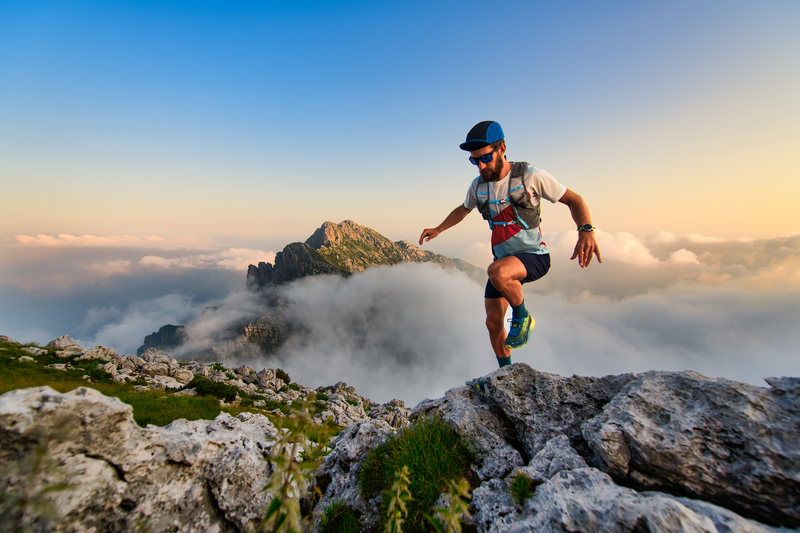
95% of researchers rate our articles as excellent or good
Learn more about the work of our research integrity team to safeguard the quality of each article we publish.
Find out more
ORIGINAL RESEARCH article
Front. Hum. Neurosci. , 31 January 2018
Sec. Motor Neuroscience
Volume 12 - 2018 | https://doi.org/10.3389/fnhum.2018.00005
This article is part of the Research Topic Motor control of gait and the underlying neural network in pediatric neurology View all 14 articles
Cerebral palsy (CP) and Duchenne muscular dystrophy (DMD) are neuromuscular disorders characterized by muscle weakness. Weakness in CP has neural and non-neural components, whereas in DMD, weakness can be considered as a predominantly non-neural problem. Despite the different underlying causes, weakness is a constraint for the central nervous system when controlling gait. CP demonstrates decreased complexity of motor control during gait from muscle synergy analysis, which is reflected by a higher total variance accounted for by one synergy (tVAF1). However, it remains unclear if weakness directly contributes to higher tVAF1 in CP, or whether altered tVAF1 reflects mainly neural impairments. If muscle weakness directly contributes to higher tVAF1, then tVAF1 should also be increased in DMD. To examine the etiology of increased tVAF1, muscle activity data of gluteus medius, rectus femoris, medial hamstrings, medial gastrocnemius, and tibialis anterior were measured at self-selected walking speed, and strength data from knee extensors, knee flexors, dorsiflexors and plantar flexors, were analyzed in 15 children with CP [median (IQR) age: 8.9 (2.2)], 15 boys with DMD [8.7 (3.1)], and 15 typical developing (TD) children [8.6 (2.7)]. We computed tVAF1 from 10 concatenated steps with non-negative matrix factorization, and compared tVAF1 between the three groups with a Mann-Whiney U-test. Spearman's rank correlation coefficients were used to determine if weakness in specific muscle groups contributed to altered tVAF1. No significant differences in tVAF1 were found between DMD [tVAF1: 0.60 (0.07)] and TD children [0.65 (0.07)], while tVAF1 was significantly higher in CP [(0.74 (0.09)] than in the other groups (both p < 0.005). In CP, weakness in the plantar flexors was related to higher tVAF1 (r = −0.72). In DMD, knee extensor weakness related to increased tVAF1 (r = −0.50). These results suggest that the non-neural weakness in DMD had limited influence on complexity of motor control during gait and that the higher tVAF1 in children with CP is mainly related to neural impairments caused by the brain lesion.
Two of the most common neurological and neuromuscular diseases in childhood are cerebral palsy (CP) and Duchenne muscular dystrophy (DMD) (Sussman, 2002; Rosenbaum et al., 2007; Graham et al., 2016). CP is defined as “a group of permanent disorders of the development of movement and posture, causing activity limitations attributed to non-progressive disturbances that occurred in the developing fetal or infant brain” (Rosenbaum et al., 2007). DMD is characterized by an altered gene on the X-chromosome, which codes for the protein dystrophin. Lack of dystrophin in muscles leads to a disbalance between damage and repair of the muscle fibers (Kobayashi and Campbell, 2012). This damage results in muscles that predominantly consist of fat and fibrous tissue (Sussman, 2002; Jones et al., 2010). Although CP and DMD have different origins and expressions, they have at least one symptom in common: muscle weakness. In both groups, muscle weakness is considered an important contributor to their pathological gait patterns (Sutherland et al., 1981; D'Angelo et al., 2009; Gage et al., 2009; Gaudreault et al., 2010; Doglio et al., 2011; Ganea et al., 2012; Steele et al., 2012).
There are only a small number of studies describing DMD gait (Sutherland et al., 1981; D'Angelo et al., 2009; Gaudreault et al., 2010; Doglio et al., 2011; Ganea et al., 2012; Ropars et al., 2016). Furthermore, none of these studies have verified to what extent these gait deviations were associated with muscle weakness (Sutherland et al., 1981; D'Angelo et al., 2009; Gaudreault et al., 2010; Doglio et al., 2011; Ganea et al., 2012; Ropars et al., 2016). In CP, several researchers have analyzed the relationship between muscle weakness and gait impairments, but their results are inconsistent (Damiano et al., 1995, 2010; Wiley and Damiano, 1998; Desloovere et al., 2006; Lee et al., 2008; Dallmeijer et al., 2011; Eek et al., 2011; Sagawa et al., 2013; Meyns et al., 2016; Shin et al., 2016). In DMD, muscle weakness is caused by non-neural changes in muscle morphology, whereas in CP, muscle weakness has neural as well as non-neural components. Neural components are considered the primary cause of weakness and are the result of the original brain injury (Gage et al., 2009). Examples include altered motor unit recruitment patterns and decreased selective motor control (Gage et al., 2009; Mockford and Caulton, 2010). Non-neural components are considered secondary causes of muscle weakness in CP (Gage et al., 2009), including changes in muscle morphology or lever-arm dysfunction due to bony deformities (Gage et al., 2009; Barrett and Lichtwark, 2010). The effect of these neural and non-neural changes on gait can be very different than their effect on strength assessments, such as maximal voluntary isometric contractions (MVICs). This could be an important reason for the discrepancies in previous studies analyzing the relationship between muscle weakness and altered gait in CP.
Despite the lack of consensus on how muscle weakness contributes to impaired gait, there is no doubt that it is a constraint the central nervous system (CNS) needs to deal with when initiating and controlling gait. Since children with CP and boys with DMD have different etiologies of weakness, the evaluation of how the CNS copes with muscle weakness in both populations has the potential to increase our understanding of the relationship between muscle weakness and gait deviations. In particular, it will help to differentiate between the relative effects of both neural and non-neural components of weakness on gait.
The regulation of human gait is not entirely understood, largely due to the abundant degrees of freedom (DOFs) and complexity of the human body (Latash, 2012). One of the theories for how humans control this abundance, is the use of muscle synergies instead of individual control of each muscle. Muscle synergies have been defined as the “consistent patterns of multi-muscle coordination that generate specific action” (Ting et al., 2015). Synergistic patterns of muscle recruitment have been well documented during various rhythmic tasks, including walking (d'Avella et al., 2003; Dietz, 2003; Nielsen, 2003; Barroso et al., 2013). Central pattern generators in the spinal cord and supra-spinal structures are thought to contribute to the regulation of these synergistic muscle activations (Lacquaniti et al., 1999; Dietz, 2002, 2003; Nielsen, 2003; Petersen et al., 2012). Synergies are flexible, thereby allowing to compensate for internal and external disturbances without affecting the outcome of the intended movement (Latash et al., 2002; Ting et al., 2015). This suggests that neural as well as non-neural components can affect synergies (Kutch and Valero-Cuevas, 2012; Bizzi and Cheung, 2013; Clark, 2015). In synergy analysis, evaluating the “total variance accounted for” (tVAF) by a given number of synergies can quantify the complexity of an individual's muscle activation patterns during dynamic tasks. The tVAF by one synergy (tVAF1) can provide a summary measure of synergy complexity. When tVAF1 is high, one synergy can explain a large part of the variance in muscle activity, representing a decrease in complexity of motor control during the analyzed task (Steele et al., 2015; Ting et al., 2015). Individuals with a CNS motor lesion, such as in CP or stroke, have higher tVAF1 during gait than age-related healthy controls (Clark et al., 2010; Clark, 2015; Steele et al., 2015; Tang et al., 2015; Ting et al., 2015). Further, this decrease in complexity of motor control in children with CP was found to be related to muscle weakness (Steele et al., 2015). However, in these prior studies, muscle weakness was measured via a global summary score from manual muscle testing (MMT) (Steele et al., 2015). Not only does MMT have low reliability in young children with developmental disabilities (Mahony et al., 2009), but these analyses also limit our understanding of whether weakness of specific muscles affects control of gait.
If muscle weakness is a constraint for the CNS, it could limit the available degrees of freedom (DOFs) and negatively influence complexity of motor control, not only in CP, but also in boys with DMD. In children with CP, there is one confounding factor: the influence of the brain lesion on synergies and muscle weakness. Alterations in the CNS, such as the brain lesion in CP, affect a substantial part of synergy regulation (Lacquaniti et al., 1999; Dietz, 2002, 2003; Nielsen, 2003; Petersen et al., 2012). This brain lesion also underlies muscle weakness (its neural component) (Gage et al., 2009; Mockford and Caulton, 2010). The relationship between muscle weakness and tVAF1 found in the previous study (Steele et al., 2015) could be caused by their mutual underlying source: the alterations in the CNS. This poses the research question: is muscle weakness contributing to higher tVAF1 during gait in children with CP or is the higher tVAF1 a quantification of the underlying brain lesion?
The primary goal of this research was to compare and contrast the impact of muscle weakness on tVAF1 extracted from synergy analysis during gait for children with CP and DMD. We evaluated tVAF1 during gait at self-selected walking speed for three groups of children: children with CP, children with DMD, and TD children. We expected decreases in complexity of motor control (increase in tVAF1) in both CP and DMD children when compared to a control group of TD children. However, in children with CP, due to the addition of a neural component of muscle weakness, a higher tVAF1 was expected than in DMD. As a secondary goal, we also sought to analyze the effect of muscle weakness of four muscle groups (knee extensors, knee flexors, plantar flexors, and dorsiflexors) on complexity of motor control. Muscle weakness was assessed via MVICs with a standardized protocol, using a hand-held dynamometer (HHD) in a fixed position (Goudriaan et al., 2018). We hypothesized that of the four measured muscle groups, the plantar flexors would be largely responsible for higher tVAF1 in both CP and DMD, because of their importance during gait (van der Krogt et al., 2012). For an overview of the complete study design we refer to Table 1.
In preparation of this study, we performed a power analysis based on a pilot study (Goudriaan et al., 2016) to determine the sample size of the three groups (CP, DMD, and TD; Table 1). The pilot study indicated that for an effect size of d = 1.23, α = 0.05, and power (1-β) = 0.80 a minimal sample size of 12 participants per group would be required to test our main hypothesis (GPower 3.1.9, Faul et al., 2007).
We recruited 15 children with CP [median age (interquartile range): 8.9 (2.2)], 15 boys with DMD [8.7 (3.1)], and 15 typical developing (TD) children [8.6 (2.7)] (Table 2). Supplementary Tables 1–3 provide detailed subject characteristics. We asked the children with CP to participate at the time of their routine clinical gait analysis at the Clinical Motion Analysis Laboratory of the University Hospital of Pellenberg (CMAL-Pellenberg) or when they agreed to take part in a large European study, namely the MD-Paedigree project: A Model-Driven Pediatric European Digital Repository, partially funded by the European Commission under P7-ICT-2011-9 program (600932). Inclusion criteria were: (1) diagnosed with bilateral or unilateral CP without signs of dyskinesia, (2) Gross Motor Function Classification System (GMFCS) Levels I-II, (3) no Botulinum Toxin-A treatment within 6 months prior to the assessments, and (4) no history of lower limb surgery.
The children with DMD were recruited from the database of the neuromuscular reference center in the University Hospital of Gasthuisberg. If they agreed to participate in MD-Paedigree, we asked them to perform the additional strength measurements needed for the current study. For the DMD children the inclusion criteria were: (1) diagnosed with DMD and (2) no history of lower-limb surgery.
Colleagues and students working at the Clinical Motion Analysis Laboratory of the University Hospital of Pellenberg (CMAL-Pellenberg) assisted with the recruitment of the TD children. The inclusion criteria for the TD children was that they should not have any neurological or neuromuscular problems.
All children were evaluated at the CMAL-Pellenberg. The local ethics committee (Commissie Medische Ethiek KU Leuven) approved this study (S56041), under the Declaration of Helsinki. All the participants' parents or caretakers signed a written informed consent. All participants of 12 years of age or older also signed the informed consent.
We collected gait kinematics, kinetics, and muscle activity data at self-selected walking speed with 3D motion analysis. We used the marker set of the lower limb Plug-in-Gait (PiG) model and marker trajectories were tracked using a 10 to 15-camera VICON system (Nexus 1.8.4. Vicon-UK, Oxford, UK), sampled at 100 Hz. Muscle activity data were collected with surface electromyography (sEMG) bilaterally from the rectus femoris (REF), vastus lateralis (VAL), medial hamstrings (MEH), biceps femoris (BIF), medial gastrocnemius (GAS), soleus (SOL), tibialis anterior (TIA) and the gluteus medius (GLU), with a 16-channel telemetric sEMG system (Zerowire, Cometa, Italy) at 1,000 or 1,500 Hz. Based on the guidelines of Seniam, we attached circular Ag/AgCl electrodes with an area of 1 cm2 and an interelectrode distance of 2 cm on the skin (Hermens et al., 1999).
All participants performed MVICs of the knee extensors (KE), knee flexors (KF), dorsiflexors (DF) and plantar flexors (PF) evaluated using a telemetric hand-held dynamometer (HHD) MicroFet® 2 (Hogan Health Industries, West Jordan, UT USA). To decrease compensatory mechanisms and influence of the assessor on MVIC-outcomes, we used a custom-made chair in which the participants were secured with straps around the pelvis and upper legs, and the HHD was fixed to the chair. We placed the HHD at 75% of the segment length (Figure 1) and applied a gravity correction for those MVICs where gravity influenced the output data (KF MVIC and PF MVIC), by subtracting the gravitational torque in rest position from the MVIC-outcomes (Boiteau et al., 1995; Goudriaan et al., 2018). The children first performed one test trial, followed by three actual MVICs, with a duration between 3 and 5 s. Between each trial, the children rested at least 10 s, and between each muscle group, they had a resting period of at least 2 min. During the measurements, the children had visual feedback and were verbally encouraged.
Figure 1. Custom made chair used for the MVIC measurements (A,B), including an example of the normalized net joint torque (Nm/kg) curves collected during the MVIC measurements (C,D). (A) Test position for KE MVIC. The black + red lines represent the segment length (fibula head—lower border of lateral malleolus). The black line indicates the moment arm (75% of the segment length). (B) Test position for the DF MVIC. The red line represents the segment length (projection of lateral malleolus on lateral border of the foot—distal metacarpal head V). The black line indicates the moment arm (75% of the segment length). (C) Normalized knee extension torque (Nm/kg) of a representative KE MVIC of one child with TD (gray), a boy with DMD (blue) and a CP child (black) of similar age. (D) Normalized dorsiflexion torque in (Nm/kg) during a representative DF MVIC of the same children as used in (C). Please note the scaling of the axes in (C,D) is not the same, due to difference between the knee extensors and the dorsiflexors in torque output. CP, cerebral palsy; DMD, Duchenne muscular dystrophy; DF, dorsiflexion; KE, knee extension; MVIC, maximal voluntary isometric contraction; Nm/kg, Newton meter per kilogram bodyweight; TD, typical developing; s, seconds.
In the children with CP and the boys with DMD, we first collected the gait analysis data and a standard clinical exam (range of motion, spasticity levels by Modified Ashworth and Tardieu scales, and strength by MMT) and then measured the MVICs by means of dynamometry. Based on the individual child's cooperation during and after the gait analysis and whether the child was fatigued, we decided to collect either bilateral or unilateral MVICs. In case of unilateral MVICs, we always chose the most involved side, based on the outcomes of the standard clinical exam. For the children with CP or DMD, we only included their most involved side in the analyses. In the TD children, MVICs were always collected bilaterally after the gait analysis. Based on the outcomes of the MVICs, we used the weakest leg for further analyses. All available clinical outcome measures are reported in Supplementary Tables 1–3.
We analyzed the sEMG data from five (REF, MEH, TIA, GAS, and GLU) of the eight muscles that were measured during gait. We excluded the VAL, the BIF, and the SOL from all analyses, because their activation patterns (and function) during gait are roughly the same as the REF, MEH, and GAS respectively (Winter, 1987). Also, these five muscles are the most common muscles to be evaluated during standard clinical gait and synergy analyses. For all participants, we selected 10 representative steps. The sEMG signals were filtered with a 6th order Butterworth bandpass filter with cut-off frequencies of 20 and 450 Hz. The signals were rectified and smoothed with a 4th order Butterworth lowpass filter with a frequency of 10 Hz (Shuman et al., 2017). We resampled the filtered sEMG signals of each step at 101 data points, representing 0–100% of a gait cycle. We then concatenated all resampled gait cycles and normalized the signals to the average amplitude of the 10 steps per muscle for each child.
We calculated synergies using non-negative matrix factorization (NNMF) (Lee and Seung, 1999; Ting and Macpherson, 2005; Oliveira et al., 2014; Shuman et al., 2016a) with the NNMF function in MATLAB (The Mathworks Inc., Natick, M.A., 2010) using the following settings: 50 replicates, 1,000 max iterations, 1*10−4 minimum threshold for convergence, and a 1*10−6 threshold for completion (Shuman et al., 2016a). NNMF decomposes the sEMG signals into two matrices: W containing the synergy weights, which are the weighted contributions of each included muscle to each synergy, and C, the synergy activations, such that:
In Equation (1), n is the number of synergies (one in this study), m is the number of muscles (five in this study), t is the number of data points (10*101 = 1,010 in this study), and error is the difference between the measured sEMG data and the reconstructed sEMG signals from the calculated synergies. The error value was then used to calculate tVAF as:
From an early age, contractile tissue of the muscles in children with DMD is replaced with fibrofatty tissue (Jansen et al., 2012). Fibrofatty tissue in the muscles might function as an additional lowpass filter (Farina et al., 2002), reducing tVAF1 (van der Krogt et al., 2016; Shuman et al., 2017). We therefore calculated the power spectral density (PSD) of the bandpass filtered (20–450 Hz) sEMG signals with the PWELCH function in MATLAB using the following inputs: a window size of 1,024 samples, an overlap of 512 samples, 500 points to use in the Fourier transform, and the sample frequency of the sEMG signals (1,000 or 1,500 Hz). From the PSDs, we calculated the median frequency curves to compare group differences.
Walking speed (in m/s) was extracted from the gait data for each child and converted to a non-dimensional value with the formula of Hof (1996) to determine whether differences in walking speed could explain potential differences in tVAF1 between the three groups (Ivanenko, 2005; Shuman et al., 2016a). Force data (in Newtons) from the MVICs was resampled to 100 Hz and the average maximal force out of three trials was calculated (Willemse et al., 2013; Goudriaan et al., 2018). Subsequently, the net joint torque normalized to bodyweight (Nm/kg) was determined for all MVICs for each participant (Supplementary Tables 1–3).
Since the data were not normally distributed, we used non-parametric tests in SPSS (SPSS Inc., Chicago, IL). We used a Kruskal-Wallis H-test and a post-hoc Mann-Whitney U-test with Bonferroni correction (resulting in the critical p = 0.005) to determine if there were significant differences in tVAF1, non-dimensional walking speed, and MVICs between the three groups (CP, DMD, and TD). The PSD-plots were visually inspected for each muscle. We analyzed the relationship between tVAF1 and muscle weakness in each individual muscle group with Spearman's rank correlation coefficients. We used the Altman classification (<0.20 = poor; 0.21–0.40 = fair; 0.41–0.60 = moderate; 0.61–0.80 = good; 0.81–1.00 = very good) to interpret the correlation coefficients (Altman, 1991).
In the three study groups (CP, DMD, and TD), all five muscles showed good quality sEMG data for all 10 concatenated steps and could be included in the NNMF analysis, with the exception of the GLU for one of the TD children. The outcomes of the Kruskal-Wallis H-Test showed significant group differences for all assessed parameters (all p < 0.005). The results of the post-hoc Mann Whitney U-test on all parameters are plotted in Figure 2.
Figure 2. Group differences (TD (gray), DMD (blue) and CP (black)) between tVAF1, walking speed, and MVIC-outcomes. Significant differences (p ≤ 0.005) based on the Mann-Whitney U-test are indicated with *. Please note the scaling on MVIC axes are not the same, due to differences in maximal net joint torque between muscle groups. CP, cerebral palsy; DMD, Duchenne muscular dystrophy; DF, dorsiflexion; KE, knee extension; KF, knee flexion; Nm/kg, Newton meter per kilogram bodyweight; MVIC, maximal voluntary isometric contraction; PF, plantar flexion; tVAF1, total variance accounted for by one synergy; TD, typical developing.
The tVAF1 was significantly higher in the children with CP compared to DMD and TD children (both p < 0.005). No significant differences in tVAF1 were found between the boys with DMD and the TD children. Median values for tVAF1 were 0.74 in CP, 0.60 in DMD, and 0.65 in the TD children. The interquartile ranges (IQRs) were similar for the three groups, 0.09 for the children with CP, and 0.07 for both the boys with DMD and the TD children.
The children with CP and DMD walked slower than the TD children, but this was only significant for the children with CP (p < 0.005). Median values and IQRs for non-dimensional walking speed were: 0.40 (0.07) for the CP children, 0.42 (0.05) in the boys with DMD, and 0.48 (0.10) in the TD children.
The TD children were significantly stronger in all four muscle groups than the children in the other two groups (CP and DMD, all p < 0.005). The TD children showed more inter-subject variability in MVICs compared to CP and DMD, which was indicated by the larger IQRs. Median values and IQRs in Nm/kg of the knee extensors were: 0.62 (0.26) in CP, 0.72 (0.37) in DMD, and 1.29 (0.83) in TD. For the knee flexors, these values were: 0.43 (0.37) in CP, 0.50 (0.21) in DMD, and 1.04 (0.41) in TD. The dorsiflexors had the lowest MVIC values in all groups, with median values (IQRs) of 0.07 (0.07) in CP, 0.10 (0.04) in DMD, 0.27 (0.08) in TD. For the plantar flexors, median MVIC values of 0.22 (0.19), 0.33 (0.22), and 0.86 (0.45) were found for the CP, DMD, and TD groups, respectively.
Only two moderate-to-high correlations (r ≤ 0.41) were found between muscle weakness and tVAF1. Increased weakness in the plantar flexors was associated with higher tVAF1 in the CP children (r = −0.72). In the boys with DMD, weaker knee extensors were associated with higher tVAF1 (r = −0.50) (Figure 3).
Figure 3. (A) Spearman's rank correlation coefficients between MVIC outcomes and tVAF1. Moderate or higher correlations (r ≤ 0.41) are indicated in bold. There were significant associations between (B). PF MVIC and tVAF1 in children with CP and (C). KE MVIC and tVAF1 in children with DMD. Please note the scaling of the axes in (B,C) are not the same, due to differences in maximal net joint torque. CP, cerebral palsy; DMD, Duchenne muscular dystrophy; DF, dorsiflexion; KE, knee extension; KF, knee flexion; MVIC, maximal voluntary isometric contraction; PF, plantar flexion; TD, typical developing; tVAF1, total variance accounted for by one synergy.
When examining the PSD-plots of the three groups, they showed similar frequency bands, but in DMD the power was lower in the proximal muscle groups (Figure 4).
Figure 4. Power spectrum density plots of filtered sEMG signals (20–450 Hz). Median curves are plotted for each group: TD (gray), DMD (blue) and CP (black). Please note the scaling of the axes is not the same, due to differences between muscles. CP, cerebral palsy; DMD, Duchenne muscular dystrophy; Hz, Herz; TD, typical developing; μV, microvolts.
This study evaluated synergy complexity and strength in two common neuromuscular disorders to explore the impact of the neural and non-neural factors of muscle weakness on neuromuscular control during walking. Due to the differing etiology of these populations, this analysis helps to evaluate which factors serve as constraints to the CNS and influence impaired movement. We hypothesized that muscle weakness contributes to altered synergies and complexity of control during gait in children with CP and DMD, expressed by increased tVAF1 during gait compared to TD children. Contrary to this hypothesis, our results suggested that the complexity of control was not influenced by the non-neural constraints of muscle weakness, since tVAF1 was not significantly different between the children with DMD and the TD children. However, we confirmed that children with CP had reduced synergy complexity and that muscle weakness in the plantar flexors was related to higher tVAF1 during gait. In children with DMD, increased weakness in the knee extensors influenced tVAF1, although not enough to result in significantly different tVAF1 between DMD and TD.
Similar to previously reported results, tVAF1 was higher in the children with CP than in the group of TD children (Steele et al., 2015; Tang et al., 2015; Shuman et al., 2016a). The children with CP walked significantly slower than the TD children, which suggests that the differences in tVAF1 between CP and the TD children might be more pronounced than expressed in our results, since a faster walking speed can result in higher tVAF1 (Ivanenko, 2005; Shuman et al., 2016a). It could be that tVAF1 gives an indirect representation of the child's neural capacity, with a higher tVAF1 reflecting a higher level of involvement and increased muscle weakness. This agrees with the findings of Rose and McGill (2005) who determined that muscle weakness in children with CP is largely caused by neural factors. Further, the region on the motor cortex responsible for the distal muscle groups of the lower limb, is closer to the phylogenetic older parts of the brain (Volpe, 2000; Stiles and Jernigan, 2010) and it has been suggested that the older regions of the motor cortex are involved in synergy regulation (Bizzi and Cheung, 2013). Combined with the importance of the plantar flexors during gait, this might explain why we only found a strong correlation between weakness of the plantar flexors and tVAF1 during gait and not between tVAF1 and the other muscle groups in CP.
We checked if fibrofatty tissue in the muscles of the boys with DMD could act as an additional lowpass filter (Farina et al., 2002; Jansen et al., 2012) thereby reducing tVAF1 (van der Krogt et al., 2016; Shuman et al., 2017). In DMD, the proximal muscle groups are more involved than the distal muscle groups, which was also represented in the PSD-plots. While the three groups showed similar frequency bands for all five muscles, the power was lower in the REF, MEH, and GLU muscles in the children with DMD. In children with DMD, fiber type IIb is the first fiber type to degenerate, which will have an influence on the frequency distribution, since these are the fast fibers connected to the motor units with the higher firing frequencies (Stackhouse et al., 2005; Jones et al., 2010).
In the children with DMD, the knee extensors are one of the most involved muscle groups (Sussman, 2002), which could explain the moderate negative correlation between the outcomes of the KE MVIC and tVAF1. But, this non-neural weakness of the knee extensors did not sufficiently limit the complexity of control to create a difference in tVAF1 between the children with DMD and the TD children. In other words, non-neural weakness appears to be only a small constraint for the CNS with respect to complexity of motor control.
Our results suggest that complexity of motor control, represented by tVAF1, might be considered the neural capacity of a child, which could be difficult to alter with current treatments. Although tVAF1 measured before treatment has been shown to be associated with changes in gait after treatment (Schwartz et al., 2016), prior research has also demonstrated that there are minimal changes in tVAF1 after botulin toxin injections, selective dorsal rhizotomy, and single event multilevel surgeries among children with CP (Oudenhoven et al., 2016; Shuman et al., 2016b).
There are several important limitations in this research. First, we only correlated weakness with tVAF1, whereas in children with CP and DMD, other clinical symptoms could have contributed to an increase in tVAF1. Steele et al. (2015) determined that a higher level of spasticity and decreased selective motor control were also related to higher tVAF1 in children with CP, although to a lesser extent than muscle weakness. Similar to muscle weakness, if a higher tVAF1 indicates a higher level of involvement, this would not only be associated with more muscle weakness, but also with spasticity and decreased selective motor control (Ostensjø et al., 2004). In this study we focused on weakness, since DMD provides a comparison group to probe the relative impacts of non-neural factors that contribute to weakness on the results of synergy analysis. However, in children with DMD, other non-neurological symptoms besides muscle weakness are also present, such as decreased passive range motion due to contractures (Sussman, 2002). This only strengthens our conclusion that tVAF1 represents the decreased DOFs in the CNS due to the brain lesion and that non-neural constraints have negligible influence on the complexity of motor control.
Further, due to the decreased selective motor control, an increase in level of co-contraction during strength assessments has been reported in children with CP (Mockford and Caulton, 2010). This increase in co-contraction has been suggested to be an important reason for the decrease in maximal torque output during a MVIC (Elder et al., 2003; Stackhouse et al., 2005). However, in a previous pilot study, while using the same protocol to measure MVICs, we determined that the levels of co-contraction were comparable between children with CP and TD children (Goudriaan et al., 2015). Similar results have been reported by Damiano et al. (2000), who determined that, although children with CP had higher levels of co-contraction during knee extension and knee flexion MVICs, this did not influence the outcomes of the MVICs.
Although the plantar flexors are important in maintaining a normal gait pattern, other muscle groups such as the hip abductors also play an important role (van der Krogt et al., 2012). Unfortunately, our MVIC setup did not allow for standardized strength measurements of the hip muscles, thus the influence of weakness in these muscle groups on tVAF1 during gait should be analyzed in the future. Finally, tVAF1 outcomes in this study were only representative of the five muscles that were included in the analysis. If other muscles were to be analyzed or more muscles included, the value of tVAF1 could differ since synergy analyses can be dependent on the number and choice of muscles (Steele et al., 2013). However, it is expected that the relative differences in tVAF1 between the three groups (CP, DMD and TD) would be similar.
The lack of significant differences in tVAF1 between boys with DMD and TD children suggests that non-neural muscle weakness has little influence on complexity of motor control during gait. Although, weakness in the plantar flexors was negatively correlated with tVAF1 in the children with CP, this is most likely the result of the common underlying cause: alterations in the CNS. Our results imply that despite the predictive value of tVAF1 on treatment outcomes, a child's baseline tVAF1 (i.e., the child's neural capacity) could be difficult to influence with pre-surgery therapy or may require novel intervention strategies that more directly target neural capacity.
All authors contributed to the work either to the design, data collection, analysis, interpretation, writing, or editing. KD and MG designed the experiment. Patient recruitment was performed by MVdH, NG, and GM. Data collection was done by MG. BS and KS created the original software for synergy calculation, modifications were made by MG. MG and MVdH performed quality checks on the data. Data analysis was done by MG, BS, and KS. Statistical tests were run by MG. Interpretation of the results was done by KD, KS, BS, and MG. MG and KD wrote the paper, which was edited by KS, BS, MVdH, NG, and GM. The entire process supervised by KD.
MG was funded by the MD-Paedigree project: A Model-Driven Pediatric European Digital Repository, partially funded by the European Commission under P7-ICT-2011-9 program (600932). KS was funded by the National Institute of Neurological Disorders and Stroke (NINDS) of the National institutes of Health under award number R01NS091056 and BS was funded by the Washington Research Foundation Funds for Innovation in Neuroengineering.
The authors declare that the research was conducted in the absence of any commercial or financial relationships that could be construed as a potential conflict of interest.
We would like to thank all the colleagues of the CMAL-Pellenberg for their help during collection of the 3D gait data of the children with CP and the boys with DMD.
The Supplementary Material for this article can be found online at: https://www.frontiersin.org/articles/10.3389/fnhum.2018.00005/full#supplementary-material
Barrett, R. S., and Lichtwark, G. A. (2010). Gross muscle morphology and structure in spastic cerebral palsy: a systematic review. Dev. Med. Child Neurol. 52, 794–804. doi: 10.1111/j.1469-8749.2010.03686.x
Barroso, F., Torricelli, D., Moreno, J. C., Taylor, J., Gómez-Soriano, J., Esteban, E. B., et al. (2013). Similarity of muscle synergies in human walking and cycling: preliminary results. Conf. Proc. IEEE Eng. Med. Biol. Soc. 2013, 6933–6966. doi: 10.1109/EMBC.2013.6611152
Bizzi, E., and Cheung, V. C. (2013). The neural origin of muscle synergies. Front. Comput. Neurosci. 7:51. doi: 10.3389/fncom.2013.00051
Boiteau, M., Malouin, F., and Richards, C. L. (1995). Use of a hand-held dynamometer and a Kin-Com® dynamometer for evaluating spastic hypertonia in children: a reliability study. Phys. Ther. 75, 796–802. doi: 10.1093/ptj/75.9.796
Clark, D. J. (2015). Automaticity of walking: functional significance, mechanisms, measurement and rehabilitation strategies. Front. Hum. Neurosci. 9:246. doi: 10.3389/fnhum.2015.00246
Clark, D. J., Ting, L. H., Zajac, F. E., Neptune, R. R., and Kautz, S. A. (2010). Merging of healthy motor modules predicts reduced locomotor performance and muscle coordination complexity post-stroke. J. Neurophysiol. 103, 844–857. doi: 10.1152/jn.00825.2009
D'Angelo, M. G., Berti, M., Piccinini, L., Romei, M., Guglieri, M., Bonato, S., et al. (2009). Gait pattern in Duchenne muscular dystrophy. Gait Posture 29, 36–41. doi: 10.1016/j.gaitpost.2008.06.002
d'Avella, A., Saltiel, P., and Bizzi, E. (2003). Combinations of muscle synergies in the construction of a natural motor behavior. Nat. Neurosci. 6, 300–308. doi: 10.1038/nn1010
Dallmeijer, A. J., Baker, R., Dodd, K. J., and Taylor, N. F. (2011). Association between isometric muscle strength and gait joint kinetics in adolescents and young adults with cerebral palsy. Gait Posture 33, 326–332. doi: 10.1016/j.gaitpost.2010.10.092
Damiano, D. L., Arnold, A. S., Steele, K. M., and Delp, S. L. (2010). Can strength training predictably improve gait kinematics? A pilot study on the effects of hip and knee extensor strengthening on lower-extremity alignment in cerebral palsy. Phys. Ther. 90, 269–279. doi: 10.2522/ptj.20090062
Damiano, D. L., Kelly, L. E., and Vaughn, C. L. (1995). Effects of quadriceps femoris muscle strengthening on crouch gait in children with spastic diplegia. Phys. Ther. 75, 658–667. discussion: 668–671. doi: 10.1093/ptj/75.8.658
Damiano, D. L., Martellotta, T. L., Sullivan, D. J., Granata, K. P., and Abel, M. F. (2000). Muscle force production and functional performance in spastic cerebral palsy: relationship of cocontraction. Arch. Phys. Med. Rehabil. 81, 895–900. doi: 10.1053/apmr.2000.5579
Desloovere, K., Molenaers, G., Feys, H., Huenaerts, C., Callewaert, B., and Van de Walle, P. (2006). Do dynamic and static clinical measurements correlate with gait analysis parameters in children with cerebral palsy? Gait Posture 24, 302–313. doi: 10.1016/j.gaitpost.2005.10.008
Dietz, V. (2002). Proprioception and locomotor disorders. Nat. Rev. Neurosci. 3, 781–790. doi: 10.1038/nrn939
Dietz, V. (2003). Spinal cord pattern generators for locomotion. Clin. Neurophysiol. 114, 1379–1389. doi: 10.1016/S1388-2457(03)00120-2
Doglio, L., Pavan, E., Pernigotti, I., Petralia, P., Frigo, C., and Minetti, C. (2011). Early signs of gait deviation in Duchenne muscular dystrophy. Eur. J. Phys. Rehabil. Med. 47, 587–594. doi: 10.1016/j.gaitpost.2008.10.044
Eek, M. N., Tranberg, R., and Beckung, E. (2011). Muscle strength and kinetic gait pattern in children with bilateral spastic CP. Gait Posture 33, 333–337. doi: 10.1016/j.gaitpost.2010.10.093
Elder, G. C., Kirk, J., Stewart, G., Cook, K., Weir, D., Marshall, A., et al. (2003). Contributing factors to muscle weakness in children with cerebral palsy. Dev. Med. Child Neurol. 45, 542–550. doi: 10.1111/j.1469-8749.2003.tb00954.x
Farina, D., Cescon, C., and Merletti, R. (2002). Influence of anatomical, physical, and detection-system parameters on surface EMG. Biol. Cybern. 86, 445–456. doi: 10.1007/s00422-002-0309-2
Faul, F., Erdfelder, E., Lang, A.-G., and Buchner, A. (2007). GPOWER: a general power analysis program. Behav. Res. Methods 39, 175–191. doi: 10.3758/BF03193146
Gage, J. R., Schwartz, M. H., Koop, S. E., and Novacheck, T. F. (2009). The Identification and Treatment of Gait Problems in Cerebral Palsy, 2nd Edn., London, UK: John Wiley & Sons.
Ganea, R., Jeannet, P. Y., Paraschiv-Ionescu, A., Goemans, N. M., Piot, C., Van den Hauwe, M., et al. (2012). Gait assessment in children with duchenne muscular dystrophy during long-distance walking. J. Child Neurol. 27, 30–38. doi: 10.1177/0883073811413581
Gaudreault, N., Gravel, D., Nadeau, S., Houde, S., and Gagnon, D. (2010). Gait patterns comparison of children with Duchenne muscular dystrophy to those of control subjects considering the effect of gait velocity. Gait Posture 32, 342–347. doi: 10.1016/j.gaitpost.2010.06.003
Goudriaan, M., Nieuwenhuys, A., Schless, S., Goemans, N., Molenaers, G., and Desloovere, K. (2018). A new strength assessment to evaluate the association between muscle weakness and gait pathology in children with cerebral palsy. PLoS One 828, 1–22. doi: 10.1371/journal.pone.0191097
Goudriaan, M., Smets, K., Biermans, T., and Desloovere, K. (2015). Differences in co-contraction level between cp and td children during a functional and isometric strength assessment. Gait Posture 42, S12–S13. doi: 10.1016/j.gaitpost.2015.06.029
Goudriaan, M., Van den Hauwe, M., Shuman, B., Steele, K. M., Molenaers, G., Goemans, N., et al. (2016). Differences in synergy complexity during gait between children with Cerebral Palsy and Duchenne Muscular Dystrophy. Gait Posture. 49S:107. doi: 10.1016/j.gaitpost.2016.07.163
Graham, H. K., Rosenbaum, P., Paneth, N., Dan, B., and Lin, J. (2016). Cerebral palsy. Nat Rev. 2:15082. doi: 10.1038/nrdp.2015.82
Hermens, H. J., Freriks, B., Merletti, R., Stegeman, D., Blok, J., Rau, G., et al. (1999). European Recommendations for Surface ElectroMyoGraphy. Enschede: Roessingh Research and Development.
Hof, A. L. (1996). Scaling gait data to body size. Gait Posture 4, 222–223. doi: 10.1016/0966-6362(95)01057-2
Ivanenko, Y. P. (2005). Spinal cord maps of spatiotemporal alpha-motoneuron activation in humans walking at different speeds. J. Neurophysiol. 95, 602–618. doi: 10.1152/jn.00767.2005
Jansen, M., van Alfen, N., Nijhuis van der Sanden, M. W., van Dijk, J. P., Pillen, S., and de Groot, I. J. M. (2012). Quantitative muscle ultrasound is a promising longitudinal follow-up tool in Duchenne muscular dystrophy. Neuromuscul. Disord. 22, 306–317. doi: 10.1016/j.nmd.2011.10.020
Jones, D., Round, J., and de Haan, A. (2010). Skeletal Muscles, From Molecules to Movement. A Textbook of Muscle Physiology for Sport Exercise, Physiotherapy and Medicine. London: Elsevier.
Kobayashi, Y. M., and Campbell, K. P. (2012). “Skeletal muscle dystrophin- glycoprotein complex and muscular dystrophy,” in Muscle Fundamental Biology and Mechanisms of Disease, eds J. A. Hill and E. N. Olson (San Diego, CA: Elsevier), 935–942.
Kutch, J. J., and Valero-Cuevas, F. J. (2012). Challenges and new approaches to proving the existence of muscle synergies of neural origin. PLoS Comput. Biol. 8:e1002434. doi: 10.1371/journal.pcbi.1002434
Lacquaniti, F., Grasso, R., and Zago, M. (1999). Motor patterns in walking. News Physiol. Sci. 14, 168–174. doi: 10.1152/physiologyonline.1999.14.4.168
Latash, M. L., Scholz, J. P., and Schöner, G. (2002). Motor control strategies revealed in the structure of motor variability. Exerc. Sport Sci. Rev. 30, 26–31. doi: 10.1097/00003677-200201000-00006
Lee, D. D., and Seung, H. S. (1999). Learning the parts of objects by non-negative matrix factorization. Nature 401, 788–791. doi: 10.1038/44565
Lee, J. H., Sung, I. Y., and Yoo, J. Y. (2008). Therapeutic effects of strengthening exercise on gait function of cerebral palsy. Disabil. Rehabil. 30, 1439–1444. doi: 10.1080/09638280701618943
Mahony, K., Hunt, A., Daley, D., Sims, S., and Adams, R. (2009). Inter-tester reliability and precision of manual muscle testing and hand-held dynamometry in lower limb muscles of children with spina bifida. Phys. Occup. Ther. Pediatr. 29, 44–49. doi: 10.1080/01942630802574858
Meyns, P., Van Gestel, L., Bar-On, L., Goudriaan, M., Wambacq, H., Aertbeliën, E., et al. (2016). Children with spastic cerebral palsy experience difficulties adjusting their gait pattern to weight added to the waist, while typically developing children do not. Front. Hum. Neurosci. 10:657. doi: 10.3389/fnhum.2016.00657
Mockford, M., and Caulton, J. M. (2010). The pathophysiological basis of weakness in children with cerebral palsy. Pediatr. Phys. Ther. 22, 222–233. doi: 10.1097/PEP.0b013e3181dbaf96
Nielsen, J. B. (2003). How we walk: central control of muscle activity during human walking. Neuroscientist. 9, 195–204. doi: 10.1177/1073858403009003012
Oliveira, A. S., Gizzi, L., Farina, D., and Kersting, U. G. (2014). Motor modules of human locomotion: influence of EMG averaging, concatenation, and number of step cycles. Front. Hum. Neurosci. 8:335. doi: 10.3389/fnhum.2014.00335
Ostensjø, S., Carlberg, E. B., and Vøllestad, N. K. (2004). Motor impairments in young children with cerebral palsy: relationship to gross motor function and everyday activities. Dev. Med. Child Neurol. 46, 580–589. doi: 10.1111/j.1469-8749.2004.tb01021.x
Oudenhoven, L., van der Krogt, M. M., Buizer, A. I., Dominici, N., and Harlaar, J. (2016). Selective motor control before and after selective dorsal rhizotomy in ambulant children with cerebral palsy. Gait Posture 49:29. doi: 10.1016/j.gaitpost.2016.07.093
Petersen, T. H., Willerslev-Olsen, M., Conway, B. A., and Nielsen, J. B. (2012). The motor cortex drives the muscles during walking in human subjects. J. Physiol. 590(Pt 10), 2443–2452. doi: 10.1113/jphysiol.2012.227397
Ropars, J., Lempereur, M., Vuillerot, C., Tiffreau, V., Peudenier, S., Cuisset, J. M., et al. (2016). Muscle activation during gait in children with duchenne muscular dystrophy. PLoS ONE 11:e0161938. doi: 10.1371/journal.pone.0161938
Rose, J., and McGill, K. C. (2005). Neuromuscular activation and motor-unit firing characteristics in cerebral palsy. Dev. Med. Child. Neurol. 47, 329–336. doi: 10.1017/S0012162205000629
Rosenbaum, P., Paneth, N., Leviton, A., Goldstein, M., Bax, M., Damiano, D., et al. (2007). A report: the definition and classification of Cerebral Palsy. Dev. Med. Child Neurol. 49, 8–14. doi: 10.1111/j.1469-8749.2007.tb12610.x
Sagawa, Y., Watelain, E., De Coulon, G., Kaelin, A., Gorce, P., and Armand, S. (2013). Are clinical measurements linked to the gait deviation index in cerebral palsy patients? Gait Posture 38, 276–280. doi: 10.1016/j.gaitpost.2012.11.026
Schwartz, M. H., Rozumalski, A., and Steele, K. M. (2016). Dynamic motor control is associated with treatment outcomes for children with cerebral palsy. Dev. Child Med. 58, 1139–1145. doi: 10.1111/dmcn.13126
Shin, H. I., Sung, K. H., Chung, C. Y., Lee, K. M., Lee, S. Y., Lee, I. H., et al. (2016). Relationships between isometric muscle strength, gait parameters, and gross motor function measure in patients with cerebral palsy. Yonsei Med J. 57, 217–224. doi: 10.3349/ymj.2016.57.1.217
Shuman, B., Goudriaan, M., Bar-On, L., Schwartz, M. H., Desloovere, K., and Steele, K. M. (2016a). Repeatability of muscle synergies within and between days for typically developing children and children with Cerebral Palsy. Gait Posture 45, 127–132. doi: 10.1016/j.gaitpost.2016.01.011
Shuman, B. R., Schwartz, M. H., and Steele, K. M. (2017). Electromyography data processing impacts muscle synergies during gait for unimpaired children and children with Cerebral Palsy. Front. Comput. Neurosci. 11:50. doi: 10.3389/fncom.2017.00050
Shuman, B., Schwartz, M. H., Goudriaan, M., Desloovere, K., and Steele, K. M. (2016b). “Muscle Synergies during gait are similar before after surgery in children with cerebral palsy,” in 40th Annual Meeting of the American Society of Biomechanics (Raleigh, NC), 646.
Stackhouse, S. K., Binder-Macleod, S. A., and Lee, S. C. K. (2005). Voluntary muscle activation, contractile properties, and fatigability in children with and without cerebral palsy. Muscle Nerve 31, 594–601. doi: 10.1002/mus.20302
Steele, K. M., Rozumalski, A., and Schwartz, M. H. (2015). Muscle synergies and complexity of neuromuscular control during gait in cerebral palsy. Dev. Med. Child. Neurol. 57, 1176–1182. doi: 10.1111/dmcn.12826
Steele, K. M., Tresch, M. C., and Perreault, E. J. (2013). The number and choice of muscles impact the results of muscle synergy analyses. Front. Comput. Neurosci. 7:105. doi: 10.3389/fncom.2013.00105
Steele, K. M., van der Krogt, M. M., Schwartz, M. H., and Delp, S. L. (2012). How much muscle strength is required to walk in a crouch gait? J. Biomech. 45, 2564–2569. doi: 10.1016/j.jbiomech.2012.07.028
Stiles, J., and Jernigan, T. L. (2010). The basics of brain development. Neuropsychol. Rev. 20, 327–348. doi: 10.1007/s11065-010-9148-4
Sussman, M. (2002). Duchenne muscular dystrophy. J. Am. Acad. Orthop. Surg. 10, 138–151. doi: 10.5435/00124635-200203000-00009
Sutherland, D. H., Olshen, R., Cooper, L., Wyatt, M., Leach, J., Mubarak, S., et al. (1981). The pathomechanics of gait in Duchenne muscular dystrophy. Dev. Med. Child Neurol. 23, 3–22. doi: 10.1111/j.1469-8749.1981.tb08442.x
Tang, L., Li, F., Cao, S., Zhang, X., Wu, D., and Chen, X. (2015). Muscle synergy analysis in children with cerebral palsy. J. Neural Eng. 12:46017. doi: 10.1088/1741-2560/12/4/046017
Ting, L. H., Chiel, H. J., Trumbower, R. D., Allen, J. L., McKay, J. L., Hackney, M. E., et al. (2015). Neuromechanical principles underlying movement modularity and their implications for rehabilitation. Neuron 86, 38–54. doi: 10.1016/j.neuron.2015.02.042
Ting, L. H., and Macpherson, J. M. (2005). A limited set of muscle synergies for force control during a postural task. J. Neurophysiol. 93, 609–613. doi: 10.1152/jn.00681.2004
van der Krogt, M. M., Delp, S. L., and Schwartz, M. H. (2012). How robust is human gait to muscle weakness? Gait Posture 36, 113–119. doi: 10.1016/j.gaitpost.2012.01.017
van der Krogt, M. M., Oudenhoven, L., Buizer, A. I., Dallmeijer, A., Dominici, N., and Harlaar, J. (2016). The effect of EMG processing choices on muscle synergies before and after BoNT-A treatment in cerebral palsy. Gait Posture. 49S:31. doi: 10.1016/j.gaitpost.2016.07.095
Volpe, J. J. (2000). Overview: normal and abnormal human brain development. Ment. Retard Dev. Disabil. Res. Rev. 6, 1–5. doi: 10.1002/(SICI)1098-2779(2000)6:1<1::AID-MRDD1>3.0.CO;2-J
Wiley, M. E., and Damiano, D. L. (1998). Lower-extremity strength profiles in spastic cerebral palsy. Dev. Med. Child Neurol. 40, 100–107. doi: 10.1111/j.1469-8749.1998.tb15369.x
Willemse, L., Brehm, M. A., Scholtes, V. A., Jansen, L., Woudenberg-Vos, H., and Dallmeijer, A. J. (2013). Reliability of isometric lower-extremity muscle strength measurements in children with cerebral palsy: implications for measurement design. Phys. Ther. 93, 935–941. doi: 10.2522/ptj.20120079
Keywords: cerebral palsy, Duchenne muscular dystrophy, motor control, muscle synergies, gait analysis, muscle weakness
Citation: Goudriaan M, Shuman BR, Steele KM, Van den Hauwe M, Goemans N, Molenaers G and Desloovere K (2018) Non-neural Muscle Weakness Has Limited Influence on Complexity of Motor Control during Gait. Front. Hum. Neurosci. 12:5. doi: 10.3389/fnhum.2018.00005
Received: 26 July 2017; Accepted: 08 January 2018;
Published: 31 January 2018.
Edited by:
Jae Kun Shim, University of Maryland, College Park, United StatesReviewed by:
Yi-Ning Wu, University of Massachusetts Lowell, United StatesCopyright © 2018 Goudriaan, Shuman, Steele, Van den Hauwe, Goemans, Molenaers and Desloovere. This is an open-access article distributed under the terms of the Creative Commons Attribution License (CC BY). The use, distribution or reproduction in other forums is permitted, provided the original author(s) and the copyright owner are credited and that the original publication in this journal is cited, in accordance with accepted academic practice. No use, distribution or reproduction is permitted which does not comply with these terms.
*Correspondence: Marije Goudriaan, bWFyaWplLmdvdWRyaWFhbkBnbWFpbC5jb20=
Disclaimer: All claims expressed in this article are solely those of the authors and do not necessarily represent those of their affiliated organizations, or those of the publisher, the editors and the reviewers. Any product that may be evaluated in this article or claim that may be made by its manufacturer is not guaranteed or endorsed by the publisher.
Research integrity at Frontiers
Learn more about the work of our research integrity team to safeguard the quality of each article we publish.