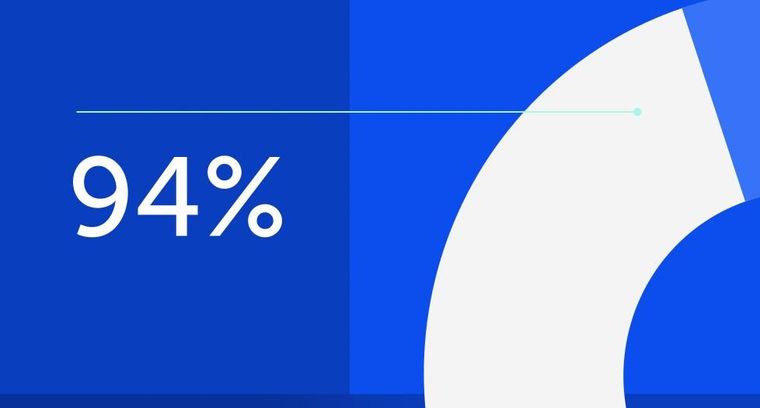
94% of researchers rate our articles as excellent or good
Learn more about the work of our research integrity team to safeguard the quality of each article we publish.
Find out more
ORIGINAL RESEARCH article
Front. Hum. Neurosci., 11 November 2011
Sec. Sensory Neuroscience
volume 5 - 2011 | https://doi.org/10.3389/fnhum.2011.00137
The present fMRI study investigated the neural areas involved in implicit perceptual sequence learning. To obtain more insight in the functional contributions of the brain areas, we tracked both the behavioral and neural time course of the learning process, using a perceptual serial color matching task. Next, to investigate whether the neural time course was specific for perceptual information, imaging results were compared to the results of implicit motor sequence learning, previously investigated using an identical serial color matching task (Gheysen et al., 2010). Results indicated that implicit sequences can be acquired by at least two neural systems: the caudate nucleus and the hippocampus, having different operating principles. The caudate nucleus contributed to the implicit sequence learning process for perceptual as well as motor information in a similar and gradual way. The hippocampus, on the other hand, was engaged in a much faster learning process which was more pronounced for the motor compared to the perceptual task. Interestingly, the perceptual and motor learning process occurred on a comparable implicit level, suggesting that consciousness is not the main determinant factor dissociating the hippocampal from the caudate learning system. This study is not only the first to successfully and unambiguously compare brain activation between perceptual and motor levels of implicit sequence learning, it also provides new insights into the specific hippocampal and caudate learning function.
Sequence learning lies at the heart of human cognition and forms the basis for efficient and adaptive behavior (Lashley, 1951). Not only are our everyday motor skills highly structured in a sequential manner, also our surrounding perceptual world is characterized by sequential regularities, e.g., the sequence of sounds in a song, letters in a word, or scenes during our daily walk home. The finding that such complex sequences can be learned outside of conscious awareness (Stadler and Frensch, 1998) has prompted researchers for many years to investigate the brain mechanisms underlying implicit sequence learning. To date, neuroimaging data converge on the idea that multiple neural systems are involved in implicit sequence learning (Grafton et al., 1995; Peigneux et al., 2000; Skosnik et al., 2002; Schendan et al., 2003). However, no consensus has been reached on the specific contribution of these different neural systems. In the present study, we wanted to test the idea that the neural systems and the pattern of neural activation involved in implicit sequence learning are defined by the type of information that is being learned. More specifically, we aimed to identify the neural basis of implicit perceptual sequence learning and to clarify whether the neural activation is unique for perceptual information, or can be generalized to other (e.g., motor related) types of information.
Previous studies investigating the neural correlates of perceptual forms of implicit sequence learning mostly used artificial grammar learning (AGL) or visual statistical learning (VSL) paradigms. Essentially these paradigms comprise similar incidental, perceptual learning conditions: during the learning phase, participants are exposed to several chains of stimuli in which the succession follows a predetermined sequential regularity. In a subsequent test phase, participants are informed about the presence (but not the identity) of this regularity and sequence learning is tested by asking the participants to classify chains of stimuli as being conform to the regularities or not. Successful classification can take place even though participants cannot consciously describe the acquired sequence rule (e.g., Lieberman et al., 2004; Turk-Browne et al., 2009). The retrieval of perceptually acquired sequence rules by means of the AGL task has been linked with the left superior occipital and left angular gyrus (Skosnik et al., 2002), the caudate nucleus and hippocampus (Lieberman et al., 2004), the left inferior frontal cortex (Forkstam et al., 2006; Udden et al., 2008), and bilateral middle occipital and left frontal gyri (Seger et al., 2000). During a VSL task, Turk-Browne et al. (2009) reported activation in the caudate nucleus, the hippocampus, and stimulus-specific visual cortical areas.
Although these previous imaging studies have provided valuable insights into the underlying brain areas and have pointed toward areas common to both AGL and VSL paradigms (e.g., caudate nucleus, hippocampus), little is understood about the specific function of these areas and the specificity of these previous imaging results for perceptual information. This can be attributed to at least two factors. AGL and VSL tasks typically assess behavioral sequence learning off-line by means of sequence judgments after the learning phase. Since there is consequently no information about the behavioral time course of the sequence learning process, clarifying the specific function of the reported brain areas is difficult. Second, it remains uncertain whether the brain areas are specifically engaged for perceptual sequential information or whether their function can be generalized to other modalities of sequence information (e.g., motor sequences). Comparing the imaging results of AGL and VSL paradigms with imaging studies using motor-related paradigms such as the serial reaction time (SRT) task (Nissen and Bullemer, 1987; e.g., Rauch et al., 1997; Schendan et al., 2003; Destrebecqz et al., 2005) is far from optimal to answer the question if there exists neural specificity for perceptual levels of implicit sequence learning. Differences in task context, procedure, stimulus characteristics, and sequence complexity do not allow for a direct comparison of the previous reported imaging results.
The present study aimed at contributing to the understanding of the neural basis of implicit perceptual sequence learning by targeting these two factors specifically. Participants were scanned during two sessions while performing the perceptual serial color matching task. A major advantage of this task is that perceptual sequence learning is tested on-line through reaction time (RT) performance: by continuously alternating short blocks of sequence and random test trials, the time course of behavioral sequence learning can be assessed (RT difference between sequence and random test trials) and can be related to the neural time course (signal difference between sequence and random test trials). Crucially, this perceptual sequence learning task can be changed into a motor sequence learning task with identical visuo-motor and cognitive task demands. In the serial color matching task, participants are instructed to match the colors of three small squares with the color of a subsequently presented large target square. In the perceptual version, a sequence structure is tied to the colors of the large target square (perceptual sequence learning; Figure 1A) whereas in the motor version, an identical sequence structure is tied to the manual responses (motor sequence learning; Figure 1B). Independent implicit perceptual and motor sequence learning has been demonstrated previously using this paradigm (Gheysen et al., 2009).
Figure 1. The serial color matching paradigm: on each trial, participants have to match the colors of the small squares with the color of the large target square. Four different responses can be given: response 1 corresponds to the condition where none of the colors of the small squares match the color of the target square; response 2 to the condition where one color of the small squares match the color of the target square; response 3 to the condition where two colors of the small squares match the color of the target square and response 4 to the condition where all three colors of the small squares match the color of the target square. (A) Perceptual version: without being informed, participants observe a repeating sequence of target colors (color 2–4–3–1–4); the colors of the small squares however are always randomly selected, hence making the response selection unpredictable from trial to trial and dissociating perceptual sequence learning from motor sequence learning. (B) Motor version: without being informed, participants perform a repeating sequence of finger responses (response 2–4–3–1–4); stimulus combinations, however, vary randomly according to color and configuration.
In sum, the present study investigated the following two questions: (1) which brain areas support implicit perceptual sequence learning and how do their neural time course relate to the behavioral time course of sequence learning; (2) are these brain areas specific for perceptual information or do they play a similar role for motor information, i.e., can their function be generalized to different modalities of implicit sequence learning? To address the first question, participants were scanned over two sessions while performing the perceptual serial color matching task. Analyses were focused on brain areas showing an activation pattern specifically related to the behavioral pattern of sequence learning. The second question was investigated by comparing the present results to those of an earlier study in which we investigated the neural correlates of implicit motor sequence learning using the motor version of the serial color matching paradigm (Gheysen et al., 2010). Importantly, because identical task demands, materials, sequence structure, procedure, and statistical analyses were used in both studies, a meaningful comparison could be made between the neural areas underlying implicit perceptual versus motor sequence learning. The analyses to answer the second question were focused on differences in behavioral and brain activation measures between both types (perceptual versus motor) of sequence information.
Twenty-two healthy volunteers were paid to participate in this study and gave written informed consent according to the guidelines of the ethical committee of the Medical Department of Ghent University Hospital. Participants were screened on their psychiatric, neurological and medical history, and the presence of ferromagnetic material in the body. All of them were right-handed and reported normal or corrected-to-normal vision and color perception. To ensure implicit levels of sequence learning, participants were selected who had no prior experience with the task and did not participate in the previous motor study (Gheysen et al., 2010). Data from two participants were excluded because of excessive head movements (>3 mm) as well as high error rates (mean error rate > 2 SD above group mean). Data analysis was thus based on 20 participants: 10 men and 10 women, aged between 19 and 30 years including High school graduates (N = 2), University students (N = 14), and University graduates (N = 4).
The perceptual version of the serial color matching task was used (Figure 1A). Tscope software (Stevens et al., 2006) was used to run the experiment and to collect the behavioral data. On each trial, a black fixation cross was presented during 200 ms and was followed by three small colored squares (2 cm × 2 cm; 1.91° × 1.91° of visual angle) that appeared in the center of the screen against a white background. These small squares were presented with a small gap (0.48° of visual angle) in between. After 600 ms, they disappeared from the screen and were replaced by a large colored square, further referred to as the target square (side = 17 cm, 15.82° of visual angle). Participants were instructed to pay attention to the colors of the small squares and to match them with the color of the target square. Responses were made by index and middle finger of both hands and were collected via magnet compatible two-button response boxes. Four different responses could be given: response 1 corresponded to the condition where none of the colors of the small squares matched the color of the target square; responses 2, 3, and 4 corresponded to the conditions where respectively one, two, or all three colors of the small squares matched the color of the target square. Trial duration was fixed on 1800 ms, meaning that the response deadline was 1000 ms.
Two color sets were used (set 1: yellow, green, red, and blue; set 2: brown, purple, orange, and gray). One color set was assigned to the sequence trials and the other color set to the random trials, counterbalanced between participants. The sequence structure was a five item deterministic sequence: 2–4–3–1–4 consisting of first as well as second order contingencies. In sequence trials this structure defined the succession of the colors of the target square: red–green–blue–yellow–green if color set 1 was assigned to the sequence trials or gray–purple–orange–brown–purple if color set 2 was assigned to the sequence trials. On each trial (sequence or random), the colors of the small squares were randomly selected, hence making the succession of response selections unpredictable and eliminating any motor sequence learning. In contrast to the sequence trials, the random trials consisted of a randomly generated succession of target colors so that no perceptual (color) sequence could be learned during this random condition. Importantly, for random trials the same relative frequencies of colors were imposed as in the sequence trials: in each random phase of 25 trials, colors 1, 2, and 3 had an equal frequency of occurrence (i.e., 5 times each phase) and color 4 occurred twice as much as the other colors (i.e., 10 times each phase). Moreover, no immediate repetitions of target colors occurred during the random trials. These constraints ascertained that RT and brain activation differences between sequence and random trials can only be attributed to respectively the presence versus absence of a repeating sequence structure. At the end of the experiment, a new target color sequence replaced the old color sequence in the last four sequence phases. The associations within this new deterministic sequence (3–4–1–3–2, i.e., blue–green–yellow–blue–red or orange–purple–brown–orange–gray) all differed from the old sequence. The last four random phases were controlled based on this new structure with respect to the relative frequency and repetition of colors (as explained above). Importantly, during the entire experiment, the frequency of the four response conditions was controlled for both sequence and random phases: each response occurred 6 or 7 times per phase of 25 trials.
Prior to scanning, participants performed the serial color matching task to familiarize with the task demands. During the practice session, 30 trials with color set 1 and 30 trials with color set 2 were alternated six times. To ascertain optimal practice, trial duration in this phase was not fixed and the maximum response time limit was set to 3000 ms; feedback on accuracy was given on each trial (for the first alternation) or after each set of 30 trials (for the next five alternations). Participants were urged to focus on accuracy first, once they understood task demands they were allowed to respond faster. Practice time took approximately 15–20 min depending on participants’ response times. During the practice session, the succession of target colors was randomly determined for all trials.
After practice and explanation of the experimental procedure but without being informed about the presence of a sequence, participants performed the task during a first scanning session. Following the acquisition of a high-resolution structural brain image, functional scans were obtained over five continuous blocks. Each scanning block (Figure 2) consisted of four repetitions of a fixation phase, an instruction phase, a sequence learning phase, an instruction phase and a random control phase. During the fixation phase, participants had to watch a black fixation cross on a white background for 15 s; during this phase they were also informed when one quarter, two quarters, and three quarters of the session had passed. During the instruction phases, participants were briefly (3 s) informed that they had to perform the task with color set 1 (first instruction) or color set 2 (second instruction) to ascertain optimal levels of preparedness and attentional set. In the sequence learning phase 25 sequence trials were presented, meaning that the target color sequence was repeated five times, whereas in the random control phase 25 trials with random target colors were to be performed. At the end of the session participants received feedback on accuracy.
Figure 2. The procedure of one scanning block included four repetitions of a fixation phase, an instruction phase, a sequence learning phase, an instruction phase and a random control phase. One scanning session comprised five blocks.
After a 10-min pause, additional training was provided outside the scanner room for approximately 30 min with 450 sequence trials and 450 random trials in total (divided over 6 sets of 150 trials). Before the start of the second scanning session, all participants received another 10-min pause.
The second scanning session was almost identical to the first session. During the first four blocks, the same target color sequence was used as in the first scanning session and the training phase. However, during the last block, a new sequence structure was introduced without informing the participants. This sequence manipulation was implemented to provide additional behavioral evidence of sequence learning and to better pinpoint the neural areas crucial for perceptual sequence learning.
After the last scanning session, participants were tested on their explicit knowledge of the color sequence. In a first test (consistent with Curran, 1997) participants were asked how they would best describe the colors of the target square in color set 1 and color set 2: (1) random, (2) some colors occurred more often than others: color(s)… occurred more often than color(s)…, (3) the succession of colors was often predictable, (4) one sequence of colors often occurred, or (5) one sequence of colors was repeated throughout the experiment. Then, participants were informed that, within one color set, the same sequence of target colors was repeated. In a second test (consistent with Destrebecqz and Cleeremans, 2001) they were asked to reproduce this color sequence for 30 trials long (recall under inclusion condition), and subsequently to generate a color sequence of 30 trials that differed as much as possible from the trained color sequence (recall under exclusion condition). In both conditions, they were instructed to avoid immediate repetitions of colors. The proportion of triplets consistent with the sequence, under inclusion and exclusion instructions, was computed. Participants that were able to correctly recall the color sequence and to refrain from that sequence when generating a different one (i.e., having a high inclusion score and low exclusion score), were considered to have explicit knowledge.
Participants were positioned head first and supine in the magnet bore. They were instructed to keep their head as still as possible. Moreover, head movements were minimized using foam padding in the head coil. Images were collected with a 3-T Siemens Magnetom Trio MRI scanner system. An eight channel head coil was used for radiofrequency excitation and signal reception. Shimming of the magnetic field was followed by the collection of 176 high-resolution anatomical images using a T1-weighted 3D anatomical sequence [TR = 1550 ms, TE = 2.39 ms, image matrix = 256 × 256, FOV = 220 mm, flip angle = 9°, slice thickness = 0.9 mm, voxel size = 0.9 mm × 0.9 mm × 0.9 mm (resized to 1 mm × 1 mm × 1 mm)]. Whole brain functional images were acquired using a T2*-weighted EPI sequence (TR = 2700 ms, TE = 35 ms, image matrix = 64 × 64, FOV = 224 mm, flip angle = 80°, slice thickness = 3.0 mm, distance factor = 17%, voxel size 3.5 mm × 3.5 mm × 3 mm, 40 axial slices). The total experiment consisted of two scanning sessions, each containing 840 scans.
The fMRI data were analyzed with the SPM5 software (Wellcome Department of Cognitive Neurology, London, UK). For each session separately, all functional scans were realigned to the first image, providing estimates of head motion. The functional images of both sessions were coregistered with the high-resolution anatomical image of the first session. Next, segmentation and normalization to the Montreal Neurological Institute template was performed. Functional images were smoothed with a Gaussian kernel of 8-mm full-width half maximum (FWHM).
Subject-level statistical analyses were performed using the general linear model (GLM). The GLM consisted of four main regressors. A first regressor modeled the general effect of (sequence and random) task. A second regressor modeled linear changes occurring over time common to both conditions to ensure that overall increasing/decreasing activation was removed (main effect of block). The third regressor searched for activations uniquely related to the sequence or to the random trials (main effect of order). Crucially, the fourth regressor defined the interaction between the previous two regressors, i.e., it modeled changes over time in the difference between sequence and random trials (order × block interaction) and was therefore assumed to catch the activations specifically contributing to the sequence learning process (identical results were obtained with a function based on RT measurements rather than a linear function). Eight additional regressors were entered into the design matrix and modeled as effects of no interest: the fixation and instruction phases and the six motion parameters obtained from the realignment procedure. The data were high-pass filtered with a cutoff of 128 s to remove low-frequency drift. Model parameters were estimated and used for single subject contrasts on our effects of interest: (1) order × block interaction and (2) main effect of order (sequence > random and random > sequence). Next, group-level random effects analyses using one-sample t-tests on the contrast images were performed. Note that the very same parameters for preprocessing and GLM analyses were used in Gheysen et al. (2010). Since behavioral results indicated implicit perceptual sequence learning to be a slow and subtle learning process and since we focused on very specific and constraining contrasts (order × block interaction, see below), imaging results were first evaluated at an uncorrected p < 0.001 level, in order to avoid type II errors. Then, to specify the pattern of brain activation in clusters resulting from this whole brain GLM analysis, we performed more specific region of interest (ROI) analyses. Clusters of brain activation were labeled according to anatomical automatic labeling (AAL; Tzourio-Mazoyer et al., 2002).
To define ROIs, we used the functional (group level) clusters, resulting from the whole brain GLM analysis of one session. To specify the pattern of brain activation over the rest of the learning process, percent signal change (psc) data were extracted from these ROIs using Marsbar (Brett et al., 2002). For each participant, psc data were computed for each block of the first and second scanning session for the sequence and random trials separately. Block × Order (sequence and random) ANOVAs were conducted on these psc data and significant effects were reported with a threshold of p < 0.05. To compare brain activation between perceptual and motor sequence learning (present study and Gheysen et al., 2010 respectively), sequence minus random psc difference scores (dpsc, reflecting sequence specific activation) were computed across all blocks of the learning process for each subject and entered into between-subjects ANOVAs and regression analyses.
Using Bayesian statistics, posterior probability maps (PPMs) were created to assess the probability for activation in the ROI to exceed the contrast-specific activation threshold (which was set at 1 SD of the prior variance of activation computed over all voxels, consistent with Friston and Penny, 2003).
The mean rate of incorrect responses was 6.78% (SD = 2.33) for the first scanning session and 5.48% (SD = 3.27) for the second scanning session. For RT analyses, incorrect responses and trials following incorrect responses were removed from further analyses. For each participant, median RTs were computed for each sequence and random phase of 25 trials and then averaged over each block (Figure 3). For the first scanning session, a 5 (Block: 1–5) × 2 (Order: sequence and random) repeated measures ANOVA only revealed a significant main effect of block [F(4,76) = 21.99, MSE = 1012, p < 0.001] reflecting general learning of task demands. The absence of an effect of order [F(1,19) = 2.66, MSE = 966, p = 0.12] or interaction effect [F(4,76) = 0.90, MSE = 531, p = 0.47] implies that there were no sequence learning effects yet in the first scanning session. Also for the training phase between the two scanning sessions, no significant sequence learning RT effects were observed [main effect of order, F(1,19) = 0.00, MSE = 1707, p = 0.99, and order × block effect, F(2,38) = 0.38, MSE = 808, p = 0.70]. The second scanning session consisted of an extended sequence learning condition, i.e., four blocks of performance with the old color sequence (i.e., the same sequence as observed in the first session) and a last block where a new, untrained color sequence was inserted. For the extended sequence learning phase, a 4 (block) × 2 (order) repeated measures ANOVA showed no main effect of block [F(3,57) = 1.08, MSE = 932, p = 0.36], but a significant main effect of order [F(1,19) = 5, MSE = 870, p = 0.038] and a significant interaction effect [F(3,57) = 4.06, MSE = 262, p = 0.011]. Pairwise comparisons between sequence and random trials for each block separately, specified that a significant difference between sequence and random trials was not yet present in block 1 (p = 0.90) or block 2 (p = 0.67) but took place in block 3 (p = 0.0006) and block 4 (p = 0.046), although this last comparison did not reach full significance after Bonferroni correction for the four comparisons. With the introduction of a new sequence structure on the last block 5, a paired t-test indicated that the significant difference between sequence and random trials disappeared [t(19) = −0.93, p = 0.36]. This manipulation on the last block confirmed that the observed RT effects in the second session were induced by the perceptual sequence and not by other general or time-related factors.
Figure 3. Behavioral performance during scanning session 1 and 2: mean of the median reaction times in each block, for sequence and random trials separately. Error bars represent the within-subject 95% confidence intervals (Loftus and Masson, 1994), *p < 0.05, ***p < 0.001.
Following the second scanning session, conscious knowledge of the color sequence was assessed. A first multiple choice question asked participants how they would best describe the target colors for both color sets. The average rating for the target colors following the sequence was 1.7 (SD = 1.13) meaning that the repeating color sequence was not noticed by the participants. Moreover this rating did not differ significantly from the average rating for target colors following a random order [M = 1.45, SD = 0.99; t(19) = 1.75, p = 0.10] (Figure 4A). In a second test, participants were asked to generate the trained sequence of target colors (inclusion condition) and to generate a succession of colors different from that trained sequence (exclusion condition). A paired t-test revealed no difference in the proportion of sequence consistent generated triplets between conditions [t(19) = −1.32, p = 0.20]. Moreover, the mean inclusion proportion [M = 0.13, SD = 0.16; t(19) = −0.34, p = 0.74] as well as the mean exclusion proportion [M = 0.19, SD = 0.19; t(19) = 1.30, p = 0.21] did not differ significantly from chance performance (0.139; Figure 4B). Similar results were obtained when analyzing sequence consistent pairs instead of triplets. Altogether, these findings suggest that participants were not aware of the repeating sequence of target colors.
Figure 4. Results on the explicit awareness tests. (A) Mean ratings for sequence trials and random trials. (B) Mean proportion of sequence consistent generated triplets, under inclusion and exclusion conditions. Error bars represent the within-subject 95% confidence intervals.
Because behavioral results showed that perceptual sequence learning RT effects were only present from the second session onward, GLM analyses were focused on the second scanning session. Furthermore, because a new sequence was introduced in the fifth block of this session, the analyses focused on the first four blocks. To capture the temporal dynamics of sequence specific learning in brain activation, an order × block interaction contrast was performed over the whole brain. To ensure the relation with sequence learning, this contrast was masked inclusively (p < 0.05) with the sequence > random contrast. Results indicated that the head of the right caudate nucleus (Figure 5, top left) demonstrated such pattern, thereby mimicking the pattern observed in RT.
Figure 5. Upper panel: Activation in the right anterior caudate nucleus (cluster found during perceptual sequence learning; MNI coordinates: 10, 18, − 6; 9 voxels), mapped onto an anatomical mean image of all participants and defining the caudate ROI for further percent signal change (psc) analyses. (A) psc data of the caudate ROI plotted over all blocks of first and second scanning session of the perceptual sequence learning study. (C) psc data of the caudate ROI plotted over all blocks of first and second scanning session of the motor sequence learning study. Lower panel: Activation in the left anterior hippocampus (cluster found during motor sequence learning (Gheysen et al., 2010); MNI coordinates: −20, −10, −14; 8 voxels), mapped onto an anatomical mean image of all participants and defining the hippocampus ROI for further psc analyses. (B) psc data of the hippocampus ROI plotted over all blocks of first and second scanning session of the perceptual sequence learning study. (D) psc data of the hippocampus ROI plotted over all blocks of first and second scanning session of the motor sequence learning study. Color bars denote the t-value. Error bars represent 95% confidence intervals, (*) p < 0.06, *p < 0.05, **p < 0.01.
In the present task, it could be that the RT advantages for sequence versus random trials were preceded by a phase where reliable learning of the color sequence took place at a neural level without being expressed in behavioral performance. To evaluate this possibility, we additionally performed the same GLM analysis on the first scanning session. Yet, this analysis did not reveal any sequence learning-related activation even by lowering the threshold to p < 0.01 uncorrected, suggesting that the brain picked up the sequential regularity only after a substantial amount of training.
The sequence > random contrast computed over the four blocks of the second session resulted in significant clusters of activation over a right lingual area and a left middle occipital area. Whereas perceptual sequence learning dynamically changed over this period of time, their function reflects continuous higher levels of activation for sequence versus random trials. Furthermore, the GLM analysis on the first scanning session demonstrated that these same areas were already activated in the sequence > random contrast of the first session. Since these areas were clearly not related to the behavioral process of sequence learning (which forms the main interest of this study), the interpretation of their specific contribution is unclear and will not be discussed further.
To better define the specific functional contribution of the right anterior caudate over the whole learning process, psc data were extracted and analyzed (Figure 5A). For the first scanning session, a 5 (block: 1–5) × 2 (order: sequence and random) repeated measures ANOVA showed no main effect of block [F(4,76) = 1.75, MSE = 0.002, p = 0.15], no main effect of order [F(1,19) = 0.34, MSE = 0.0006, p = 0.56], and no order × block interaction [F(4,76) = 0.98, MSE = 0.0003, p = 0.42]. These results indicate that the caudate had no significant function during the first scanning session. For the second scanning session, a 4 (block) × 2 (order) repeated measures ANOVA on the psc data was performed over the extended learning phase of the old sequence. This analysis yielded no main effect of block [F(3,57) = 0.80, MSE = 0.002, p = 0.50] but did indicate a main effect of order [F(1,19) = 5.11, MSE = 0.0003, p = 0.036] with overall higher levels of activation for sequence versus random trials. Moreover, a significant order × block interaction was found: F(1,19) = 2.77, MSE = 0.0003, p = 0.05. Importantly, similar to the behavioral results, pairwise comparisons showed no difference between sequence and random trials in block 1 (p = 0.53) and block 2 (p = 0.94) but did show higher levels of caudate activation for sequence versus random trials in block 3 (p = 0.040; marginally significant after Bonferroni correction for the four comparisons) and block 4 (p = 0.007; full significance after Bonferroni correction). Furthermore, replacing the old color sequence by a new color sequence on the last block of the second session had a clear impact on caudate activation: differences between sequence and random activation were no longer observed [t(19) = 1.09, p = 0.29]. Altogether, these results strongly suggest that caudate activation is closely related to the behavioral pattern of the perceptual sequence learning process. Correlation analyses confirmed this tight relationship: for each participant, a correlation coefficient was computed between the behavioral sequence learning effect (RTrandom–RTsequence) and the sequence learning-related caudate activation (pscsequence–pscrandom) over all blocks of the second scanning session. Overall, the behavioral and the caudate sequence learning pattern correlated significantly: a one-sample t-test demonstrated that correlation coefficients over all participants were reliably different from zero [mean r = 0.20, SD = 0.42, t(19) = 2.18, p = 0.042].
To compare learning between perceptual and motor sequences, random minus sequence RT difference scores (dRTs) were computed and entered into a 2 (sequence type: perceptual and motor) × 10 (block: 1–10) ANOVA. This analysis revealed a significant main effect of sequence type [F(1,40) = 8.43, MSE = 5964, p = 0.006]: sequence learning effects were overall larger for the motor compared to the perceptual sequence learning group. Effects of sequence learning across blocks also tended to differ between types of sequence information [sequence type × block interaction, F(9,360) = 1.83, MSE = 1204, p = 0.061]. To better specify these differences between the perceptual and motor sequence learning process, for each participant, a linear regression analysis was computed on the dRTs for both the early (blocks 1–5 session 1) and the extended period (blocks 6–9 session 2) of sequence learning (consistent with Lorch and Myers, 1990). This resulted in an intercept as a measure for the mean sequence learning effect over blocks (because predictors were mean-centered), and a slope reflecting the time course of the sequence learning process. As shown in Figure 6, different patterns of sequence learning were observed between types of sequence information: for the perceptual learning group, sequence learning effects in the first scanning session did not differ from zero [mean intercept = 7.17, SD = 19.65, t(19) = 1.63, p = 0.12] and slopes indicated no significant increase of sequence learning effects yet [mean slope = 2.56, SD = 10.24, t(19) = 1.12, p = 0.28]. In the second scanning session, however, sequence learning effects significantly increased [mean slope = 6.45, SD = 11.43, t(19) = 2.52, p = 0.021] and differed reliably from zero [mean intercept = 10.43, SD = 20.85, t(19) = 2.24, p = 0.038]. On the other hand, for the motor learning group, sequence learning effects already differed from zero during the first scanning session [mean intercept = 29.34, SD = 40.86, t(21) = 3.37, p = 0.003] and reliably increased across time [mean slope = 7.26, SD = 10.51, t(21) = 3.24, p = 0.004]. In the second scanning session, the sequence learning process stabilized: overall sequence learning effects were still significantly higher than zero [mean intercept = 39.33, SD = 25.57, t(21) = 7.21, p < 0.001] but did not increase further [mean slope = −1.18, SD = 12.08, t(21) = −0.49, p = 0.65].
Figure 6. Random minus sequence RT difference scores (dRTs) reflecting sequence learning effects for each block of scanning session 1 and 2 (old sequence) for implicit perceptual and motor sequence learning. Error bars represent 95% confidence intervals.
For the first test, participants’ ratings were entered into a mixed ANOVA with sequence type (perceptual versus motor) as between-subjects factor and order (sequence versus random) as within-subjects factor. This analysis showed no significant sequence type × order interaction [F(1,40) = 0.78, MSE = 0.17, p = 0.38], implying that differences in ratings for sequence trials versus random trials were comparable between perceptual and motor sequence learning. For the second test, a mixed ANOVA with sequence type as between-subjects factor and condition (inclusion versus exclusion) as within-subjects factor was performed on the proportions of sequence consistent triplets. Results indicated no significant sequence type × condition interaction [F(1,40) = 0.72, MSE = 0.03, p = 0.40], implying that differences between inclusion and exclusion generation scores did not differ between perceptual and motor sequence learning. Additional analysis on the proportion of sequence consistent pairs (assessing participants’ awareness of first-order contingencies) also revealed no significant interaction between sequence type and condition [F(1,40) = 0.03, MSE = 0.05, p = 0.86]. Altogether, these results indicate that perceptual and motor sequence learning occurred in a comparable implicit way.
In the current perceptual sequence learning fMRI study, the time course of the right anterior caudate activation was found to be closely related to the behavioral sequence learning process (Figure 5A) while in the motor sequence learning study (Gheysen et al., 2010), it was the left anterior hippocampus (Figure 5D) that matched the behavioral pattern. To further investigate whether an interaction of any kind took place between these brain regions, a more specific ROI approach was used. In the perceptual study, psc data were extracted from the functional hippocampal cluster found during motor learning (i.e., the cluster resulting from the whole brain order × block interaction contrast of session 1; Figure 5B). In the motor study, psc data were extracted from the functional caudate cluster that was found to support perceptual sequence learning (i.e., the cluster resulting from the whole brain order × block interaction contrast of session 2, blocks 1–4; Figure 5C). A 2 (sequence type: perceptual and motor) × 2 (area: caudate nucleus and hippocampus) × 10 (block: 1–10) ANOVA on the dpsc scores (see Methods) revealed a significant sequence type × area × block interaction [F(9,360) = 2.90, MSE = 0.001, p = 0.003] and thus indicated different patterns of sequence learning-related activation between areas and sequence types. Next, separate (sequence type × block) ANOVAs were performed for the hippocampus and caudate, which yielded different results per area. For the caudate nucleus, no main effect of sequence type [F(1,40) = 0.20, MSE = 0.001, p = 0.66] and no interaction between sequence type and block [F(9,360) = 0.44, MSE = 0.001, p = 0.91] were found. Thus, the overall sequence learning-related activation in the caudate nucleus as well as its involvement across blocks did not differ between the perceptual and motor sequence learning process. Additional analyses indeed indicated significant differences between sequence and random trials on the same time points (see Figures 5A,C). On the other hand, for the hippocampus, marginally significant effects of sequence type [F(1,40) = 3.39, MSE = 0.004, p = 0.073] and sequence type × block interaction [F(9,360) = 1.75, MSE = 0.002, p = 0.078] were found, suggesting that its overall contribution as well as pattern of contribution tended to differ for the perceptual and motor sequence learning process. To better delineate these differences, linear regression analyses were performed on the dpsc scores of the hippocampus per participant (cf. behavioral differences analyzed above), with the intercept indicating mean sequence learning-related activation over blocks and the slope reflecting the change of sequence learning-related activation. The results, summarized in Table 1, reveal a significant hippocampal contribution during both sessions of the sequence learning process for the motor group but not for the perceptual group. The hippocampus thus showed a significant contribution during motor learning but not during perceptual learning. Additional analyses in the perceptual sequence learning group, comparing hippocampal activation between sequence and random trials separately per block, all revealed non-significant results (all ps > 0.18; see Figures 5B,D).
Table 1. Hippocampal function during the perceptual and motor sequence learning task analyzed via linear regression analyses.
To ensure that the absence of significant hippocampal activation during perceptual sequence learning was not merely due to the inability of classical inference to detect an effect, Bayesian analyses were applied over the four blocks of the second scanning session showing evidence of perceptual sequence learning. PPMs (see Methods) indicated a maximum probability (p) of 63% for activation in the hippocampus ROI to gradually change as a function of sequence versus random trials (order × block interaction contrast). Crucially, p values were smaller for the sequence > random contrast (8%) than for the random > sequence contrast (13%), suggesting a lack of hippocampal implication during perceptual sequence learning. The same Bayesian analyses were performed on hippocampal ROI’s previously reported in the literature (Schendan et al., 2003; Lieberman et al., 2004; Albouy et al., 2008; Turk-Browne et al., 2009; spheres of 5 mm radius around the center). Again, this did not reveal higher probabilities for a hippocampal sequence learning-related contribution during both sessions of the perceptual task than the current functional hippocampal cluster.
This study aimed at a better understanding of the brain areas crucial to implicit perceptual sequence learning. By using the serial color matching task, both the behavioral and neural time course of implicit perceptual sequence learning were assessed. This represents a major advantage over other paradigms investigating implicit perceptual sequence learning (e.g., AGL and VSL paradigms) where sequence learning is typically assessed off-line.
Results indicated that perceptual sequence learning was expressed in RT performance during the second scanning session. Subsequent tests on explicit awareness indicated that sequence learning took place in an implicit manner. Importantly, sequence and random trials only differed in the presence of a repeating sequence of colors and were completely balanced with respect to all other task aspects and color and response frequency information. Therefore, we can safely conclude that the observed RT differences between sequence and random trials reflect the learning of a specific perceptual sequence of colors and cannot be related to the learning of general task aspects or simple frequency information. Implicit perceptual sequence learning was found to be a slower learning process eliciting smaller RT effects than implicit motor sequence learning. This is in line with our previous behavioral study (Gheysen et al., 2009) and with previous research showing that, in contrast with motor learning, perceptually based sequence learning is more vulnerable since it was observed only with simple sequence structures (Deroost and Soetens, 2006) or salient stimulus material (Kelly et al., 2003).
On a neural level, the caudate nucleus was found to be closely related to the behavioral RT pattern: the difference in activation for sequence versus random trials gradually increased across the second scanning session with overall higher levels of activation for sequence versus random trials. Furthermore, replacing the old color sequence by a new color sequence at the end of the second scanning session significantly influenced the caudate activation, hence confirming its function in sequence specific perceptual learning. In our study, a right anterior part of the caudate nucleus was activated. Although this area is close to the caudate region found in the implicit sequence learning study of Destrebecqz et al. (2005) and Lieberman et al. (2004), it remains to be investigated whether our findings can be generalized or whether the reported caudate activation is specific for the current task and sequence material being used.
A second goal of the study was to clarify whether or not the reported brain areas display a pattern of activation that is specific for perceptual sequences. Therefore, imaging results were compared to the results of implicit motor sequence learning previously investigated using an identical serial color matching task (Gheysen et al., 2010). To our knowledge, this is the first study to successfully and unambiguously compare brain activation between implicit perceptual and motor sequence learning. One previous fMRI study attempted to dissociate the neural correlates of motor sequence retrieval from perceptual sequence retrieval using a transfer SRT task (Bischoff-Grethe et al., 2004). However, because participants in the implicit perceptual transfer group showed no evidence of learning the sequence of stimulus locations and failed to show significant brain activation, the neural activation between modalities could not be compared. In the present study, the comparison of imaging results was focused on two brain areas: the caudate nucleus and the hippocampus. Results indicated that the right anterior caudate nucleus not only had a key function during implicit learning of perceptual sequences but showed a similar time course for motor sequences. The hippocampal contribution, however, differed for perceptual versus motor sequences. The significant sequence learning-related activation that was found for motor sequences (Gheysen et al., 2010), was not observed for perceptual sequences. Additional analyses on other hippocampal ROI’s previously reported in the literature (Schendan et al., 2003; Lieberman et al., 2004; Albouy et al., 2008; Turk-Browne et al., 2009), suggested that the lack of a significant hippocampal implication during the perceptual sequence learning task was not related to the specific subregion of the hippocampus.
Over the last years, a growing body of evidence has shown that the caudate nucleus, and the striatum in general, has a key role in implicit sequence learning. Its function has been reported for motor sequences (Grafton et al., 1995; Rauch et al., 1997; Peigneux et al., 2000; Destrebecqz et al., 2005) as well as perceptual sequences (Lieberman et al., 2004; Turk-Browne et al., 2009) using different paradigms. Our results are in line with these previous imaging studies. Findings of caudate recruitment during both perceptual and motor sequence learning are reasonable given its connections with sensory, motor, and associative cortices (Da Cunha et al., 2009). Yet, our study is the first to show a similar time course of caudate activation for perceptual versus motor sequence information. The same right anterior caudate area displayed learning-dependent activation for perceptual and motor sequences at the same time point during scanning which suggests that this area fulfilled a similar function for both learning processes.
During perceptual and motor sequence learning, this caudate function was characterized by a relatively slow and gradual time course. This agrees with previous accounts of the caudate (striatal) learning process (Jog et al., 1999; Da Cunha et al., 2009). Moreover, it has been suggested to be a process where the repeated and convergent sequence information, projected into the striatum, is gradually fragmented into chunks (Graybiel, 1998; Boyd et al., 2009), a mechanism known to be highly efficient for information processing and memory (as first shown by Miller, 1956). Interestingly, this gradual chunking mechanism of the striatum fits within the computational chunking-model proposed to potentially underlie implicit sequence learning (Cleeremans and Dienes, 2008).
In our previous motor study, the early phase of implicit motor sequence learning was supported by the hippocampus (Gheysen et al., 2010). Similar findings of hippocampal recruitment during early and striatal activation during more advanced stages of implicit motor sequence learning have been reported (Schendan et al., 2003; Albouy et al., 2008). Such dynamic cerebral interaction between the hippocampus and the striatum is consistent with numerous animal and human studies demonstrating that both systems interact during various learning situations (Packard and Knowlton, 2002; Poldrack and Packard, 2003). In the present study, however, no significant hippocampal learning function was found for perceptual sequences. At first, this seems remarkable as the hippocampus is not regarded as a motor system and has been repeatedly associated with perceptual forms of associative learning (e.g., Fortin et al., 2002; Van Opstal et al., 2008) even for implicit and more complex higher-order sequence information (Lieberman et al., 2004; Turk-Browne et al., 2009).
In the current study, implicit perceptual sequence learning elicited smaller RT effects than implicit motor sequence learning. It could therefore be argued that the lack of significant hippocampal contribution during perceptual sequence learning might be related to the weaker learning effect, and consequently, that hippocampal activity is related to better learning in general. This hypothesis however conflicts with current knowledge on the hippocampal learning system: in recent literature, it is commonly agreed based on theoretical and empirical arguments that the hippocampus represents a fast learning system that acts during early stages of learning. At a theoretical level, O’Reilly and Rudy (2001), for instance, presented a computational model of the hippocampal learning system as an area specialized in the rapid encoding of conjunctive information. At an empirical level, studies on the hippocampal place cells are in line with this framework by demonstrating that the firing rate of these cells alters following only minor changes of the environment (Colgin et al., 2008). Likewise, human fMRI studies using a variety of learning tasks, such as sequence learning (Schendan et al., 2003; Albouy et al., 2008), VSL (Turk-Browne et al., 2009), or transitive inference tasks (Van Opstal et al., 2008), reported a significant contribution of the hippocampus during early stages of the learning process. Importantly, it has been shown that the hippocampal learning function is taken over by more modality specific cortical brain areas when learning advances further (Takashima et al., 2009; Van Opstal et al., 2009). Thus, the hypothesis that the hippocampus might be related to better learning in general (and therefore failed to demonstrate significant activation in the perceptual study) does not fit with current computational and imaging literature. If the function of the hippocampus would indeed be related to better learning, its contribution is expected to be pronounced in later, advanced stages of learning rather than early stages.
Traditionally, the hippocampus has been associated with explicit/declarative and the striatum with implicit/non-declarative forms of learning (Squire, 2009). However, nowadays, neuropsychological and neuroimaging data are accumulating that such simple dichotomy is no longer tenable (Chun and Phelps, 1999; Ryan et al., 2000; Schendan et al., 2003; Lieberman et al., 2004; Albouy et al., 2008; Turk-Browne et al., 2009). Moreover, even if we would assume a gradual dissociation between both learning systems with higher levels of awareness being associated with the hippocampus, it can not explain our imaging findings because our behavioral results clearly indicated comparable levels of sequence awareness between the motor and perceptual sequence learning process. Another traditional view on the hippocampus, mostly coming from experimental animal studies, is that the hippocampus functions predominantly in spatial learning conditions (O’Keefe, 1999). This might lead to the hypothesis that the involvement of the hippocampus in the motor study but not in the perceptual study may be due to the spatial nature of the motor sequence and the absence of any spatial context into the perceptual sequence. Yet, previous studies indicated a learning function for the hippocampus regardless of any spatial attributes (Fortin et al., 2002; Brasted et al., 2003; Lieberman et al., 2004; Turk-Browne et al., 2009).
Altogether, the above theories cannot provide a convincing explanation for the less pronounced hippocampal activation during the perceptual serial color matching task. Another theoretical framework (O’Reilly and Rudy, 2001) might give a reasonable account for the differential involvement of the hippocampus in our motor and perceptual learning study. According to this framework, the hippocampal system is specialized for the rapid, incidental development of conjunctive representations. Lesion studies in rodents and human fMRI studies have shown a particular function for the hippocampus in processing multimodal information and combining detailed information from multiple cortical streams into a unified representation rather than in processing simple, elemental information (Phillips and Ledoux, 1992; Iordanova et al., 2009; Staresina and Davachi, 2009). Neuroanatomical data are also compatible with this theory; they indicate that the hippocampus is particularly suited for configural integration since it receives information from virtually all cortical association areas (Suzuki and Amaral, 2004; Dickerson and Eichenbaum, 2010).
Consequently, the notion that the hippocampus plays an important role in configural processing but not in the processing of elemental information might give a reasonable explanation for the higher contribution of the hippocampus in the motor compared to the perceptual learning study. Motor responses composing the sequence structure in the former study entail a multitude of information going from the obvious motor-related information to proprioceptive, tactile, and spatial information whereas the colors composing the sequence structure in the latter study constitute elemental visual information. Possibly, the motor sequence learning condition triggered the hippocampal function more than the perceptual sequence learning condition since it naturally contained richer information.
The current study provides interesting new insights into the specific function of the caudate and hippocampal learning system. Results indicated an important function for the caudate nucleus in implicit perceptual sequence learning. By using a carefully controlled paradigm, we were able to demonstrate that the caudate learning function was not specific for perceptual information: a similar time course of sequence learning-related activation was found for perceptual and motor sequence information. In both learning processes, the caudate system reflected a relatively slow and gradual learning mechanism. The hippocampal learning system, on the other hand, displayed a much faster learning process which was less pronounced in the perceptual task compared to the motor task. Previous literature suggests that this differential involvement of the hippocampus in our tasks probably was not related to the perceptual versus motor nature of the sequence structure per se, but to the unimodal versus multimodal character of the sequence information. Future research is however necessary to elaborate this hypothesis. Furthermore, the findings of the present study are in line with an important, more recent notion that the traditional explicit–hippocampus and implicit–striatum framework proves insufficient. To fully understand the distinct learning function of both neural systems, it appears that other factors, such as the type of information, need to be taken into account.
The authors declare that the research was conducted in the absence of any commercial or financial relationships that could be construed as a potential conflict of interest.
Freja Gheysen is supported by a grant from the Research Council of Ghent University. Filip Van Opstal is a Postdoctoral fellow of the Research Foundation – Flanders (FWO-Vlaanderen). This work was supported by the Ghent University Multidisciplinary Research Partnership “The integrative neuroscience of behavioral control” and by grant P6/29 from the Interuniversitary Attraction Poles program of the Belgian federal government.
Albouy, G., Sterpenich, V., Balteau, E., Vandewalle, G., Desseilles, M., Dang-Vu, T., Darsaud, A., Ruby, P., Luppi, P., Degueldre, C., Peigneux, P., Luxen, A., and Maquet, P. (2008). Both the hippocampus and striatum are involved in consolidation of motor sequence memory. Neuron 58, 261–272.
Bischoff-Grethe, A., Goedert, K. M., Willingham, D. T., and Grafton, S. T. (2004). Neural substrates of response-based sequence learning using fMRI. J. Cogn. Neurosci. 16, 127–138.
Boyd, L. A., Edwards, J. D., Siengsukon, C. S., Vidoni, E. D., Wessel, B. D., and Linsdell, M. A. (2009). Motor sequence chunking is impaired by basal ganglia stroke. Neurobiol. Learn. Mem. 92, 35–44.
Brasted, P. J., Bussey, T. J., Murray, E. A., and Wise, S. P. (2003). Role of the hippocampal system in associative learning beyond the spatial domain. Brain 126, 1202–1223.
Brett, M., Anton, J.-L., Valabregue, R., and Poline, J.-B. (2002). Region of interest analysis using an SPM toolbox [abstract]. Presented at the 8th International Conference on Functional Mapping of the Human Brain, Sendai. [Available on CD-ROM in Neuroimage, Vol. 16, No. 2].
Chun, M. M., and Phelps, E. A. (1999). Memory deficits for implicit contextual information in amnesic subjects with hippocampal damage. Nat. Neurosci. 2, 844–847.
Cleeremans, A., and Dienes, Z. (2008). “Computational models of implicit learning,” in The Cambridge Handbook of Computational Modeling, ed. R. Sun (Cambridge: Cambridge University Press), 396–421.
Colgin, L. L., Moser, E. I., and Moser, M. B. (2008). Understanding memory through hippocampal remapping. Trends Neurosci. 31, 469–477.
Curran, T. (1997). Higher-order associative learning in amnesia: evidence from the serial reaction time task. J. Cogn. Neurosci. 9, 522–533.
Da Cunha, C., Wietzikoski, E. C., Dombrowski, P., Bortolanza, M., Santos, L. M., Boschen, S. L., and Miyoshi, E. (2009). Learning processing in the basal ganglia: a mosaic of broken mirrors. Behav. Brain Res. 199, 157–170.
Deroost, N., and Soetens, E. (2006). Perceptual or motor learning in SRT tasks with complex sequence structures. Psychol. Res. 70, 88–102.
Destrebecqz, A., and Cleeremans, A. (2001). Can sequence learning be implicit? New evidence with the process dissociation procedure. Psychon. Bull. Rev. 8, 343–350.
Destrebecqz, A., Peigneux, P., Laureys, S., Degueldre, C., Del Fiore, G., Aerts, J., Luxen, A., Van Der Linden, M., Cleeremans, A., and Maquet, P. (2005). The neural correlates of implicit and explicit sequence learning: interacting networks revealed by the process dissociation procedure. Learn. Mem. 12, 480–490.
Dickerson, B. C., and Eichenbaum, H. (2010). The episodic memory system: neurocircuitry and disorders. Neuropsychopharmacology 35, 86–104.
Forkstam, C., Hagoort, P., Fernandez, G., Ingvar, M., and Petersson, K. M. (2006). Neural correlates of artificial syntactic structure classification. Neuroimage 32, 956–967.
Fortin, N. J., Agster, K. L., and Eichenbaum, H. B. (2002). Critical role of the hippocampus in memory for sequences of events. Nat. Neurosci. 5, 458–462.
Friston, K. J., and Penny, W. (2003). Posterior probability maps and SPMs. Neuroimage 19, 1240–1249.
Gheysen, F., Gevers, W., De Schutter, E., Van Waelvelde, H., and Fias, W. (2009). Disentangling perceptual from motor implicit sequence learning with a serial color-matching task. Exp. Brain Res. 197, 163–174.
Gheysen, F., Van Opstal, F., Roggeman, C., Van Waelvelde, H., and Fias, W. (2010). Hippocampal contribution to early and later stages of implicit motor sequence learning. Exp. Brain Res. 202, 795–807.
Grafton, S. T., Hazeltine, E., and Ivry, R. (1995). Functional mapping of sequence learning in normal humans. J. Cogn. Neurosci. 7, 497–510.
Graybiel, A. M. (1998). The basal ganglia and chunking of action repertoires. Neurobiol. Learn. Mem. 70, 119–136.
Iordanova, M. D., Burnett, D. J., Aggleton, J. P., Good, M., and Honey, R. C. (2009). The role of the hippocampus in mnemonic integration and retrieval: complementary evidence from lesion and inactivation studies. Eur. J. Neurosci. 30, 2177–2189.
Jog, M. S., Kubota, Y., Connolly, C. I., Hillegaart, V., and Graybiel, A. M. (1999). Building neural representations of habits. Science 286, 1745–1749.
Kelly, S. W., Burton, A. M., Riedel, B., and Lynch, E. (2003). Sequence learning by action and observation: evidence for separate mechanisms. Br. J. Psychol. 94, 355–372.
Lashley, K. S. (1951). “The problem of serial order in behaviour,” in Cerebral Mechanisms in Behaviour, ed. L. A. Jeffress (New York, NY: Wiley).
Lieberman, M. D., Chang, G. Y., Chiao, J., Bookheimer, S. Y., and Knowlton, B. J. (2004). An event-related fMRI study of artificial grammar learning in a balanced chunk strength design. J. Cogn. Neurosci. 16, 427–438.
Loftus, G. R., and Masson, M. E. J. (1994). Using confidence-intervals in within-subject designs. Psychon. Bull. Rev. 1, 476–490.
Lorch, R. F., and Myers, J. L. (1990). Regression-analyses of repeated measures data in cognitive research. J. Exp. Psychol. Learn. Mem. Cogn. 16, 149–157.
Miller, G. A. (1956). The magical number 7, plus or minus 2 – some limits on our capacity for processing information. Psychol. Rev. 63, 81–97.
Nissen, M. J., and Bullemer, P. (1987). Attentional requirements of learning – evidence from performance-measures. Cogn. Psychol. 19, 1–32.
O’Keefe, J. (1999). Do hippocampal pyramidal cells signal non-spatial as well as spatial information? Hippocampus 9, 352–364.
O’Reilly, R. C., and Rudy, J. W. (2001). Conjunctive representations in learning and memory: principles of cortical and hippocampal function. Psychol. Rev. 108, 311–345.
Packard, M. G., and Knowlton, B. J. (2002). Learning and memory functions of the basal ganglia. Annu. Rev. Neurosci. 25, 563–593.
Peigneux, P., Maquet, P., Meulemans, T., Destrebecqz, A., Laureys, S., Degueldre, C., Delfiore, G., Aerts, J., Luxen, A., Franck, G., Van Der Linden, M., and Cleeremans, A. (2000). Striatum forever, despite sequence learning variability: a random effect analysis of PET data. Hum. Brain Mapp. 10, 179–194.
Phillips, R. G., and Ledoux, J. E. (1992). Differential contribution of amygdala and hippocampus to cued and contextual fear conditioning. Behav. Neurosci. 106, 274–285.
Poldrack, R. A., and Packard, M. G. (2003). Competition among multiple memory systems: converging evidence from animal and human brain studies. Neuropsychologia 41, 245–251.
Rauch, S. L., Whalen, P. J., Savage, C. R., Curran, T., Kendrick, A., Brown, H. D., Bush, G., Breiter, H., and Rosen, B. (1997). Striatal recruitment during an implicit sequence learning task as measured by functional magnetic resonance imaging. Hum. Brain Mapp. 5, 124–132.
Ryan, J. D., Althoff, R. R., Whitlow, S., and Cohen, N. J. (2000). Amnesia is a deficit in relational memory. Psychol. Sci. 11, 454–461.
Schendan, H. E., Searl, M. M., Melrose, R. J., and Stern, C. E. (2003). An fMRI study of the role of the medial temporal lobe in implicit and explicit sequence learning. Neuron 37, 1013–1025.
Seger, C. A., Prabhakaran, V., Poldrack, R. A., and Gabrieli, J. D. (2000). Neural activity differs between explicit and implicit learning of artificial grammar strings: an fMRI study. Psychobiology 28, 283–292.
Skosnik, P. D., Mirza, F., Gitelman, D. R., Parrish, T. B., Mesulam, M. M., and Reber, P. J. (2002). Neural correlates of artificial grammar learning. Neuroimage 17, 1306–1314.
Staresina, B. P., and Davachi, L. (2009). Mind the gap: binding experiences across space and time in the human hippocampus. Neuron 63, 267–276.
Stevens, M., Lammertyn, J., Verbruggen, F., and Vandierendonck, A. (2006). Tscope: a C library for programming cognitive experiments on the MS Windows platform. Behav. Res. Methods 38, 280–286.
Suzuki, W. A., and Amaral, D. G. (2004). Functional neuroanatomy of the medial temporal lobe memory system. Cortex 40, 220–222.
Takashima, A., Nieuwenhuis, I. L. C., Jensen, O., Talamini, L. M., Rijpkema, M., and Fernandez, G. (2009). Shift from hippocampal to neocortical centered retrieval network with consolidation. J. Neurosci. 29, 10087–10093.
Turk-Browne, N. B., Scholl, B. J., Chun, M. M., and Johnson, M. K. (2009). Neural evidence of statistical learning: efficient detection of visual regularities without awareness. J. Cogn. Neurosci. 21, 1934–1945.
Tzourio-Mazoyer, N., Landeau, B., Papathanassiou, D., Crivello, F., Etard, O., Delcroix, N., Mazoyer, B., and Joliot, M. (2002). Automated anatomical labeling of activations in SPM using a macroscopic anatomical parcellation of the MNI MRI single-subject brain. Neuroimage 15, 273–289.
Udden, J., Folia, V., Forkstam, C., Ingvar, M., Fernandez, G., Overeem, S., van Elswijk, G., Hagoort, P., and Petersson, K. (2008). The inferior frontal cortex in artificial syntax processing: an rTMS study. Brain Res. 1224, 69–78.
Van Opstal, F., Fias, W., Peigneux, P., and Verguts, T. (2009). The neural representation of extensively trained ordered sequences. Neuroimage 47, 367–375.
Keywords: implicit sequence learning, perceptual sequence learning, motor sequence learning, fMRI, caudate nucleus, hippocampus
Citation: Gheysen F, Van Opstal F, Roggeman C, Van Waelvelde H and Fias W (2011) The neural basis of implicit perceptual sequence learning. Front. Hum. Neurosci. 5:137. doi: 10.3389/fnhum.2011.00137
Received: 06 June 2011;
Accepted: 28 October 2011;
Published online: 11 November 2011.
Edited by:
Robert T. Knight, University of California Berkeley, USAReviewed by:
Leighton B. Hinkley, University of California, USACopyright: © 2011 Gheysen, Van Opstal, Roggeman, Van Waelvelde and Fias. This is an open-access article subject to a non-exclusive license between the authors and Frontiers Media SA, which permits use, distribution and reproduction in other forums, provided the original authors and source are credited and other Frontiers conditions are complied with.
*Correspondence: Freja Gheysen, Department of Experimental Psychology, Ghent University, Henri Dunantlaan 2, 9000 Ghent, Belgium. e-mail:ZnJlamEuZ2hleXNlbkB1Z2VudC5iZQ==
Disclaimer: All claims expressed in this article are solely those of the authors and do not necessarily represent those of their affiliated organizations, or those of the publisher, the editors and the reviewers. Any product that may be evaluated in this article or claim that may be made by its manufacturer is not guaranteed or endorsed by the publisher.
Research integrity at Frontiers
Learn more about the work of our research integrity team to safeguard the quality of each article we publish.