- 1 Beckman Institute for Advanced Science and Technology, University of Illinois Urbana-Champaign, Urbana, IL, USA
- 2 Department of Psychology, University of Illinois Urbana-Champaign, Urbana, IL, USA
Amnesic patients have difficulties recognizing when stimuli are repeated, even though their responses to stimuli can change as a function of repetition in indirect tests of memory – a pattern known as priming without recognition. Likewise, experimental manipulations can impair recognition in healthy individuals while leaving priming relatively unaffected, and priming and recognition have been associated with distinct neural correlates in these circumstances. Does this evidence necessarily indicate that priming and recognition rely on distinct brain systems? An alternative explanation is that recognition is merely more sensitive to amnestic insults and experimental manipulations than is priming, and that both priming and recognition are produced by a single brain system. If so, then experimental manipulations would tend to drive priming and recognition in the same direction, albeit to a greater extent for one versus the other in some circumstances. We found evidence to the contrary – that manipulating study duration has opposite effects on priming versus recognition. Studying objects for one-quarter second led to worse recognition than studying objects for 2 s, whereas the opposite was true for priming (greater for one-quarter-second study than two-second study). Furthermore, distinct electrophysiological repetition effects were associated with priming versus recognition. We therefore conclude that study duration had opposite effects on priming and recognition, and on the engagement of implicit versus explicit memory systems. These findings call into question single-process accounts of priming and recognition, and substantiate previous behavioral, neuropsychological, and neuroimaging dissociations between implicit and explicit memory.
Introduction
Priming and recognition are distinct behavioral expressions of memory that feature prominently in the human memory literature. Priming is generally defined as faster, more accurate, or otherwise facilitated responding in tests that measure memory implicitly, that is, without the subject’s awareness that memory is being tested (Roediger, 1990; Schacter and Buckner, 1998b; Wiggs and Martin, 1998; Henson, 2003; Schacter et al., 2007). Recognition involves the discrimination between previously studied and novel information, and is considered an explicit expression of memory, in that it generally involves conscious awareness that memory is being expressed and confidence in the decisions that are made (Tulving, 1985; Yonelinas, 2001). Priming and recognition are widely thought to result from the operation of two distinct memory systems, an implicit memory system that supports priming and an explicit memory system that supports recognition (Gabrieli, 1998; Squire, 2004). Nonetheless, the distinction between priming and recognition has been challenged based on work showing that a single-process based on signal detection can account for priming and recognition (Berry et al., 2008a,b; see also Reder et al., 2009). The question that we address in the current study is whether processes that support priming also support recognition, and vice versa.
Neuroanatomical dissociations between recognition and priming have typically been identified in amnesic patients, whereby damage to the hippocampus and surrounding medial temporal lobe (MTL) cortex disrupts recognition but not priming (Warrington and Weiskrantz, 1970; Shimamura, 1986; Hamann and Squire, 1997; Schacter and Buckner, 1998b; Squire, 2004). However, an alternative interpretation is that both recognition and priming rely on MTL structures, but that recognition is more sensitive to damage than priming (see Berry et al., 2008a,b). A “double-dissociation” (Teuber, 1955) between recognition and priming would be required to show that they rely on distinct brain structures, such that recognition would be disrupted by damage to structure A but not structure B and priming would be disrupted by damage to structure B but not A. Cortical damage has been shown to impair priming but not recognition (reviewed by Gabrieli, 1998), but recent studies have suggested a more complex relationship between cortical damage and priming (e.g., Kroll et al., 2003) that undermines any clear double-dissociation between neural substrates of priming and recognition. Additional evidence is therefore needed to show conclusively that priming and recognition are neuroanatomically distinct (see also Kinder and Shanks, 2003).
Studies on healthy individuals have measured neural correlates of either priming or of recognition, and different patterns of brain activity have been linked to one versus the other (Gabrieli, 1998; Schacter and Buckner, 1998a,b; Henson, 2003, 2005; Wagner et al., 2005). Generally, superior prefrontal cortex, MTL, and parietal cortex are associated with recognition, whereas occipitotemporal and inferior prefrontal cortex are associated with priming. However, results from only a handful of studies that attempted to contrast neural correlates of priming and recognition obtained under highly similar circumstances are relevant to the debate over whether these memory expressions are neurally dissociable (see Berry et al., 2008b; reviewed in Voss and Paller, 2008a). Some of these studies (Rugg et al., 1998; Paller et al., 2003; Henson et al., 2005; Schott et al., 2005) compared neural measures obtained in circumstances when recognition was strong and accurate to neural measures obtained in circumstances when recognition was absent and behavioral evidence for priming was present (i.e., by focusing on recognition hits versus recognition misses when priming was operative). Dissociations were found between neural correlates of priming and recognition, and this was taken to indicate that priming and recognition rely on distinct neural substrates.
A limitation of the aforementioned neuroimaging evidence, discussed by Berry et al. (2008b), is that the behavioral effects for which neural correlates were obtained were not sufficient to dissociate priming from recognition. In fact, difficulties associated with separating implicit and explicit memory on behavioral grounds have long been appreciated (Richardson-Klavehn and Bjork, 1988). The neuroimaging studies attempted to isolate priming by examining it when subjects failed to give a correct recognition response. This strategy is tantamount to showing that a given experimental manipulation produces a greater effect on recognition than on priming; that is, priming was robust even when recognition was poor. Thus, a single memory retrieval process described by a signal-detection model could have resulted in the observed behavioral dissociations if priming and recognition were to differ in the level of noise against which decisions were made (Berry et al., 2008b). The same could be true of the observed neural dissociations if neural correlates of priming and recognition also differed in the level of noise (Henson, 2006). If priming and recognition were to rely on independent brain processes, then it should be possible to identify experimental manipulations that produce opposite effects on priming, recognition, and their neural measures. We therefore sought to identify circumstances for which these conditions are met.
Zago et al. (2005) identified a counterintuitive relationship between the amount of time that an object is studied and the magnitude of later priming for the object, measured as the speed of a category verification decision. The magnitude of priming increased as study duration increased from 20 to 250 ms, and declined as study duration further increased from 250 ms to approximately 2 s. Two processes, “sharpening” and “selection,” were hypothesized to underlie this trend that are broadly consistent with previous mechanistic accounts of priming (Desimone, 1996; Wiggs and Martin, 1998). Consonant with the finding that the magnitude of behavioral priming was heavily influenced by study duration, Zago et al. (2005) identified a rise-and-fall pattern in the magnitude of repetition suppression of neural responses in priming-associated cortical regions including occipitotemporal and inferior prefrontal cortex. These neural findings are consistent with the interpretation that priming was positively related to study duration before 250 ms and inversely related to study duration after 250 ms.
In contrast, the accuracy of recognition improves roughly as a log-linear function as study time increases (Tversky and Sherman, 1975; Loftus and Kallman, 1979), including on the brief time scale of 250 ms to 2 s (Ellis et al., 1977) used to study priming by Zago et al. (2005). We therefore reasoned that manipulating study duration for objects could exert opposite effects on priming and recognition. We had participants study objects either for 250 ms or 2 s, and later measured both priming and recognition for each object during a memory test in which all objects were presented for identical durations (Figure 1). We hypothesized that priming would be greatest and recognition would be least for objects studied for 250 ms, whereas priming would be least and recognition would be greatest for objects studied for 2 s. Furthermore, we collected event-related brain potential (ERP) responses to objects in order to test for neural dissociations between priming and recognition1. Based on previous findings (reviewed in Voss and Paller, 2008a), we hypothesized ERP correlates priming and recognition would dissociate based on the timing of the relevant neural processing, with earlier effects attributable to priming, as well as possibly in the location and/or directionality of the relevant neural processing. This latter prediction is based on consistent findings showing reduced neural activity at posterior visual regions in association with priming, in contrast to greater neural activity at fronto-parietal regions in association with recognition (see also Gabrieli, 1998; Schacter et al., 2007).
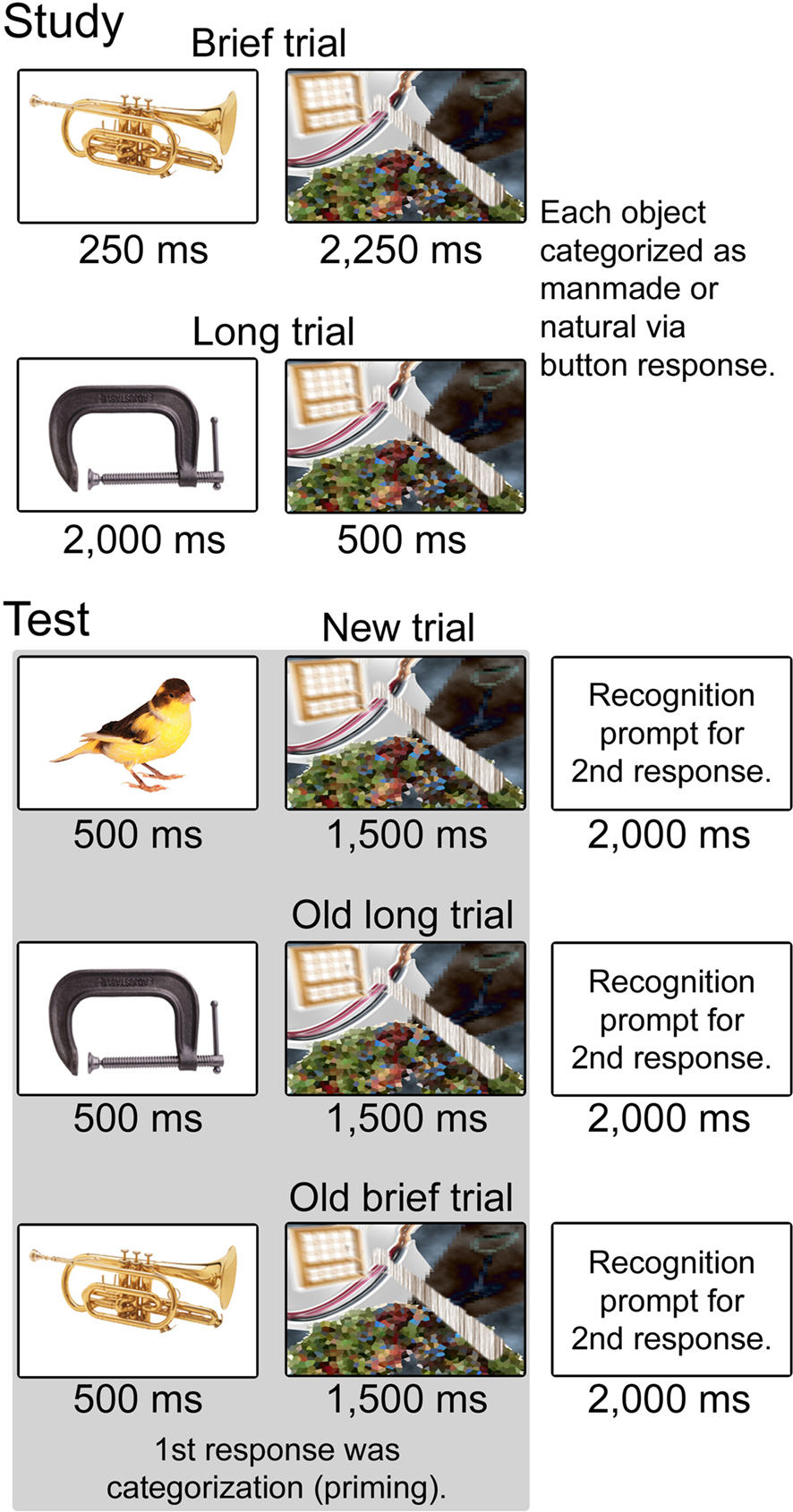
Figure 1. Schematic representation of trial types during study and test. Conditions during study included Brief (250 ms object presentation) and Long (2,000 ms object presentation). Objects in each condition were followed by a mask such that trial duration was 2,500 ms for both conditions. Subjects categorized each object as “natural” or “manufactured.” Conditions during test included Brief and Long objects repeated from the study phase as well as new objects. Objects in all conditions were presented for 500 ms with a 1,500 ms mask. Two behavioral responses were registered for each object. The first was a categorization response to measure priming, made as soon as possible after the stimulus was presented. The second was a recognition response including four confidence levels, made to a prompt that followed the mask.
Materials and Methods
Visual stimuli included 320 color digital photographs of common, nameable objects. Each image depicted a unique object. Approximately half were natural objects (e.g., an alligator, an orange, wheat), and half were manufactured objects (e.g., scissors, a chair, a balloon). Object images were displayed on a white background at the center of a computer monitor, and were sized to fit within a square subtending approximately 4° of visual angle.
During the study phase, subjects (N = 14, ages 18–24, 8 female, all right-handed) viewed 170 objects. Half of the objects were displayed for 250 ms (Brief) and half were displayed for 2,000 ms (Long). Objects assigned to the Brief and Long conditions were counterbalanced across subjects. A mask was displayed after each object, comprising a collection of distorted features of objects that were not in the main stimulus set (Figure 1). The mask was displayed for 2,250 ms following each Brief object and for 500 ms following each Long object, such that the total duration of each trial was 2,500 ms (note that different mask durations for the Brief and Long conditions could potentially have contributed to any observed behavioral or neural differences, although it seems reasonable to attribute different effects to the manipulation of stimulus duration). The same mask was used throughout the entire experiment. Subjects were required to categorize each object as natural or manmade using two buttons. Approximately half of the objects in each condition were natural. Speed was not emphasized, and subjects were instructed to respond based on their first impression regarding the category (i.e., that there was “no right answer”). Trial order was randomized.
The test phase followed the study phase after a break of approximately 3 min, during which subjects were given instructions. Subjects viewed all 320 objects, each of which belonged to one of three conditions: those presented for 250 ms during the study phase (old Brief, 85 objects), those presented for 2,000 ms during the study phase (old Long, 85 objects), and those that were not presented during the study phase (new, 150 objects). Subjects were required to make two button-press responses to each object. The first response was the natural/manufactured categorization response used during the study phase, and this response was registered as soon as possible after the object was displayed. Speed for this response was heavily emphasized. To compute accuracy for this subjective response, each object was characterized as natural or manufactured based on the majority response for all subjects during the test phase. The second response was a recognition decision crossed with a confidence rating. Subjects pressed one of four buttons to indicate that the object was old with high confidence, old with low confidence, new with low confidence, or new with high confidence. This response was made after a prompt was displayed, and accuracy was emphasized over speed. All objects were presented for 500 ms, followed by the mask used during the study phase for 2,000 ms. Immediately following the mask, the recognition prompt (the letter “R” for “respond”) was displayed for 2,000 ms. A 500-ms break separated the recognition prompt from the next trial. Trial order was randomized.
EEG was sampled continuously during the test phase from 64 scalp locations conforming to the extended International 10-20 positioning system (Chatrian et al., 1988) using a BioSemi Active II system (BioSemi Instrumentation, Amsterdam). Recordings were also made from left and right mastoids, and four additional channels were used to monitor horizontal and vertical eye movements. EEG was digitized at a rate of 1,024 Hz with a bandpass of 0.01–120 Hz. Recordings were made with the standard Biosemi reference (CMS-DRL), and rereferenced offline to averaged mastoids in order to facilitate comparisons with previous studies of ERP correlates of recognition memory, which frequently use this reference. ERPs timelocked to the onset of the visual object stimulus (not the recognition prompts) were calculated for each condition of interest in 1,000 ms epochs, beginning 100 ms prior to stimulus onset. Epochs contaminated by artifacts were discarded (9% on average) leaving a final average trial count of 76, 79, and 138 for the Brief, Long, and New conditions, respectively. Data from three additional subjects were excluded due to excessive artifacts (>25% of epochs contaminated). ERPs were averaged over latency intervals and electrode clusters for statistical assessment. Six clusters included anterior, central, and posterior electrodes for both hemispheres (Anterior left: Fp1, AF3, AF7, F1, F3, F5, F7; anterior right: Fp2, AF4, AF8, F2, F4, F6, F8; central left: FC1, FC3, FC5, C1, C3, C5, CP1, CP3, CP5; central right: FC2, FC4, FC6, C2, C4, C6, CP2, CP4, CP6; posterior left: P1, P3, P5, P7, P9, PO3, PO7, O1; posterior right: P2, P4, P6, P8, P10, PO4, PO8, O2), such that statistical analyses would transparently capture laterality and anterior–posterior distributional differences between conditions. Repeated-measures ANOVAs incorporated Geisser–Greenhouse correction when appropriate. ERP data quality was assessed by computing the signal-to-noise ratios (SNR) for each subject and condition, with signal defined as the maximum (unsigned) ERP value after stimulus onset and noise as the standard deviation of values during the pre-stimulus interval (cf. Maidhof et al., 2009). SNR values were averaged across all electrodes and between-condition comparisons were made across subjects.
Results
Behavioral Results
The magnitude of priming during the test phase was greater for objects that were studied for 250 ms (Brief) compared to objects studied for 2,000 ms (Long), whereas the opposite pattern was identified for recognition. Categorization response times varied significantly for Brief, Long, and New objects [F(2,26) = 21.7, p < 0.001], and were significantly faster both for Brief objects (586 ms, SE = 40 ms) and for Long objects (605 ms, SE = 41 ms) compared to new objects [627 ms, SE = 43 ms; t(13) = 5.5, p < 0.001, and t(13) = 4.4, p < 0.001, respectively], indicating priming for both study durations. Response times for Brief objects were significantly faster than the for Long objects [Figure 2A; t(13) = 3.2, p = 0.006], indicating greater priming magnitude for Brief objects and, thus, an inverse relationship between study duration and priming. Response times for Brief and Long objects did not differ reliably during the study phase [710 and 713 ms, respectively, t(13) = 0.26, p = 0.80], indicating that priming effects on response times during the test phase were due to repetition. Accuracy for categorization responses was high for Brief, Long, and New objects (91.2, 89.9, and 91.7%, respectively) and did not vary by condition (all pairwise p-values >0.39), indicating that speed-accuracy tradeoffs did not underlie priming effects on categorization response times.
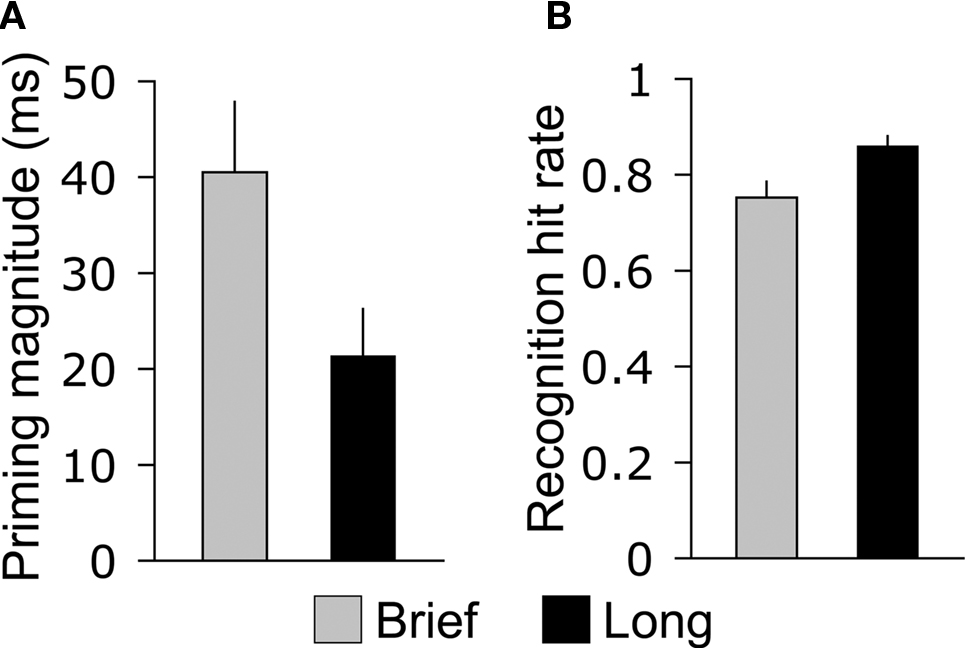
Figure 2. Opposite effects of study duration on priming and recognition. (A) The average difference between old and new objects in categorization response time during the memory test is shown for Brief and Long objects. (B) The average hit rate for the recognition response is shown for Brief and Long objects, collapsed across high- and low-confidence levels. Error bars indicate SE.
Recognition hit rates collapsed for high- and low-confidence responses differed significantly for Brief, Long, and New objects [F(2,26) = 395.7, p > 0.001], and hit rates were significantly greater for Brief objects (0.75, SE = 0.03) and for Long objects (0.86, SE = 0.02) compared to the false-alarm rate for new objects [0.13, SE = 0.02; t(13) = 18.1, p < 0.001, and t(13) = 28.2, p < 0.001, respectively]. This indicates that subjects successfully discriminated old objects of both encoding durations from new objects. Recognition hits were more prevalent for Long objects than for Brief objects [Figure 2B; t(13) = 4.8, p < 0.001], indicating a positive relationship between encoding duration and recognition. The prevalence of high-confidence and low-confidence responses also varied for Brief, Long, and New objects [F(6,78) = 134.0, p < 0.001, for the interaction of the four confidence levels with object type]. The positive relationship between encoding duration and recognition was driven by high-confidence hits, which were more prevalent for Long objects than for Brief objects [Table 1; t(13) = 6.6, p < 0.001], whereas low-confidence hits were registered at similar rates [t(13) = 1.8, p = 0.10]. Likewise, high-confidence and low-confidence misses were more prevalent for Brief objects than for Long objects [t(13) = 4.4, p < 0.001 and t(13) = 3.1, p = 0.001, respectively].
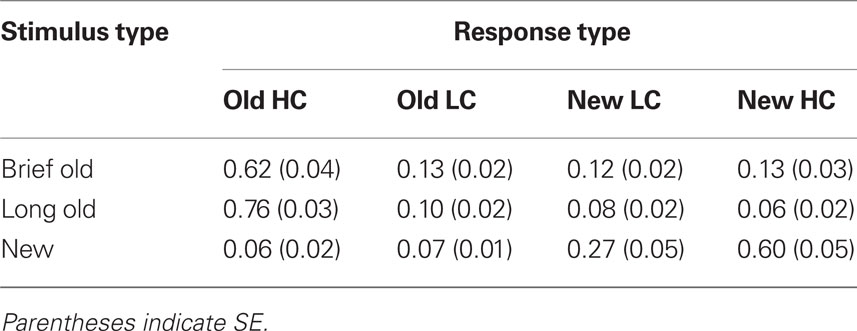
Table 1. Mean recognition endorsement rates for high confidence (HC) and low confidence (LC) responses.
Recognition response times were uninformative because they were withheld until the recognition prompt (2,500 ms after stimulus onset). Relative to the recognition prompt, response times for high- and low-confidence hits were, respectively, 365 and 412 ms for Brief objects, 566 and 299 ms for Long objects, and 479 and 415 ms for New objects (correct rejections), without no significant pairwise differences between any two conditions (p-values >0.22).
In summary, encoding duration had opposite effects on priming and recognition: brief encoding led to greater priming and worse recognition whereas Long encoding led to less priming and better recognition (Figure 2). We therefore sought to characterize ERP old/new effects that varied with encoding duration (selective for Brief versus Long) in order to identify neural correlates of priming and recognition.
ERP Results
The primary ERP analysis concerns the Brief, Long, and new object conditions, treated irrespective of recognition response type (Figure 3). SNR measures of ERP data quality for these conditions did not differ significantly (mean SNR values = 14.1, 13.8, and 13.7, respectively, F(2,26) = 0.06, p = 0.94]. Two ERP effects were evident that differentiated Brief from Long objects: (1) brief ERPs were more negative than new ERPs from approximately 200–400 ms at left parieto-occipital electrodes, and (2) long ERPs were more positive than new ERPs from approximately 400–600 ms at central–parietal electrodes. Another ERP effect was evident that was similar for Brief and Long objects: (3) brief and long ERPs were both more positive than new ERPs from approximately 300–600 ms at bilateral anterior electrodes.
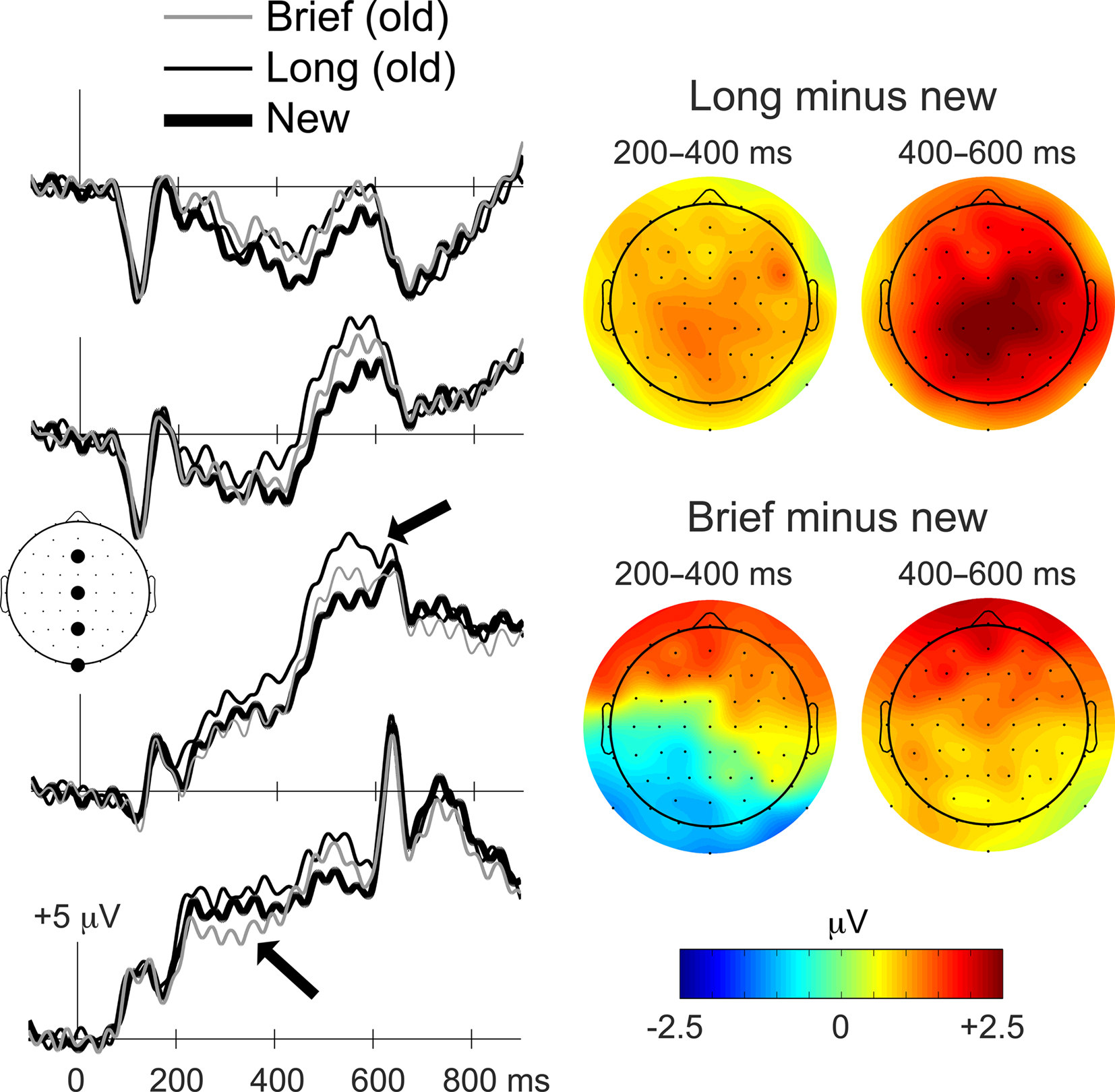
Figure 3. Distinct ERP correlates of priming and recognition. ERP waveforms during the memory test for Brief, Long, and new objects are shown on the left for the four midline electrodes indicated on the diagram of the head as viewed from above (Fz, Cz, Pz, and Iz). Waveforms are timelocked to the onset of the objects, and positive deflections are plotted upwards. Topographic distributions for old/new ERP differences are shown on the right for Brief and Long objects for 200–400 ms and 400–600 ms latency intervals. The magnitude of old minus new ERP differences is indicated by the colorscale, with red coloration indicating greater positive differences and blue coloration indicating greater negative differences. Arrows are used to highlight the ERP old/new effect attributed to recognition (upper arrow) and the effect attributed to priming (lower arrow). The distinction between ERPs attributed to priming and recognition is also apparent in the topographic maps. The upper right topographic map is characteristic of the ERP effects attributed to recognition whereas the lower left topographic map is characteristic of the ERP effects attributed to priming. A similar distinction between Brief and Long old/new effects was evident when ERPs were computed using an average scalp reference instead of the average mastoid reference used here (Figure A1 of Appendix).
These observations were confirmed using repeated-measures ANOVA, which was used to test mean amplitudes for the three conditions for the 200–400 ms and 400–600 ms latency intervals (which captured the visually evident between-condition differences described above) for six electrode clusters (anterior, central, and posterior, each on left and right scalp). A three-way interaction indicated that differences between conditions varied by latency and location [F(3.0,38.6) = 7.6, p < 0.001].
A follow-up ANOVA for the 200–400 ms interval yielded a significant condition-by-region interaction [F(3.9,51.4) < 16.2, p = 0.001], and follow-up comparisons for the three conditions were therefore performed for each cluster, yielding significant main effects for the left posterior cluster and for the left and right anterior clusters (all p-values <0.01; p-values at other clusters >0.07). A negative old/new effect for Brief objects was evident for the left posterior cluster (−0.93 mV on average, p = 0.01), whereas the old/new effect for Long objects for the same cluster was positive and of marginal significance (+0.82 mV on average, p = 0.07). The old/new effect for Brief objects was significantly more negative than for Long objects for the left posterior cluster (p < 0.001). For left and right anterior clusters, positive old/new effects were evident for both Brief and Long objects. The effects for Brief objects averaged +1.39 mV for the left cluster (p = 0.006) and +1.22 mV for the right cluster (p = 0.02), and the effects for Long objects averaged +0.87 mV for the left cluster (p = 0.007) and +0.86 mV for the right cluster (p = 0.03). The Brief and Long old/new effects did not differ reliably for either cluster (p = 0.18 and 0.46, respectively).
A follow-up ANOVA for the 400–600 ms interval yielded a significant condition-by-region interaction [F(3.2,41.5) = 3.1, p = 0.001], and follow-up comparisons for the three conditions were therefore performed for each cluster, yielding significant main effects for all clusters (p-values <0.01). For left and right anterior clusters, positive old/new effects were evident for both Brief and Long objects. The effects for Brief objects averaged +1.67 mV for the left cluster (p = 0.004) and +1.48 mV for the right cluster (p = 0.02), and the effects for Long objects averaged +1.52 for the left cluster (p < 0.001) and +1.73 for the right cluster (p = 0.001). The magnitude of the Brief and Long old/new effects did not differ reliably for either cluster (p = 0.77 and 0.67, respectively). The left and right central and posterior clusters exhibited a different pattern of old/new effects, whereby positive old/new effects were reliable for Long objects but not for Brief objects. For the central left, central right, posterior left, and posterior right clusters, the average old/new mV differences for Long objects were + 1.96 (p < 0.001), + 2.30 (p < 0.001), + 1.69 (p < 0.001), and +1.86 (p < 0.001), respectively. The same differences for Brief objects were + 1.05 (p = 0.05), + 0.97 (p = 0.10), + 0.83 (p = 0.11), and +0.64 (p = 0.24), respectively. The old/new ERP difference was significantly more positive for Long objects than for Brief objects for each of these four clusters (p = 0.03, 0.007, 0.03, and 0.004, respectively).
In summary, analyses of the 200–400 ms interval identified a negative parieto-occipital effect that was selective for Brief objects, whereas analyses of the 400–600 ms interval identified a positive central–parietal effect that was selective for Long objects. Because Brief and Long objects were differentially associated with priming and recognition, respectively, the early negative ERP effect can be attributed to priming for Brief objects and the later positive effect can be attributed to recognition for Long objects. A third ERP old/new effect was positive at bilateral anterior electrodes and spanned both latency intervals. This effect did not differ for Brief and Long objects, and therefore was not selective for priming or recognition.
The 200–400 negativity associated with repetition of Brief objects appeared to encompass a different spatial extent than did the 400–600 ms positivity associated with repetition of Long objects. We analyzed topographical differences between the two effects in order to test for whether they resulted from activity in different neural populations. Confounding influences due to variations in difference amplitudes across conditions were removed via the vector-scaling method with range normalization (McCarthy and Wood, 1985; but see Urbach and Kutas, 2002). We adopted a conservative approach by including vector-scaled amplitude values from all scalp electrodes, rather than using only a subset of electrodes at which the effects were present. This selection thus served to bias the analyses against finding topographical differences by including the subset of electrodes at which old/new effects were not observed, and therefore could not have contributed to topographic differences between the two conditions.
Comparing the 200–400 ms old/new effect for Brief objects to the 400–600 ms effect for Long objects yielded a significant condition-by-electrode interaction [F(5.4,70.0) = 10.9, p < 0.001], indicating that the topographies differed significantly. Given that old/new effects for Long objects appeared similar in topography for the 200–400 ms interval as for the 400–600 ms interval (but smaller in amplitude, Figure 3), we also tested for topographic differences between Brief and Long objects for the 200–400 ms interval. Significantly different topographies were indicated by a condition-by-electrode interaction [F(5.3,69.1) = 9.9, p < 0.001]. The same topographic differences were found when only those electrodes included in the clusters used for primary statistical assessment were tested [Brief old/new 200–400 ms versus Long old/new 400–600 ms interaction F(5.1,66.0) = 11.0, p < 0.001; Brief old/new 200–400 ms versus Long old/new 200–400 ms interaction F(5.1,66.0) = 8.6, p < 0.001]. These topographic assessments therefore suggest that old/new effects for Brief objects and for Long objects resulted from activity in different neural populations, and this dissociation is consistent with their dissociation based on polarity (negative versus positive, respectively) and functional properties (priming versus recognition, respectively).
Additional evidence that the early negative ERP effect was due to priming was obtained in an analysis that examined memory and ERPs as a function of categorization-task response times. This analysis was predicated on the assumption that faster categorization-task response times were partially due to priming, although other influences could potentially have contributed to faster response times for a subset of objects (e.g., those with features that led to rapid categorization). A within-subjects median split on categorization-task response times was used to divide old Brief, old Long, and new trials into fastest and slowest categories. The relationship between recognition and categorization-task response time was investigated by computing recognition accuracy separately for the fastest and slowest categories. Recognition responses were more accurate for the slowest versus the fastest categorization responses for Brief, Long, and new objects (Table 2), indicating that recognition and response times on the priming measure were inversely related.
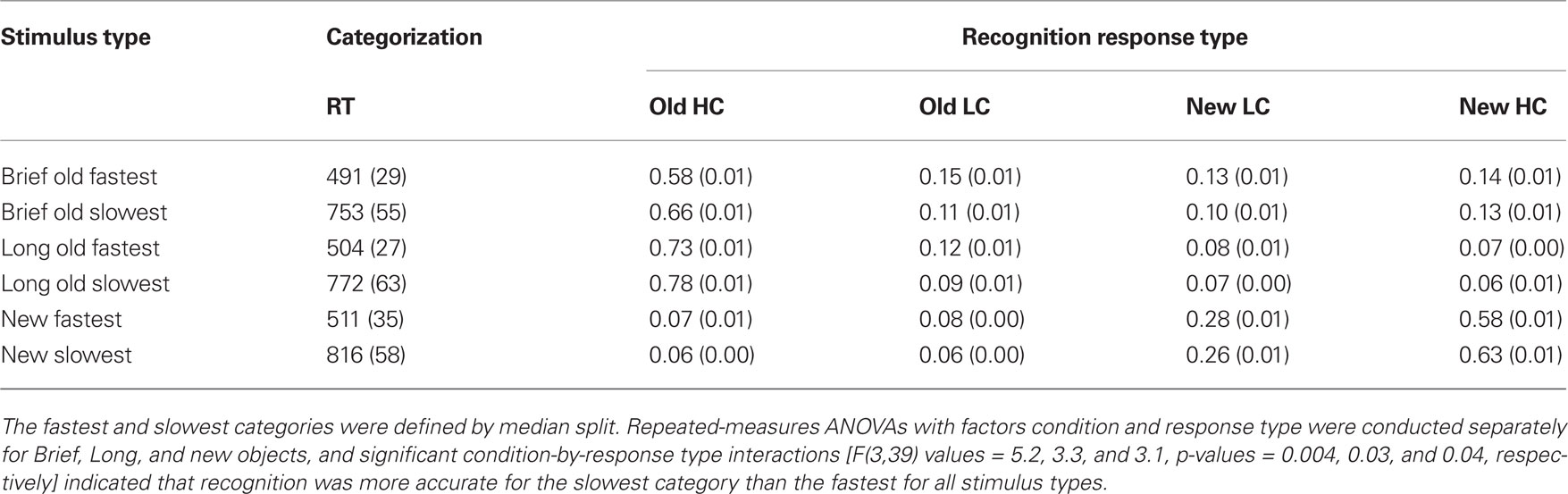
Table 2. Mean recognition endorsement rates for high confidence and low confidence responses computed as a function of response time (RT) on the priming measure (in ms).
Two ERP effects differentiated the fastest and slowest categories (Figure 4): (1) a negative parieto-occipital effect from 200 to 400 ms, similar to the effect attributed to priming for Brief objects in the primary analyses, was evident for Brief and Long objects but not new objects, and (2) a positive central effect from approximately 500–700 ms was evident for Brief, Long, and new objects. Note that SNR measures of ERP data quality did not differ significantly for the Brief fastest, Brief slowest, Long fastest, Long slowest, New fastest, and New slowest categories (mean SNR values = 10.8, 10.3, 10.2, 10.1, 10.9, and 10.7, respectively, F(5,65) = 0.27, p = 0.93].
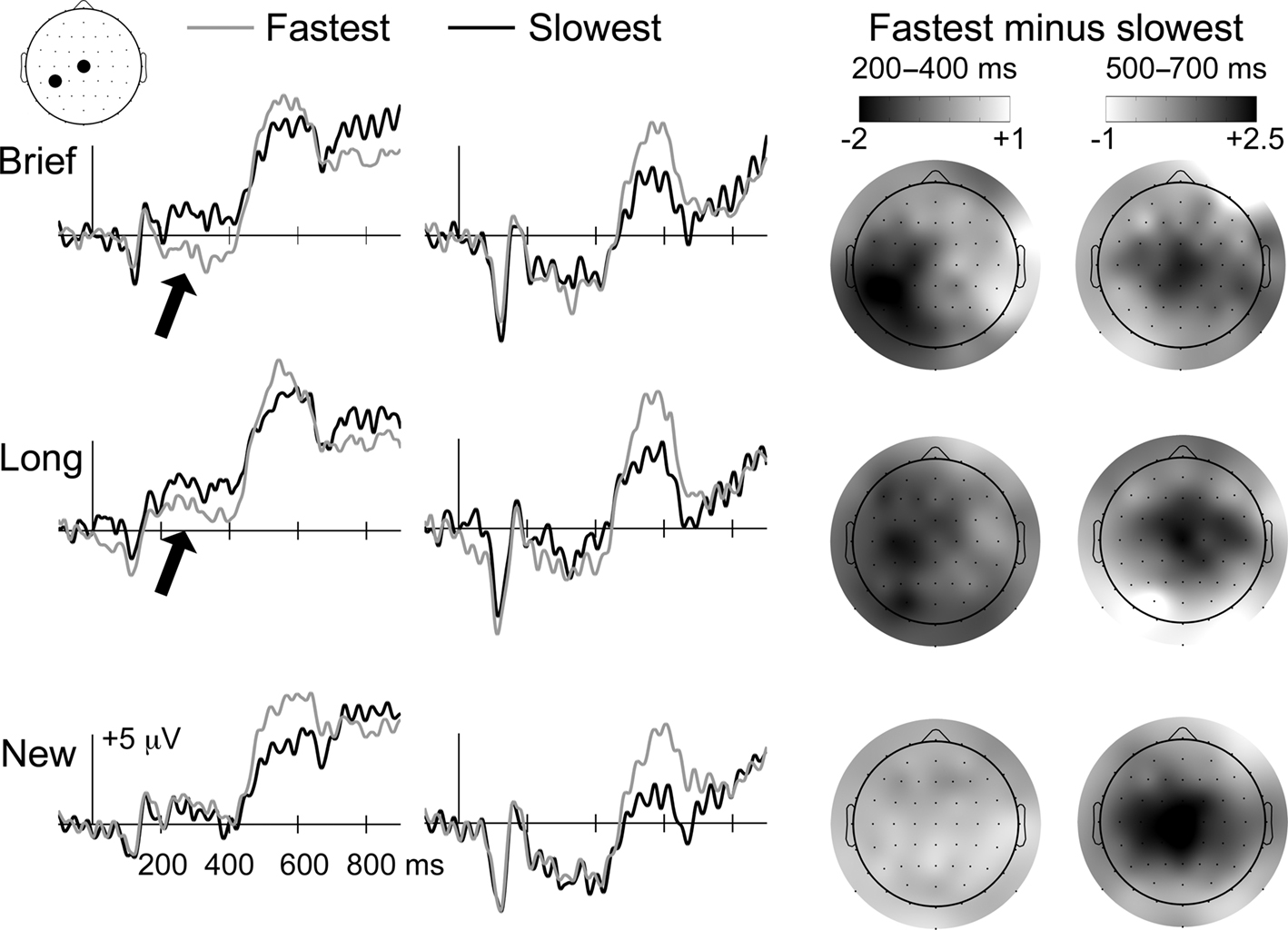
Figure 4. Priming ERP correlates scale with response times for Brief and Long objects. ERP waveforms during the memory test are shown for Brief, Long, and new objects, each subdivided into fastest and slowest categories based on median split of priming response times. Waveforms are shown for the two electrode positions indicated on the diagram of the head (CP3 and Cz). Topographic distributions for fastest/slowest ERP differences are shown on the right for Brief, Long, and new objects for 200–400 and 500–700 latency intervals. Darker colors indicate greater negative differences for 200–400 ms and greater positive differences for 500–700 ms, as indicated by the color bars. Arrows are used to highlight portions of the ERP waveforms that were attributed to priming, which was selective for Brief and Long objects. This selectivity is also apparent in the topographic maps for the 200–400 ms interval, which show negative left occipitotemporal effects for Brief and Long objects only. In contrast, the later-onset positive effect was identified for all three object types, and therefore did not reflect repetition-induced memory influences on ERPs.
Differences between the fastest and slowest categories from 200 to 400 ms were assessed separately for Brief, Long, and new objects using repeated-measures ANOVA with the six electrode clusters used in the primary analysis [a three-way interaction of study-duration condition, response-speed condition, and cluster predicated separate assessment of the Brief, Long, and new conditions, F(3.7,48.4) = 3.3, p = 0.02]. For Brief objects, a condition-by-cluster interaction indicated that differences between fastest and slowest items varied by location [F(1.6,21.0) = 4.1, p = 0.03]. Follow-up pairwise comparisons for each cluster were significant only for the left central (p = 0.03) and left posterior (p = 0.04) clusters (p-values >0.70 for other clusters). A condition-by-cluster interaction was also observed for Long objects [F(1.5,19.2) = 3.8, p = 0.04], and pairwise differences were also reliable only for the left central (p = 0.06) and left posterior (p = 0.04) clusters (p-values >0.24 for other clusters). In contrast, no fastest/slowest differences were reliable for new objects [condition main effect F(1,13) = 0.15, ns; condition-by-cluster F(5,65) = 0.31 ns; p-values >0.49 for pairwise comparisons for all clusters]. The fastest/slowest difference for Brief objects was significantly more negative than for new objects for left central (−1.5 versus +0.2 mV; p = 0.03) and left posterior (−1.5 versus +0.3 mV; p = 0.008) clusters. Likewise, the fastest/slowest difference for Long objects was more negative than for new objects for left central (−1.2 versus +0.2 mV; p = 0.07) and left posterior (−1.1 versus +0.3 mV; p = 0.06) clusters.
For the 500–700 ms interval, a three-way interaction of study-duration condition, response-speed condition, and cluster predicated separate assessment of the Brief, Long, and new conditions [F(4.5,58.5) = 2.9, p = 0.02]. The fastest category was significantly more positive than the slowest category from 500 to 700 ms for Brief, Long, and new objects [condition main effect F(1,13) values = 4.5, 4.6, and 5.0, p-values = 0.05, 0.05, and 0.04, respectively]. Non-significant condition-by-cluster interactions (p-values >0.36) indicated that, although the positive fastest/slowest difference appeared maximal at mid-central electrodes, the magnitude of the effect did not differ reliably across clusters.
The positive fastest/slowest difference from 500 to 700 ms can be attributed to preparation of motor output or other non-specific factors associated with rapid behavioral responding rather than memory because it was evident irrespective of whether objects were old or new. Furthermore, the ERP effect was opposite to what would have been expected if it were due to recognition based on results from the primary analyses (Figure 3). In other words, recognition was most accurate for the slowest category (Table 2) and ERPs were more negative for the slowest category compared to the fastest category, whereas accurate recognition for Long objects was associated with positive ERP effects (Figure 3). In contrast, the early negative fastest/slowest difference was memory-related in that it was evident for old (Brief and Long) but not new objects and closely resembled the priming effect for Brief objects identified in the primary analyses (Figure 3).
Discussion
The duration for which objects were studied had opposite behavioral effects on subsequent priming and recognition, and revealed distinct electrophysiological responses associated with each kind of memory. Objects were studied for either 250 ms (Brief) or 2 s (Long), and both priming and recognition were tested for each item during a subsequent two-stage memory test. Brief and Long items were presented for equivalent durations in the memory test (500 ms) along with new objects. The magnitude of priming was significantly greater for Brief objects than for Long objects, whereas recognition was significantly more accurate and associated with higher confidence for Long objects than for Brief objects. Note that priming and recognition were significant for both stimulus durations, underscoring the fact that these behavioral phenomena can occur for many stimulus durations, but that their magnitude varies based on manipulations of duration.
Two ERP repetition effects were distinct in time, space, and sign (positive versus negative) that paralleled the behavioral effects of study duration. An early negative repetition effect at left occipitotemporal electrodes was selective for Brief objects, and a later positive repetition effect at centroparietal electrodes was selective for Long objects. Occipitotemporal negative repetition effects from approximately 200–400 span the latencies of several ERP effects that are frequently associated with priming, including N/P150 effects (Dufau et al., 2008; Harris et al., 2009), N250 effects (Dufau et al., 2008; Neumann and Schweinberger, 2008), and N350 effects (Schendan and Maher, 2009). Zago et al. (2005) found that negative repetition effects in fMRI activity were maximal for objects studied for 250 ms (Brief) in object-sensitive visual cortex (occipitotemporal cortex and fusiform gyrus) and left inferior prefrontal cortex. Negative repetition effects in these structures have been linked to priming of perceptual representations (Schacter and Buckner, 1998a,b; Henson, 2003; Schacter et al., 2007), and might indicate repetition-related perceptual learning (Desimone, 1996; Wiggs and Martin, 1998; Schacter et al., 2007) similar to what is observed as “repetition suppression” phenomena in single unit recordings from primate visual cortex (Desimone, 1996). We propose that the early negative occipitotemporal effect identified here for Brief objects is an electrophysiological correlate of reduced activity in object-sensitive visual cortex identified previously under comparable circumstances as a neural correlate of perceptual priming (Zago et al., 2005), although neurophysiological recordings with higher spatial resolution would be required to confirm this interpretation.
Centroparietal positive repetition effects at subsequent latencies, similar to those found here from 400 to 600 ms in conjunction with recognition of Long objects, have been consistently associated with high-confidence recognition and the experience of recollection during episodic memory tests, and have been variably termed the “late positive old/new effect” or the “late positive complex” (Friedman and Johnson, 2000; Rugg and Curran, 2007; Voss and Paller, 2008a,b). For instance, the magnitude of late positive old/new effects correlate with the amount of information retrieved during a recognition test (Wilding, 2000) and with subjects’ confidence in recognition decisions (Voss and Paller, 2009b). These effects might reflect parietal-lobe contributions to episodic memory (Wagner et al., 2005; Olson and Berryhill, 2009) and/or interactions between parietal cortex and MTL in the service of explicit memory (Vincent et al., 2006; Kesner, 2009), although it is currently unclear if parietal ERPs are generated by parietal cortical activity, parietal–MTL interactions, or activity elsewhere.
The distinction that we identified between early occipitotemporal negative repetition effects and later centroparietal positive repetition effects is therefore consistent with neural correlates of priming and recognition described in the extant literature. Taken together with the behavioral finding that study duration had opposite effects on priming (greater for shorter durations) than on recognition (greater for longer durations), our electrophysiological findings indicate that the neural processes that gave rise to priming versus recognition were differentially sensitive to study duration, and are consistent with findings in amnesic patients that suggest distinct neuroanatomical substrates for priming and recognition (Gabrieli, 1998; Squire, 2004). By showing that a single manipulation can have opposite effects on behavioral and neural correlates of priming and recognition, the current results are strongly suggestive of a “double-dissociation” between priming and recognition in healthy individuals. It is worth noting that the crossover dissociation between priming and recognition we observed in the behavioral data do not meet the strict criteria for a “reversed association” (Dunn and Kirsner, 1988), and thus do not allow us to completely exclude a single-process explanation for the data. Nonetheless, the data, both behavioral and neural, are more consistent with a double-dissociation between priming and recognition, and thus provide some of the strongest evidence to date that priming and recognition do rely on distinct neural substrates.
One potential limitation of the behavioral paradigm that we employed is that the priming judgment always preceded the recognition judgment during the test, and this contingency could have influenced the relationship between priming and recognition. However, it is important to note that the main behavioral findings – priming increase for Brief versus Long study and recognition increase for Long versus Brief study – have been previously elicited when only one type of response or the other was required (reviewed in the Introduction). Therefore, it is unlikely that the behavioral response requirements that we introduced were alone responsible for the behavioral dissociation we identified between priming and recognition. Furthermore, it is unclear that reversing the order of the recognition and priming responses would yield meaningful results, given that subjects would presumably categorize objects in the course of making a recognition response, thus interfering with detection of priming on the categorization response. In contrast to effects on behavioral correlates of recognition, opposite effects of study duration on ERP measures occurred within the first second after stimulus onset, before the recognition response was made, providing further support for the notion that the order of the behavioral response was inconsequential.
Despite the fact that study duration had robust effects on behavioral and neural correlates of priming and recognition, the precise mechanisms for these effects are currently obscure. However, there are several reasonable mechanisms based on the known properties of each memory phenomenon. For example, Zago et al. (2005) propose that study duration of approximately 250 ms is ideal for later priming based on the time-varying involvement of neural “sharpening” and “selection” processes that are part of widely accepted mechanistic accounts of priming (Desimone, 1996; Wiggs and Martin, 1998), as explained above. Another possibility, consistent with transfer-appropriate processing accounts of memory (Lockhart, 2002), is that brief study enhances later priming because both similarly involve rapid stimulus identification. In contrast, longer study durations might aid later recognition by providing time to engage a variety of processes known to aid recognition memory, such as identifying and encoding the most distinctive stimulus features (Gallo et al., 2008), semantically elaborating the stimulus (Craik and Lockhart, 1972; Craik and Tulving, 1975), and integrating the stimulus with the experimental context (Fabiani and Donchin, 1995). Unfortunately, the nature of the duration manipulation complicates analyses of study-time neural mechanisms due to the inherent confound of stimulus duration. However, these various possibilities could be evaluated by comparing their effects on neural processing during retrieval to those of duration.
The current findings add to the substantial literature showing that the way in which a stimulus is initially processed has profound effects on its retention and retrieval (Craik and Lockhart, 1972; Craik and Tulving, 1975; Paller and Wagner, 2002). A general trend in previous studies is that more elaborate encoding or more attention to stimuli during study increases explicit recognition and recall but has little or no effect on implicit measures of memory (Schacter and McGlynn, 1989; Mulligan, 1998; Rajaram et al., 2001). Implicit measures are generally more sensitive to the precise nature of the representation initially activated, for example, showing modulations due to unimodal versus multimodal representations of the same objects (Murray et al., 2004, 2005; von Kriegstein and Giraud, 2006). To the best of our knowledge, our findings comprise the first evidence that an encoding manipulation can exert opposite effects on these two behavioral indices of memory and promote the differential engagement of implicit versus explicit memory systems. The current findings complement those of Schott et al. (2002, 2006) who found that different neural events during encoding predicted subsequent priming versus recognition, again suggesting different neural substrates for the two kinds of memory.
What then is the nature of the relationship between the distinct neural systems that support implicit versus explicit memory? Some findings indicate that perceptual and conceptual fluency, which support priming, can influence explicit recognition decisions (e.g., Verfaellie and Cermak, 1999; Wolk et al., 2005), indicating an influence of implicit on explicit memory systems. However, the current findings suggest that the relationship between implicit and explicit memory may be competitive, in that recognition was poorest for trials showing the greatest priming, and vice versa (Table 2; Figure 4; see also Wagner et al., 2000). Likewise, Schott et al. (2002, 2006) found that encoding activity predicted priming versus recognition, suggesting variability in the selective engagement of either an implicit memory system or an explicit memory system. Other findings have suggested a similar competitive relationship between the explicit memory system and the striatal system for implicit habit and categorization learning (e.g., Packard et al., 1989; Foerde et al., 2006; Frank et al., 2006; Nomura et al., 2007). It is likely that various experimental parameters and subject factors collectively determine the relative contributions of implicit and explicit memory systems to the measured behavior (cf., Voss and Paller, 2009a), leading to symbiotic interactions between implicit and explicit memory in some circumstances and competitive interactions in other circumstances. Future studies should consider the determinants of interactions between distinct implicit and explicit memory systems. This endeavor will likely benefit from characterizations of the interplay between the neural processes at encoding and retrieval (e.g., Rugg et al., 2008) that determine how memory is behaviorally expressed and phenomenologically experienced.
Conflict of Interest Statement
The authors declare that the research was conducted in the absence of any commercial or financial relationships that could be construed as a potential conflict of interest.
Acknowledgments
We thank Kara Federmeier, Rik Henson, and Ken Paller for their suggestions on an earlier version of this manuscript. Research support was provided by a Beckman Institute Postdoctoral Fellowship award to Joel L. Voss.
Footnote
- ^It should be noted that this paradigm is suitable for assessing relationships between priming and recognition in the visual modality, but that other methods would be needed to answer similar questions with respect to priming in other sensory systems (i.e., auditory, tactile, etc.).
References
Berry, C. J., Shanks, D. R., and Henson, R. N. (2008a). A single-system account of the relationship between priming, recognition, and fluency. J. Exp. Psychol. Learn. Mem. Cogn. 34, 97–111.
Berry, C. J., Shanks, D. R., and Henson, R. N. (2008b). A unitary signal-detection model of implicit and explicit memory. Trends Cogn. Sci. 12, 367–373.
Chatrian, G. E., Lettich, E., and Nelson, P. L. (1988). Modified nomenclature for the “10%” electrode system. J. Clin. Neurophysiol. 5, 183–186.
Craik, F. I. M., and Lockhart, R. S. (1972). Levels of processing: a framework for memory research. J. Verbal Learn. Verbal Behav. 11, 671–684.
Craik, F. I. M., and Tulving, E. (1975). Depth of processing and the retention of words in episodic memory. J. Exp. Psychol. Gen. 104, 268–294.
Desimone, R. (1996). Neural mechanisms for visual memory and their role in attention. Proc. Natl. Acad. Sci. U.S.A. 93, 13494–13499.
Dufau, S., Grainger, J., and Holcomb, P. J. (2008). An ERP investigation of location invariance in masked repetition priming. Cogn. Affect. Behav. Neurosci. 8, 222–228.
Dunn, J. C., and Kirsner, K. (1988). Discovering functionally independent mental processes: the principle of reversed association. Psychol. Rev. 95, 91–101.
Ellis, H. D., Davies, G. M., and Shepherd, J. W. (1977). Experimental studies of face recognition. Natl. J. Crim. Def. 3, 219–234.
Fabiani, M., and Donchin, E. (1995). Encoding processes and memory organization: a model of the Von Restorff effect. J. Exp. Psychol. Learn. Mem. Cogn. 21, 224–240.
Foerde, K., Knowlton, B. J., and Poldrack, R. A. (2006). Modulation of competing memory systems by distraction. Proc. Natl. Acad. Sci. U.S.A. 103, 11778–11783.
Frank, M. J., O’Reilly, R. C., and Curran, T. (2006). When memory fails, intuition reigns: midazolam enhances implicit inference in humans. Psychol. Sci. 17, 700–707.
Friedman, D., and Johnson, R. Jr. (2000). Event-related potential (ERP) studies of memory encoding and retrieval: a selective review. Microsc. Res. Tech. 51, 6–28.
Gallo, D. A., Perlmutter, D. H., Moore, C. D., and Schacter, D. L. (2008). Distinctive encoding reduces the Jacoby–Whitehouse illusion. Mem. Cognit. 36, 461–466.
Hamann, S. B., and Squire, L. R. (1997). Intact perceptual memory in the absence of conscious memory. Behav. Neurosci. 111, 850–854.
Harris, J. D., Cutmore, T. R., O’Gorman, J., Finnigan, S., and Shum, D. (2009). Neurophysiological indices of perceptual object priming in the absence of explicit recognition memory. Int. J. Psychophysiol. 71, 132–141.
Henson, R. N. (2005). A mini-review of fMRI studies of human medial temporal lobe activity associated with recognition memory. Q. J. Exp. Psychol. B 58, 340–360.
Henson, R. N. (2006). Forward inference using functional neuroimaging: dissociations versus associations. Trends Cogn. Sci. 10, 64–69.
Henson, R. N., Hornberger, M., and Rugg, M. D. (2005). Further dissociating the processes involved in recognition memory: an FMRI study. J. Cogn. Neurosci. 17, 1058–1073.
Kesner, R. P. (2009). The posterior parietal cortex and long-term memory representation of spatial information. Neurobiol. Learn. Mem. 91, 197–206.
Kinder, A., and Shanks, D. R. (2003). Neuropsychological dissociations between priming and recognition: a single-system connectionist account. Psychol. Rev. 110, 728–744.
Kroll, N. E., Yonelinas, A. P., Kishiyama, M. M., Baynes, K., Knight, R. T., and Gazzaniga, M. S. (2003). The neural substrates of visual implicit memory: do the two hemispheres play different roles? J. Cogn. Neurosci. 15, 833–842.
Lockhart, R. S. (2002). Levels of processing, transfer-appropriate processing, and the concept of robust encoding. Memory 10, 397–403.
Loftus, G. R., and Kallman, H. J. (1979). Encoding and use of detail information in picture recognition. J. Exp. Psychol. Hum. Learn. Mem. 5, 197–211.
Maidhof, C., Rieger, M., Prinz, W., and Koelsch, S. (2009). Nobody is perfect: ERP effects prior to performance errors in musicians indicate fast monitoring processes. PLoS ONE 4, e5032. doi: 10.1371/journal.pone.0005032.
McCarthy, G., and Wood, C. C. (1985). Scalp distributions of event-related potentials: an ambiguity associated with analysis of variance models. Electroencephalogr. Clin. Neurophysiol. 62, 203–208.
Mulligan, N. W. (1998). The role of attention during encoding in implicit and explicit memory. J. Exp. Psychol. Learn. Mem. Cogn. 24, 27–47.
Murray, M. M., Foxe, J. J., and Wylie, G. R. (2005). The brain uses single-trial multisensory memories to discriminate without awareness. Neuroimage 27, 473–478.
Murray, M. M., Michel, C. M., Grave de Peralta, R., Ortigue, S., Brunet, D., Gonzalez Andino, S., and Schnider, A. (2004). Rapid discrimination of visual and multisensory memories revealed by electrical neuroimaging. Neuroimage 21, 125–135.
Neumann, M. F., and Schweinberger, S. R. (2008). N250r and N400 ERP correlates of immediate famous face repetition are independent of perceptual load. Brain Res. 1270, 64–77.
Nomura, E. M., Maddox, W. T., Filoteo, J. V., Ing, A. D., Gitelman, D. R., Parrish, T. B., Mesulam, M. M., and Reber, P. J. (2007). Neural correlates of rule-based and information- integration visual category learning. Cereb. Cortex 17, 37–43.
Olson, I. R., and Berryhill, M. (2009). Some surprising findings on the involvement of the parietal lobe in human memory. Neurobiol. Learn. Mem. 91, 155–165.
Packard, M. G., Hirsh, R., and White, N. M. (1989). Differential effects of fornix and caudate nucleus lesions on two radial maze tasks: evidence for multiple memory systems. J. Neurosci. 9, 1465–1472.
Paller, K. A., Hutson, C. A., Miller, B. B., and Boehm, S. G. (2003). Neural manifestations of memory with and without awareness. Neuron 38, 507–516.
Paller, K. A., and Wagner, A. D. (2002). Observing the transformation of experience into memory. Trends Cogn. Sci. 6, 93–102.
Rajaram, S., Srinivas, K., and Travers, S. (2001). The effects of attention on perceptual implicit memory. Mem. Cogn. 29, 920–930.
Reder, L. M., Park, H., and Kieffaber, P. D. (2009). Memory systems do not divide on consciousness: reinterpreting memory in terms of activation and binding. Psychol. Bull. 135, 23–49.
Richardson-Klavehn, A., and Bjork, R. A. (1988). Measures of memory. Annu. Rev. Psychol. 39, 475–543.
Roediger, H. L. 3rd. (1990). Implicit memory. retention without remembering. Am. Psychol. 45, 1043–1056.
Rugg, M. D., and Curran, T. (2007). Event-related potentials and recognition memory. Trends Cogn. Sci. 11, 251–257.
Rugg, M. D., Johnson, J. D., Park, H., and Uncapher, M. R. (2008). Encoding-retrieval overlap in human episodic memory: a functional neuroimaging perspective. Prog. Brain Res. 169, 339–352.
Rugg, M. D., Mark, R. E., Walla, P., Schloerscheidt, A. M., Birch, C. S., and Allan, K. (1998). Dissociation of the neural correlates of implicit and explicit memory. Nature 392, 595–598.
Schacter, D. L., and Buckner, R. L. (1998a). On the relations among priming, conscious recollection, and intentional retrieval: evidence from neuroimaging research. Neurobiol. Learn. Mem. 70, 284–303.
Schacter, D. L., and McGlynn, S. M. (1989). Implicit memory: effects of elaboration depend on unitization. Am. J. Psychol. 102, 151–181.
Schacter, D. L., Wig, G. S., and Stevens, W. D. (2007). Reductions in cortical activity during priming. Curr. Opin. Neurobiol. 17, 171–176.
Schendan, H. E., and Maher, S. M. (2009). Object knowledge during entry-level categorization is activated and modified by implicit memory after 200 ms. Neuroimage 44, 1423–1438.
Schott, B. H., Henson, R. N., Richardson-Klavehn, A., Becker, C., Thoma, V., Heinze, H. J., and Duzel, E. (2005). Redefining implicit and explicit memory: the functional neuroanatomy of priming, remembering, and control of retrieval. Proc. Natl. Acad. Sci. U.S.A. 102, 1257–1262.
Schott, B. H., Richardson-Klavehn, A., Heinze, H. J., and Duzel, E. (2002). Perceptual priming versus explicit memory: dissociable neural correlates at encoding. J. Cogn. Neurosci. 14, 578–592.
Schott, B. H., Richardson-Klavehn, A., Henson, R. N., Becker, C., Heinze, H. J., and Duzel, E. (2006). Neuroanatomical dissociation of encoding processes related to priming and explicit memory. J. Neurosci. 26, 792–800.
Shimamura, A. P. (1986). Priming effects in amnesia: evidence for a dissociable memory function. Q. J. Exp. Psychol. A 38, 619–644.
Squire, L. R. (2004). Memory systems of the brain: a brief history and current perspective. Neurobiol. Learn. Mem. 82, 171–177.
Tversky, B., and Sherman, T. (1975). Picture memory improves with longer on time and off time. J. Exp. Psychol. Hum. Learn. Mem. 1, 114–118.
Urbach, T. P., and Kutas, M. (2002). The intractability of scaling scalp distributions to infer neuroelectric sources. Psychophysiology 39, 791–808.
Verfaellie, M., and Cermak, L. S. (1999). Perceptual fluency as a cue for recognition judgments in amnesia. Neuropsychology 13, 198–205.
Vincent, J. L., Snyder, A. Z., Fox, M. D., Shannon, B. J., Andrews, J. R., Raichle, M. E., and Buckner, R. L. (2006). Coherent spontaneous activity identifies a hippocampal-parietal memory network. J. Neurophysiol. 96, 3517–3531.
von Kriegstein, K., and Giraud, A. L. (2006). Implicit multisensory associations influence voice recognition. PLoS Biol. 4, e326. doi: 10.1371/journal.pbio.0040326.
Voss, J. L., and Paller, K. A. (2008a). Brain substrates of implicit and explicit memory: the importance of concurrently acquired signals of both memory types. Neuropsychologia 46, 3021–3029.
Voss, J. L., and Paller, K. A. (2008b). “Neural substrates of remembering: electroencephalographic studies,” in Learning and Memory: A Comprehensive Reference, Vol. 3, ed. J. Byrne (Oxford: Elsevier), 79–97.
Voss, J. L., and Paller, K. A. (2009a). An electrophysiological signature of unconscious recognition memory. Nat. Neurosci. 12, 349–355.
Voss, J. L., and Paller, K. A. (2009b). Remembering and knowing: electrophysiological distinctions at encoding but not retrieval. Neuroimage 46, 280–289.
Wagner, A. D., Maril, A., and Schacter, D. L. (2000). Interactions between forms of memory: when priming hinders new episodic learning. J. Cogn. Neurosci. 12, 52–60.
Wagner, A. D., Shannon, B. J., Kahn, I., and Buckner, R. L. (2005). Parietal lobe contributions to episodic memory retrieval. Trends Cogn. Sci. 9, 445–453.
Warrington, E. K., and Weiskrantz, L. (1970). The effect of prior learning on subsequent retention in amnesic patients. Neuropsychologia 12, 419–428.
Wiggs, C. L., and Martin, A. (1998). Properties and mechanisms of perceptual priming. Curr. Opin. Neurobiol. 8, 227–233.
Wilding, E. L. (2000). In what way does the parietal ERP old/new effect index recollection? Int. J. Psychophysiol. 35, 81–87.
Wolk, D. A., Schacter, D. L., Berman, A. R., Holcomb, P. J., Daffner, K. R., and Budson, A. E. (2005). Patients with mild Alzheimer’s disease attribute conceptual fluency to prior experience. Neuropsychologia 43, 1662–1672.
Yonelinas, A. P. (2001). Consciousness, control, and confidence: the 3 Cs of recognition memory. J. Exp. Psychol. Gen. 130, 361–379.
Keywords: recognition, priming, implicit memory, explicit memory, memory systems
Citation: Voss JL and Gonsalves BD (2010) Time to go our separate ways: opposite effects of study duration on priming and recognition reveal distinct neural substrates. Front. Hum. Neurosci. 4:227. doi: 10.3389/fnhum.2010.00227
Received: 06 August 2010;
Accepted: 26 November 2010;
Published online: 13 December 2010.
Edited by:
Francisco Barcelo, University of Illes Balears, SpainReviewed by:
Micah M. Murray, Université de Lausanne, SwitzerlandFrancisco Barcelo, University of Illes Balears, Spain
Copyright: © 2010 Voss and Gonsalves. This is an open-access article subject to an exclusive license agreement between the authors and the Frontiers Research Foundation, which permits unrestricted use, distribution, and reproduction in any medium, provided the original authors and source are credited.
*Correspondence: Joel L. Voss, Beckman Institute for Advanced Science and Technology, 405 N Mathews Avenue, Urbana, IL 61801, USA. e-mail: joelvoss@illinois.edu