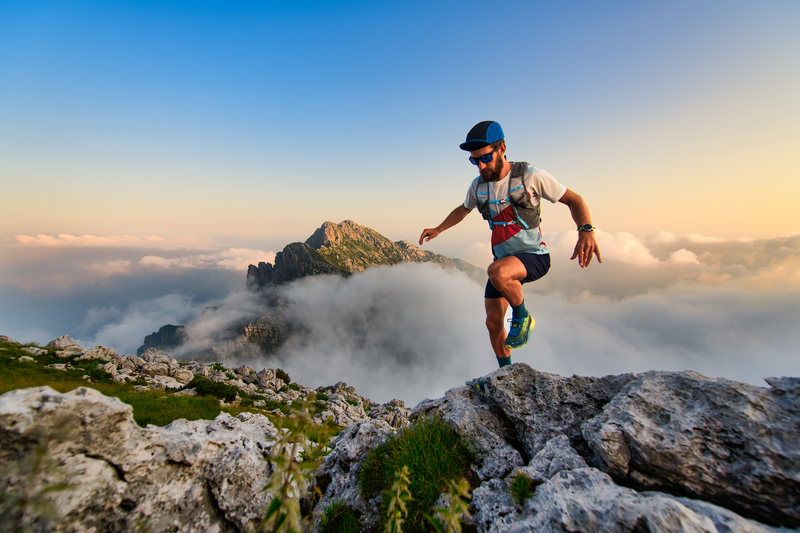
94% of researchers rate our articles as excellent or good
Learn more about the work of our research integrity team to safeguard the quality of each article we publish.
Find out more
EDITORIAL article
Front. Hum. Neurosci. , 18 September 2019
Sec. Cognitive Neuroscience
Volume 13 - 2019 | https://doi.org/10.3389/fnhum.2019.00326
This article is part of the Research Topic Physiological Computing of Social Cognition View all 7 articles
Editorial on the Research Topic
Physiological Computing of Social Cognition
Social Cognition focuses on how people process, store, and apply information about other people and social situations. It focuses on the role that cognitive processes play in our social interactions (Ostrom, 1984; Fiske and Taylor, 1991; Kunda, 1999). There has been increasing interest in the links between social cognition and brain function (Brothers, 1990). While substantial research has indicated that abilities such as theory of mind, social perception, attributional style, and emotion perception are clearly related to social outcomes (Pinkham et al., 2016), research has been slowed by problems in the measurement of these abilities.
Social interactions have been studied from physiological measurements for about 90 years (Riddle, 1925). The physiological basis of social constructs, processes and behavior has been widely investigated, firstly through social psychophysiology (Boyd and DiMascio, 1954; Kaplan and Bloom, 1960; Leiderman and Shapiro, 1964; Cacioppo and Petty, 1983), and later through social neuroscience (Brothers and Ring, 1992; Adolphs, 2001; Cacioppo and Berntson, 2002). Nowadays, Physiological Computing has emerged as a category of technology where electrophysiological data recorded directly from the human activity are used to interface with a computing device (Fairclough, 2009). This technology becomes even more relevant when computing can be integrated pervasively into everyday life environments.
“Physiological Computing of Social Cognition” should be understood as the application of physiological computing to the evaluation and/or treatment of social cognition abilities (Chanel and Mühl, 2015). It comprises a set of theoretical interdisciplinary frameworks, methodologies, methods, and hardware/software tools to interpret/act on how the human physiology mediates social interactions.
This Research Topic has provided a means for researchers and academicians who have a current or developing interest in the area of evaluation of social cognitive deficits and/or training in social cognition (e.g., cognitive enhancement therapies, attributional style training, social cognition and interaction training, or training of affect recognition, among others). Papers with a special focus on multidisciplinary approaches and multimodality were especially welcome. We finally accepted a total of six articles for this Research Topic.
Of the six papers, three are directly related to the neural computation of brain activity. The first paper “Neural Correlates of Racial Ingroup Bias in Observing Computer-Animated Social Encounters” by Katsumi and Dolcos contributes on the neural correlates of intergroup processes and non-verbal perception by analyzing fMRI recordings. According to the authors, in spite of evidence for the role of group membership in the neural correlates of social cognition, the mechanisms associated with processing non-verbal behaviors shown by racially ingroup versus outgroup individuals remain unclear. Caucasian members experienced fMRI recording while watching social encounters with ingroup and outgroup characters showing dynamic and static non-verbal behaviors. The discoveries after computing the fMRI neuroimages shed light on the mechanisms of racial ingroup bias in observing social encounters and have suggestions for understanding elements identified with successful interactions with people from diverse backgrounds.
A second paper by Park et al. denominated “EEG Beta Oscillations in the Temporoparietal Area Related to the Accuracy in Estimating Others' Preference” explores brain activity through EEG signals identified with the forecast of the inclination of other persons through thin-slicing. This implies that humans often endeavor to judge what others prefer dependent on a narrow slice of experience. In each trial of the task, participants were demonstrated an image of either a target person or self, trailed by the introduction of a film poster over which participants anticipated the target person's preference as liking or disliking. The outcomes of EEG signal processing suggest that right temporoparietal beta oscillations might be correlated with one's capacity to predict what others prefer with minimal information.
Another neuroimaging-based article titled “Transcranial Direct Current Stimulation Altered Voluntary Cooperative Norms Compliance Under Equal Decision-Making Power” by Li et al. exhibits that anodal transcranial direct current stimulation (tDCS) enhances the voluntary cooperative norms compliance compared with the sham treatment, whereas cathodal tDCS breaks it down. The outcomes checked that voluntary cooperative norms compliance of all participants was altered by activating right dorsolateral prefrontal cortex (rDLPFC). The discoveries approve that enhancing the excitability of the rDLPFC utilizing tDCS prompts high consistence in voluntary cooperation and this impact is explicit to equal as opposed to unequal decision-making power.
In another article titled “The Two-Systems Account of Theory of Mind: Testing the Links to Social- Perceptual and Cognitive Abilities” by Meinhardt-Injac et al. theory of mind (ToM) is calculated through testing four domains of abilities: holistic face perception, face recognition, relational reasoning and language. This paper represents an achievement in the investigation of the respective roles of face recognition and language in the two-systems account of ToM. To test the particular roles of explicit abilities in both processes, the researchers administered 15 experimental procedures to a large number of participants, testing capacity in face recognition and holistic perception, language, and reasoning. ToM was estimated by a set of tasks expecting capacity to track and to induce others' complex emotional and mental states. The outcomes feature the respective roles of face recognition and language, in this manner contributing closer empirical specification of the two-systems framework.
The next paper “Eye Movements During Everyday Behavior Predict Personality Traits” by Hoppe et al. shows that a person's level of neuroticism, extraversion, agreeableness, conscientiousness, and perceptual curiosity can be anticipated only from eye movements recorded during an everyday task. The authors tracked eye movements and assessed personality traits using questionnaires. Utilizing artificial intelligence and a rich set of features encoding distinctive eye movement features, the researchers were able to reliably foresee four of the Big Five personality traits as well as perceptual interest.
The last paper “Emotion Regulation in the Prisoner's Dilemma: Effects of Reappraisal on Behavioral Measures and Cardiovascular Measures of Challenge and Threat” by Chu et al. associates cooperation and cardiovascular responses in people that were defeated by their rival in the first round of an iterated Prisoner's dilemma. The following cardiovascular were computed: heart rate, ventricular contractility, cardiac output, and total peripheral resistance. Participants were either primed with the emotion-regulation strategy of reappraisal or no-emotion-regulation, and their opponent either expressed an amused smile or a polite smile after the results were presented. The authors found that cooperation behavior diminished in the no-emotion-regulation group when the rival expressed an amused smile compared to a polite smile. In the cardiovascular measures, they found significant contrasts between the emotion regulation conditions utilizing the biopsychosocial model of challenge and threat.
The six papers together have earned more than 84,000 views as of August 10th, 2019. This confirms the popularity of physiological computing of social cognition as a subject for readers. We, the guest-editors of this Research Topic, trust the growing importance of physiological computing in areas as different as computer science, engineering, biology, psychology, medicine and neuroscience. Moreover, we believe that physiological computing has not exploited all its abilities and has not yet reached its full potential. In addition, the design of physiological computing applications in the field of social cognition is only at a starting moment (Fernández-Sotos et al., 2019; García et al., 2019). We foresee a vast growth of solutions using the social cognition/physiological computing binomial in the near future.
All the authors contributed equally to the writing of the editorial.
This work has been partially supported by Spanish Ministerio de Ciencia, Innovación y Universidades, Agencia Estatal de Investigación (AEI)/European Regional Development Fund (FEDER, UE) under DPI2016-80894-R grant, and by CIBERSAM of the Instituto de Salud Carlos III.
The authors declare that the research was conducted in the absence of any commercial or financial relationships that could be construed as a potential conflict of interest.
Adolphs, R. (2001). The neurobiology of social cognition. Curr. Opin. Neurobiol. 11, 231–239. doi: 10.1016/S0959-4388(00)00202-6
Boyd, R., and DiMascio, A. (1954). Social behavior and autonomic physiology; a sociophysiologic study. J. Nervous Mental Dis. 120, 207–212.
Brothers, L., and Ring, B. (1992). A neuroethological framework for the representation of minds. J. Cogn. Neurosci. 4, 107–118.
Cacioppo, J. T., and Berntson, G. G. (2002). Foundations in Social Neuroscience. Cambridge, MA: The MIT Press.
Cacioppo, J. T., and Petty, R. E. (1983). Social Psychophysiology: A Sourcebook. New York, NY: Guildford Press.
Chanel, G., and Mühl, C. (2015). Connecting brains and bodies: applying physiological computing to support social interaction. Interact. Comput. 27, 534–550. doi: 10.1093/iwc/iwv013
Fairclough, S. H. (2009). Fundamentals of physiological computing. Interact. Comput. 21, 133–145. doi: 10.1016/j.intcom.2008.10.011
Fernández-Sotos, P., Torio, I., Fernández-Caballero, A., Navarro, E., Gonález, P., Dompablo, M., et al. (2019). Social cognition remediation interventions: a systematic mapping review. PLoS ONE 14:e218720. doi: 10.1371/journal.pone.0218720.
García, A. S., Fernández-Sotos, P., Fernández-Caballero, A., Navarro, E., Latorre, J. M., Rodriguez-Jimenez, R., et al. (2019). Acceptance and use of a multi-modal avatar-based tool for remediation of social cognition deficits. J. Amb. Intel. Human. Comput. 1–12. doi: 10.1007/s12652-019-01418-8
Kaplan, H. B., and Bloom, S. W. (1960). The use of sociological and social-psychological concepts in physiological research: a review of selected experimental studies. J. Nervous Mental Dis. 131, 128–134.
Leiderman, P. H., and Shapiro, D. (1964). Psychobiological Approaches to Social Behavior. Stanford, CA: Stanford University Press.
Ostrom, T. M. (1984). “The sovereignty of social cognition,” in Handbook of Social Cognition, eds R. S. Wyer and T. K. Srull (Hillsdale, NJ: Psychology Press), 1–37.
Keywords: socio-cognitive computing, affective computing, social interaction, virtual and augmented reality, social robots, physiological computing
Citation: Fernández-Caballero A, Miguel Latorre J, Martínez-Rodrigo A, Rodriguez-Jimenez R and Hussain A (2019) Editorial: Physiological Computing of Social Cognition. Front. Hum. Neurosci. 13:326. doi: 10.3389/fnhum.2019.00326
Received: 03 April 2019; Accepted: 05 September 2019;
Published: 18 September 2019.
Edited and reviewed by: Srikantan S. Nagarajan, University of California, San Francisco, United States
Copyright © 2019 Fernández-Caballero, Miguel Latorre, Martínez-Rodrigo, Rodriguez-Jimenez and Hussain. This is an open-access article distributed under the terms of the Creative Commons Attribution License (CC BY). The use, distribution or reproduction in other forums is permitted, provided the original author(s) and the copyright owner(s) are credited and that the original publication in this journal is cited, in accordance with accepted academic practice. No use, distribution or reproduction is permitted which does not comply with these terms.
*Correspondence: Antonio Fernández-Caballero, YW50b25pby5mZGV6QHVjbG0uZXM=
Disclaimer: All claims expressed in this article are solely those of the authors and do not necessarily represent those of their affiliated organizations, or those of the publisher, the editors and the reviewers. Any product that may be evaluated in this article or claim that may be made by its manufacturer is not guaranteed or endorsed by the publisher.
Research integrity at Frontiers
Learn more about the work of our research integrity team to safeguard the quality of each article we publish.