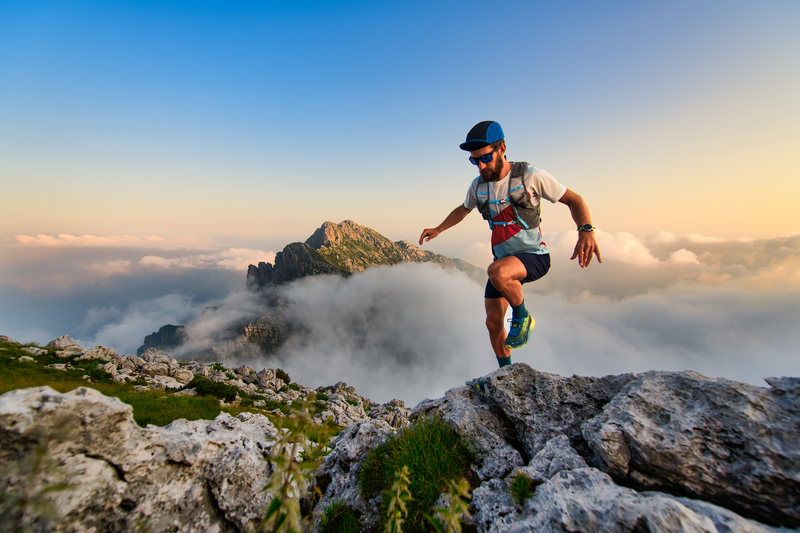
95% of researchers rate our articles as excellent or good
Learn more about the work of our research integrity team to safeguard the quality of each article we publish.
Find out more
ORIGINAL RESEARCH article
Front. Hum. Neurosci. , 18 April 2012
Sec. Sensory Neuroscience
Volume 6 - 2012 | https://doi.org/10.3389/fnhum.2012.00085
This article is part of the Research Topic Meaning in mind: Semantic richness effects in language processing View all 18 articles
Intuitively, an apple seems a fairly good example of a fruit, whereas an avocado seems less so. The extent to which an exemplar is representative of its category, referred to here as concept typicality, has long been thought to be a key dimension determining semantic representation. Concept typicality is, however, correlated with a number of other variables, in particular age of acquisition (AoA) and name frequency. Consideration of picture naming accuracy from a large case-series of semantic dementia (SD) patients demonstrated strong effects of concept typicality that were maximal in the moderately impaired patients, over and above the impact of AoA and name frequency. Induction of a temporary virtual lesion to the left anterior temporal lobe, the region most commonly affected in SD, via repetitive Transcranial Magnetic Stimulation produced an enhanced effect of concept typicality in the picture naming of normal participants, but did not affect the magnitude of the AoA or name frequency effects. These results indicate that concept typicality exerts its influence on semantic representations themselves, as opposed to the strength of connections outside the semantic system. To date, there has been little direct exploration of the dimension of concept typicality within connectionist models of intact and impaired conceptual representation, and these findings provide a target for future computational simulation.
The purpose of semantic knowledge is to allow us to recognize different instances of a particular item (e.g., that an apple and an orange are both fruits) and generalize to novel instances of that item irrespective of perceptual variation (e.g., that an avocado is also a fruit). Semantic representations are formed by extracting coherent covariation among conceptual features (i.e., seeds), allowing abstraction from the perceptual features (e.g., sweet, juicy). Of course, the perceptual features of a concept will be activated along with its semantic representation, but these are stored in separate regions of the brain. This notion of a combination of modality independent and modality-specific features that participate in semantic processing forms the basis of the hub and spoke model of semantic memory reproduced in Figure 1 (Patterson et al., 2007). Within this model, semantic representations are stored in the anterior temporal lobe (ATL) that provides the amodal hub to link the modality-specific spokes of perceptual knowledge.
Figure 1. The hub and spoke model of semantic processing. The various different modality-specific surface representations correspond to motion (yellow), colors (dark blue), shape (green), names (orange), actions (light blue) and task (purple), and are directly connected via green lines. These all connect (shown as red lines) to a shared, amodal “hub” (shown as a red area) in the anterior temporal lobes. At the hub stage, therefore, associations between different pairs of attributes (such as shape and name, shape and action, or shape and color) are all processed by a common set of neurons and synapses, regardless of the task. Adapted from Figure 1B of Patterson et al. (2007, p.977).
This model has gained considerable support from the study of patients with semantic dementia (SD), a progressive degenerative disease involving atrophy and hypometabolism of the ATLs, resulting in a selective and worsening deficit of semantic memory (Gorno-Tempini et al., 2011). These patients show deficits that cut across all modalities of testing, affecting their ability to name pictures; understand spoken words; recognize pictures, written words, environmental sounds and characteristic smells; and draw and use objects (Lambon Ralph et al., 2008). The pan-modal nature of the semantic deficits seen in SD argues strongly for the existence of amodal semantic representations housed in the ATLs, although this view is not universally accepted (Martin, 2007). It is certainly true that atrophy does spread beyond anterior temporal regions as SD progresses (Rohrer et al., 2009; Gordon et al., 2010), however evidence of the amodal nature of semantic representations in the ATLs has also been found using both distortion corrected functional imaging (Visser et al., 2010) and virtual lesion methodology using repetitive Transcranial Magnetic Stimulation (rTMS) (Pobric et al., 2010a).
To return to the apple and the avocado, it is clear that an apple is a good example of a fruit because it has features commonly found in other fruits (e.g., seeds, skin, sweet, juicy), whereas an avocado has fewer of these (e.g., seeds, skin) and also involves some more idiosyncratic features not found in many other fruits (e.g., savory, oily). The difference between the apple and the avocado can be seen as one of the typicality of their features within the fruit category. Since the proposal of the very first cognitive models of semantic memory, it has been recognized that the typicality of an item is a key dimension of its semantic representation. Smith et al. (1974) reported evidence of a concept typicality effect such that people are faster to verify that “An apple is a fruit” than “An avocado is a fruit.” Indeed, concept typicality ratings confirm that an apple is judged to be a more typical fruit than an avocado (Morrow and Duffy, 2005), given the latter's savory flavor and oily texture. Such findings motivated Collins and Loftus (1975) to incorporate spreading activation into their semantic network and indeed form the basis of the prototype theory of semantic representation proposed by Rosch and Mervis (1975).
These initial cognitive models of semantic memory are not dissimilar to more recent connectionist conceptualizations of semantic memory for concrete concepts, which incorporate the same characteristics of featural representations, spreading activation, and semantic distance (e.g., Plaut, 1996; McRae et al., 1997, 1999; Rogers et al., 2004; Dilkina et al., 2008, 2010). McRae et al. presented models in which the written word-form activated semantic representations that were made up of transparent features listed by human participants, and the connections between them were indexed to the extent to which a given feature pair co-occurred across the description of all items (e.g., seeds and sweet co-occur together in more concepts than seeds and savory). In this framework, McRae et al. were concerned with the typicality of features, rather than concepts: seeds is a typical feature of apple because apple contains other features that often co-occur with seeds (e.g., sweet and juicy), whereas it is an atypical feature of an avocado because avocado contains other features that do not often co-occur with seeds (e.g., savory and oily). Nevertheless, these models illustrate an important aspect of typicality, which is that it is determined not by the number or frequency of features, but rather by the intercorrelation between those features. In the case of concept typicality, an apple's features are highly intercorrelated with those of many other fruits, hence it represents a typical exemplar of the category, whereas an avocado's features are less intercorrelated with others in the category, hence it represents a more atypical exemplar.
The weakly intercorrelated features that characterize the representation of atypical items would be expected to be more vulnerable to damage than the strongly intercorrelated features of typical items. Hence there is a clear prediction that amongst those who suffer from a deficit in semantic memory arising from damage to the ATLs, atypical concepts like avocado will suffer more than typical ones like apple. Woollams et al. (2008) provided the first assessment of this prediction in a large case-series study of picture naming in SD, and found an overwhelmingly strong effect of typicality upon picture naming accuracy. Moreover, the patients' errors of commission were increasingly more typical than the target (e.g., an avocado might initially be called a mango and then later a pear). This influence of typicality upon performance in SD has since been confirmed in a receptive matching to sample task (Lambon Ralph et al., 2010; Mayberry et al., 2011).
There are, however, a number of other factors that may affect the susceptibility of items to the progressive semantic damage that defines SD (Lambon Ralph et al., 1998; Woollams et al., 2008). One is Age of Acquisition (AoA), which has been shown to correlate with typicality—more typical exemplars like apple tend to be learnt earlier than less typical ones like avocado (Holmes and Ellis, 2006). Another is name frequency, as more typical items like apple have names that are used more often than less typical ones like avocado. The goal of this paper is to explore the extent to which concept typicality uniquely affects the nature of the meaning we have in mind. This will be achieved in two ways: firstly, by considering its impact upon picture naming accuracy across the range of severity in SD, and secondly, by exploring how it responds to a temporary virtual lesion to the left ATL (lATL) induced by offline rTMS.
The present data set was derived from all patients listed in the Cambridge MemBrain patient database who had a clinical diagnosis of SD and who had completed picture naming on at least one occasion. This yielded a total pool of 225 observations from 78 patients, collected between 1991 and 2006. Demographic and background neuropsychological data for five severity groups containing 45 observations each can be found in Table 1. These five severity groups were created on the basis of overall picture naming accuracy and are provided for expository purposes to illustrate the key features of this patient group over the course of the disease. These data demonstrated selective and progressive semantic deficit that characterizes SD: MMSE (Folstein et al., 1975) scores were universally low; nonverbal intelligence/problem solving remained high as indicated by stable performance on Raven's Coloured Progressive Matrices (Raven, 1962); visuo-spatial processing was preserved, as seen by normal scores on the Rey Immediate Copy Test (Lezak, 1976); normal performance on the Delayed Recall version indicated preserved episodic memory; and normal forward and backward digit span (Wechsler, 1987) scores showed preserved short term memory function. These contrast with the impaired and worsening performance apparent on both verbal and nonverbal measures of comprehension [the Cambridge Spoken Word Picture Matching Test (Bozeat et al., 2000) and the picture version of the Pyramids and Palm Trees Test (Howard and Patterson, 1992)] and most prominently, the striking anomia apparent on the Cambridge Picture Naming Test.
Table 1. Demographic information and neuropsychological test scores associated with each of the 225 observations of picture naming data from semantic dementia patients included in the present study, grouped according to level of severity (Reproduced from Table 1 of Woollams et al. (2008, p.2505).
To assess the impact of concept typicality upon naming performance in SD, the objects in the 48 or 64 item Cambridge Picture Naming Tests were subdivided into equal-N High and Low Typicality sets, based upon scores for these items in the Morrow and Duffy (2005) ontological concept typicality norms for a group of older adults on a scale from one (low typicality) to seven (high typicality). Typicality scores were not available for all items: for the 48-item version, there were 40 scores available, and for the 64-itemversion, there were 50 scores available. When combining across tests, 20 items appeared in both, and hence there was a total of 70 unique items, yielding 35 lower typicality items (mean = 5.46, SD = 0.60) and 35 higher typicality items (mean = 6.55, SD = 0.27). A full listing of these items is provided in the Table A1.
In order to assess the significance of this typicality effect, the 225 observations for the higher and lower typicality items were analyzed according to their severity group (1–15) in a 2 (typicality) by 15 (severity) ANOVA with typicality as a within-participants factor and severity as a between participants factor. Severity groups based upon overall naming accuracy were created rather than using severity as a continuous predictor as the use of groups allowed a parallel analysis of the impact of typicality and severity across items. Fifteen groups of fifteen observations were used as this balanced the need to keep group sizes reasonable whilst at the same time keeping group variation low. The by-participants analysis revealed strong main effects of typicality (F(1, 210) = 1079.19, p < 0.0001), severity (F(14, 210) = 1218.08, p < 0.0001) and their interaction (F(14, 210) = 1181.76, p < 0.0001). A parallel analysis was conducted where the accuracy for each group of 15 participants was averaged across the 40 higher and 40 lower typicality items, allowing a 2 (typicality) by 15 (severity) ANOVA with typicality as a between items factor and severity as a within items factor. This confirmed the significant main effects of typicality (F(1, 68) = 11.43, p = 0.001), severity (F(5.40, 367.08) = 272.99, p < 0.0001)1, and their interaction (F(5.40, 367.08) = 4.35, p = 0.001). As can be seen in Figure 2, the concept typicality effect is most pronounced for the moderately impaired patients. Nonetheless, the typicality effect is significant at every level of severity.
Figure 2. Average magnitude of the concept typicality effect in picture naming accuracy (high typicality minus low typicality) for the 15 severity groups of 15 observations each, according to average overall level of accuracy, with cubic regression line and associated equation and fit. Error bars represent ±90% confidence intervals, hence those points in which they do not encompass the origin correspond to significant effects at an alpha level of 0.05 using a paired one-tailed t-test. Adapted from Figure 2 of Woollams et al., (2008, p.2507).
Yet in this set of 70 unique items, the correlation between concept typicality and AoA (taken from the Morrow and Duffy (2005) ratings from older adults) was −0.479, p < 0.001 and between concept typicality and CELEX (Baayen et al., 1993) spoken frequency [from the N-watch database (Davis, 2005)] was 0.239, p = 0.047. As indicated by the correlation of typicality with AoA and frequency, these items were not selected to provide a controlled assessment of concept typicality effects. One way to determine the unique contribution of concept typicality to naming performance in SD is to control for AoA and frequency in the items analysis that considered severity. Item values for each variable were, therefore, entered as a linear predictor of the item accuracy for each of the 15 severity groups. The results revealed significant main effects of frequency (F(1, 66) = 5.08, p = 0.028) and AoA (F(1, 66) = 10.20, p = 0.002) but not concept typicality (F(1, 66) = 2.39, p = 0.127). Critically, however, the significant interaction between concept typicality and severity (F(5.63, 371.82) = 3.11, p = 0.007) remained. An interaction between AoA and severity (F(5.63, 371.82) = 2.51, p = 0.023) was also apparent, but the interaction between severity and frequency did not reach significance (F(5.63, 371.82) = 1.69, p = 0.151). The results of this analysis show that concept typicality exerts an appreciable effect upon SD naming performance over and above AoA and frequency, particularly in patients with a moderately severe semantic deficit.
The present results revealed strong effects of concept typicality upon picture naming performance in SD, with the lower typicality items being most vulnerable to semantic damage, as expected. The largest impact of concept typicality was observed in the moderately severe SD patients, and this effect survived controlling for the correlated dimensions of AoA and frequency. The nonlinear relationship of severity to the size of the concept typicality effect indicates that initially it is the lower typicality items that decline most rapidly, and as the disease progresses, the decline in performance for higher typicality items accelerates until both are severely impaired. This result is consistent with the notion that concept typicality affects the nature of central amodal semantic representations housed in the ATLs that are damaged by progressive atrophy in SD, because the representations of lower typicality items are more vulnerable to semantic damage by virtue of their more idiosyncratic features. This interpretation produces the clear prediction that it should be possible to enhance the concept typicality effect seen in picture naming in normal participants via application of a temporary virtual lesion using rTMS to the lATL, the region most reliably affected in SD (Rohrer et al., 2008) and associated with the strongest levels of anomia (Lambon Ralph et al., 2001).
Sixteen individuals participated in this picture naming experiment. All were students from the University of Manchester and participated in the study in exchange for £20. All spoke English as a first language and had normal or corrected-to-normal vision; three participants were male. The laterality quotient yielded by the Edinburgh Handedness Inventory (Oldfield, 1971) was 44.17 points on average (SD = 69.44). The mean age of the participants was 20.5 years old (SD = 2.84). None of the participants were taking medication and all were free from any history of neurological disease or mental illness.
The study used a repeated measures design in which participants named a set of 96 pictures before the application of rTMS and then another set of 96 pictures after the application of TMS, with the order of sets counterbalanced according to the order of enlistment. Ninety-three of these items had concept typicality ratings in the Morrow and Duffy (2005) norms for a group of younger adults. Of these, the 40 items with the highest and the 40 items with the lowest typicality ratings were selected for consideration. The mean ratings for these items on a variety of dimensions are provided in Table 2. Between items t-tests confirmed that the high and low typicality items differed significantly in their typicality ratings and also in their rated AoA and spoken frequency (ts(1, 78) > 2.43, ps < 0.017). High and low typicality items did not differ significantly in terms of their visual complexity, name agreement or number of phonemes (ts(1, 78) < 1.19, ps > 0.237). A full listing of these items is provided in the Table A1.
Table 2. Means and standard deviations on a range of stimulus properties for the 40 low and 40 high typicality pictures used in the lATL rTMS study.
The DMDX experimental software package (Forster and Forster, 2003) was used to record RTs, vocal responses, and to display instructions and stimuli. Responses were collected by a voice key plus headset connected to an IBM compatible Pentium III computer with a 60 Hz refresh rate at 1280 by 1024 pixel screen resolution. Vocal responses were recorded from the beginning of the trial for a period of 1000 ms after the voice key triggered. Order of trial presentation was randomized anew for each participant within each block, and stimuli were presented in white on a black background. Mispronunciations and measurement errors were recorded by hand. Participants were instructed to name the centrally presented pictures as rapidly and accurately as possible. Trials began with a 500 ms fixation cross followed by the picture, which disappeared from the screen upon response or after 2000 ms. Each block of 96 pictures took around 5 min to complete.
The study used the virtual lesion method in which there was (a) a naming task (baseline), then (b) rTMS stimulation, and immediately after (c) an analogous naming task (probe). This meant that rTMS was delivered without a concurrent task and that the probe task was performed during the rTMS refractory period, which has been estimated as lasting for approximately 20 min (see Pobric et al., 2007). Focal magnetic stimulation was delivered using a 70 mm figure-of-eight coil attached to a MagStim Rapid2 stimulator (Magstim). Before experimental stimulation, motor threshold (MT) was determined for every participant as a visible twitch in the relaxed contralateral abductor pollicis brevis muscle in three out of six trials. Stimulation intensity for the experiment was then set at 120% of MT for each participant which resulted in an average of 62.42% (SD = 2.27) of the stimulator maximum output, and consisted of 10 min of 1 Hz stimulation. A structural T1-weighted MRI scan was acquired for each participant to guide TMS stimulation. As per Pobric et al. (2007), the ATL site was defined as the region 10 mm posterior from the tip of the left temporal lobe along the middle temporal gyrus, corresponding to the MNI co-ordinates of −53, 4, −32 (see Figure 3). For stimulation, this site was determined by co-registering cortical surface with 11 anatomical landmarks (inion, tip of the nose, left/right ear canals and left/right ear projections) some of which were marked prior to the scan with oil capsules (vertex, nasion, left/right ear tragus, and beneath lip in chin indentation). Co-registration was made using Ascension miniBIRD magnetic tracking system and MRIreg software (www.sph.sc.edu/comd/rorden/mrireg.html).
Figure 3. Location of the left Anterior Temporal Lobe site to which repetitive Transcranial Magnetic Stimulation was applied. Crosshairs represent site of stimulation, corresponding to the co-ordinates −53, 4, −32 in MNI space.
The correct reaction times and error rates were analyzed by two (typicality) by two (rTMS) ANOVAs, with typicality considered a within participants and between items variable, and rTMS considered as a within participants and within items variable. Analysis of the RT data, displayed in Figure 4, revealed a non-significant main effect of rTMS (F1(1, 15) = 0.51, p = 0.486; F2(1, 78) = 0.81, p = 0.371) and a main effect of typicality reliable by participants but not by items (F1(1, 15) = 5.48, p = 0.033; F2(1, 78) = 0.78, p = 0.389), which were qualified by an reliable interaction between the two, albeit it of marginal significance by participants (F1(1, 15) = 3.67, p = 0.075; F2(1, 78) = 9.99, p = 0.002). Follow-up one-tailed t-tests revealed that while the typicality effect was not significant prior to rTMS (t1(15) = 0.11, p = 0.480; t2(78) =.34, p = 0.368), it was after rTMS (t1(15) = 2.74, p = 0.008; t2(78) = 1.90, p = 0.031). Similarly, the effect of rTMS for low typicality items was significant (t1(15) = −1.84, p = 0.043; t2(39) = −2.64, p = 0.006) whereas that for high typicality items was not reliable (t1(15) = 0.85, p = 0.204; t2(39) = 1.77, p = 0.042). The parallel analyses of error rates revealed no reliable effects by participants (F(1, 15) < 2.50, p > 0.135), and only a significant main effect of rTMS by items (F(1, 78) = 4.01, p = 0.049, all other effects F(1, 78) < 1.44, p > 0.233).
Figure 4. Average reaction times for naming of high and low typicality pictures before and after the application of repetitive Transcranial Magnetic Stimulation to the left Anterior Temporal Lobe. Error bars represent ± within-participants 90% confidence intervals computed according to Loftus and Masson (1994, Equation 4, p. 485).
Across this set of 80 items, the correlation between concept typicality and AoA [taken from the Morrow and Duffy (2005) ratings from younger adults] was −0.498, p < 0.0005 and between concept typicality and CELEX (Baayen et al., 1993) spoken frequency [from the N-watch database (Davis, 2005)] was 0.261, p = 0.019. To determine the key stimulus dimension that was interacting with rTMS, item values for each variable were, therefore, entered as a linear predictor of the item RT and error rate pre and post rTMS. The results revealed a marginally significant main effect of typicality (F(1, 76) = 3.44, p = 0.068), a significant main effect of AoA (F(1, 76) = 24.43 p < 0.0001), but no reliable effect of frequency (F(1, 76) = 0.74, p = 0.392). There was, however, a significant interaction between typicality and rTMS (F(1, 76) = 5.79, p = 0.019), but not between AoA and rTMS (F(1, 76) = 0.21, p = 0.647) or frequency and rTMS (F(1, 76) = 0.58, p = 0.448). The main result of this analysis is that it is low typicality concepts that are particularly vulnerable to disruption due to lATL rTMS, again consistent with the notion that concept typlicality affects semantic representations housed in this area.
This study is the first to demonstrate a selective effect of lATL rTMS on the naming of low relative to high typicality concepts. In a previous study considering the impact of lATL stimulation on picture naming, Pobric et al. (2007) found a selective effect of lATL rTMS on naming at the specific level, in line with the greater deficits seen for this level in both SD patients (Adlam et al., 2006) and recent connectionist models of semantic representation (Rogers et al., 2004). Interestingly, Pobric et al. (2007) did not obtain an effect of lATL rTMS on basic level naming, in contrast to a later study (Pobric et al., 2010b). The stronger effect of lATL rTMS on low than high typicality concepts observed here suggests that the concept typicality of the stimuli to be named is a critical property to consider, and previous inconsistencies in the effects of lATL rTMS on basic level naming may have resulted from variation on this dimension.
The observed interaction between concept typicality and rTMS not only survived statistical control for the correlated variables of AoA and name frequency, but strikingly, neither of these variables interacted with rTMS. Although AoA has been shown to be a strong determinant of picture naming accuracy in SD (Lambon Ralph et al., 1998; Woollams et al., 2008), and frequency influences performance in SD across a variety of expressive and receptive tasks (Patterson et al., 2006; Caine et al., 2009; Jefferies et al., 2009), the impact of ATL rTMS on these effects has yet to be directly investigated. The present results demonstrate that future investigations of the impact of AoA and frequency on semantic representations in the ATL will need to take into account the correlated dimension of concept typicality.
The present findings have shown a unique effect of concept typicality in picture naming when the function of the ATL is compromised. For picture naming in SD, the lower typicality concepts were those most vulnerable to damage across the full range of severity. Performance on lower typicality concepts also declined more rapidly, producing the largest typicality effect in picture naming accuracy for the moderately impaired patients. For normal picture naming, the lower typicality concepts were those most susceptible to disruption via offline rTMS of the lATL, the region that is most commonly affected in SD (Rohrer et al., 2008) and is associated with higher levels of anomia (Lambon Ralph et al., 2001). rTMS produced a significant effect of concept typicality in RTs that was not apparent in the baseline picture naming performance of normal participants for the same items.
The concept typicality effects observed here under conditions of semantic disruption are in accordance with the predictions of the early prototype theory of meaning representation proposed by Rosch and Mervis (1975): an apple is a closer to the prototype fruit than an avocado, hence it enjoys a processing advantage. Recent connectionist models of meaning representation implement many aspects of these early accounts, such as featural representations, spreading activation and semantic distance [see Dilkina et al. (2010) and Plaut (1996)]. Particularly relevant to the present result is a simulation reported by Rogers and McClelland (2004, p.203) within a connectionist model of concrete concepts that is able to generate names for items in response to activation of their visual features via a distributed semantic system. As these semantic representations are learnt in the course of mapping inputs to outputs, they bear an opaque relationship to those features found in empirically derived feature norms. Nevertheless, typicality can be quantified for each item in the model by means of computing the similarity of its representation with that of an averaged representation for the category (the group centroid). A strong relationship was found between the typicality of an item and the number of epochs taken to generate its basic level name. To date, however, the impact of damage to the semantic level upon the magnitude of this typicality effect in naming remains unexplored.
As mentioned earlier, McRae et al. (1997, 1999) used empirically derived norms to produce models of the impact of feature typicality upon written word processing. Their work clearly illustrates the important point that concept typicality is determined by the intercorrelation between component features, rather than their number or frequency: an apple is a more typical fruit than an avocado by virtue of the stronger intercorrelation of its features with those of other fruits. The impact of concept typicality observed here under conditions of semantic impairment is in accordance with this notion that the connections within the semantic layer are determined by their degree of intercorrelation—it is an “S<>S” effect. This is consistent with the finding that concept typicality effects are also seen in semantic judgments upon objects and written words, which do not require spoken output (Morrison et al., 1992; Larochelle and Pineau, 1994; Holmes and Ellis, 2006). Although both AoA and frequency were strong determinants of picture naming performance for the SD patients, neither variable interacted with lATL rTMS, in contrast to the interaction seen for concept typicality. These results introduce the possibility that the impact of AoA and frequency may derive from their influence on connections outside the semantic system, in contrast to Steyvers and Tenenbaum (2005) proposal that AoA influences the formation of semantic representations themselves.
It is possible that the effects of AoA and frequency seen here at least partially derive from the speech production aspect of the picture naming task, which is consistent with the observation of weak effects of these variables relative to typicality in semantic categorization tasks (Barbon and Cuetos, 2006). Indeed, Lambon Ralph and Ehsan (2006) have demonstrated that while AoA may be considered a semantic effect in that it is more influential in picture naming than reading aloud, this is because its influence is most pronounced in tasks involving arbitrary input-output mappings. Given that the model they presented was successfully able to simulate this effect without any within semantic level connections (S<>S), it would seem that AoA may determine the weights on the connections mapping between semantics and phonology (“S>P”). Further, it may well be that the name frequency variable considered here exerted its influence primarily on the connections within the phonological output layer (“P<>P”), although the influence of frequency is pervasive within connectionist models (Plaut et al., 1996). Further simulation work explicitly considering the impact of concept typicality upon naming within connectionist models is clearly required to determine the locus of these effects, which in turn can generate hypotheses about the neural regions in which the impact of different stimulus variables should be most apparent.
The impact of concept typicality under conditions of semantic disruption observed here speaks to the importance of this variable in the representation of meaning, but this conclusion applies of course only to concrete concepts, due to the use of the picture naming task. To date, the vast bulk of research into semantic representation has focused on concrete concepts, which for the most part fall into natural categories, making concept typicality a pertinent dimension. This does, however, seem less applicable to the representation of abstract concepts, which form the majority of our semantic knowledge. Nevertheless, if the dimension of concept typicality is implemented in terms of the degree of intercorrelation of component features, this same general principle would also seem applicable to abstract concepts. Abstract concepts tend to have lower feature intercorrelation than concrete concepts by virtue of their more fluid and contextually dependent meanings (Harm and Seidenberg, 2004). As abstract concepts are more likely to have meanings that vary according to context, only a subset of their features are activated together on any given occasion, and thus their components co-occur less reliably than is the case for concrete concepts. Indeed, recent case-series studies have found abstract concepts to be more vulnerable to degradation than concrete concepts in SD (Jefferies et al., 2009; Hoffman and Lambon Ralph, 2011) and after lATL rTMS (Pobric et al., 2009). The common mechanism of differential feature intercorrelation functions to align these concreteness effects with the concept typicality effects found in the present study.
The unique influence of concept typicality upon picture naming performance under conditions of semantic disruption reported here illustrates the complementary roles that case-series neuropsychology and rTMS play in revealing the nature of the meaning that we have in mind and where it resides in the brain. The greater susceptibility of lower than higher typicality concepts to ATL damage in SD combined with the selective effect of lATL rTMS on naming of lower relative to higher typicality concepts clearly provides target data for future connectionist models of semantic representation and generates hypotheses for neuroimaging studies. This investigation has shown that a key aspect of conceptual representation is the extent to which component semantic features co-occur, with higher typicality items composed of features which are often activated together, leading to richer and more robust semantic representations. Hence although apples are not the only fruit, they are the most typical by virtue of their highly intercorrelated semantic features.
The author declares that the research was conducted in the absence of any commercial or financial relationships that could be construed as a potential conflict of interest.
I am very grateful to Professor John Hodges for permission to publish data from patients in his care, to Professor Karalyn Patterson for helpful discussion of the issues considered in this paper, and to Dr. Gaston Madrid for collecting the rTMS data. The rTMS work reported here was funded by a Leverhulme Trust Research Fellowship RF/2/RFG/2008/0298.
Adlam, A. L. R., Patterson, K., Rogers, T. T., Nestor, P. J., Salmond, C. H., Acosta-Cabronero, J., and Hodges, J. R. (2006). Semantic dementia and fluent primary progressive aphasia: two sides of the same coin? Brain 129, 3066–3080.
Baayen, R. H., Piepenbrock, R., and van Rijn, H. (1993). The CELEX Lexical Database (CD-ROM). Philadelphia, PA: Linguistic Data Consortium, University of Pennsylvania.
Barbon, A., and Cuetos, F. (2006). Age-of-acquisition effects in semantic categorization tasks. Psicologica 27, 207–223.
Bozeat, S., Lambon Ralph, M. A., Patterson, K., Garrard, P., and Hodges, J. R. (2000). Non-verbal semantic impairment in semantic dementia. Neuropsychologia 38, 1207–1215.
Caine, D., Breen, N., and Patterson, K. (2009). Emergence and progression of ‘non-semantic’ deficits in semantic dementia. Cortex 45, 483–494.
Collins, A. M., and Loftus, E. F. (1975). A spreading-activation theory of semantic processing. Psychol. Rev. 82, 407–428.
Davis, C. J. (2005). N-watch: a program for deriving neighborhood size and other psycholinguistic statistics. Behav. Res. Methods 37, 65–70.
Dilkina, K., McClelland, J. L., and Plaut, D. C. (2008). A single-system account of semantic and lexical deficits in five semantic dementia patients. Cogn. Neuropsychol. 25, 136–164.
Dilkina, K., McClelland, J. L., and Plaut, D. C. (2010). Are there mental lexicons? The role of semantics in lexical decision. Brain Res. 1365, 66–81.
Folstein, M. F., Folstein, S. E., and McHugh, P. R. (1975). Mini-mental state: a practical method for grading the cognitive state of patients for the clinician. J. Psychiatr. Res. 12, 189–198.
Forster, K. F., and Forster, J. C. (2003). DMDX: a Windows display program with millisecond accuracy. Behav. Res. Methods Instrum. Comput. 35, 116–124.
Gordon, E., Rohrer, J. D., Kim, L. G., Omar, R., Rossor, M. N., Fox, N. C., and Warren, J. D. (2010). Measuring disease progression in frontotemporal lobar degeneration: a clinical and MRI study. Neurology 74, 666–673.
Gorno-Tempini, M. L., Hillis, A. E., Weintraub, S., Kertesz, A., Mendez, M., Cappa, S. F., Ogar, J. M., Rohrer, J. D., Black, S., Boeve, B. F., Manes, F., Dronkers, N. F., Vandenberghe, R., Rascovsky, K., Patterson, K., Miller, B. L., Knopman, D. S., Hodges, J. R., Mesulam, M. M., and Grossman, M. (2011). Classification of primary progressive aphasia and its variants. Neurology 76, 1006–1014.
Harm, M. W., and Seidenberg, M. S. (2004). Computing the meanings of words in reading: cooperative division of labor between visual and phonological processes. Psychol. Rev. 111, 662–720.
Hoffman, P., and Lambon Ralph, M. A. (2011). Reverse concreteness effects are not a typical feature of semantic dementia: evidence for the hub-and-spoke model of conceptual representation. Cereb. Cortex 21, 2103–2112.
Holmes, S. J., and Ellis, A. W. (2006). Age of acquisition and typicality effects in three object processing tasks. Vis. Cogn. 13, 884–910.
Howard, D., and Patterson, K. (1992). Pyramids and Palmtrees: A Test of Semantic Access from Pictures and Words. Bury St. Edmunds: Thames Valley Test Company.
Jefferies, E., Patterson, K., Jones, R. W., and Lambon Ralph, M. A. (2009). Comprehension of concrete and abstract words in semantic dementia. Neuropsychology 23, 492–499.
Lambon Ralph, M. A., and Ehsan, S. (2006). Age of acquisition effects depend on the mapping between representations and the frequency of occurrence: empirical and computational evidence. Vis. Cogn. 13, 928–948.
Lambon Ralph, M. A. L., Graham, K. S., Ellis, A. W., and Hodges, J. R. (1998). Naming in semantic dementia - What matters? Neuropsychologia 36, 775–784.
Lambon Ralph, M. A., McClelland, J. L., Patterson, K., Galton, C. J., and Hodges, J. R. (2001). No right to speak? The relationship between object naming and semantic impairment: neuropsychological evidence and a computational model. J. Cogn. Neurosci. 13, 341–356.
Lambon Ralph, M. A., and Patterson, K. (2008). Generalization and differentiation in semantic memory. Ann. N.Y. Acad. Sci. 1124, 61–76.
Lambon Ralph, M. A., Sage, K., Jones, R. W., and Mayberry, E. J. (2010). Coherent concepts are computed in the anterior temporal lobes. Proc. Natl. Acad. Sci. U.S.A. 107, 2717–2722.
Larochelle, S., and Pineau, H. (1994). Determinants of response times in the semantic verification task. J. Mem. Lang. 33, 796–823.
Loftus, G. R., and Masson, M. E. J. (1994). Using confidence intervals in within-subject designs. Psychon. Bull. Rev. 1, 476–490.
Martin, A. (2007). The representation of object concepts in the brain. Annu. Rev. Psychol. 58, 25–45.
Mayberry, E. J., Sage, K., and Lambon Ralph, M. A. (2011). At the edge of semantic space: the breakdown of coherent concepts in semantic dementia is constrained by typicality and severity but not modality. J. Cogn. Neurosci. 23, 2240–2251.
McRae, K., Cree, G. S., Westmacott, R., and De Sa, V. R. (1999). Further evidence for feature correlations in semantic memory. Can. J. Exp. Psychol. 53, 360–373.
McRae, K., De Sa, V. R., and Seidenberg, M. S. (1997). On the nature and scope of featural representations of word meaning. J. Exp. Psychol. Gen. 126, 99–130.
Morrison, C. M., Chappell, T. D., and Ellis, A. W. (1997). Age of acquisition norms for a large set of object names and their relation to adult estimates and other variables. Q. J. Exp. Psychol. A 50, 528–559.
Morrison, C. M., Ellis, A. W., and Quinlan, P. T. (1992). Age of acquisition, not word frequency, affects object naming, not object recognition. Mem. Cognit. 20, 705–714.
Morrow, L. I., and Duffy, M. F. (2005). The representation of ontological category concepts as affected by healthy aging: normative data and theoretical implications. Behav. Res. Methods 37, 608–625.
Oldfield, R. C. (1971). The assessment and analysis of handedness: the edinburgh inventory. Neuropsychologia 9, 97–113.
Patterson, K., Nestor, P. J., and Rogers, T. T. (2007). Where do you know what you know? The representation of semantic knowledge in the human brain. Nat. Rev. Neurosci. 8, 976–987.
Patterson, K., Ralph, M. A. L., Jefferies, E., Woollams, A., Jones, R., Hodges, J. R., and Rogers, T. T. (2006). “Presemantic” cognition in semantic dementia: six deficits in search of an explanation. J. Cogn. Neurosci. 18, 169–183.
Plaut, D. C. (1996). Relearning after damage in connectionist networks: toward a theory of rehabilitation. Brain Lang. 52, 25–82.
Plaut, D. C., McClelland, J. L., Seidenberg, M. S., and Patterson, K. (1996). Understanding normal and impaired word reading: computational principles in quasi-regular domains. Psychol. Rev. 103, 56–115.
Pobric, G., Lambon Ralph, M. A., and Jefferies, E. (2009). The role of the anterior temporal lobes in the comprehension of concrete and abstract words: rTMS evidence. Cortex 45, 1104–1110.
Pobric, G., Jefferies, E., and Lambon Ralph, M. A. (2010a). Amodal semantic representations depend on both anterior temporal lobes: evidence from repetitive transcranial magnetic stimulation. Neuropsychologia 48, 1336–1342.
Pobric, G., Jefferies, E., and Lambon Ralph, M. A. (2010b). Category-specific versus category-general semantic impairment induced by transcranial magnetic stimulation. Curr. Biol. 20, 964–968.
Pobric, G., Jefferies, E., and Ralph, M. A. L. (2007). Anterior temporal lobes mediate semantic representation: mimicking semantic dementia by using rTMS in normal participants. Proc. Natl. Acad. Sci. U.S.A. 104, 20137–20141.
Rogers, T. T., Lambon Ralph, M. A., Garrard, P., Bozeat, S., McClelland, J. L., Hodges, J. R., and Patterson, K. (2004). Structure and deterioration of semantic memory: a neuropsychological and computational investigation. Psychol. Rev. 111, 205–235.
Rogers, T. T., and McClelland, J. L. (2004). Semantic Cognition: A Parallel Distributed Processing Approach. Cambridge, MA: MIT Press.
Rohrer, J. D., McNaught, E., Foster, J., Clegg, S. L., Barnes, J., Omar, R., Warrington, E. K., Rossor, M. N., Warren, J. D., and Fox, N. C. (2008). Tracking progression in frontotemporal lobar degeneration: serial MRI in semantic dementia. Neurology 71, 1445–1451.
Rohrer, J. D., Warren, J. D., Modat, M., Ridgway, G. R., Douiri, A., Rossor, M. N., Ourselin, S., and Fox, N. C. (2009). Patterns of cortical thinning in the language variants of frontotemporal lobar degeneration. Neurology 72, 1562–1569.
Rosch, E., and Mervis, C. B. (1975). Family resemblances: studies in the internal structure of categories. Cognit. Psychol. 7, 573–605.
Smith, E. E., Shoben, E. J., and Rips, L. J. (1974). Structure and process in semantic memory: a featural model for semantic decisions. Psychol. Rev. 81, 214–241.
Steyvers, M., and Tenenbaum, J. B. (2005). The large-scale structure of semantic networks: statistical analyses and a model of semantic growth. Cogn. Sci. 29, 41–78.
Visser, M., Embleton, K. V., Jefferies, E., Parker, G. J., and Ralph, M. A. L. (2010). The inferior, anterior temporal lobes and semantic memory clarified: novel evidence from distortion-corrected fMRI. Neuropsychologia 48, 1689–1696.
Woollams, A. M., Cooper-Pye, E., Hodges, J. R., and Patterson, K. (2008). Anomia: a doubly typical signature of semantic dementia. Neuropsychologia 46, 2503–2514.
Keywords: concept typicality, picture naming, semantic dementia, repetitive transcranial magnetic stimulation, age of acquisition, frequency
Citation: Woollams AM (2012) Apples are not the only fruit: the effects of concept typicality on semantic representation in the anterior temporal lobe. Front. Hum. Neurosci. 6:85. doi: 10.3389/fnhum.2012.00085
Received: 20 January 2012; Accepted: 25 March 2012;
Published online: 18 April 2012.
Edited by:
Penny M. Pexman, University of Calgary, CanadaReviewed by:
Chris McNorgan, Northwestern University, USACopyright: © 2012 Woollams. This is an open-access article distributed under the terms of the Creative Commons Attribution Non Commercial License, which permits non-commercial use, distribution, and reproduction in other forums, provided the original authors and source are credited.
*Correspondence: Anna M. Woollams, Neuroscience and Aphasia Research Unit, School of Psychological Sciences, Zochonis Building, University of Manchester, Brunswick Street, Manchester M13 9PL, UK. e-mail:YW5uYS53b29sbGFtc0BtYW5jaGVzdGVyLmFjLnVr
Disclaimer: All claims expressed in this article are solely those of the authors and do not necessarily represent those of their affiliated organizations, or those of the publisher, the editors and the reviewers. Any product that may be evaluated in this article or claim that may be made by its manufacturer is not guaranteed or endorsed by the publisher.
Research integrity at Frontiers
Learn more about the work of our research integrity team to safeguard the quality of each article we publish.