- 1Neurophysics, Max Planck Institute for Human Cognitive and Brain Sciences, Leipzig, Germany
- 2Cognitive Neurology, Max Planck Institute for Human Cognitive and Brain Sciences, Leipzig, Germany
The habenula is a small but important nucleus located next to the third ventricle in front of the pineal body. It helps to control the human reward system and is considered to play a key role in emotion, showing increased activation in major depressive disorders. Its dysfunction may underlie several neurological and psychiatric disorders. It is now possible to visualize the habenula and its anatomical subdivisions—medial habenula (MHB) and lateral habenula (LHB)—using MR techniques. The aim of this study was to further differentiate substructures within human lateral habenula (LHB) using ex vivo ultra-high field MR structural imaging, distinguishing between a medial part (m-LHB) and a lateral part (l-LHB). High resolution T1w images with 0.3-mm isotropic resolution and T2*w images with 60-micrometer isotropic resolution were acquired on a 7T MR scanner and quantitative maps of T1 and T2* were calculated. Cluster analysis of image intensity was performed using the Fuzzy and Noise Tolerant Adaptive Segmentation Method (FANTASM) tool. Ultra-high resolution structural MRI of ex vivo brain tissue at 7T provided sufficient SNR and contrast to discriminate the medial and lateral habenular nuclei. Heterogeneity was observed in the lateral habenula (LHB) nuclei, with clear distinctions between lateral and medial parts (m-LHB, l-LHB) and with the neighboring medial habenula (MHB). Clustering analysis based on the T1 and T2* maps strongly showed 4–6 clusters as subcomponents of lateral and medial habenula.
Introduction
The habenula (HB) is a small but important nucleus located next to the third ventricle in front of the pineal body (Figure 1). It is considered to play a key role in controlling emotion (Hikosaka et al., 2008; Hikosaka, 2010), and its dysfunction may underlie several neurological and psychiatric disorders: Overactivation is associated with depression (Morris et al., 1999; Sartorius and Henn, 2007). The habenula is divided into a medial and lateral habenula based on histological investigations (Herkenham and Nauta, 1977, 1979), mainly receiving inputs from limbic and basal ganglia forebrain structures through the stria medullaris and projecting via fasciculus retroflexus to dopaminergic, serotonergic, and noradrenergic midbrain areas.
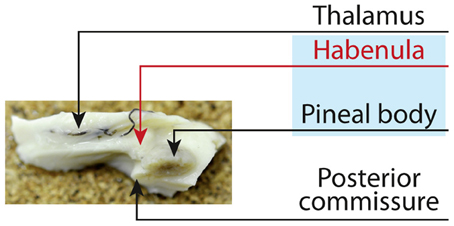
Figure 1. The Habenula, the small rein, sits next to the third ventricle above the thalamus prior to the posterior commissure. Together with the pineal body the habenula is regarded as the epithalamus. The habenular commissure connects the habenula on both hemispheres and forms a trigone in front of the posterior commissure [published in Strotmann et al. (2013)].
Herkenham and Nauta (1977) proposed a heterogeneous architecture of the lateral habenula that included a medial part (m-LHB) associated with the limbic system and a lateral part (l-LHB) associated with the motor system. Diversity within lateral habenula has also been reported by Iwahori (1977) and Andres et al. (1999). Histology of the human brain has shown the division into a medial and lateral habenula (Riley, 1943; Ranft et al., 2010). Alterations of habenula volume associated with a cell loss have been reported in studies investigating patients with major depressive disorder both in vivo (Savitz et al., 2011) and ex vivo (Ranft et al., 2010).
Few studies have investigated the anatomical complexity of the habenula. However, these studies have mainly focused on the histology of the rat and cat brain. At standard field strengths (=3T) MRI of the habenula shows few internal details. The habenula shares properties of both gray and white matter that only become visible at a high enough field strength and spatial resolution.
It has recently been shown that human habenula and its subdivisions—medial habenula (MHB) and lateral habenula (LHB)—can now be clearly visualized using MRI (Strotmann et al., 2013). This study aimed to use ex vivo ultra-high field MR structural imaging to further differentiate between volumetrically different subregions within the lateral habenula (LHB), and also to distinguish between m-LHB and l-LHB.
Materials and Methods
Data Acquisition
MRI experiments were performed on a 7T whole-body MR scanner (MAGNETOM 7T, Siemens Healthcare, Erlangen, Germany) with a custom-built miniature loop coil for post mortem brain samples (Figure 2). To this end, one post mortem brain was fixed in 4% formalin within 24 h after death (female, 65 years old, cardiac failure). Formalin fixation of brain tissue decreases relaxation parameters (Tovi and Ericsson, 1992; Dawe et al., 2009), which needs to be considered when choosing parameters for MR image acquisition.
These post mortem results are consistent with in vivo data obtained from volunteer human subjects (Strotmann et al., 2013), given the poorer spatial resolution available in vivo.
The coil consisted of a single loop made of tin-plated semi-rigid coax (coax diameter 2.2 mm) with a 13-mm inner diameter (Figure 3). The sample was placed in a plastic pipette centered in the loop. The cylindrical shape of the pipette avoided unwanted B0 distortions.
The loop was tuned to 297.2 MHz and matched to 50 Ohm with variable capacitors (Thin-Trim 9402 series, Johanson Manufacturing, USA) that allowed the coil to be adjusted to different loads. Fixed value capacitors were connected in parallel to compensate for the small quality factor of the trimmer capacitors. A bazooka balun was used to suppress unwanted cable waves. The supporting frame was made of polypropylene, a low loss dielectric material. A separate Transmit/Receive-Switch (Stark Contrast, Erlangen, Germany) was used for interfacing with the scanner.
We acquired high resolution maps of T1 with 0.3-mm isotropic resolution using an MP2RAGE sequence (Marques et al., 2010) (TR = 3000 ms, TE = 2.61 ms, TI1 = 150 ms, TI2 = 900 ms, 4 averages). For T*2 contrast we obtained data with 60-μm isotropic resolution using a 3D FLASH (Fast Low Angle Shot) multi-echo gradient echo sequence (GRE) with the following parameters (TR = 54 ms, 288 slices, flip angle = 25°, 9 averages). Echo times (TE) used were 10, 20, 29.9, 39.8, and 54 ms.
Image Analysis
MRI images and brain sections were compared with macroanatomical landmarks (Mai et al., 2008), and FSL (FMRIB Software Library, University of Oxford 2006, http://www.fmrib.ox.ac.uk/fsl) and the software package MIPAV [Medical Image Processing, Analyzing and Visualization (http://mipav.cit.nih.gov/, version 5.4.4)] from the National Institute of Health (NIH) with its plug-ins were used for further analysis of the data. Given the high degree of inter-subject variability and asymmetry of the structure, defining subcompartments of the habenula properly required an accurate delineation of the complete tissue for the data set. Boundaries were defined based on the structural identification of anatomical features (Riley, 1943; Mai et al., 2008).
The use of different contrasts provided additional information regarding the properties of habenular tissue. For this purpose the MRI parameters T1 (using the MP2RAGE sequence) and T*2 (using a GRE sequence) were quantitatively mapped.
Initially, we selected four anatomical landmarks (on the brain tissue surface close to the habenula, as indicated in Figure 4) to register the T1 map to the higher resolution T*2 image, and minimized the distance between landmarks selected in the two images with a least square algorithm (Arun et al., 1987). This approximation was further improved by an optimized automated registration algorithm (Jenkinson and Smith, 2001).
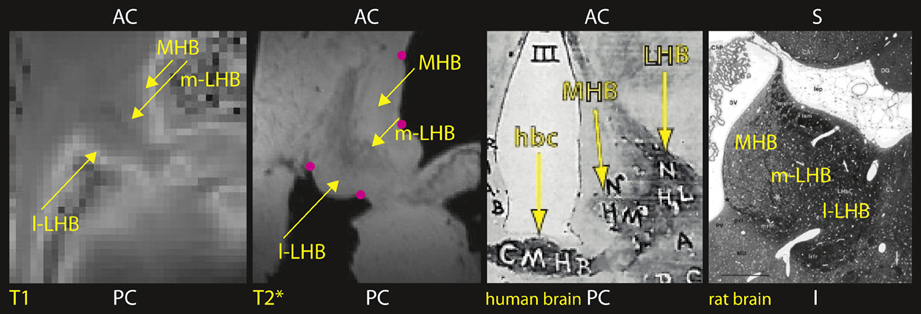
Figure 4. Human Habenula ex vivo (axial view, from left to right): Maps of T1 and T2* show distinct habenular subcompartments: lateral habenula (LHB) with a lateral part (l-LHB) and a medial part (m-LHB) and medial habenula (MHB). In comparison, histological stain section of human (axial view) and rat brain (Andres et al., 1999) (coronal view). Anatomical landmarks used for registration as indicated by pink dots.
To improve the accuracy of the registration, the inversion recovery image with the longest inversion time (INV2) of the MP2RAGE data was co-registered to the T*2 image using the same registration procedure and the results were compared. The inversion recovery image with the second inversion time shows much better contrast-to-noise ratio across different tissue types. Therefore, we registered the T1 map to the results of the INV2 to the T*2 data.
As extraneous tissue might contaminate the clustering results, we created a habenula mask based on the higher resolution T*2 image, used both modalities to cross-check the mask and applied to both contrasts. To distinguish between subcompartments within habenula, images were segmented by the Fuzzy and Noise Tolerant Adaptive Segmentation Method (FANTASM) tool (Pham and Prince, 1999) of the TOADS-CRUISE plugins for MIPAV (http://www.nitrc.org/projects/toads-cruise/). For a given input number of tissue classes, this tool classifies each pixel of the images as belonging to a particular class, and further estimates inhomogeneity, attributing a membership value from 0 to 1 for each class (Bazin et al., 2007). After the number of clusters has been selected, the intensity centroids, gain fields, and membership values are calculated. We performed this analysis for k = 2, 3, 4, 5, 6, 7 clusters within the habenula.
Results
Figure 4 shows, in axial sections through the habenula, computed parameter maps of the relaxation times T1 and T*2. Lateral habenula (LHB) and medial habenula (MHB) are clearly visible. The habenula can be visualized on both maps and therefore shows signal characteristics of both gray and white matter. Subdivisions of lateral habenula, medial (m-LHB), and lateral (l-LHB) can be distinguished. The medial part (m-LHB) shows a higher contrast from the surrounding brain tissue and differs from l-LHB and medial habenula (MHB), and we compared to the histology of human and rat brain (Riley, 1943; Andres et al., 1999).
Figure 5 shows color-coded images based on image intensities of all voxels within the habenular nucleus based on T1 and T*2 contrast for a given number of clusters. The weight of each cluster is represented by an arbitrary gray-white intensity scale where the lowest intensity is represented by the darkest shade and the brightest shade indicates high mean values. It depicts the difference between clusters when a Gaussian distribution of intensity in each cluster is assumed.
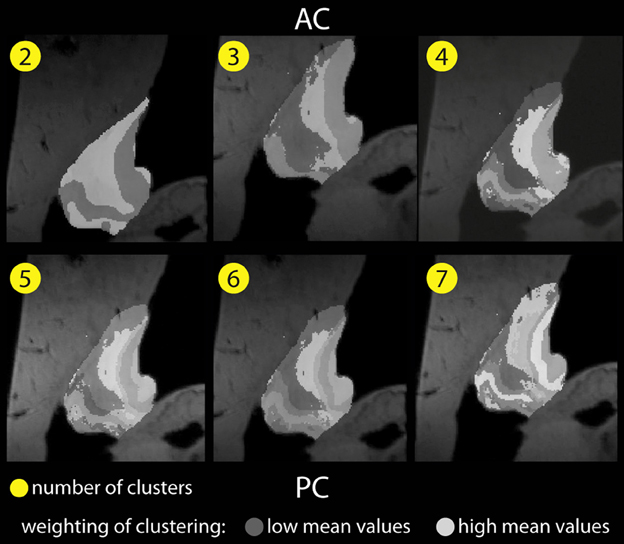
Figure 5. Habenular clustering based on image intensities in T1 (MP2RAGE) and T*2 (GRE) images of ex vivo data. K = 2, 3, 4, 5, 6, 7 clusters (from left above to right below).
Anatomic details present in the ex vivo T1 and T*2 contrast MR data become more apparent, and show several compartments within the habenula. As the number of clusters increases, we find a distribution of intensities in the lateral habenula (LHB) that is more diverse than in medial habenula (MHB).
When 4 to 6 clusters are assumed, similar patterns are revealed and the clustering found appears to be less dependent on the number of clusters. The spatial configuration of the clusters found is also more consistent.
Figure 6 shows the mean intensity values of T1 and T*2 for each clustering with its standard error as an ellipse and the distribution of the mean intensities. Mean intensity values for each clustering reveal different subdivisions in lateral and medial habenula.
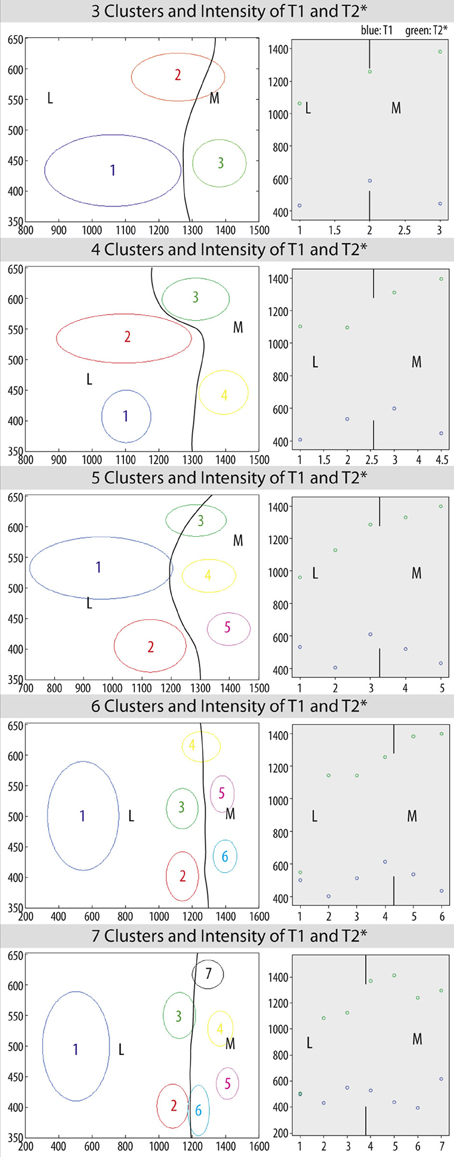
Figure 6. Mean intensity values of T1 and T2* with standard error for each cluster (k = 3, 4, 5, 6, 7) as an ellipse (left) and distribution of mean intensity values for T1 (blue) and T2* (green) (right) and subdivisions of habenula as marked by lines and indicated by M for medial habenula (MHB) and L for lateral habenula (LHB).
Discussion
Ex vivo ultra-high resolution 7T MR imaging of the human habenula provides sufficient signal-to-noise and contrast-to-noise ratio to enable a clear visualization and identification of the lateral and medial nuclei of this important brain component.
Our data revealed heterogeneity of the lateral habenular (LHB) nuclei as suggested by Herkenham and Nauta (1977), Iwahori (1977), and Andres et al. (1999). A lateral and a medial part (l-LHB, m-LHB) can be clearly discriminated, and distinguished from the neighboring medial habenula (MHB).
Furthermore, advanced registration procedures and clustering methods showed subcomponents of lateral habenular nucleus as well as in medial habenula based on the contrast in quantitative maps of T1 and T2*. Between 4 and 6 clusters having different image intensities within the habenular nucleus can be observed, which distinguish between subdivisions of the lateral and medial habenular nuclei.
Lateral and medial habenula have previously been shown with histology in the human cadaver brain, and several subdivisions were shown in lateral and medial habenula in the animal brain. For the first time, we can observe subdivisions of lateral and medial habenula in the human brain. Using MRI, in particular, we can confirm heterogeneity of lateral habenula, which have previously been reported in the rat and cat brain.
Our findings are in line with previous findings based on structural and diffusion data of the human habenula that showed in vivo a subcompartment between medial and lateral habenula (Strotmann et al., 2013).
The foci of this study were high-field post-mortem MRI, the analysis of these images, and the potential for transfer of these findings for the interpretation of in vivo MRI scans. Because of the unique ability of MRI to visualize brain structures in three dimensions, ex vivo MRI is becoming increasingly important (e.g., Leuze et al., 2013). Future studies will include detailed correlation of these findings to histology.
Our ex vivo results should help to interpret in vivo scans of the habenula region, when the increasing well-understood reduction in relaxation parameters after fixation has been taken into account.
Use of a small-animal MR system will provide results with still higher spatial resolution. However, to facilitate comparison with future in vivo experiments, we performed this study using a 7T whole-body system, which enabled easy transfer of scanning parameters and sequences. The loss of SNR that might occur when using small tissue blocks and whole brain RF coils was avoided by the use of a custom-built miniature single loop coil. Similar image quality within reasonable scanning time and with (0.15 mm)3 resolution may be achievable in vivo, with optimized scan parameters and a 64-channel phased array RF receive coil. In addition, simultaneous slice excitation pulse techniques using only 2D phase encoding might be used for this purpose.
Our analytical approach reveals only the clustering of habenular tissue based on prior assumptions (k = 2–7 clusters). We compared clustering patterns and found that assuming 4 to 6 clusters gives similar findings, which appeared to be less dependent on the number of assumed clusters. Our analysis approach is based on this prior assumption, whereas a probabilistic clustering approach such as k-means clustering might be less prone to a potential bias, but harder to evaluate, especially due to the small sample size (N = 1).
The layered pattern we found is consistent with Iwahori (1977) description of the neuronal organization in the cat, where MHB was found to consist of small compactly arranged neurons of two types (piriform and fusiform shape), and LHB contained four different types of neurons (large, medium, small sized projections neurons, and small cells with short axons). Andres et al. (1999) described MHB in the rat brain as containing small, densely packed neurons, m-LHB with smaller cells and fewer myelinated fibers, l-LHB consisted of larger neurons and more myelinated fibers forming the lateral root of fasciculus retroflexus.
This finer parcellation of the habenula may be particularly useful in patients suffering from major depression where altered function and structure of the habenula, including cell loss and reduced volumes, has been reported (Ranft et al., 2010; Savitz et al., 2011). Inhibition of activity in the lateral habenula via pharmacology or deep brain stimulation (Sartorius et al., 2010; Winter et al., 2010) is thought to decrease the symptoms of depression toward remission.
Medial lateral habenula (m-LHB) is considered to be associated with the limbic system, and its lateral part (l-LHB) is thought to be linked to the motor system (Herkenham and Nauta, 1977).
The habenula's connectivity to the limbic emotional system and the motor system might be crucial to gain a deeper understanding of its role in psychiatric and neurological disorders. In major depression, the illness is described as a combination of different symptoms, mainly characterized by a negative mood which absorbs all energy and involves motor, vegetative, and cognitive symptoms. A sudden remission of treatment-resistant patients with major depression has been reported after DBS of lateral habenula by Sartorius et al. (2010). Therefore, future investigations and pharmacological research should target the lateral and medial part of lateral habenula and its role in this “core illness circuit” in major depression.
These findings may also help to guide fMRI studies of the habenula which explore the role of lateral habenula in healthy brain function and in disease.
Conflict of Interest Statement
The authors declare that the research was conducted in the absence of any commercial or financial relationships that could be construed as a potential conflict of interest.
References
Andres, K. H., von Düring, M., and Veh, R. W. (1999). Subnuclear organization of the rat habenular complexes. J. Comp. Neurol. 407, 130–150. doi: 10.1002/(SICI)1096-9861(19990428)407:1<130::AID-CNE10>3.0.CO;2-8
Arun, K. S., Huang, T. S., and Blostein, S. D. (1987). Least-squares fitting of two 3-D point sets. IEEE Trans. Pattern Anal. Mach. Intell. 9, 698–700. doi: 10.1109/TPAMI.1987.4767965
Bazin, P. L., Cuzzocroe, J. L., Yassa, M. A., Gandler, W., McAuliffe, M. J., Bassett, S. S., et al. (2007). Volumetric neuroimage analysis extensions for the MIPAV software package. J. Neurosci. Methods 165, 111–121. doi: 10.1016/j.jneumeth.2007.05.024
Dawe, R. J., Bennett, D. A., Schneider, J. A., Vasireddi, S. K., and Arfanakis, K. (2009). Postmortem MRI of human brain hemispheres: T2 relaxation times during formaldehyde fixation. Magn. Reson. Med. 61, 810–818. doi: 10.1002/mrm.21909
Herkenham, M., and Nauta, W. J. (1977). Afferent connections of the habenular nuclei in the rat. A horseradish peroxidase study, with a note on the fiber-of-passage problem. J. Comp. Neurol. 173, 123–146. doi: 10.1002/cne.901730107
Herkenham, M., and Nauta, W. J. (1979). Efferent connections of the habenular nuclei in the rat. J. Comp. Neurol. 187, 19–47. doi: 10.1002/cne.901870103
Hikosaka, O. (2010). The habenula: from stress evasion to value-based decision-making. Nat. Rev. Neurosci. 11, 503–512. doi: 10.1038/nrn2866
Hikosaka, O., Sesack, S. R., Lecourtier, L., and Shepard, P. D. (2008). Habenula: crossroad between the basal ganglia and the limbic system. J. Neurosci. 28, 11825–11829. doi: 10.1523/JNEUROSCI.3463-08.2008
Iwahori, N. (1977). A Golgi study on the habenular nucleus of the cat. J. Comp. Neurol. 72, 319–344. doi: 10.1002/cne.901710303
Jenkinson, M. and Smith, S. (2001). A global optimisation method for robust affine registration of brain images. Med. Image Anal. 5, 143–156. doi: 10.1016/S1361-8415(01)00036-6
Leuze, C., Anwander, A., Bazin, P.-L., Dhital, B., Stüber, C., Reimann, K., et al. (2013). Layer-specific intracortical connectivity revealed with Diffusion MRI. Cereb. Cortex 49, 2569–2582. doi: 10.1093/cercor/bhs311
Mai, J. K., Paxinos, G., and Voss, T. (2008). Atlas of the Human Brain, 3rd Edn. New York, NY: Elsevier.
Marques, J. P., Kober, T., Krueger, G., van der Zwaag, W., Van de Moortele, P. F., and Gruetter, R. (2010). MP2RAGE, a self bias-field corrected sequence for improved segmentation and T1-mapping at high field. Neuroimage 49, 1271–1281. doi: 10.1016/j.neuroimage.2009.10.002
Morris, J. S., Smith, K. A., Cowen, P. J., Friston, K. J., and Dolan, R. J. (1999). Covariation of activity in habenula and dorsal raphe nuclei following tryptophan depletion. Neuroimage 10, 163–172. doi: 10.1006/nimg.1999.0455
Pham, D. L., and Prince, J. L. (1999). Adaptive fuzzy segmentation of magnetic resonance images. IEEE Trans. Med. Imaging 18, 737–752. doi: 10.1109/42.802752
Ranft, K., Dobrowolny, H., Krell, D., Bielau, H., Bogerts, B., and Bernstein, H. G. (2010). Evidence for structural abnormalities of the human habenula complex in affective disorders but not in schizophrenia. Psychol. Med. 40, 557–567. doi: 10.1017/S0033291709990821
Riley, H. (1943). An Atlas of the Basal Ganglia, Brain Stem and Spinal Cord. Baltimore, MD: Williams & Wilkins.
Sartorius, A., and Henn, F. A. (2007). Deep brain stimulation of the lateral habenula in treatment resistant major depression. Med. Hypotheses 69, 1305–1308. doi: 10.1016/j.mehy.2007.03.021
Sartorius, A., Kiening, K. L., Kirsch, P., von Gall, C. C., Haberkorn, U., Unterberg, A.W. et al. (2010). Remission of major depression under deep brain stimulation of the lateral habenula in a therapy-refractory patient. Biol. Psychiatry 67, 9–11. doi: 10.1016/j.biopsych.2009.08.027
Savitz, J. B., Nugent, A. C., Bogers, W., Roiser, J. P., Bain, E. E., Neumeister, A., et al. (2011). Habenula volume in bipolar disorder and major depressive disorders. A high resolution magnetic resonance imaging study. Biol. Psychiatry 69, 336–343. doi: 10.1016/j.biopsych.2010.09.027
Strotmann, B., Heidemann, R. M., Weiss, M., Trampel, R., Anwander, A., Villringer, A., et al. (2013). High-resolution MRI and diffusion-weighted imaging of the human habenula at 7T. J. Magn. Reson. Imaging. doi: 10.1002/jmri.24252. [Epub ahead of print].
Tovi, M., and Ericsson, A. (1992). Measurements of T1 and T1 over time in formalin-fixed human whole brain specimens. Acta Radiol. 33, 400–4004. doi: 10.1080/02841859209172021
Keywords: human habenula, habenular nuclei, lateral habenula, medial habenula, ex vivo, MRI, 7T
Citation: Strotmann B, Kögler C, Bazin P-L, Weiss M, Villringer A and Turner R (2013) Mapping of the internal structure of human habenula with ex vivo MRI at 7T. Front. Hum. Neurosci. 7:878. doi: 10.3389/fnhum.2013.00878
Received: 03 September 2013; Paper pending published: 01 October 2013;
Accepted: 02 December 2013; Published online: 23 December 2013.
Edited by:
Fritz A. Henn, Mt. Sinai Medical Center, USAReviewed by:
Alexander Sartorius, Zentralinstitut für Seelische Gesundheit, GermanyJunqian Xu, Icahn School of Medicine at Mount Sinai, USA
Copyright © 2013 Strotmann, Kögler, Bazin, Weiss, Villringer and Turner. This is an open-access article distributed under the terms of the Creative Commons Attribution License (CC BY). The use, distribution or reproduction in other forums is permitted, provided the original author(s) or licensor are credited and that the original publication in this journal is cited, in accordance with accepted academic practice. No use, distribution or reproduction is permitted which does not comply with these terms.
*Correspondence: Barbara Strotmann, Department of Neurophysics, Max Planck Institute for Human Cognitive and Brain Sciences, Stephanstraße 1a, 04103 Leipzig, Germany e-mail:c3Ryb3RtYW5uQGNicy5tcGcuZGU=