- 1 Department of Cognitive Science, Macquarie University, Sydney, NSW, Australia
- 2 Department of Neurophysiology, Max Planck Institute for Brain Research, Frankfurt am Main, Germany
- 3 Department of Psychology, The Australian National University, Canberra, ACT, Australia
- 4 Australian Research Council Centre of Excellence in Cognition and Its Disorders, Perth, WA, Australia
- 5 Brain, Body and Self Laboratory, Department of Neuroscience, Karolinska Institute, Stockholm, Sweden
Face perception generates specific neural activity as early as 170 ms post-stimulus onset, termed the M170 when measured with Magnetoencephalography (MEG). We examined the M170 in six people with congenital prosopagnosia (CP) and 11 typical controls. Previous research indicates that there are two neural generators for the M170 (one within the right lateral occipital area – rLO and one within the right fusiform gyrus – rFG), and in the current study we explored whether these sources reflect the processing of different types of information. Individuals with CP showed face-selective M170 responses within the rLO and right rFG, which did not differ in magnitude to those of the controls. To examine possible links between neural activity and behavior we correlated the CPs’ MEG activity generated within rLO and rFG with their face perception skills. The rLO-M170 correlated with holistic/configural face processing, whereas the rFG-M170 correlated with featural processing. Hence, the results of our study demonstrate that individuals with CP can show an M170 that is within the normal range, and that the M170 in the rLO and rFG are involved in different aspects of face processing.
Introduction
The ability to recognize faces is supported by a number of brain regions (Haxby et al., 2000; Kanwisher, 2010), some of which are specifically dedicated to the processing of faces (Kanwisher, 2010). Psychophysiological studies have shown that responses to faces are larger than those to non-face objects from ∼170 ms post-stimulus onset as measured with event-related potentials (ERPs; labeled as the N170, Bentin et al., 1996) and magnetoencephalography (MEG; labeled as M170, Liu et al., 2000). Most studies of the M/N170 have focused on individuals without face recognition difficulties, who are typically able to effortlessly recognize hundreds, if not thousands of people by their face. However, since the M/N170 reflects early face-sensitive processing (Eimer, 2000b; Zheng et al., 2011), more recently there has been increased interest in studying its characteristics in people suffering from prosopagnosia. Prosopagnosia is a selective impairment in recognizing faces which can be acquired via neurologic conditions such as stroke or a head injury (De Renzi et al., 1991; Barton, 2008; Riddoch et al., 2008), or congenital with the impairment being life long and in the absence of any brain injury (Duchaine, 2000; Behrmann and Avidan, 2005; Duchaine and Nakayama, 2006b; Rivolta et al., 2010, 2012a; Palermo et al., 2011)1.
The findings of eight published single-case investigations of the N170 in acquired prosopagnosia (AP) are contradictory. While three APs showed a lack of face-selective neural processing (i.e., an N170 of equal magnitude for faces and objects; Eimer, 2000b; Dalrymple et al., 2011), five APs showed a face selective N170 just as controls (Bobes et al., 2004; Alonso Pietro et al., 2011; Dalrymple et al., 2011). Similarly, the results of previous investigations of the N/M170 in congenital prosopagnosia (CP) are varied (see Table A1 in Appendix for a summary). Nine of the described CPs did not show any face-selective neural activity, whereas the other five did (Bentin et al., 1999, 2007; Kress and Daum, 2003; Harris et al., 2005; DeGutis et al., 2007; Minnebusch et al., 2007). The reason for this heterogeneity is not clear, and previous investigations have failed to find any correspondence between the magnitude of the CPs behavioral impairment on tasks assessing both familiar and unfamiliar face processing, and the face-selectivity of their N170 (Harris et al., 2005; Minnebusch et al., 2007).
A number of studies in typical subjects have localized the neural generators of the M/N170 within “core” face processing regions, such as the lateral part of the occipital cortex (LO) and the fusiform gyrus (FG; Linkenkaer-Hansen et al., 1998; Watanabe et al., 2003; Deffke et al., 2007; Itier et al., 2007). However, even after many years of research on the typical features of the M/N170, it still remains unclear whether these two generators work in concert in giving rise to a single M/N170 (recorded at the electrode or sensor level in occipito-temporal areas), or whether each generator might give rise to a face component with specific features. It is known that upright face processing is mediated by both holistic/configural mechanisms, which involve an analysis of the whole face rather than just individual features, and by featural mechanisms, which are responsible for the processing of specific facial features (Young et al., 1987; Tanaka and Farah, 1993; Maurer et al., 2002). It has been shown that the N/M170 is sensitive to manipulations that disrupt holistic/configural face processing, such as face inversion, which delays the N/M170 by ∼10–13 ms (Bentin et al., 1996; Liu et al., 2000; Rossion et al., 2000; Itier et al., 2006). In addition, adaptation paradigms have revealed the N/M170 to be sensitive to variations of both face configuration and facial features (Harris and Aguirre, 2008; Harris and Nakayama, 2008; Eimer et al., 2010).
In the current study, we correlated MEG activity, as indexed by the face-specific M170, with holistic/configural and featural processing skills in a group of six individuals with CP. It has been suggested that neural activity within the lateral occipital cortex mainly mediates processing of features, whereas FG activity mediates both features and holistic/configural processing (Yovel and Kanwisher, 2005; Schiltz and Rossion, 2006; Maurer et al., 2007; Pitcher et al., 2007; Liu et al., 2009). Given the above mentioned heterogeneity of CP both in terms of both neural (N/M170) and behavioral characteristics (featural and configural processing), we envisaged that CPs would represent an ideal population to further study the correlation between the two.
In sum, the main aims of the current study were to investigate whether our group of six CPs would show a typical M170 response to faces and whether there is a correlation between the CPs neural responses to faces and their behavioral face processing skills, by examining the correlation between their M170 and their performance on configural and featural face processing tasks.
Materials and Methods
This study was approved by the Macquarie University Ethics Committee, and written consent was obtained from all participants.
Participants
Congenital prosopagnosics
Six CPs (3 female) with a mean age of 42.7 years (Range: 21–57, SD: 13.78) completed a behavioral diagnostic assessment session, followed by an MEG testing session between 8 and 12 months later (Table 1). All of them reported lifelong difficulties in face recognition and were recruited via the MACCS Prosopagnosia Register (https://www.maccs.mq.edu.au/research/projects/prosopagnosia/register). None of them had any history of psychiatric or neurological conditions, and all had normal or corrected-to-normal vision.
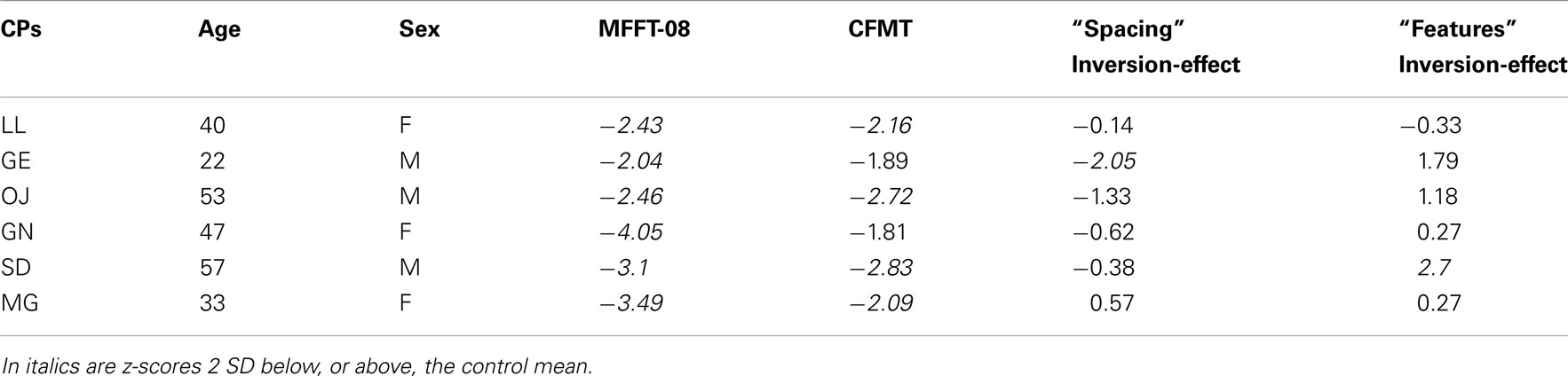
Table 1. Biographical information for the six CPs, z-scores on the MACCS Famous Face Task 2008 (MFFT-08), the Cambridge Face Memory Test (CFMT) adjusted for age (see Bowles et al., 2009 for normative data), and z-scores for the face-inversion effect on the spacing and features sets of the Jane task.
Behavioral Diagnostic Assessment Session
All CPs completed a behavioral assessment session during which they were administered both general visual processing and face processing tasks. This session had the aim to provide a “diagnosis” of CP. The performance of each CP was compared to standard norms or, when not available, to a control sample that we collected.
Non-face processing assessment
Congenital prosopagnosics completed the Functional Acuity Contrast Test (FACT, Vision Sciences Research Corporation, 2002) for the assessment of contrast sensitivity and the Ishihara Test for Color Blindness (Ishihara, 1925) for the assessment of color perception. Object recognition was assessed with the length, size, orientation, and picture naming (long version) subtests of the Birmingham Object Recognition Battery (BORB; Riddoch and Humphreys, 1993), and IQ was estimated with the Raven Colored Progressive Matrices (Raven et al., 1998). Finally, CPs completed the Autism-Spectrum Quotient (AQ, Baron-Cohen et al., 2001) to exclude impairment in social functioning.
Face processing assessment
Face memory: famous faces. Memory for familiar faces was assessed with the MACCS Famous Face Test 2008 (MFFT-08, Palermo et al., 2011). The MFFT-08 contains the faces of 20 people who are famous to the Australian population, and 20 who are unfamiliar. On each trial, a face was presented and participants judged whether it was familiar or not. If the face was that of a famous person, participants were then asked to identify the face by providing the name or specific autobiographical information (i.e., an answer like “she is an American actress” was considered incorrect). Participants were then shown the name of the famous person, accompanied by relevant autobiographical details, and participants were asked to report whether the famous person was actually known to them. The face of any person that was unknown was excluded from further analyzes.
Face memory: unfamiliar faces. Memory for unfamiliar faces was assessed with the Cambridge Face Memory Test (CFMT, Duchaine and Nakayama, 2006a). On this task participants were asked to learn to identify six individuals and then recognize the previously seen faces when shown in novel views and/or degraded by noise.
Holistic/configural processing. Perceiving the identity of faces is more difficult when they are upside-down than upright (Yin, 1969), and the effects of inversion are larger for faces than non-face objects (Robbins and McKone, 2007). The face-inversion effect has generally been attributed to a disruption of holistic/configural processing mechanisms with inversion (Tanaka and Farah, 1993; McKone, 2010). Here, we assessed holistic/configural face processing by investigating the effect of face inversion on the discrimination of sequential pairs of faces that varied in their features (i.e., the eyes, nose, and mouth of the original face were replaced with features from a different face) or the spacing of their internal features (i.e., eyes were either moved in or out; eyes and mouth were moved either up or down)2. Spacing processing has been considered as an index of holistic/configural mechanisms (Maurer et al., 2002; McKone and Yovel, 2009). This task, known as the “Jane task,” has been used in numerous studies with typical participants, who show a greater effect of inversion for faces that vary in spacing as compared to those that vary by feature, indicating a greater role of holistic/configural processing for detecting “spacing” rather than “feature” changes (Mondloch et al., 2002; Le Grand et al., 2006)3
On each trial of the Jane task, a face was shown for 200 ms, followed by a 300-ms interval, and then a second face was shown until participants made a response as to whether the faces were the same or different. The trials were blocked (upright spacing, upright feature, inverted spacing, inverted feature), with 30 trials in each block. For each participant, a face-inversion effect (upright minus inverted) was calculated for the spacing and feature conditions (see Figure A1 in Appendix for example of the Jane task stimuli).
Magnetoencephalography Session
Participants
The six CPs as well as 11 controls subjects with typical face processing skills (5 Female, Mean age: 37.3, Range: 23–55, SD: 11.21) participated in the MEG experiment.
Experimental design
Magnetoencephalography activity was recorded while participants performed a “Target detection task” (Rivolta et al., 2012b). In this task, 240 faces and 240 places were shown, where 120 faces and 120 places were famous. Each trial consisted of a pair of stimuli (S1 and S2), either two faces, or two places, shown for 1000 ms with an inter-stimulus interval (ISI) of 1000 ms during which only a central red fixation cross was present. The fixation cross was also superimposed on all stimuli to avoid saccades and facilitate central fixation. On the face trials, each pair consisted of either two familiar or two unfamiliar faces, while on the place trials each pair consisted of either two familiar or two unfamiliar places. The pairs of stimuli were either “Repeated,” where S2 depicted the same picture as S1, or “Unrepeated” where S2 was a different face/place to S1 (Figure 1).
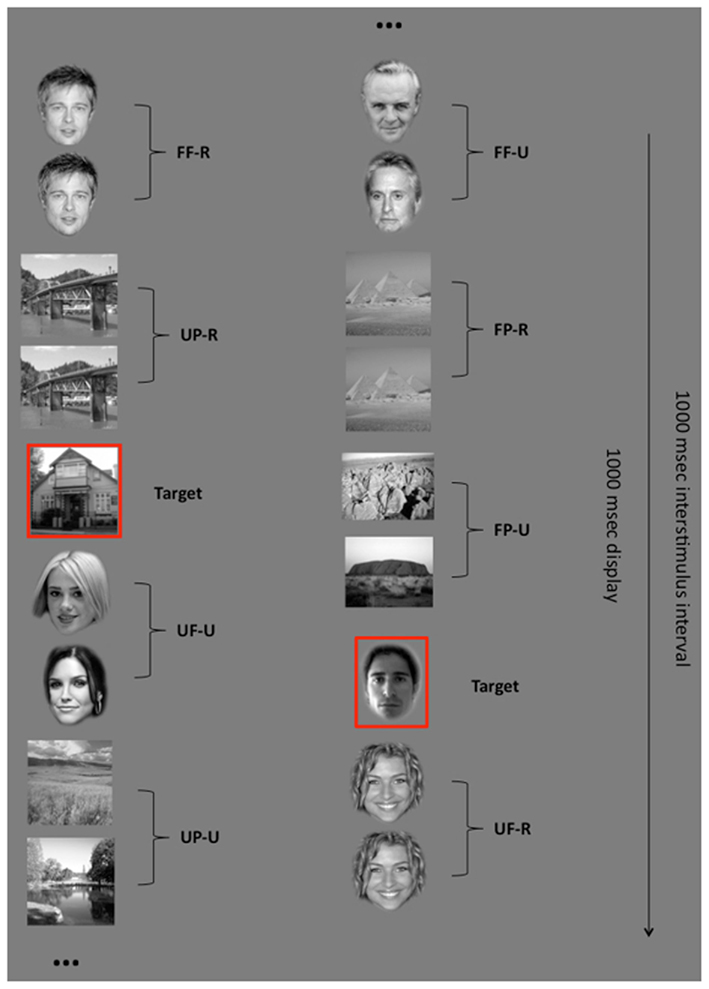
Figure 1. Experimental design. Participants had to press a button whenever they saw a previously selected target (either a face or a place). Stimuli were shown in pairs. Each pair consisted of faces or places. Both faces and places could be familiar or unfamiliar as well as repeated or unrepeated (FF-R: familiar face repeated; FF-U: familiar face unrepeated; UF-R: unfamiliar faces repeated; UF-U: unfamiliar face unrepeated; FP-R: familiar place repeated; FP-U: familiar place unrepeated; UP-R: unfamiliar place repeated; UP-U: unfamiliar place unrepeated). The superimposed red fixation cross that was present during the experiment is not indicated in this figure for clearer viewing of the stimuli.
Participants were not informed that they were viewing pairs of stimuli but were instructed to fixate centrally and press a button whenever they saw one of the two previously specified target stimuli (one face and one place). Each of the two target stimuli was shown 48 times during the task. The task was divided into 8 blocks of 120 trials, each including the presentation of 12 targets, for a total of 960 trials (half face pairs and half place pairs). All stimuli were shown in the center of a screen (size: 38 cm × 35 cm; resolution: 800 × 600 pixels) installed inside the magnetically shielded room, and placed at a distance of ∼40 cm from the participant’s head. The MEG experiment was programmed and delivered with Presentation software (Neurobehavioral Systems, Albany, CA, USA).
Stimuli
Familiar faces were downloaded from the internet and included famous actors, politicians, and athletes. Unfamiliar faces were also downloaded from the internet to closely match the familiar faces in term of sex, age, and approximate level of attractiveness. These unfamiliar faces belonged to the same categories of familiar faces but come from a different country (e.g., Italy), and were thus not familiar to the Australian population. Familiar places included famous landscapes or famous buildings. Unfamiliar places were matched to the familiar places for category and visual similarity (for example the Eiffel Tower was matched with an unfamiliar tower). One additional face and one additional place were used as target stimuli. All stimuli were converted to grayscale using Adobe Photoshop software (Adobe Systems Incorporated). Places were presented within a 7.5 cm × 5 cm frame. Faces were edited so that the internal facial configuration (but not hair) fitted into a 6 cm × 4 cm oval template. On average, places covered a visual angle of 10.7° × 7.2°, whereas faces subtended 5.7° × 8.6°. The mean luminance of the places and faces did not differ [t(238) = −1.82, p = 0.070].
MEG data acquisition
A 160-channel whole-head first-order axial gradiometer system (50 mm baseline; sampling rate: 1000 Hz) was used to record MEG activity. A digital head-shape was recorded for each participant before entering the magnetically shielded room. Five head position indicators (HPI) coils were attached to a tightly fitting elastic cap, and the 3D locations of three cardinal landmarks (the nasion and bilateral preauricular points), as well as ∼400 randomly selected points on the participant’s head surface, were digitized using a Fastrak system (Polhemus, Colchester, VT, USA). This allowed subsequent registration of the MEG data to the structural MRI. To correct for movement errors, the participants’ head position within the MEG system was determined at the start of each recording block from the five HPI coils.
MEG data processing
The minimum-norm estimate (MNE) was used for the estimation of the source current distribution at each cortical location (Hamalainen and Sarvas, 1989). The cortical surfaces were reconstructed from MRI of each participant using FreeSurfer software (Fischl et al., 1999). The MEG source space was constrained to a cortical surface that comprised 4098 sources per hemisphere with an average of 7 mm spacing between adjacent source locations. MEG signals were segmented into time epochs spanning from 200 ms before stimulus onset to 800 ms following stimulus onset, with the pre-stimulus epoch of −200 to 0 ms as baseline. Movement less than 5 mm was tolerated and noisy MEG channels (individuated offline in the raw data) were excluded in the analysis. Event-related magnetic fields were digitally filtered (50 Hz high-pass filter). MEG data associated with the target stimuli were ignored in the analysis to avoid motor artifact from responses. Automated filtering excluded neuromagnetic activity caused by eye blinks and gross eye movement artifacts.
The single-layer boundary element method (BEM; Hamalainen and Ilmoniemi, 1994) was implemented to calculate forward solutions from estimated source configurations. The noise-covariance matrix, computed from the 200-ms pre-stimulus activity, and the forward solution were together used to create a linear inverse operator (Dale et al., 2000a). At each cortical location, the current estimate was normalized to the estimated baseline variance, resulting in z-scores. This noise-normalized solution provides a dynamic statistical parametric map (dSPM), which indicates the signal-to-noise ratio (SNR) of the current estimate at each cortical location as a function of time (Dale et al., 2000b). Thus MNE enables to investigate the MEG activity directly on the inflated cortical surface of each subject.
MRI data acquisition
A 3D-magnetization prepared rapid gradient echo (MPRAGE) sequence was adopted to acquire high-resolution anatomical MRI scans for each participant. Scanning was performed with a 3 Tesla Philips Scanner at St Vincent’s Hospital, Sydney, Australia. An expert neuroradiologist, naïve to the aims of the project, evaluated the structural MRIs for both controls and patients and noted no abnormalities.
Regions of interest selection
We utilized an a priori approach to define regions of interest (ROIs). Firstly, due to converging evidence positing for its dominant role in face processing (Sergent et al., 1992; Barton et al., 2002; Barton, 2008; Fox et al., 2009; Eimer et al., 2010) and similar to previous investigations (Pitcher et al., 2011) we focused our analysis on the right hemisphere only. Secondly, our investigation focused on the right lateral occipital cortex (rLO) and the right fusiform gyrus (rFG), because, within the right hemisphere, these two regions are critically involved in face recognition (Kanwisher et al., 1997; Haxby et al., 2000; Rotshtein et al., 2005; Rossion, 2008). Thus, based on the assumption of two differential neural generators for the M170, we explored whether these reflect the processing of different types of information.
The main reason why we adopted an a priori approach for ROIs selection on inflated brain surfaces was to include data from all participants in the analysis. It is common in neuroimaging investigations that not all the ROIs under investigation are significant in all subjects when using the classic “statistical-threshold” approach. This issue is especially relevant when considering CPs who, as suggested by previous research (see Table A1 in Appendix), show a big heterogeneity in face-specific (M170) activity. This scenario in which not all participants are included in the analysis can negatively affect the power of the study. Using an anatomical selection of our ROIs ensures data from all locations and from all participants is included, thus maximizing the power and inference of our investigation.
For each participant, the rLO and rFG were selected by manually drawing a mask on the inflated brain surfaces reconstructed from individual structural MRIs. In particular, rLO was selected by drawing the mask within the lateral surface of the right occipital lobe. The rFG was selected by drawing the mask on the ventral surface of the temporal lobe, including the FG (Figure 2). On average, masks had a mean area of 1066 mm2 (SD = 86.05). MEG activity within rLO and rFG were then averaged between participants within each of the two groups4
Picture Recognition Task and Name Familiarity Task
Following the MEG experiment, we assessed the ability of each participant to recognize the famous faces and places that were shown on the Target detection task. The aim was to confirm: (i) whether CPs were (as hypothesized) in fact significantly worse than controls at recognizing famous faces; and (ii) whether the CPs’ recognition impairment was face specific, and not a more general deficit involving both face and place recognition.
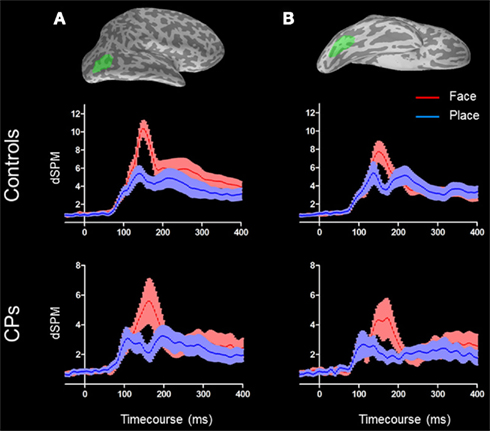
Figure 2. Right lateral occipital area [(A) top] and rFG [(B) top] are shown in green on an inflated surface of a subject. M170 activity for both controls [(A,B) middle] and CPs [(A,B) bottom] are represented. Face activity (red) is higher than place activity (blue) in both groups and in both ROIs. For both conditions, the shades around the mean activity represent the SEM.
In the “Picture recognition” task participants were instructed to type the name and/or specific semantic information about the person or place (a general description like “He is an actor” or “This tower is somewhere in Europe” was considered incorrect). The first block (Face recognition block) consisted of the 120 famous faces from the MEG experiment, the second block (Place recognition block) the 120 famous places. The task was programmed using SuperLab (Cedrus Corporation, 2007), and administered on a 15′′ Macintosh Power Book G4. In the “Name familiarity” task participants were asked whether they were familiar with the names of the 120 famous faces (Face block) and the names of the 120 famous places (Place block). Names belonging to faces/places that were not familiar to participants were excluded from further analysis. Names were presented on a Microsoft Excel spreadsheet.
Correlating Behavioral Performance and MEG
The correlation between brain activity and behavioral performance represents a very important and sensitive procedure for neuroimaging investigations (Rotshtein et al., 2007). To understand the role the M170 plays in face processing, we investigated the correlation between the amplitude of the M170 face-selectivity effect in the rLO and the rFG, and the inversion effect in the spacing and feature conditions of the Jane task.
Results
Behavioral Diagnostic Assessment Session
Non-face processing assessment
All CPs displayed normal contrast sensitivity as assessed by the FACT and normal color perception with the Ishihara Test for Color Blindness. Performance on the length, size, orientation and picture naming (long version) subtests of the BORB confirmed that basic object recognition skills were intact. The Raven Colored Progressive Matrices further indicated that the IQ of all participants with CP was within the normal range. None of the CPs scored within the autistic range on the Autism-Spectrum Quotient. As such, the everyday face recognition difficulties reported by the CPs (and confirmed on the two tests of face-memory reported below) are not due to general visual recognition difficulties, low IQ, or impaired social functioning.
Face processing assessment
MACCS famous face test 2008. For each participant with CP, the percentage of correctly recognized faces of known famous people was calculated, and then transformed to an age-adjusted z-score (using age-based norms reported in Bowles et al., 2009). The CPs scored between −4.05 and −2.04 below Australian norms (see Table 1). This suggests an impairment in familiar face recognition.
Cambridge face memory test. Total scores on the upright CFMT of CPs were transformed to age-adjusted z-scores (using age-based norms reported in Bowles et al., 2009), with the CPs scoring between −2.83 and −1.81 below the Australian norms (see Table 1). These results suggest an impairment in the processing of unfamiliar faces.
Holistic/configural processing (Jane task). To provide a normative dataset for comparison, 55 people without face recognition difficulties (38 female, Mean age = 25 years, range: 19–62 years, SD = 8.9) completed the Jane task. For both the spacing and feature sets, upright face processing in controls was more accurate than inverted face processing [F(1, 54) = 144, p < 0.001]. In agreement with previous studies, the face-inversion effect (as expressed in % of accuracy reduction) was greater for spacing (M = 20%, SD = 14.10) than features [M = 6.7%, SD = 11.06; F(2, 108) = 18.43, p < 0.001], suggesting that the spacing set of the Jane task represents a measure of holistic/configural processing (see Appendix for a complete analysis of the Jane task in controls).
As there were no sex differences (similar to Le Grand et al., 2006), and performance did not decline with age, we used the whole control sample as a reference for our participants with CP. For CPs, inversion scores on the spacing and feature sets of the Jane task were transformed to z-scores, with the prosopagnosics scoring between −2.05 and 0.57 on the spacing set and from −0.33 to 2.70 on the feature set (Table 1). Such variability on performance of tests of spacing and features in CP is consistent with previous findings (Le Grand et al., 2006; Schmalzl et al., 2008; Lee et al., 2010)5
Results: Magnetoencephalography Session
MEG results – M170
Behavioral data showed that performance on the Target detection task did not differ between controls (M = 96.03%, SD = 3.12) and CPs (M = 94.96%, SD = 2.41; Mann–Whitney, p = 0.311), suggesting that all participants were paying attention to the task (Figure 3). This was consistent with expectations, as CPs can typically perform easy discrimination tasks. However, we do not know whether CPs were identifying the target face in the same way as controls – as they might rely on a different strategy such as focusing on low-level characteristics of the target face.
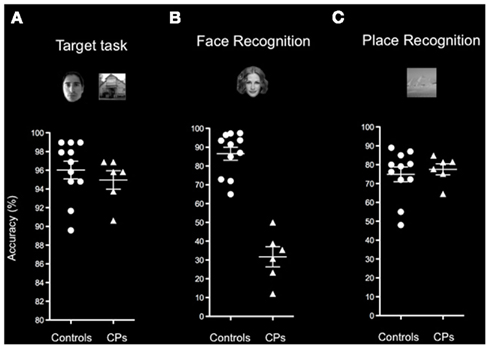
Figure 3. Behavioral performance of healthy controls and participants with CP on the (A) Target detection task, (B) Face recognition block, and (C) Place recognition block of the Picture recognition task. Indicated are means and SEM. Results show that CPs (triangles) and controls (circles) show similar accuracy on the Target detection task and on the Place block of the Picture recognition task. The two groups differ in their ability to recognize familiar faces as shown on the Face block of the Picture recognition task.
The analysis of MEG data focused on the M170. For each participant, the M170 was computed by averaging MEG amplitude on the 24-ms around the biggest peak recorded between 130 and 180 ms post-stimulus onset generated by S2. We performed a three factor (Category: face, place; Familiarity: familiar, unfamiliar; Repetition: repeated, unrepeated) repeated measures ANOVA for each ROI and for each group separately (for total of four ANOVAs). We did not run a single ANOVA for each ROI, considering the “group” as the between factor, because we did not want to inflate Type I error by considering unequal group size (11 controls versus 6 CPs). Instead, we first determined the presence/absence of a face specific M170 within both ROIs and both groups (by running four separate ANOVAs), and second, compared the face-selectivity of the M170 between controls and CPs by using a non-parametric test (Mann–Whitney).
In the rLO, controls showed a category effect for faces (M = 10.36 dSPM, SEM = 0.83) generating greater activity than for places [M = 4.87 dSPM, SEM = 0.70; F(1, 10) = 60.41, p < 0.001], and a repetition effect, with unrepeated stimuli (M = 7.75 dSPM, SEM = 0.69) showing greater MEG activity than repeated stimuli [M = 7.45 dSPM, SEM = 0.67; F(1, 10) = 5.96, p = 0.035]. No other main effects or interactions were statistically significant (all ps > 0.05). In the rFG, controls showed a category effect for faces (M = 8.16 dSPM, SEM = 1.06) generating greater activity than for places [M = 5.10 dSPM, SEM = 0.94; F(1, 10) = 6.88, p = 0.026]. No other main effects or interactions were statistically significant (all ps > 0.05; Figure 2).
The group of CPs also showed a category effect within the rLO, with faces (M = 6.32 dSPM, SEM = 1.35) generating greater activity than places [M = 2.62 dSPM, SEM = 0.57; F(1, 5) = 14.03, p = 0.013]. The same category effect (i.e., activity greater for faces than places) was found within rFG [Face: M = 3.05 dSPM, SEM = 1.16; Place: M = 2.74 dSPM, SEM = 1.08; F(1, 5) = 6.58, p = 0.05]. For both rLO and rFG no other main effects or interactions were statistically significant (all ps > 0.05).
Both controls and CPs showed neural activity that was greater for faces than places in both the rLO and rFG. In line with previous investigations on the M170 in CP (Bentin et al., 1999; Harris et al., 2005), we calculated the “Face-selectivity effect” as a measure of face distinctiveness of the M170. The Face-selectivity effect refers to the difference between the average MEG activity generated for faces and the average MEG activity generated for places. It is an indication of the face specificity of the M170 that takes into account differences in the baseline activity potentially due to a different distance from the sensors of the two groups. The determination of the face-selectivity effect, which represents a group × category interaction (Nieuwenhuis et al., 2011), is important, since just the finding of an M170 within CPs may not be sufficient to demonstrate differences between groups. CPs may show an M170 for faces, but this might have a smaller amplitude than in controls. This was not the case in these data. A Mann–Whitney test showed that the face-selectivity of the M170 did not differ between controls and CPs, both when recorded within the rLO (M ± SEM: Controls = 5.48 dSPM ± 0.70; CPs: 3.70 dSPM ± 0.99; p = 0.159) and rFG (Controls: M dSPM = 3.05, SEM = 1.16; CPs: M = 2.74 dSPM, SEM = 1.08; p = 0.688; Figure 4).
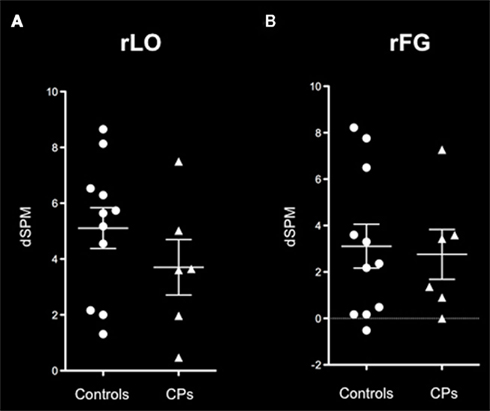
Figure 4. M170 “face-selectivity” effects within (A) rLO and (B) rFG show no difference between healthy controls (circles) and individuals with CP (triangles). On each graph the mean and the SEM are represented.
In addition, given that controls only showed a repetition effect within the rLO, we calculated the group × repetition interaction to understand whether the two groups differed in the magnitude of their repetition effect within the rLO. Results showed that the effect of repetition (i.e., unrepeated – repeated stimuli) did not differ between controls (Mean = 0.29; SEM = 0.12) and CPs (Mean = 0.05; SEM = 0.17), p = 0.269. In summary, the group of individuals with CP show a normal M170.
MEG results – M100
Previous research in clinical populations (i.e., Doniger et al., 2002) has shown normal electrophysiological activity at around 170 ms post-stimulus interval, despite abnormal activity of earlier components such as the one peaking at around 100 ms post-stimulus onset. To ascertain that this was not the case in our study, we investigated the features of the M100 (considered as an interval of 12 ms around the peak activity between 80 and 120 ms post-stimulus onset generated by S2) in controls as well as CPs. For both the rLO and rFG, we performed the same analysis performed for the M170.
Analysis of the M100 generating within rLO showed that, in controls, there were no statistically significant main effects or interactions (all ps > 0.05), indicating that the M100 recorded within the rLO reflects early a specific visual activity. CPs, on the other side, reported a main effect of Familiarity [F(1, 5) = 8.60, p = 0.033], with familiar stimuli (Mean: 3.66 dSPM, SEM = 0.68) showing bigger activity than unfamiliar stimuli (Mean: 3.41 dSPM, SEM = 0.67). Importantly however, when we investigated whether the difference between familiar and unfamiliar stimuli in CPs (Mean = 0.25 dSPM, SEM = 0.09) was bigger than in controls (Mean = −0.04 dSPM, SEM = 0.14) using a Mann–Whitney test, we did not find any statistical significant difference (p = 0.269). This last comparison, which constitutes a group × familiarity interaction (Nieuwenhuis et al., 2011), indicates that the two groups show, overall, similar M100 activity within the rLO. The same analysis was performed for the M100 generating within the rFG and revealed no statistically significant main effects or interactions in both groups (all ps > 0.05). Altogether, analysis of the M100 suggests that this component has similar features in the two groups.
Picture Recognition Task and Name Familiarity Task
Control subjects were familiar with 90.10% (SD = 8.40) of the famous individuals, and recognized 86.54% (SD = 11.51) of these faces, whereas CPs were familiar with 87.36% (SD = 8.54) of the famous individuals, but recognized only 31.70% (SD = 13.10) of them. There was a statistically significant difference (Mann–Whitney, p = 0.001) between the recognition accuracy of control subjects and CPs, further confirming the difficulties people with CP have in face recognition (Figure 2B).
Control subjects were familiar with 78.48% (SD = 12.52) of famous places, and recognized 74.90% (SD = 12.88) of them, whereas people with CP were familiar with 82.22% (SD = 16.84) of the famous places, and recognized 77.53% (SD = 7.18) of them. Controls and CPs did not differ in their ability to identify famous places (Mann–Whitney, p = 0.920; Figure 3C).
Overall, these results confirmed that: (i) CPs were significantly poorer than controls in famous face identification; and (ii) the recognition impairment of CPs was specific to faces6
Correlation between MEG activity and behavior in CP
Since CPs show variability in holistic/configural and featural processing (see Table 1), they represent an ideal population with which to investigate the neural correlates of these two types of processing. Given the small sample size we performed a non-parametric correlation. Results showed a correlation between the face-selectivity effect of the M170 within rLO (rLO-M170) and inversion effect z-scores on the “spacing” set of the Jane task (Spearman correlation = 0.867; p = 0.015), indicating a relation between holistic/configural processing and MEG activity in the lateral occipital cortex. There was also a correlation between the face-selectivity effect of the M170 recorded within rFG (rFG-M170) and inversion effect (z-scores) on the “feature” set of the Jane task (Spearman correlation = −0.828; p = 0.022; see Figure 5), indicating a relationship between feature processing and MEG activity in the lateral occipital cortex. Both statistically significant correlations indicated a similar pattern: the more the M170 was face-sensitive, the more behavioral performance approached normal (typical) values. We also correlated face memory with MEG activity. Results showed an absence correlation between face memory (for both familiar and unfamiliar faces) and MEG activity recorded within either rLO or rFG. Even though the lack of relation between M170 and face memory is in agreement with Harris et al. (2005), it could however be the consequence of the small variability shown by CPs on the CFMT and the MFFT-08 and/or of the small number of CPs (see Table A2 in Appendix). Overall, these results indicate that the M170 in CP is primary involved in the coding of holistic/configural and featural processing, but not for face-memory skills.
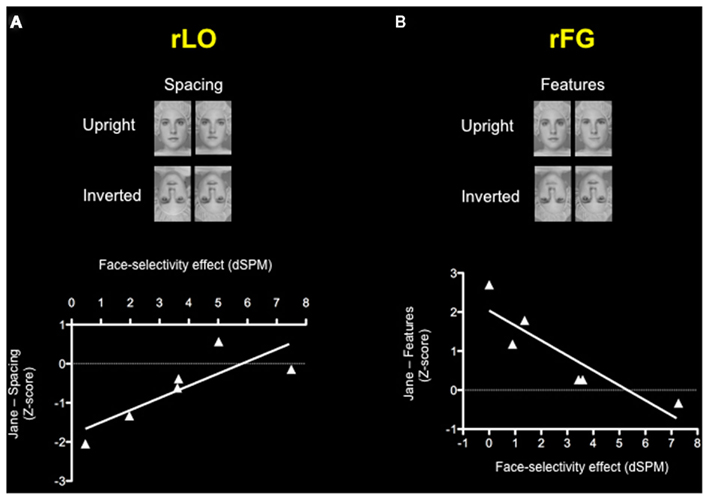
Figure 5. Correlations between the face-selectivity of the M170 in CP and the face-inversion effect (z-scores) on the Jane task. Each triangle represents one participant with CP. Results show (A) a positive correlation between the face-selectivity of the M170 and inversion effect on the spacing subset of the Jane task within rLO and (B) negative correlation between the face-selectivity of the M170 and inversion effect on the features subset of the Jane task within rFG, indicating how the M170 codes different processing according to the locus of its origin.
General Discussion
People with CP fail to acquire typical face processing skills in the absence of any brain damage and in the context of normal exposure to faces throughout their lives (Behrmann and Avidan, 2005; Duchaine and Nakayama, 2006b). The results of our study illustrate however that despite their behavioral impairment in face recognition, CPs can show typical M170 responses to faces that originate from two differential neural sources. Furthermore, we were able to use the individual variation in the CPs’ performance on tasks of configural and featural processing to explore the role of the M170 in detail. Specifically, by correlating the M170 and the behavioral performance of our group of CPs, we showed that the M170 generating within the lateral occipital cortex (rLO-M170) is primarily involved in the coding of holistic/configural processing, whereas the M170 generating within the rFG (rFG-M170) is primarily involved in featural processing. This is consistent with previous evidence describing two anatomical generators (i.e., the inferior lateral occipital lobe and the FG) for the M170 (Linkenkaer-Hansen et al., 1998; Watanabe et al., 2003; Deffke et al., 2007; Itier et al., 2007). Moreover, our results revealed that, at least in our group of participants, the face-selectivity of the M170 of CPs did not differ from that of controls. These findings indicate that neuromagnetic activity generated within rLO and rFG at around 170 ms post-stimulus onset can be face selective and of normal magnitude even in people who have never developed normal face processing skills. Thus, an abnormal M170 does not seem to represent a necessary neurophysiological correlate of CP.
Next we would like to briefly discuss our results in relation to familiarity and repetition effects. The lack of familiarity effects for face stimuli in both CPs and controls and is in line with previous findings indicating that familiarity effects tend to occur either at earlier (e.g., 100 ms) or later (e.g., 400 ms) latencies than the M170 (George et al., 1997; Seeck et al., 1997; Eimer, 2000a; Harris and Aguirre, 2008; Rivolta et al., 2012b). In terms of repetition effects, we found differential effects for the rLO-M170 and the rFG-M170. Controls showed a significantly reduced amplitude of the rLO-M170 for repeated compared to unrepeated stimuli (independent of their familiarity). It is possible that this may reflect a general adaptation phenomenon potentially driven by low-level features of the stimuli. Unlike controls, CPs did not show a general repetition effect of the rLO-M170. While the reason for this remains unclear, it is possible that it might simply reflect the reduced statistical power due to the reduced size of the CP group (six CPs compared to 11 controls)7
As for the rFG-M170, neither controls nor CPs showed a repetition effect. A speculative explanation for this could be that neural activity within rFG may be mediated by repetition only after ∼250 ms post-stimulus onset. In line with this hypothesis, previous research has described interactions between stimulus repetition and familiarity occurring at such latency, indicating that the specific identity of visually presented faces is accessed after the M170 (Schweinberger et al., 2002). Future research will be needed to clarify whether such later MEG components (i.e., the M250 or M400) that are sensitive to the familiarity of face stimuli (Eimer, 2000a; Schweinberger et al., 2002; Harris and Aguirre, 2008) may show abnormal features in CP.
One of the fundamental goals of neuroimaging is to establish the relationship between neural activity and behavior. In fact, much information about neural coding can be missed if its relation to behavioral performance is not taken into account (Rotshtein et al., 2007). The current study sheds light on the coupling between brain activity and behavioral performance by correlating face perception performance with MEG component amplitude. The most important result of our study in this regard is the finding that the two neural generators of the M170 seem to underlie two different aspects of face processing: The rLO-M170 seems to be primarily involved in holistic/configural processing, whereas the rFG-M170 seems to be primarily involved in feature processing. Hence, not only does the M170 generate from two separable neural sources, but the two generators actually seem to code for different aspects of face processing. Our results confirm previous MEG findings showing the engagement of the M170 in both holistic/configural and feature processing (Harris and Aguirre, 2008). However, the improved spatial resolution in our study due to the coregistration of MEG data with structural MRIs, allowed us to pinpoint the differential role played by the rLO and the rFG in this context. In line with our findings, recent ERP work (Zheng et al., 2011) demonstrated that the N170 is sensitive to different face characteristics such as eye color, eye width, facial layout, and face width, supporting the multiple source account of the N/M170 (Linkenkaer-Hansen et al., 1998; Watanabe et al., 2003). These results are also in agreement with findings in monkeys showing that that holistic/configural and feature processing are mediated by the activity of different neural populations (Freiwald et al., 2009). However, a direct comparison between the findings of studies on primates and humans is rendered difficult by the lack of a clear correspondence between the posterior, middle, and anterior face patches on the monkey brain assessed via single cell recordings, and human face regions assessed with MEG or ERP.
Previous research using fMRI has also investigated the neural correlates of holistic/configural versus feature processing in humans. Some authors suggest that the lateral occipital lobe, and in particular the occipital face area (OFA; Gauthier et al., 2000), is mainly engaged by feature processing (Yovel and Kanwisher, 2004; Liu et al., 2009), whereas others suggest this region is involved in both holistic/configural and feature processing (Schiltz and Rossion, 2006; Rotshtein et al., 2007; Harris and Aguirre, 2008; Rossion et al., 2011). In any case, there is general consensus on the role played by the FG, and in particular the fusiform face area (FFA; Kanwisher et al., 1997), in both holistic/configural and feature processing (Yovel and Kanwisher, 2004; Schiltz and Rossion, 2006; Rotshtein et al., 2007; Harris and Aguirre, 2008).
Our current results are at least partially inconsistent with these past fMRI findings. However, of course one needs to keep in mind that MEG and fMRI measure neural signals of a different nature, which can be a confounding factor when directly comparing the data obtained with either method (Singh, 2006; Logothetis, 2008). Moreover, we need to underline the fact that our correlation analysis was limited to our CP sample, since our control group was not assessed with the complete face processing battery including configural and featural processing tasks. Hence, further research will be needed to clarify whether the differential properties of the rLO-M170 and the rFG-M170 are a specific characteristic of CP, or also evident in individuals without face processing difficulties.
Finally, we wish to stress that in the context of our current study we deliberately refer to the more general terms of rLO and rFG, as opposed to right OFA and right FFA. We believe that given the size and location of our ROIs, in addition to the fact that we found very reliable face specific M170 responses in both CPs and controls, we can confidently conclude that that our results are largely driven by the activity of face specific areas. However, we cannot claim that the observed activity was specifically mediated by the OFA or FFA.
Conclusion
In sum, the face-sensitive M170 signal generating within both the rLO and the rFG seem to be typical in people with CP. In addition, by taking the behavioral variability of our CP sample into account, we found that the rLO and the rFG seem to have different functional characteristics: The rLO seems to be primarily involved in holistic/configural processing, whereas the rFG seems to be primarily involved in featural processing. Further studies will be needed to clarify whether this differential involvement of the rLO and rFG represents a general feature of the human face processing network, or one that distinguishes people with face recognition difficulties.
Conflict of Interest Statement
The authors declare that the research was conducted in the absence of any commercial or financial relationships that could be construed as a potential conflict of interest.
Acknowledgments
We wish to thank members of the Kanwisher Lab, Anina Rich, Rachel Robbins, and Patrik Vuilleumier for their insightful comments on this work. We wish to also thank Loes Koelewijn, Melanie Reid, Christopher Sewell, and Graciela Tesan, for the support provided in the MEG data acquisition and processing, and C. Ellie Wilson for the support given in participants’ recruitment. Stimuli for the Jane task were provided by Daphne Maurer (McMaster Visual Development Lab). This work was supported by the Macquarie University Research Excellence Scholarship (iMQRES) to Davide Rivolta. Mark A. Williams is supported by the Australian Research Council Fellowship Schemes (DP0984919). Laura Schmalzl is supported by the European Research Council. We wish to thank the Max Planck Society for supporting the publication costs.
Footnotes
- ^Even though there is no report of evident neurological damage in CPs, recent studies underlined that reduced connectivity between posterior and anterior face regions and reduced volume of the anterior temporal lobe may represent the potential anatomical underpinning of the condition (Behrmann et al., 2007; Thomas et al., 2009).
- ^A contour condition was also included, in which the internal portion of the original face was combined with the outer contour of a different face (data not reported here).
- ^McKone and Yovel (2009) suggest that the dissociation between the two sets may be consequence of the characteristics of the “features set,” where stimuli change not only in the shape, but also in the brightness of their features. However, equivalent face-inversion effects have recently been demonstrated for shape only versus shape and brightness changes on the “feature set” of the Jane task (Mondloch et al., 2010). Thus, in the current investigation the magnitude of the inversion effect on the spacing set was considered an index of primarily holistic/configural processing whereas the magnitude of the inversion effect on the feature set was considered an index of primarily feature processing.
- ^There were no differences between the sizes of the two ROIs within or between groups.
- ^Yovel and Duchaine (2006) however showed that eight CPs were, as a group, impaired both on the spacing and feature processing when assessed with a different task (i.e. “The Albert task”).
- ^Note however that CPs can show variability in the face-specificity of their recognition problems (Duchaine et al., 2007; Wilson et al., 2010).
- ^In order to investigate whether the lack of repetition effect in CPs might be simply due to lack of power, we performed the same analysis on the six best age matched controls. We found no significant main effect of repetition [F(1, 5) = 1.56, p = 0.267], suggesting that the lack of repetition effect in our CP group might indeed be due to a lack of power.
References
Alonso Pietro, E., Caharel, S., Henson, R. N., and Rossion, B. (2011). Early (N170/M170) face-sensitivity despite right lateral occipital brain damage in acquired prosopagnosia. Front. Hum. Neurosci. 5:138. doi:10.3389/fnhum.2011.00138
Baron-Cohen, S., Wheelwright, S., Skinner, R., Martin, J., and Clubley, E. (2001). The autism-spectrum quotient (AQ): evidence from Asperger syndrome/high-functioning autism, males and females, scientists and mathematicians. J. Autism Dev. Disord. 31, 5–17.
Barton, J. J. (2008). Structure and function in acquired prosopagnosia: lessons from a series of 10 patients with brain damage. J. Neuropsychol. 2, 197–225.
Barton, J. J., Press, D. Z., Keenan, J. P., and O’Connor, M. (2002). Lesions of the fusiform face area impair perception of facial configuration in prosopagnosia. Neurology 58, 71–78.
Behrmann, M., and Avidan, G. (2005). Congenital prosopagnosia: face-blind from birth. Trends Cogn. Neurosci. (Regul. Ed.) 9, 180–187.
Behrmann, M., Avidan, G., Gao, F., and Black, S. (2007). Structural imaging reveals anatomical alterations in inferotemporal cortex in congenital prosopagnosia. Cereb. Cortex 17, 2354–2363.
Bentin, S., DeGutis, J. M., D’Esposito, M., and Robertson, L. C. (2007). Too many trees to see the forest: performance, event-related potential, and functional magnetic resonance imaging manifestations of integrative congenital prosopagnosia. J. Cogn. Neurosci. 19, 132–146.
Bentin, S., Deouell, L. Y., and Soroker, N. (1999). Selective visual streaming in face recognition: evidence from developmental prosopagnosia. Neuroreport 10, 823–827.
Bentin, S., McCarthy, G., Perez, E., Puce, A., and Allison, T. (1996). Electrophysiological studies of face perception in humans. J. Cogn. Neurosci. 8, 551–565.
Bobes, M. A., Lopera, F., Comas, L., Galan, L., Carbonell, F., Bringas, M., and Valdes-Sosa, M. (2004). Brain potentials reflect residual face processing in a case of prosopagnosia. Cogn. Neuropsychol. 21, 691–718.
Bowles, D. C., McKone, E., Dawel, A., Duchaine, B., Palermo, R., Schmalzl, L., Rivolta, D., Wilson, E. C., and Yovel, G. (2009). Diagnosing prosopagnosia: effects of aging, sex, and participant-stimulus ethnic match on the Cambridge Face Memory Test and Cambridge Face Perception Test. Cogn. Neuropsychol. 26, 423–455.
Dale, A. M., Fischl, B. R., and Sereno, M. I. (2000a). Cortical surface-based analysis: I. Segmentation and surface reconstruction. Neuroimage 9, 179–194.
Dale, A. M., Liu, A. K., Fischl, B. R., Buckner, R. L., Belliveau, J. W., Lewine, J. D., and Halgren, E. (2000b). Dynamic statistical parametric mapping: combining fMRI and MEG for high-resolution imaging of cortical activity. Neuron 26, 55–67.
Dalrymple, K., Oruc, I., Duchaine, B., Fox, C. J., Iaria, G., Handy, T. C., and Barton, J. S. J. (2011). The neuroanatomic basis of the face-selective N170 in acquired prosopagnosia, a combined ERP/fMRI study. Neuropsycholgia 49, 2553–2563.
De Renzi, E., Faglioni, P., Grossi, D., and Nichelli, P. (1991). Apperceptive and associative forms of prosopagnosia. Cortex 27, 213–221.
Deffke, I., Sander, T., Heidenreich, J., Sommer, W., Curio, G., Trahms, L., and Lueschow, A. (2007). MEG/EEG sources of the 170-ms response to faces are co-localized in the fusiform gyrus. Neuroimage 35, 1495–1501.
DeGutis, J. M., Bentin, S., Robertson, L. C., and D’Esposito, M. (2007). Functional plasticity in ventral temporal cortex following cognitive rehabilitation of a congenital prosopagnosic. J. Cogn. Neurosci. 19, 1790–1802.
Doniger, G. M., Foxe, J. J., Murray, M. M., Higgins, B. A., and Javitt, D. C. (2002). Impaired visual object recognition and dorsal/ventral stream interaction in schizophrenia. Arch. Gen. Psychiatry 59, 1011–1020.
Duchaine, B. (2000). Developmental prosopagnosia with normal configural processing. Neuroreport 11, 79–83.
Duchaine, B., and Nakayama, K. (2006a). The Cambridge Face Memory Test: results for neurologically intact individuals and an investigation of its validity using inverted face stimuli and prosopagnosic participants. Neuropsychologia 44, 576–585.
Duchaine, B., and Nakayama, K. (2006b). Developmental prosopagnosia: a window to content-specific face processing. Curr. Opin. Neurobiol. 16, 166–173.
Duchaine, B., Germine, L., and Nakayama, K. (2007). Family resemblance: ten family members with prosopagnosia and within-class object agnosia. Cogn. Neuropsychol. 24, 419–430.
Eimer, M. (2000a). Event-related brain potentials distinguish processing stages involved in face perception and recognition. Clin. Neurophysiol. 111, 694–705.
Eimer, M. (2000b). The face-specific N170 component reflects late stages in the structural encoding of faces. Cogn. Neurosci. 11, 2319–2324.
Eimer, M., Kiss, M., and Nicholas, S. (2010). Response profile of the face-sensitive N170 component: a rapid adaptation study. Cereb. Cortex 20, 2442–2452.
Fischl, B. R., Sereno, M. I., and Dale, A. M. (1999). Cortical surface-based analysis: II. Inflation, flattering, and surface-based coordinate system. Neuroimage 9, 125–207.
Fox, C. J., Iaria, G., and Barton, J. S. J. (2009). Defining the face processing network: optimization of the functional localizer in fMRI. Hum. Brain Mapp. 30, 1637–1651.
Freiwald, W. A., Tsao, D. Y., and Livingstone, M. S. (2009). A face feature space in the macaque temporal lobe. Nat. Neurosci. 12, 1187–1196.
Gauthier, I., Tarr, M. J., Moylan, J., Skudlarski, P., Gore, J. C., and Anderson, A. W. (2000). The fusiform “face area” is part of a network that processes faces at the individual level. J. Cogn. Neurosci. 12, 495–504.
George, N., Jemel, B., Fiori, J., and Renault, B. (1997). Race and shape repetition effects in humans: a spatio-temporal ERP study. Neuroreport 8, 1417–1423.
Hamalainen, M. S., and Ilmoniemi, R. J. (1994). Interpreting magnetic fields of the brain – minimum norm estimates. Med. Biol. Eng. Comput. 32, 35–42.
Hamalainen, M. S., and Sarvas, J. (1989). Realistic conductivity geometry model of the human head for interpretation of neuromagnetic data. IEEE Trans. Biomed. Eng. 36, 165–171.
Harris, A., and Aguirre, G. K. (2008). The effects of parts, wholes, and familiarity on face-selective responses in MEG. J. Vis. 8, 1–12.
Harris, A., Duchaine, B. C., and Nakayama, K. (2005). Normal and abnormal face selectivity of the M170 response in developmental prosopagnosics. Neuropsychologia 43, 2125–2136.
Harris, A., and Nakayama, K. (2008). Rapid adaptation of the M170 response: importance of face parts. Cereb. Cortex 18, 467–476.
Haxby, J. V., Hoffman, E. A., and Gobbini, M. I. (2000). The distributed human neural system for face perception. Trends Cogn. Sci. (Regul. Ed.) 4, 223–233.
Itier, R. J., Alain, C., Sedore, K., and McIntosh, A. (2007). Early face processing specificity: it’s in the eyes! J. Cogn. Neurosci. 19, 1815–1826.
Itier, R. J., Herdman, A. T., George, N., Cheyne, D., and Taylor, M. J. (2006). Inversion and contrast-reversal effects on face processing assessed by MEG. Brain Res. 1115, 108–120.
Kanwisher, N. (2010). Functional specificity in the human brain: a window into the functional architecture of the mind. Proc. Natl. Acad. Sci. U.S.A. 107, 11163–11170.
Kanwisher, N., McDermott, J., and Chun, M. M. (1997). The fusiform face area: a module in human extrastriate cortex specialized for face perception. J. Neurosci. 17, 4302–4311.
Le Grand, R., Cooper, P. A., Mondloch, C. J., Lewis, T. L., Sagiv, N., de Gelder, B., and Maurer, D. (2006). What aspects of face processing are impaired in developmental prosopagnosia? Brain Cogn. 61, 139–158.
Lee, Y., Duchaine, B. C., Wilson, H. R., and Nakayama, K. (2010). Three cases of developmental prosopagnosia from one family: detailed neuropsychological and psychophysical investigation of face processing. Cortex 46, 949–964.
Linkenkaer-Hansen, K., Palva, J. M., Sams, M., Hietanen, J. K., Aronen, H. J., and Ilmoniemi, R. J. (1998). Face-selective processing in human extrastriate cortex around 120 ms after stimulus onset revealed by magneto- and electroencephalography. Neurosci. Lett. 253, 147–150.
Liu, J., Harris, A., and Kanwisher, N. (2009). Perception of face parts and face configurations: an fMRI study. J. Cogn. Neurosci. 22, 203–211.
Liu, J., Higuchi, M., Marantz, A., and Kanwisher, N. (2000). The selectivity of the occipitotemporal M170 for faces. Cogn. Neurosci. Neuropsychol. 11, 337–341.
Maurer, D., Le Grand, R., and Mondloch, C. J. (2002). The many faces of configural processing. Trends Cogn. Sci. (Regul. Ed.) 6, 255–260.
Maurer, D., O’Craven, K. M., Le Grand, R., Mondloch, C. J., Springer, M. V., Lewis, T. L., and Grady, C. L. (2007). Neural correlates of processing facial identity based on features versus their spacing. Neuropsychologia 45, 1438–1451.
McKone, E. (2010). “Face and object recognition: how do they differ?” in Tutorials in Visual Cognition, ed. V. Coltheart (New York, NY: Psychology Press), 261–303.
McKone, E., and Yovel, G. (2009). Why does picture-plane inversion sometimes dissociate perception of features and spacing in faces, and sometimes not? Toward a new theory of holistic processing. Psychon. Bull. Rev. 16, 778–797.
Minnebusch, D. A., Suchan, B., Ramon, M., and Daum, I. (2007). Event-related potentials reflect heterogeneity of developmental prosopagnosia. Eur. J. Neurosci. 25, 2234–2247.
Mondloch, C., Le Grand, R., and Maurer, D. (2002). Configural face processing develops more slowly than featural processing. Perception 31, 553–566.
Mondloch, C., Robbins, R., and Maurer, D. (2010). Discrimination of facial features by adults, 10-year-olds, and cataract-reversal patients. Perception 39, 184–194.
Nieuwenhuis, S., Forstmann, B. U., and Wagenmakers, E.-J. (2011). Erroneous analyses of interactions in neuroscience: a problem of significance. Nat. Neurosci. 14, 1105–1107.
Palermo, R., Willis, M. L., Rivolta, D., McKone, E., Wilson, C. E., and Calder, A. J. (2011). Impaired holistic coding of facial expression and facial identity in congenital prosopagnosia. Neuropsychologia 49, 1226–1235.
Pitcher, D., Duchaine, B., Walsh, V., Yovel, G., and Kanwisher, N. (2011). The role of lateral occipital face and object areas in the face inversion effect. Neuropsycholgia 49, 3448–3453.
Pitcher, D., Walsh, V., Yovel, G., and Duchaine, B. (2007). TMS evidence for the involvement of the right occipital face area in early face processing. Curr. Biol. 17, 1568–1573.
Raven, J., Raven, J. C., and Court, J. H. (1998). Section 4: The Advanced Progressive Matrices Manual for Raven’s Progressive Matrices and Vocabulary Scales. San Antonio, TX: Harcourt Assessment.
Riddoch, M. J., and Humphreys, G. W. (1993). BORB: The Birmingham Object Recognition Battery. Hove, UK: Lawrence Erlbaum Associates.
Riddoch, M. J., Johnston, R. A., Bracewell, B. M., Boutsen, L., and Humphreys, G. W. (2008). Are faces special? A case of pure prosopagnosia. Cogn. Neuropsychol. 25, 3–26.
Rivolta, D., Schmalzl, L., Coltheart, M., and Palermo, R. (2010). Semantic information can facilitate covert face recognition in congenital prosopagnosia. J. Clin. Exp. Neuropsychol. 32, 1002–1016.
Rivolta, D., Palermo, R., Schmalzl, L., and Coltheart, M. (2012a). Covert face recognition in congenital prosopagnosia: a group study. Cortex 48, 344–352.
Rivolta, D., Palermo, R., Schmalzl, L., and Williams, M. A. (2012b). An early category-specific neural response for the perception of both places and faces. Cogn. Neurosci. 3, 45–51.
Robbins, R., and McKone, E. (2007). No face-like processing for objects-of-expertise in three behavioural tasks. Cognition 103, 331–336.
Rossion, B. (2008). Constraining the cortical face network by neuroimaging studies of acquired prosopagnosia. Neuroimage 40, 423–426.
Rossion, B., Dricot, L., Goebel, R., and Busigny, T. (2011). Holistic face categorization in higher order visual areas of the normal and prosopagnosic brain: toward a non-hierarchical view of face perception. Front. Hum. Neurosci. 4:225. doi:10.3389/fnhum.2010.00225
Rossion, B., Gauthier, I., Tarr, M. J., Despland, P., Bruyer, R., Linotte, S., Crommelinck, M., and Guerit, J. M. (2000). The N170 occipito-temporal component is delayed and enhanced to inverted faces but not to inverted objects: an electrophysiological account of face-specific processes in the human brain. Neuroreport 11, 69–74.
Rotshtein, P., Geng, J. J., Driver, J., and Dolan, R. (2007). Role of features and second-order relations in face discrimination, face recognition, and individual face skills: behavioural and fMRI data. J. Cogn. Neurosci. 19, 1435–1452.
Rotshtein, P., Henson, R. N. A., Treves, A., Driver, J., and Dolan, R. (2005). Morphing Marilyn into Maggie dissociates physical and identity face representations in the brain. Nat. Neurosci. 8, 107–113.
Schiltz, C., and Rossion, B. (2006). Faces are represented holistically in the human occipito-temporal cortex. Neuroimage 32, 1385–1394.
Schmalzl, L., Palermo, R., and Coltheart, M. (2008). Cognitive heterogeneity in genetically based prosopagnosia: a family study. J. Neuropsychol. 2, 99–117.
Schweinberger, S. R., Pickering, E. C., Jentzsch, I., Burton, A. M., and Kaufmann, J. M. (2002). Event-related brain potential evidence for a response of inferior temporal cortex to familiar face repetitions. Brain Res. Cogn. Brain Res. 14, 398–409.
Seeck, M., Michel, C. M., Mainwarning, N., Cosgrove, R., Blume, H., Ives, J., Landis, T., and Schomer, D. L. (1997). Evidence for rapid face recognition from human scalp and intracranial electrodes. Neuroreport 8, 2749–2754.
Sergent, J., Ohta, S., and macdonald, B. (1992). Functional neuroanatomy of face and object processing. Brain 115, 15–36.
Singh, K. D. (2006). “Magnetoencephalography,” in Methods in Mind, eds C. Senior, T. Russell, and M. Gazzaniga (Cambridge, MA: MIT Press), 291–326.
Tanaka, J. W., and Farah, M. J. (1993). Parts and wholes in face recognition. Q. J. Exp. Psychol. A 46, 225–245.
Thomas, C., Avidan, G., Kate, H., Kwan-jin, J., Gao, F., and Behrmann, M. (2009). Reduced structural connectivity in ventral visual cortex in congenital prosopagnosia. Nat. Neurosci. 12, 29–31.
Vision Sciences Research Corporation. (2002). Functional Acuity Contrast Test (FACT). Chicago, IL: Author.
Watanabe, S., Kakigi, R., and Puce, A. (2003). The spatiotemporal dynamics of the face inversion effect: a magneto- and electro-encephalographic study. Neuroscience 116, 879–895.
Wilson, C. E., Palermo, R., Schmalzl, L., and Brock, J. (2010). Specificity of impaired facial identity recognition in children with suspected developmental prosopagnosia. Cogn. Neuropsychol. 27, 30–45.
Young, A. W., Hellawell, D., and Hay, D. C. (1987). Configurational information in face perception. Perception 16, 747–759.
Yovel, G., and Kanwisher, N. (2004). Face perception: domain specific, not process specific. Neuron 44, 747–748.
Yovel, G., and Kanwisher, N. (2005). The neural basis of the behavioral face-inversion effect. Curr. Biol. 15, 2256–2262.
Yovel, G., and Duchaine, B. (2006). Specialized face perception mechanisms extract both part and spacing information: evidence from developmental prosopagnosia. J. Cogn. Neurosci. 18, 580–593.
Zheng, H., Mondloch, C. J., Nishimura, M., Vida, M. D., and Segalowitz, S. J. (2011). Telling one face from another: electrocortical correlates of facial characteristics among individual female faces. Neuropsychologia 49, 3254–3264.
Appendix
Methods
Jane task analysis (control subjects)
A 2 × 3 repeated measures ANOVA on accuracy, with orientation (upright, inverted) and set (spacing, features, contour) as within-subject factors, revealed an orientation effect [F(1, 54) = 144, p < 0.001], a set effect [F(2, 108) = 75.81, p < 0.001], and an orientation by set interaction [F(2, 108) = 18.43, p < 0.001]. In the upright condition a set of pairwise comparisons (Bonferroni corrected for multiple comparisons) showed that accuracy was greater on the feature set (M = 90% SD = 9.60) than on the spacing (M = 76.7% SD = 12.83; p < 0.001) and contour (M = 80%, SD = 10.30; p < 0.001) sets, whereas the spacing and contour conditions did not differ (p = 0.226).
In the inverted condition, accuracy on the feature set (M = 83.3%, SD = 11.50) was significantly higher than on the spacing (M = 60%, SD = 10.40; p < 0.001) and contour (M = 66.7%, SD = 10.40; p < 0.001) sets. Accuracy on the contour condition set was significantly higher than the spacing set (p = 0.002). Additional planned contrasts were calculated to investigate the effect of inversion on all the three sets. Results showed that the inversion effect of the feature set (M = 6.7%, SD = 11.06) was significantly smaller than the inversion effect on the spacing set (M = 20%, SD = 14.10; p < 0.001) and the contour set (M = 16.7%, SD = 12.93; p < 0.001). There was no difference between the inversion effect of the spacing and contour conditions (p < 0.085).
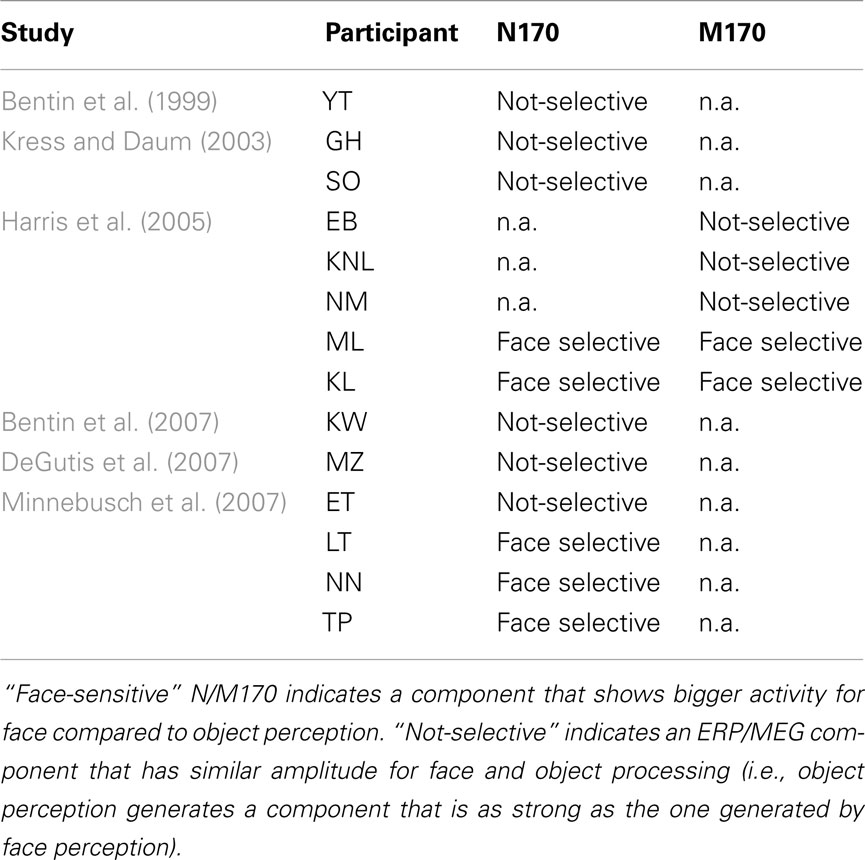
Table A1. Studies investigating the face-selectivity of the N170 and/or the M170 in different case studies of CP.
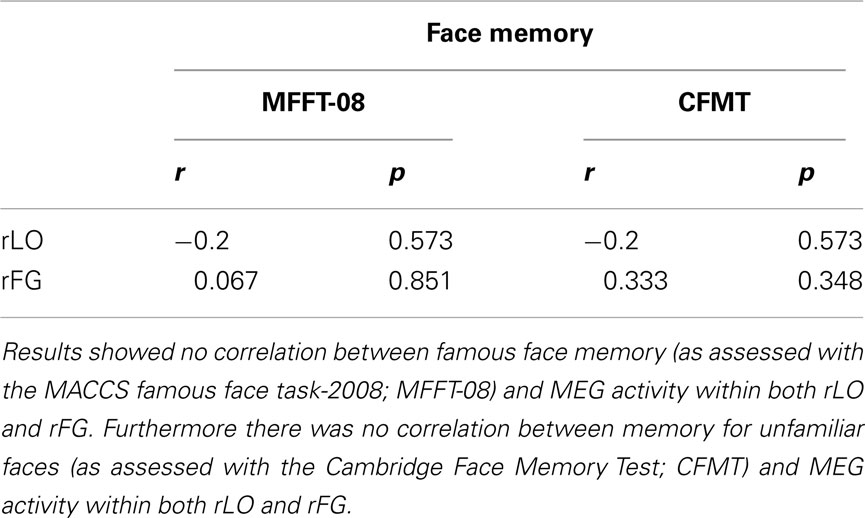
Table A2. Correlation between MEG activity (M170 face-selectivity) and performance on the face-memory tasks.
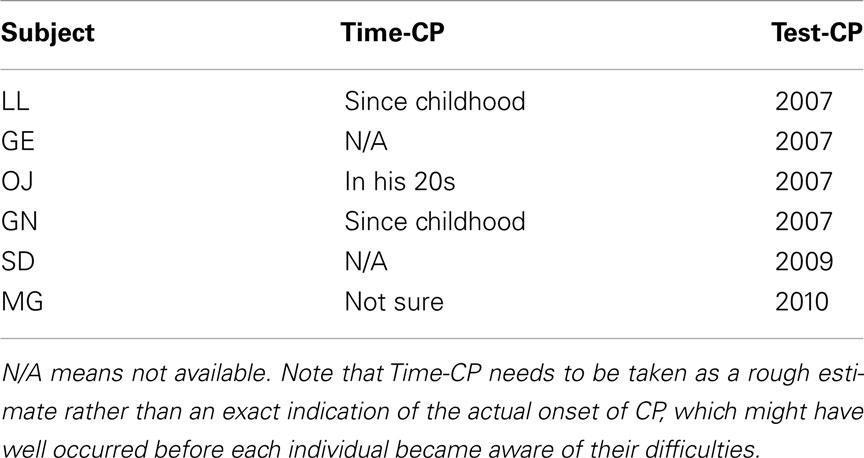
Table A3. Information regarding the time at which each of the CPs participating in our study first became aware of their face recognition difficulties (Time-CP), as well as the year they started their collaboration with us (Test-CP) – both taken from the MACCS prosopagnosia database.
Keywords: congenital prosopagnosia, face processing, M170, MEG, scene processing
Citation: Rivolta D, Palermo R, Schmalzl L and Williams MA (2012) Investigating the features of the M170 in congenital prosopagnosia. Front. Hum. Neurosci. 6:45. doi: 10.3389/fnhum.2012.00045
Received: 10 November 2011; Accepted: 22 February 2012;
Published online: 12 March 2012.
Edited by:
Hans-Jochen Heinze, University of Magdeburg, GermanyReviewed by:
Micah M. Murray, Université de Lausanne, SwitzerlandLeslie J. Carver, University of California, San Diego, USA
Copyright: © 2012 Rivolta, Palermo, Schmalzl and Williams. This is an open-access article distributed under the terms of the Creative Commons Attribution Non Commercial License, which permits non-commercial use, distribution, and reproduction in other forums, provided the original authors and source are credited.
*Correspondence: Davide Rivolta, Department of Neurophysiology, Max Planck Institute for Brain Research, Deutschordenstraße, 46, Frankfurt am Main, Germany. e-mail:ZGF2aWRlLnJpdm9sdGFAYnJhaW4ubXBnLmRl