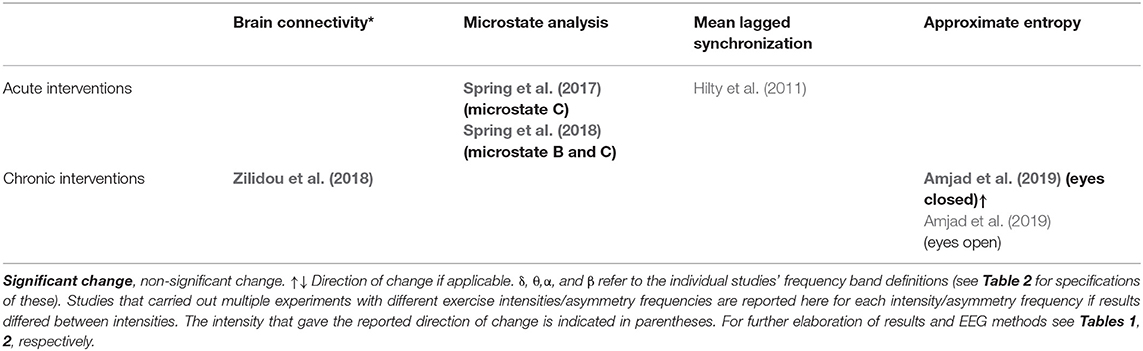
Table 6. A summary of main findings of other methods of analysis of post- vs. pre-exercise measurements.
Acute Interventions
In total, three studies reported findings from investigations involving microstate analysis and mean lagged synchronization. Studies on microstates used a definition of four distinct EEG microstates called A, B, C, and D, generally accepted in the literature (Lehmann et al., 2009). Significant findings were reported for microstate analysis, showing that the global variance explained (Spring et al., 2017), mean duration of Spring et al. (2017, 2018), and time coverage (Spring et al., 2017, 2018) for microstate C and mean duration of microstate B (Spring et al., 2018) were all increased after exercise.
Chronic Interventions
In total, two studies reported on investigations involving brain connectivity and approximate entropy. The authors of one study considered graph theory based brain networks before and after a 24-week traditional Greek dance program (Zilidou et al., 2018). Zilidou et al. modeled the cortex with 20,000 fixed dipoles which were grouped into 512 cortical regions of interest (ROIs) Significant time × intervention group interactions were found when measuring how much participants brain behaved as a small world, which essentially is an expression of local information processing and brain connectivity, when the threshold of connections between regions-of-interest (ROIs) were set at 10,000 and 12,500, which is an arbitrary measure used to model the network system. Several measures pertaining to graph theory were investigated including the shortest path length between two ROIs, which had a significant time × intervention group interaction, when a connection threshold of 15,000 was applied. Further, measurements of information flow through particular ROIs, the tendency of specific ROIs to behave as hubs for information, the connection distributions and connection strengths were similarly significantly changed within different brain networks. In another study, it was found that the approximate entropy, which is a measure of how complex a network is, was found to be increased in the eyes closed condition after a chronic exercise intervention (Amjad et al., 2019). We refer to the original studies for in-depth explanations of specific measures investigated in these included studies.
Risk of Bias
The summarized results of the risk of bias assessment for each study are shown in Figure 3. Figure 4 gives the percentages of individual assessments of specific domains addressed in the risk-of-bias tool. An overweight of studies were rated as having an overall high risk of bias (N = 41), whereas the rest of the included studies were rated as having some concerns overall (N = 13). No studies were rated as having low risk of bias within the domain pertaining to selection of the reported result. Overall bias ratings were mainly influenced by the domains “Selection of the reported result,” “Randomization process” (see Figure 4).
Discussion
Summary of Main Findings
We carried out a systematic review of studies investigating the effects of exercise interventions on resting state EEG/MEG. After screening, 54 studies were included in the final qualitative synthesis. Results of this synthesis showed that studies in general were small, and carried out in healthy, young individuals applying an acute exercise intervention. The most often used methodology for EEG analysis was frequency analysis and its derivatives. The results of summarized frequency analysis findings for the acute and chronic effects of exercise were inconsistent for most frequency bands. There was some indication of a delta band activity decrease after chronic interventions and a null effect in the theta and gamma band, and an alpha peak shift after acute exercise with high intensity. Anatomical source localization studies using LORETA reported few localized increases within the delta (frontal) and theta band (temporal) in acute exercise and decreases within delta and theta (both parietal) after chronic exercise interventions. Moreover, more widespread alpha and beta activity increases were shown after acute exercise, although decreases in some of these areas were also reported demonstrating the inconsistency across studies. Other methods of analyzing EEG data revealed significant changes in brain connectivity after a chronic dance intervention. Replicated changes of microstate C after acute exercise, which were adjusted for multiple comparisons, were also found, although not independently validated. In all but 13 studies, where there were some concerns regarding risk of bias, there was an overall high risk of bias indicating a low quality of studies. No studies were found reporting on MEG in exercise interventions.
In the present review, we aimed to include all available data on exercise interventions and resting state EEG measurements. Previous reviews on the subject have had other focus areas, such as the ability of EEG to predict mood states in exercise interventions (Lattari et al., 2014), and one review had the inclusion criteria that the included studies could be assessed by quantitative analysis (Crabbe and Dishman, 2004). Our conclusions are somewhat in contrast with what Crabbe and Dishman reported, which is the review closest in aim to ours. They found widespread increase of activity in all frequency bands after exercise. Unexpectedly, we did not find this to be the case when we considered all available data. Differences in the selection process of included studies might have influenced this discordance in conclusions. Moreover, several studies have subsequently been published, which we were able to include, and which may have influenced our finding. We also included studies on patient populations, and it could be argued that these results may have biased our conclusions, e.g., that different brain pathologies interfere with the effects of exercise. However, the only study showing a decrease in delta activity in chronic interventions was done on patients with mild cognitive impairment (Amjad et al., 2019). This would contradict such a notion, although the generalizability of this finding is limited. As such, only few of the included studies were performed in patients, and it is unlikely that the results from these studies impaired the conclusions that could be drawn from our synthesis. Our rationale for including patient populations was the fact that exercise has been proposed as a possible therapeutic option in some diseases (Pedersen and Saltin, 2015), and as such it could interesting to see if an effect was evident in patient populations.
Within most frequency bands, findings were inconsistent for studies reporting on frequency analysis. A single study reported a decrease in delta activity in chronic interventions in an eyes closed condition in patients with mild cognitive impairment (Amjad et al., 2019), and it was not replicated by other groups. A null effect seemed present in acute interventions for gamma activity, and in chronic interventions for theta activity, while for the rest of frequency bands, both in acute and chronic interventions, the results were too inconsistent to draw any conclusions regarding the direction of change. The review by Crabbe and Dishman did not look at gamma activity and thus we are the first to synthesize results on this frequency band in exercise interventions. Gamma activity is related to working memory (Uhlhaas et al., 2011) and since exercise has been shown to improve memory (Smith et al., 2010) a connection between exercise and gamma activity might be probable. There were two studies investigating this band (Schneider et al., 2009a; Devilbiss et al., 2019). The study by Devilbiss et al. recorded EEG from a single frontally placed electrode, which means that changes in gamma activity will have been missed if this occurred in other brain areas than in frontal areas. The results by Devilbiss et al. were adjusted for multiple comparisons. The study by Schneider et al. was done in 24 participants. Thus, a true effect could have been missed due to low statistical power. It was also very rare that studies reporting on frequency analysis adjusted for multiple comparisons, which means that results in effect could be due to randomness, as adequate adjustment would include the number of frequency bands, number of groups and the number of time points tested against each other, in general. Further research is needed in the area of gamma activity and exercise as few groups looked into this aspect.
A considerable amount of studies reported using LORETA as an EEG analysis method. Generally, studies reporting on LORETA showed great heterogeneity with regards to which areas were affected by exercise, and while several studies found increases or decreases confined to individual Brodmann-defined areas, no widespread consistent intra-lobal increase/decrease was reproducibly shown. A single region, the dorsal anterior cingulate area, was shown to have increases in delta activity (Schneider et al., 2010b) and concurrent decrease in alpha activity (Ohmatsu et al., 2014) in acute intervention studies. The cingulate region is involved in executive control, emotion and spatial memory among other brain functions (Bubb et al., 2018), and delta oscillations are also implicated in memory tasks (Harmony, 2013), which in turn can be modulated by exercise (Smith et al., 2010) making a connection possible, but must at present remain speculative. A limiting factor in both studies is the number of electrodes used to localize this activity. A recent review, commissioned by International Federation of Clinical Neurophysiology (IFCN), states that a minimum of 48–64 (and upwards of 128–256) electrodes might be warranted to achieve this approximation with a high enough degree of certainty (Babiloni et al., 2019). Nonetheless, application of LORETA analysis methods represents an interesting area to elucidate anatomical areas affected by exercise. As shown by the few studies identified by this review, it should be explored further with more strict methodology to confirm the exact anatomical localization of these effects.
We chose to include studies on different patient groups in the present review, with the exclusion of sleep disorders and epilepsy, which are diseases where a different EEG/MEG approach might be warranted than the ones generally reported here. Generally, few studies were carried out in diseased individuals with our inclusion criteria and there was great heterogeneity in the diseases studied. A few studies deserve some special attention, since they focused on the same disease, namely patients with spinal cord injuries (Brümmer et al., 2011a; Sato et al., 2017), and thus offer some unique insights. The study by Brümmer et al. involved participants who were semi-professional hand-cycling athletes and were included as a validation group to confirm specific exercise preferences related to frontal activity, whereas Sato et al. studied a less selected cohort of patients with spinal cord injury as their primary group and wished to investigate this group of patients specifically. Both interventions applied to this group consisted of aerobic arm exercises, namely wheelchair propulsion (Sato et al., 2017) and arm crank exercise (Brümmer et al., 2011a). Both studies reported on the alpha freqeuency, where Brümmer et al. found decreased frontal alpha activity, and Sato et al. found a shift to a higher alpha peak frequency, which is also found in healthy individuals (Gutmann et al., 2015) in chronic interventions. This could indicate that the effect on the alpha peak shift is preserved in patients with spinal cord injury.
The present review also identified studies using methods other than freqeuncy analysis and LORETA to analyze the EEG. An area that seems promising is the study of brain connectivity, which was studied by one group, investigating whether a 24-week intervention consisting of Greek traditional dancing had an effect on this aspect of brain function in healthy individuals (Zilidou et al., 2018). The study used LORETA based estimations of current source density and then applied graph theory based understandings of brain function assigning nodes to specific clusters of EEG activity. This enabled the group to estimate the network properties of the brain and the changes that occur when a chronic complex exercise intervention was applied in senior citizens. Within this paradigm, the group found changes in network connectivity when applying a threshold of 10,000 and 12,500 nodes in the small world property which is a measurement of how the network is connected (see for example Watts and Strogatz, 1998 for an introduction to graph theory). The group showed similarly significant changes in several areas of brain connectivity. No consensus currently exist as to the node threshold that should be applied (Rossini et al., 2019) and thus the results need to replicated to confirm these findings with the presently applied thresholds.
Many studies reported on the effects of exercise on asymmetry, a quantitative measure of the incongruence between hemispheric oscillatory activity. Results were generally inconsistent regarding this aspect, both for acute and chronic interventions, with an overweight of studies rated with an overall high risk of bias and many studies reporting a null effect (Petruzzello and Tate, 1997; Schneider et al., 2009a; Woo et al., 2009; Deslandes et al., 2010; Lattari et al., 2016, 2018; Hicks et al., 2018). Several theories have been proposed as to the nature and meaning of differential hemispheric activation, the most prominent being that lateralization of brain activity is specifically connected with affective states (Davidson, 1992), which is also the hypothesis that many of these studies build upon in their investigations into exercise. In line with this assumption the participants' state of mind was often investigated with mood state questionnaires before and after exercise. If the idea of affective states being associated with specific mood states holds true, then it should follow that if exercise somehow modulated the mood states of participants an effect could then be measured using asymmetry as an indicator of this change. Our synthesis seems to point to a null effect of exercise on asymmetry in all frequency bands, and even though this is unexpected, since a growing body of literature points to a mood altering effect of exercise (Mikkelsen et al., 2017), it could be due to non-validity of the underlying hypothesis of the connection between asymmetry and mood states or simply that the exercise intervention applied did not result in such a change in mood, a focus area which we did not explore in the present review.
No studies reported on MEG in exercise interventions and it can only be speculated as to why we were unable to retrieve studies reporting on this aspect. Generally, MEG is expensive to undertake and as such may not always be readily available to researchers. It could be interesting to see if results found using EEG could be replicated and build upon using MEG, since MEG should also capture deeper electromagnetic sources, that are not reachable by EEG. This is an area, where future research is needed.
Limitations
We acknowledge the limitations of the present review. The employment of a wider search method with more databases included could potentially have revealed additional relevant records although our strategy was among some of the highest rated combinations in a recent review of optimal databases for literature searches (Bramer et al., 2017). We chose to include studies on populations with different diseases in our synthesis, which could have biased our results. Studies reporting on diseased participants were few and in general no discernable trends in the findings convincingly indicated a different response to those studies reporting on healthy individuals. We employed a qualitative synthesis of results. The reason for this choice of synthesis was based on the a priori assumption of large heterogeneity in studies. It could be argued that a quantitative synthesis should have been carried out, but the trade-off is the exclusion of studies not conforming to such an analysis, which we believe leaves out important data. We did not consider relative power contra total power nor did we analyze eyes-open vs. eyes-closed conditions, which might have affected the interpretation of results. Further, we excluded studies involving an event-related potential as an outcome and studies involving sleep EEG. While these are valid areas of research, where knowledge seems limited, it is beyond the scope of the present review. We also must refer to reviews commissioned by the IFCN for detailed descriptions of analytical methods as an in-depth guide for researchers is beyond the scope of this review (Babiloni et al., 2019; Rossini et al., 2019).
Conclusions
We conducted a comprehensive systematic review within a validated combination of science databases on studies using EEG as an outcome measure in exercise interventions. Our synthesis showed that the included studies were inadequately powered to assess the impact on EEG signal by exercise interventions. Further methodological issues leading to low quality of the identified studies, limits the conclusions which may be drawn. The most often reported methods of analysis were frequency analysis and LORETA. The results when assessed for all studies were inconclusive regarding any changes observed in a pre- and post-exercise comparison and the significance that could be attached to any statistically significant results was severely impaired due to a large proportion of studies being rated with an overall high risk of bias as well as due to missing adjustment for multiple comparisons. There are indications that short bouts of exercise are associated with changes in EEG microstates, although independent validation studies should be carried out in larger populations. No studies were retrieved by our searches were MEG was the outcome, which means research in this area is lacking. EEG remains an interesting methodology to examine underlying effects of exercise on brain functions. Future studies should investigate larger populations and adhere to stricter methodology as well as report and carry out statistically meaningful power estimations prior to conducting experimental research with EEG as an outcome measure.
Author Contributions
KF developed the idea for the review. MG crafted and conducted the bibliographic searches. MG and KF screened articles for inclusion and extracted data and assessed risk of bias. MG drafted the manuscript. KF, GW, and SH revised critically for important intellectual content and approved the manuscript.
Funding
The salary of MG was partially funded by the Research Fund at Rigshospitalet, Copenhagen, Denmark.
Conflict of Interest
The authors declare that the research was conducted in the absence of any commercial or financial relationships that could be construed as a potential conflict of interest.
Supplementary Material
The Supplementary Material for this article can be found online at: https://www.frontiersin.org/articles/10.3389/fnhum.2020.00155/full#supplementary-material
References
Amjad, I., Toor, H., Niazi, I. K., Afzal, H., Jochumsen, M., Shafique, M., et al. (2019). Therapeutic effects of aerobic exercise on EEG parameters and higher cognitive functions in mild cognitive impairment patients. Int. J. Neurosci. 129, 551–562. doi: 10.1080/00207454.2018.1551894
Babiloni, C., Barry, R. J., Başar, E., Blinowska, K. J., Cichocki, A., Drinkenburg, W. H. I. M., et al. (2019). International federation of clinical neurophysiology (IFCN) – EEG research workgroup: recommendations on frequency and topographic analysis of resting state EEG rhythms. Part 1: applications in clinical research studies. Clin. Neurophysiol. 131, 285–307. doi: 10.1016/j.clinph.2019.06.234
Bailey, S. P., Hall, E. E., Folger, S. E., and Miller, P. C. (2008). Changes in EEG during graded exercise on a recumbent cycle ergometer. J. Sport. Sci. Med. 7, 505–511.
Baillet, S. (2017). Magnetoencephalography for brain electrophysiology and imaging. Nat. Neurosci. 20, 327–339. doi: 10.1038/nn.4504
Barha, C. K., Galea, L. A., Nagamatsu, L. S., Erickson, K. I., and Liu-Ambrose, T. (2017). Personalising exercise recommendations for brain health: considerations and future directions. Br. J. Sports Med. 51, 636–639. doi: 10.1136/bjsports-2016-096710
Bixby, W. R., Spalding, T. W., and Hatfield, B. D. (2001). Temporal dynamics and dimensional specificity of the affective response to exercise of varying intensity: differing pathways to a common outcome. J. Sport Exerc. Psychol. 23, 171–190. doi: 10.1123/jsep.23.3.171
Boutcher, S. H., and Landers, D. M. (1988). The effects of vigorous exercise on anxiety, heart rate, and alpha activity of runners and nonrunners. Psychophysiology 25, 696–702. doi: 10.1111/j.1469-8986.1988.tb01911.x
Bramer, W. M., Rethlefsen, M. L., Kleijnen, J., and Franco, O. H. (2017). Optimal database combinations for literature searches in systematic reviews: a prospective exploratory study. Syst. Rev. 6:245. doi: 10.1186/s13643-017-0644-y
Brümmer, V., Schneider, S., Abel, T., Vogt, T., and Strüder, H. K. (2011a). Brain cortical activity is influenced by exercise mode and intensity. Med. Sci. Sports Exerc. 43, 1863–1872. doi: 10.1249/MSS.0b013e3182172a6f
Brümmer, V., Schneider, S., Strüder, H. K., and Askew, C. D. (2011b). Primary motor cortex activity is elevated with incremental exercise intensity. Neuroscience 181, 150–162. doi: 10.1016/j.neuroscience.2011.02.006
Bubb, E. J., Metzler-Baddeley, C., and Aggleton, J. P. (2018). The cingulum bundle: anatomy, function, and dysfunction. Neurosci. Biobehav. Rev. 92, 104–127. doi: 10.1016/j.neubiorev.2018.05.008
Carvalho, A., Barbirato, D., Araujo, N., Martins, J. V. S. A, Cavalcanti, J. L., Santos, T. M., et al. (2015). Comparison of strength training, aerobic training, and additional physical therapy as supplementary treatments for parkinson's disease: pilot study. Clin. Interv. Aging 10, 183–191. doi: 10.2147/CIA.S68779
Cassilhas, R. C., Tufik, S., and de Mello, M. T. (2016). Physical exercise, neuroplasticity, spatial learning and memory. Cell. Mol. Life Sci. 73, 975–983. doi: 10.1007/s00018-015-2102-0
Chapotot, F., Gronfier, C., Jouny, C., Muzet, A., and Brandenberger, G. (1998). Cortisol secretion is related to electroencephalographic alertness in human subjects during daytime wakefulness. J. Clin. Endocrinol. Metab. 83, 4263–4268. doi: 10.1210/jc.83.12.4263
Christensen, N. J., and Galbo, H. (1983). Sympathetic nervous activity during exercise. Annu. Rev. Physiol. 45, 139–153. doi: 10.1146/annurev.ph.45.030183.001035
Ciria, L. F., Perakakis, P., Luque-Casado, A., and Sanabria, D. (2018). Physical exercise increases overall brain oscillatory activity but does not influence inhibitory control in young adults. Neuroimage 181, 203–210. doi: 10.1016/j.neuroimage.2018.07.009
Cohen, D., and Cuffin, B. N. (1983). Demonstration of useful differences between magnetoencephalogram and electroencephalogram. Electroencephalogr. Clin. Neurophysiol. 56, 38–51. doi: 10.1016/0013-4694(83)90005-6
Crabbe, J. B., and Dishman, R. K. (2004). Brain electrocortical activity during and after exercise: a quantitative synthesis. Psychophysiology 41, 563–574. doi: 10.1111/j.1469-8986.2004.00176.x
Davidson, R. J. (1992). Anterior cerebral asymmetry and the nature of emotion. Brain Cogn. 20, 125–151. doi: 10.1016/0278-2626(92)90065-T
Deslandes, A. C., Moraes, H., Alves, H., Pompeu, F. A. M. S., Silveira, H., Mouta, R., et al. (2010). Effect of aerobic training on EEG alpha asymmetry and depressive symptoms in the elderly: a 1-year follow-up study. Braz. J. Med. Biol. Res. 43, 585–592. doi: 10.1590/S0100-879X2010007500041
Devilbiss, D. M., Etnoyer-Slaski, J. L., Dunn, E., Dussourd, C. R., Kothare, M. V., Martino, S. J., et al. (2019). Effects of exercise on EEG activity and standard tools used to assess concussion. J. Healthcare Eng. 2019, 1–14. doi: 10.1155/2019/4794637
Dishman, R. K., Thom, N. J., Puetz, T. W., O'Connor, P. J., and Clementz, B. A. (2010). Effects of cycling exercise on vigor, fatigue, and electroencephalographic activity among young adults who report persistent fatigue. Psychophysiology 47, 1066–1074. doi: 10.1111/j.1469-8986.2010.01014.x
Frederiksen, K. S., Gjerum, L., Waldemar, G., and Hasselbalch, S. G. (2018). Effects of physical exercise on alzheimer's disease biomarkers : a systematic review of intervention studies. J. Alzheimers Dis. 61, 359–372. doi: 10.3233/JAD-170567
Frederiksen, K. S., Gjerum, L., Waldemar, G., and Hasselbalch, S. G. (2019). Physical activity as a moderator of alzheimer pathology: a systematic review of observational studies. Curr. Alzheimer Res. 16, 1–17. doi: 10.2174/1567205016666190315095151
Fumoto, M., Oshima, T., Kamiya, K., Kikuchi, H., Seki, Y., Nakatani, Y., et al. (2010). Ventral prefrontal cortex and serotonergic system activation during pedaling exercise induces negative mood improvement and increased alpha band in EEG. Behav. Brain Res. 213, 1–9. doi: 10.1016/j.bbr.2010.04.017
Fuss, J., Steinle, J., Bindila, L., Auer, M. K., Kirchherr, H., Lutz, B., et al. (2015). A runner's high depends on cannabinoid receptors in mice. Proc. Natl. Acad. Sci. U.S.A. 112, 13105–13108. doi: 10.1073/pnas.1514996112
Gutmann, B., Hülsdünker, T., Mierau, J., Strüder, H. K., and Mierau, A. (2018a). Exercise-induced changes in EEG alpha power depend on frequency band definition mode. Neurosci. Lett. 662, 271–275. doi: 10.1016/j.neulet.2017.10.033
Gutmann, B., Mierau, A., Hülsdünker, T., Hildebrand, C., Przyklenk, A., Hollmann, W., et al. (2015). Effects of physical exercise on individual resting state EEG alpha peak frequency. Neural Plast. 2015:717312. doi: 10.1155/2015/717312
Gutmann, B., Zimmer, P., Hülsdünker, T., Lefebvre, J., Binnebößel, S., Oberste, M., et al. (2018b). The effects of exercise intensity and post-exercise recovery time on cortical activation as revealed by EEG alpha peak frequency. Neurosci. Lett. 668, 159–163. doi: 10.1016/j.neulet.2018.01.007
Gwin, J. T., Gramann, K., Makeig, S., and Ferris, D. P. (2010). Removal of movement artifact from high-density EEG recorded during walking and running. J. Neurophysiol. 103, 3526–3534. doi: 10.1152/jn.00105.2010
Harmony, T. (2013). The functional significance of delta oscillations in cognitive processing. Front. Integr. Neurosci. 7:83. doi: 10.3389/fnint.2013.00083
Herold, F., Wiegel, P., Scholkmann, F., and Müller, N. (2018). Applications of functional near-infrared spectroscopy (fNIRS) neuroimaging in exercise–cognition science: a systematic, methodology-focused review. J. Clin. Med. 7:466. doi: 10.3390/jcm7120466
Hicks, R. A., Hall, P. A., Staines, W. R., and McIlroy, W. E. (2018). Frontal alpha asymmetry and aerobic exercise: are changes due to cardiovascular demand or bilateral rhythmic movement? Biol. Psychol. 132, 9–16. doi: 10.1016/j.biopsycho.2017.10.011
Hicks, S. D., Jacob, P., Perez, O., Baffuto, M., Gagnon, Z., and Middleton, F. A. (2019). The transcriptional signature of a runner's high. Med. Sci. Sports Exerc. 51, 970–978. doi: 10.1249/MSS.0000000000001865
Hill, E. E., Zack, E., Battaglini, C., Viru, M., Viru, A., and Hackney, A. C. (2008). Exercise and circulating cortisol levels: the intensity threshold effect. J. Endocrinol. Invest. 31, 587–591. doi: 10.1007/BF03345606
Hilty, L., Langer, N., Pascual-Marqui, R., Boutellier, U., and Lutz, K. (2011). Fatigue-induced increase in intracortical communication between mid/anterior insular and motor cortex during cycling exercise. Eur. J. Neurosci. 34, 2035–2042. doi: 10.1111/j.1460-9568.2011.07909.x
Hottenrott, K., Taubert, M., and Gronwald, T. (2013). Cortical brain activity is influenced by cadence in cyclists. Open Sports Sci. J. 6, 9–14. doi: 10.2174/1875399X01306010009
Hübner, L., Godde, B., and Voelcker-Rehage, C. (2018). Acute exercise as an intervention to trigger motor performance and EEG beta activity in older adults. Neural Plast. 2018:4756785. doi: 10.1155/2018/4756785
Kamp, A., and Troost, J. (1978). EEG signs of cerebrovascular disorder, using physical exercise as a provocative method. Electroencephalogr. Clin. Neurophysiol. 45, 295–298. doi: 10.1016/0013-4694(78)90015-9
Kubitz, K. A., and Landers, D. M. (1993). The effects of aerobic training on cardiovascular responses to mental stress: an examination of underlying mechanisms. J. Sport Exerc. Psychol. 15, 326–337. doi: 10.1123/jsep.15.3.326
Kubitz, K. A., and Mott, A. A. (1996). EEG power spectral densities during and after cycle ergometer exercise. Res. Q. Exerc. Sport 67, 91–96. doi: 10.1080/02701367.1996.10607929
Kubitz, K. A., and Pothakos, K. (1997). Does aerobic exercise decrease brain activation? J. Sport Exerc. Psychol. 19, 291–301. doi: 10.1123/jsep.19.3.291
Labarrera, C., Deitcher, Y., Dudai, A., Weiner, B., Kaduri Amichai, A., Zylbermann, N., et al. (2018). Adrenergic modulation regulates the dendritic excitability of layer 5 pyramidal neurons in vivo. Cell Rep. 23, 1034–1044. doi: 10.1016/j.celrep.2018.03.103
Lattari, E., Budde, H., Paes, F., Neto, G. A. M., Appolinario, J. C., Nardi, A. E., et al. (2018). Effects of aerobic exercise on anxiety symptoms and cortical activity in patients with panic disorder: a pilot study. Clin. Pract. Epidemiol. Ment. Health 14, 11–25. doi: 10.2174/1745017901814010011
Lattari, E., Portugal, E., Junior, R. S. M., Oliveira, B. R. R., Santos, T. M., Mura, G., et al. (2016). Acute affective responses and frontal electroencephalographic asymmetry to prescribed and self-selected exercise. Clin. Pract. Epidemiol. Ment. Health 12, 108–119. doi: 10.2174/1745017901612010108
Lattari, E., Portugal, E., Moraes, H., Machado, S., Santos, T., and Deslandes, A. (2014). Acute effects of exercise on mood and EEG activity in healthy young subjects: a systematic review. CNS Neurol. Disord. Drug Targets 13, 972–980. doi: 10.2174/1871527313666140612125418
Lehmann, D., Pascual-Marqui, R., and Michel, C. (2009). EEG microstates. Scholarpedia 4:7632. doi: 10.4249/scholarpedia.7632
Ludyga, S., Hottenrott, K., and Gronwald, T. (2017). Four weeks of high cadence training alter brain cortical activity in cyclists. J. Sports Sci. 35, 1377–1382. doi: 10.1080/02640414.2016.1198045
Mechau, D., Mücke, S., Weiß, M., and Liesen, H. (1998). Effect of increasing running velocity on electroencephalogram in a field test. Eur. J. Appl. Physiol. Occup. Physiol. 78, 340–345. doi: 10.1007/s004210050429
Mellow, M. L., Goldsworthy, M. R., Coussens, S., and Smith, A. E. (2019). Acute aerobic exercise and neuroplasticity of the motor cortex: a systematic review. J. Sci. Med. Sports 23, 408–414. doi: 10.1016/j.jsams.2019.10.015
Mierau, A., Schneider, S., Abel, T., Askew, C., Werner, S., and Strüder, H. K. (2009). Improved sensorimotor adaptation after exhaustive exercise is accompanied by altered brain activity. Physiol. Behav. 96, 115–121. doi: 10.1016/j.physbeh.2008.09.002
Mikkelsen, K., Stojanovska, L., Polenakovic, M., Bosevski, M., and Apostolopoulos, V. (2017). Exercise and mental health. Maturitas 106, 48–56. doi: 10.1016/j.maturitas.2017.09.003
Moher, D., Liberati, A., Tetzlaff, J., Altman, D. G., and Group, T. P. (2009). Preferred reporting items for systematic reviews and meta-analyses: the PRISMA statement. PLoS Med. 6:e1000097. doi: 10.1371/journal.pmed.1000097
Moraes, H., Deslandes, A., Silveira, H., Ribeiro, P., Cagy, M., Piedade, R., et al. (2011). The effect of acute effort on EEG in healthy young and elderly subjects. Eur. J. Appl. Physiol. 111, 67–75. doi: 10.1007/s00421-010-1627-z
Moraes, H., Ferreira, C., Deslandes, A., Cagy, M., Pompeu, F., Ribeiro, P., et al. (2007). Beta and alpha electroencephalographic activity changes after acute exercise. Arq. Neuropsiquiatr. 65, 637–641. doi: 10.1590/S0004-282X2007000400018
Mott, A. A., Dyer, R. A., and Kubitz, K. A. (1995). Effects of exercise on the coherence of human EEG- signals at selected hemispheric sites. Biomed. Sci. Instrum. 31, 1–6.
Ohmatsu, S., Nakano, H., Tominaga, T., Terakawa, Y., Murata, T., and Morioka, S. (2014). Activation of the serotonergic system by pedaling exercise changes anterior cingulate cortex activity and improves negative emotion. Behav. Brain Res. 270, 112–117. doi: 10.1016/j.bbr.2014.04.017
Pascual-Marqui, R. D., Michel, C. M., and Lehmann, D. (1994). Low resolution electromagnetic tomography: a new method for localizing electrical activity in the brain. Int. J. Psychophysiol. 18, 49–65. doi: 10.1016/0167-8760(84)90014-X
Pedersen, B. K., and Saltin, B. (2015). Exercise as medicine - evidence for prescribing exercise as therapy in 26 different chronic diseases. Scand. J. Med. Sci. Sports 25(Suppl. 3), 1–72. doi: 10.1111/sms.12581
Petruzzello, S. J., and Landers, D. M. (1994). State anxiety reduction and exercise: does hemispheric activation reflect such changes? Med. Sci. Sports Exerc. 26, 1028–1035. doi: 10.1249/00005768-199408000-00015
Petruzzello, S. J., and Tate, A. K. (1997). Brain activation, affect, and aerobic exercise: an examination of both state-independent and state-dependent relationships. Psychophysiology 34, 527–533. doi: 10.1111/j.1469-8986.1997.tb01739.x
Pineda, A., and Adkisson, M. A. (1961). Electroencephalographic studies in physical fatigue. Tex. Rep. Biol. Med. 19, 332–42.
Raichlen, D. A., and Alexander, G. E. (2017). Adaptive capacity: an evolutionary neuroscience model linking exercise, cognition, and brain health. Trends Neurosci. 40, 408–421. doi: 10.1016/j.tins.2017.05.001
Rossini, P. M., Di Iorio, R., Bentivoglio, M., Bertini, G., Ferreri, F., Gerloff, C., et al. (2019). Methods for analysis of brain connectivity: an IFCN-sponsored review. Clin. Neurophysiol. 130, 1833–1858. doi: 10.1016/j.clinph.2019.06.006
Sato, G., Osumi, M., and Morioka, S. (2017). Effects of wheelchair propulsion on neuropathic pain and resting electroencephalography after spinal cord injury. J. Rehabil. Med. 49, 136–143. doi: 10.2340/16501977-2185
Schneider, S., Askew, C. D., Abel, T., Mierau, A., and Strüder, H. K. (2010a). Brain and exercise: a first approach using electrotomography. Med. Sci. Sports Exerc. 42, 600–607. doi: 10.1249/MSS.0b013e3181b76ac8
Schneider, S., Askew, C. D., Abel, T., and Strüder, H. K. (2010b). Exercise, music, and the brain: is there a central pattern generator? J. Sports Sci. 28, 1337–1343. doi: 10.1080/02640414.2010.507252
Schneider, S., Askew, C. D., Diehl, J., Mierau, A., Kleinert, J., Abel, T., et al. (2009a). EEG activity and mood in health orientated runners after different exercise intensities. Physiol. Behav. 96, 709–716. doi: 10.1016/j.physbeh.2009.01.007
Schneider, S., Brümmer, V., Abel, T., Askew, C. D., and Strüder, H. K. (2009b). Changes in brain cortical activity measured by EEG are related to individual exercise preferences. Physiol. Behav. 98, 447–452. doi: 10.1016/j.physbeh.2009.07.010
Severtsen, B., and Bruya, M. A. (1986). Effects of meditation and aerobic exercise on EEG patterns. J. Neurosci. Nurs. 18, 206–210. doi: 10.1097/01376517-198608000-00010
Silveira, H., Deslandes, A. C., de Moraes, H., Mouta, R., Ribeiro, P., Piedade, R., et al. (2010). Effects of exercise on electroencephalographic mean frequency in depressed elderly subjects. Neuropsychobiology 61, 141–147. doi: 10.1159/000279304
Skosnik, P. D., Hajos, M., Cortes-Briones, J. A., Edwards, C. R., Pittman, B. P., Hoffmann, W. E., et al. (2018). Cannabinoid receptor-mediated disruption of sensory gating and neural oscillations: a translational study in rats and humans. Neuropharmacology 135, 412–423. doi: 10.1016/j.neuropharm.2018.03.036
Smith, P. J., Blumenthal, J. A., Hoffman, B. M., Cooper, H., Strauman, T. A., Welsh-Bohmer, K., et al. (2010). Aerobic exercise and neurocognitive performance: a meta-analytic review of randomized controlled trials. Psychosom. Med. 72, 239–252. doi: 10.1097/PSY.0b013e3181d14633
Sparling, P. B., Giuffrida, A., Piomelli, D., Rosskopf, L., and Dietrich, A. (2003). Exercise activates the endocannabinoid system. Neuroreport 14, 2209–2211. doi: 10.1097/00001756-200312020-00015
Spring, J. N., Bourdillon, N., and Barral, J. (2018). Resting EEG microstates and autonomic heart rate variability do not return to baseline one hour after a submaximal exercise. Front. Neurosci. 12:460. doi: 10.3389/fnins.2018.00460
Spring, J. N., Tomescu, M. I., and Barral, J. (2017). A single-bout of endurance exercise modulates EEG microstates temporal features. Brain Topogr. 30, 461–472. doi: 10.1007/s10548-017-0570-2
Sterne, J. A. C., Savović, J., Page, M. J., Elbers, R. G., Blencowe, N. S., Boutron, I., et al. (2019). RoB 2: a revised tool for assessing risk of bias in randomised trials. BMJ 366:l4898. doi: 10.1136/bmj.l4898
Styliadis, C., Kartsidis, P., Paraskevopoulos, E., Ioannides, A. A., and Bamidis, P. D. (2015). Neuroplastic effects of combined computerized physical and cognitive training in elderly individuals at risk for dementia: an eLORETA controlled study on resting states. Neural Plast. 2015:172192. doi: 10.1155/2015/172192
Teixeira Guimaraes, T., Macedo da Costa, B., Silva Cerqueira, L., de Carlo Andrade Serdeiro, A., Augusto Monteiro Saboia Pompeu, F., Sales de Moraes, H., et al. (2014). Acute effect of different patterns of exercise on mood, anxiety and cortical activity. Arch. Neurosci. 2:e18781. doi: 10.5812/archneurosci.18781
Uhlhaas, P. J., Pipa, G., Neuenschwander, S., Wibral, M., and Singer, W. (2011). A new look at gamma? high- (>60 Hz) γ-band activity in cortical networks: function, mechanisms and impairment. Prog. Biophys. Mol. Biol. 105, 14–28. doi: 10.1016/j.pbiomolbio.2010.10.004
Villafaina, S., Collado-Mateo, D., Fuentes, J. P., Rohlfs-Domínguez, P., and Gusi, N. (2019). Effects of exergames on brain dynamics in women with fibromyalgia: a randomized controlled trial. J. Clin. Med. 8:1015. doi: 10.3390/jcm8071015
Vogt, T., Schneider, S., Abeln, V., Anneken, V., and Strueder, H. K. (2012). Exercise, mood and cognitive performance in intellectual disability-a neurophysiological approach. Behav. Brain Res. 226, 473–480. doi: 10.1016/j.bbr.2011.10.015
Vogt, T., Schneider, S., Brümmer, V., and Strüder, H. K. (2010). Frontal EEG asymmetry: the effects of sustained walking in the elderly. Neurosci. Lett. 485, 134–137. doi: 10.1016/j.neulet.2010.09.001
Wang, M., Ramos, B. P., Paspalas, C. D., Shu, Y., Simen, A., Duque, A., et al. (2007). Alpha2A-adrenoceptors strengthen working memory networks by inhibiting cAMP-HCN channel signaling in prefrontal cortex. Cell 129, 397–410. doi: 10.1016/j.cell.2007.03.015
Watts, D. J., and Strogatz, S. H. (1998). Collective dynamics of “small-world” networks. Nature 393, 440–442. doi: 10.1038/30918
Wegner, M., Helmich, I., Machado, S., Nardi, A. E., Arias-Carrion, O., and Budde, H. (2014). Effects of exercise on anxiety and depression disorders: review of meta- analyses and neurobiological mechanisms. CNS Neurol. Disord. Drug Targets 13, 1002–1014. doi: 10.2174/1871527313666140612102841
Wollseiffen, P., Ghadiri, A., Scholz, A., Strüder, H. K., Herpers, R., Peters, T., et al. (2016a). Short bouts of intensive exercise during the workday have a positive effect on neuro-cognitive performance. Stress Heal. 32, 514–523. doi: 10.1002/smi.2654
Wollseiffen, P., Schneider, S., Martin, L. A., Kerherve, H. A., Klein, T., and Solomon, C. (2016b). The effect of 6 h of running on brain activity, mood, and cognitive performance. Exp. Brain Res. 234, 1829–1836. doi: 10.1007/s00221-016-4587-7
Woo, M., Kim, S., Kim, J., Petruzzello, S. J., and Hatfield, B. D. (2009). Examining the exercise-affect dose-response relationship: does duration influence frontal EEG asymmetry? Int. J. Psychophysiol. 72, 166–172. doi: 10.1016/j.ijpsycho.2008.12.003
Woo, M., Kim, S., Kim, J., Petruzzello, S. J., and Hatfield, B. D. (2010). The influence of exercise intensity on frontal electroencephalographic asymmetry and self-reported affect. Res. Q. Exerc. Sport 81, 349–359. doi: 10.1080/02701367.2010.10599683
Keywords: electroencephalography (EEG), exercise, intervention, power, LORETA (low resolution electromagnetic tomography), brain connectivity, asymmetry
Citation: Gramkow MH, Hasselbalch SG, Waldemar G and Frederiksen KS (2020) Resting State EEG in Exercise Intervention Studies: A Systematic Review of Effects and Methods. Front. Hum. Neurosci. 14:155. doi: 10.3389/fnhum.2020.00155
Received: 03 February 2020; Accepted: 08 April 2020;
Published: 07 May 2020.
Edited by:
Raffaella Franciotti, Università degli Studi G. d'Annunzio Chieti e Pescara, ItalyReviewed by:
Angel Nevado, Complutense University of Madrid, SpainCem Seref Bediz, Dokuz Eylul Üniversity, Turkey
Copyright © 2020 Gramkow, Hasselbalch, Waldemar and Frederiksen. This is an open-access article distributed under the terms of the Creative Commons Attribution License (CC BY). The use, distribution or reproduction in other forums is permitted, provided the original author(s) and the copyright owner(s) are credited and that the original publication in this journal is cited, in accordance with accepted academic practice. No use, distribution or reproduction is permitted which does not comply with these terms.
*Correspondence: Mathias Holsey Gramkow, mathias.holsey.gramkow@regionh.dk