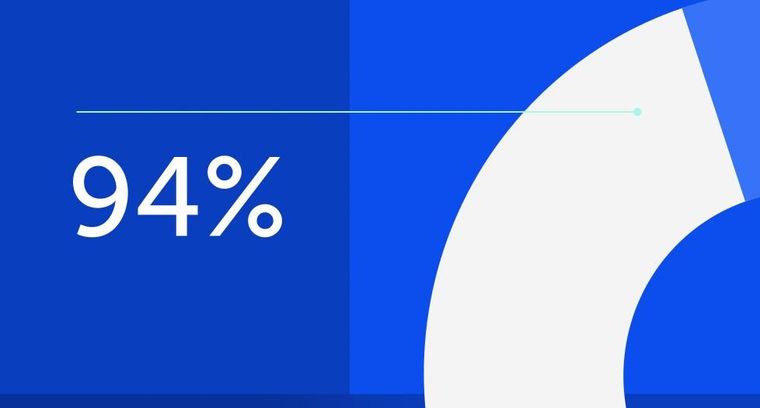
94% of researchers rate our articles as excellent or good
Learn more about the work of our research integrity team to safeguard the quality of each article we publish.
Find out more
ORIGINAL RESEARCH article
Front. Hum. Dyn., 13 February 2025
Sec. Digital Impacts
Volume 6 - 2024 | https://doi.org/10.3389/fhumd.2024.1487671
Introduction: The primary objective of the current paper is to understand the impact of Generative AI Tools on the recruitment process, on their effectiveness in addressing bias, enhancing efficiency, and ensuring accurate candidate evaluation and looking at the moderating role of familiarity and the mediating role of the size of the organization and level of employee.
Methods: A quantitative survey approach, with 469 professionals participating in an online survey, was used. Structural Equation Modelling (SEM) in Amos SPSS was used in the analysis of the relationships between Generative AI Tools, User Familiarity with AI, and key outcomes in the recruitment process.
Results: The study reveals a significant reduction in bias during candidate screening, attributed to the algorithmic objectivity, data driven decision making, and consistency inherent in Generative AI Tools. Efficiency gains and heightened accuracy in shortlisting candidates were also observed. However, User Familiarity with AI emerged as a moderating factor in influencing the relationship between Generative AI Tools and efficiency improvement.
Discussion: As a recommendation, organizations are encouraged to invest in continuous training programs to harness the full potential of Generative AI Tools in optimizing efficiency and ensuring a fair and accurate recruitment process.
The impact of generative artificial intelligence (AI), particularly large language models such as ChatGPT, on recruitment has become a subject of growing interest as organizations increasingly leverage AI driven tools to streamline recruitment and improve candidate quality. Recruitment processes have traditionally involved significant manual labor, such as drafting job descriptions, screening resumes, and conducting initial candidate outreach according to Mohamed (2022). These activities are both time consuming and prone to human bias, which can lead to inconsistent results. With the integration of generative AI into recruitment workflows, organizations aim to increase efficiency, improve the quality of candidates, and ultimately reduce time to hire, allowing recruiters to focus on higher value tasks (Brynjolfsson and McAfee, 2022). The ability of generative AI to analyze vast datasets, process natural language, and interact with candidates has begun to transform recruitment practices in meaningful ways, making AI an invaluable tool for modern human resources (HR) operations (Davenport and Ronanki, 2023).
Generative AI applications like ChatGPT offer significant efficiency advantages by automating many of the repetitive tasks involved in recruitment. For instance, AI systems can quickly scan, sort, and rank resumes, identifying candidates who most closely match a given job description’s requirements. This automation reduces the time required to process large volumes of applications, particularly useful for highvolume recruitment, allowing recruiters to focus on strategic decision making and candidate engagement (García-Morales et al., 2023). Additionally, AI driven automation helps streamline communication with candidates, as chat bots and automated emails provide prompt responses to frequently asked questions, thereby enhancing the candidate experience. This efficient, prompt interaction contributes to a positive perception of the organization, which is crucial in a competitive hiring environment (Kaplan and Haenlein, 2022).
Another key advantage of generative AI in recruitment is its potential to improve the quality of candidates selected. Through machine learning algorithms, ChatGPT and similar AI models, evaluate candidate qualifications and experience more objectively than human recruiters, reducing bias and promoting diversity in the workforce (Chen et al., 2022). Generative AI models are programmed to evaluate resumes and application materials consistently, applying the same criteria to all candidates, which minimizes the risk of subjective biases that can creep into manual evaluations. Furthermore, some AI tools are capable of analyzing candidates’ soft skills and personality traits by examining written responses, thereby offering deeper insights into candidates’ cultural fit and alignment with organizational values (Liao and Tong, 2023). By improving both the accuracy and objectivity of candidate assessments, AI driven systems can enhance candidate quality and contribute to better long-term hiring outcomes for organizations according to Registre and Saba (2024).
The level of process automation within an organization, however, influences the relationship between generative AI usage and recruitment outcomes. Process automation mediates the impact of AI on recruitment efficiency by enabling AI to handle multiple stages of the hiring process, including prescreening, skills testing, interview scheduling, and feedback collection. The higher the level of automation, the greater the efficiency gains, as human intervention is minimized in routine tasks, allowing recruitment teams to focus on strategic roles (Manyika et al., 2017). Studies indicate that organizations with high levels of automation in recruitment experience faster cycle times, reduced costs, and higher consistency in hiring outcomes (Levy and Murnane, 2022). Automation enhances AI’s ability to scale recruitment efforts effectively, which is especially valuable during periods of high demand or for organizations with extensive recruitment needs.
Organizational size also plays a moderating role in how generative AI affects recruitment efficiency and candidate quality. Large organizations, which often manage a more extensive recruitment pipeline, are better positioned to invest in advanced AI systems that handle high volumes of applications and offer sophisticated analytical capabilities (Brynjolfsson and McAfee, 2022). For example, large organizations often require specialized roles across multiple departments, creating a demand for high quality candidate matching. AI driven automation allows these firms to quickly filter through candidates and identify those who best align with complex job requirements. Additionally, larger firms tend to have more structured recruitment frameworks and dedicated teams to oversee AI implementation, which enables them to fully leverage AI’s capabilities and achieve optimal recruitment efficiency (Demirkan and Delen, 2023). In contrast, smaller organizations may not experience as significant an impact from AI in recruitment due to their lower recruitment volumes and simpler hiring needs, which do not necessitate the extensive application of AI driven process automation.
According to Shyr et al. (2024), the implementation of generative AI in recruitment thus holds significant promise for organizations, providing substantial gains in recruitment efficiency and candidate quality. By automating key recruitment tasks and offering enhanced data analysis capabilities, generative AI allows recruiters to identify high quality candidates quickly and reduce the time to hire, leading to more efficient operations and better hiring outcomes (Srinivasan and Kim, 2023). However, the full extent of these benefits is mediated by the level of process automation within an organization and moderated by organizational size. Larger organizations with high levels of automation are more likely to realize the maximum potential of AIdriven recruitment, while smaller firms may derive only limited benefits from these technologies. In an increasingly competitive job market, understanding these dynamics provides a valuable perspective on the strategic implementation of AI in HR practices, supporting organizations in their efforts to enhance recruitment efficiency, attract top talent, and ultimately build a more skilled and diverse workforce (Burtch et al., 2023).
According to the TOE framework, an organization’s adoption of new technologies is influenced by three contextual factors: technology, organization, and environment. Numerous technological, industrial, and national contexts have shown the explanatory power of the TOE model (Malik et al., 2021; Nguyen et al., 2022).
Technological contexts encompass the attributes and accessibility of current and developing technologies that are essential to an organization (Mäkipää et al., 2022). Later research has expanded the TOE model and added reliable constructs, despite the fact that the original model does not provide descriptive details of technological factors (Nguyen et al., 2022). Relative advantage and technology complexity are considered technological factors in this study because they are highly relevant and have been used most frequently in previous research (Mndzebele, 2013). Relative advantage generally refers to the extent to which innovation can benefit an organization. As a result, researchers discover that relative advantage influences the adoption of technology at higher levels (Ekong et al., 2012). According to scholars of artificial intelligence and early adopters in the field, AI has a great deal of potential to gain a comparative advantage by carrying out complex tasks in the same way that human recruiters do (Hamm and Klesel, 2021).
Previous IS research (e.g., Hsu et al., 2006; Oliveira and Martins, 2010) measures organizational context in the TOE model using a variety of proxies. Organization size and position level are two of the most widely used proxies of organizational context that is identified in the present study. One of the organizational contextual factors that is most commonly discussed is company size (Baker, 2011; Oliveira and Martins, 2010; Zhu et al., 2006). Numerous studies (Baker, 2011; Hsu et al., 2006; Rogers, 2003; Wang et al., 2010) have found that the size of the organization has a positive impact on technology adoption.
In addition to the TOE Framework, the current study employs the Unified Theory of Acceptance and Use of Technology (UTAUT) to explore the factors influencing the impact of Generative AI Tools on bias reduction, efficiency improvement, and accuracy in candidate screening within the recruitment process. According to UTAUT, behavioral intentions (BI) are a key predictor of actual technology use (Venkatesh et al., 2003). In this context, performance expectancy, which refers to an individual’s belief that using a system will enhance job performance, is a crucial determinant of BI. However, intentions alone may not always result in actual use due to other influencing factors (Sheppard et al., 1998). Additionally, performance expectancy may not fully capture all user expectations of AI tools, especially in regulated sectors. AlQudah et al. (2021) suggest using benefit expectations (BE), a more comprehensive construct encompassing performance, usefulness, and applicability, to better capture user expectations in such contexts. This study investigates how user familiarity with AI and organizational factors, such as Level of Position and Size of the Organization, moderate and mediate the effectiveness of Generative AI Tools in recruitment, aligned with UTAUT’s emphasis on understanding the determinants of technology adoption and use.
The potential for generative AI to transform recruitment efficiency lies in its ability to automate labor-intensive processes. Recruitment often involves time consuming tasks such as resume screening, job description generation, interview scheduling, and initial candidate communication—all of which generative AI can streamline (Brynjolfsson and McAfee, 2022). For instance, natural language processing (NLP) algorithms within AI powered systems can scan and categorize resumes much faster than human recruiters, significantly reducing processing time and allowing recruitment teams to focus on strategic decision making (Davenport and Ronanki, 2023). This efficiency extends to candidate interactions, where AI driven chat bots provide immediate responses to inquiries, enhancing the candidate experience and reducing the workload on human recruiters (Kaplan and Haenlein, 2022).
Another aspect of efficiency is seen in AI’s capacity for data analysis. Recruitment teams must assess numerous metrics to identify top candidates and refine their strategies. AI systems can analyze data on candidate engagement, success rates of past hires, and the effectiveness of different recruitment channels, providing insights that would take much longer to compile manually (García-Morales et al., 2023). Additionally, generative AI aids in generating tailored job descriptions that had better attract qualified candidates by aligning with industry specific language and requirements. This targeted approach not only accelerates recruitment but also improves the quality of applicants attracted to the position, ultimately resulting in a more efficient recruitment cycle (Liao and Tong, 2023).
Generative AI also improves efficiency by reducing bias in preliminary selection processes, ensuring a more diverse and extensive talent pool from the outset (Chen et al., 2022). By automating initial steps and providing unbiased evaluations, AI increases both the speed and fairness of hiring processes, leading to better matches and less need for repeat recruitment cycles. Therefore, the use of generative AI not only reduces recruitment cycle time but also streamlines complex workflows, allowing recruiters to allocate resources more effectively and meet organizational demands efficiently (Abdelhay et al., 2023). We propose that
H1: Generative AI usage in recruitment has a positive impact on recruitment efficiency.
According to Lippert (2024), The quality of candidates identified through recruitment is crucial for long-term organizational success, and generative AI significantly contributes to this by refining the candidate matching process. Generative AI applications, particularly those employing machine learning algorithms, improve candidate quality by analyzing and cross-referencing candidates’ skills, experience, and educational background against job requirements with a level of precision unattainable through traditional methods (Liao and Tong, 2023). This depth of analysis helps recruitment teams avoid costly mismatches, as candidates who are better suited to the role are more likely to succeed and contribute meaningfully to the organization (Chen et al., 2022).
Generative AI’s data driven insights also allow recruiters to tailor candidate selection criteria based on proven indicators of success for specific roles, leveraging historical data on high performing employees to assess potential candidates. By integrating this level of sophistication, AI enhances the objectivity of candidate evaluations, as it applies consistent criteria that minimize subjective biases that often affect traditional recruitment processes (Srinivasan and Kim, 2023). For example, generative AI can objectively assess whether a candidate’s prior experience aligns with key performance indicators (KPIs) associated with success in a given position, thus helping recruiters make data backed decisions (Budhwar et al., 2023).
Moreover, generative AI can assess cultural fit by analyzing soft skills and personality traits in ways that go beyond resume qualifications. Some advanced AI tools evaluate online profiles, past project work, and other publicly available information to construct a well-rounded candidate profile, resulting in a higher probability of a strong organizational fit (García-Morales et al., 2023). This comprehensive approach to candidate assessment positively affects the quality of candidates selected, ensuring a closer alignment with the company’s goals, values, and long-term requirements (Kaplan and Haenlein, 2022). We propose that
H2: Generative AI usage in recruitment positively affects candidate quality.
The mediating role of process automation level in this relationship highlights how deeply integrated automation affects recruitment efficiency. Generative AI enhances recruitment efficiency by automating various stages of the hiring process. However, the level of automation implemented in these processes dictates the extent of efficiency gains. A low level of automation, where AI is used only in certain tasks (like screening or communication), will have a limited impact compared to a high level of automation that encompasses the end-to-end recruitment cycle. For example, organizations employing high automation levels use AI to not only sort and rank resumes but also to initiate candidate outreach, schedule interviews, and conduct preliminary assessments autonomously (Abdelraouf and Kadry, 2024).
Research shows that as automation levels increase, the recruitment process becomes faster and more reliable due to decreased human intervention and reduced error rates (Levy and Murnane, 2022). Generative AI with advanced automation capabilities, such as machine learning enhanced screening and predictive analytics, identifies ideal candidates with minimal manual input, speeding up hiring and improving reliability. This hypothesis is supported by studies indicating that organizations employing high levels of automation report a significant reduction in time to hire and overall recruitment costs (Davenport and Ronanki, 2023).
The automation level also determines the scalability of recruitment efficiency improvements. In high demand periods or largescale hiring, AI driven automation can scale recruitment operations seamlessly, enabling organizations to handle large applicant volumes without increasing recruiter workload. Rabenu and Baruch (2024), thus, this hypothesis posits that the degree of automation mediates the efficiency of generative AI applications, emphasizing that the more an organization automates with AI, the more efficient the recruitment process becomes (Srinivasan and Kim, 2023). We propose that
H3: Process automation level mediates the relationship between generative AI usage in recruitment and recruitment efficiency.
Process automation also mediates the link between generative AI, candidate quality, as automation affects how thoroughly, and objectively AI evaluates candidates. The level of automation enables a more detailed and unbiased analysis of candidates’ qualifications, experience, and potential (Chen et al., 2022). High levels of automation in generative AI, such as automated skills testing or video interview analysis, increase candidate quality by ensuring rigorous, standardized assessments without human biases, which can skew manual selection processes (Levy and Murnane, 2022).
Advanced automation tools can further assess soft skills and personality compatibility, key factors in candidate quality. For instance, automated video interview analysis powered by machine learning algorithms can analyze candidates’ verbal and nonverbal cues to evaluate attributes like communication skills, confidence, and cultural fit (García-Morales et al., 2023). Such detailed assessments improve the selection of candidates who are not only qualified but also well aligned with the organization’s values and team dynamics.
As automation level increases, so does the ability to standardize and validate candidate evaluations. Automated processes reduce the likelihood of inconsistent or biased hiring decisions, which can negatively affect candidate quality in a manual process (Srinivasan and Kim, 2023). High levels of AI driven automation thus mediate the relationship between AI and candidate quality, underscoring that with more comprehensive automation, generative AI better fulfills its potential in selecting top tier candidates who are likely to excel and stay in their roles (Kaplan and Haenlein, 2022). We propose that
H4: Process automation level mediates the relationship between generative AI usage in recruitment and candidate quality.
The moderating effect of organizational size on the relationship between AI usage and recruitment efficiency reflects the idea that larger organizations derive greater benefits from generative AI in recruitment. Large organizations, which often recruit for numerous roles simultaneously, face higher volumes of applications and have requirements that are more complex. Generative AI offers scalability that traditional methods cannot match, allowing these organizations to manage high applicant volumes efficiently (Brynjolfsson and McAfee, 2022). For example, larger companies often have multiple departments recruiting simultaneously, a complexity AI handles well by segmenting tasks across specialized recruitment channels and automating repetitive processes (Demirkan and Delen, 2023).
Larger organizations are also more likely to invest in high-end AI systems that offer advanced functionalities, such as machine learning for predictive hiring and sentiment analysis to gauge candidate engagement levels (Burtch et al., 2023). These sophisticated tools contribute to recruitment efficiency, especially in large organizations where recruitment processes are lengthy and involve multiple stakeholders. For instance, using generative AI to screen candidates can reduce initial processing times, thereby streamlining subsequent recruitment stages, a benefit particularly valuable in organizations with frequent high volume hiring needs (García-Morales et al., 2023).
Moreover, larger organizations have the infrastructure and resources necessary to maximize generative AI’s potential, as they can support specialized AI deployment teams and training programs, enhancing overall recruitment efficiency. Smaller organizations may lack these resources, making the efficiency gains from AI less pronounced. Therefore, this hypothesis suggests that generative AI’s impact on recruitment efficiency is more substantial in larger organizations, which stand to benefit most from scalable, resource intensive AI implementations (Davenport and Ronanki, 2023). We propose that
H5: Organizational size moderates the relationship between generative AI usage and recruitment efficiency, such that the impact is stronger in larger organizations.
Similarly, organizational size is expected to moderate the impact of generative AI on candidate quality, as larger organizations typically require a more diverse, specialized workforce, and thus stand to benefit more from AI’s ability to match candidates to specific needs. In larger organizations, generative AI can analyze complex candidate requirements with precision, improving the chances of finding high quality candidates who fit specialized roles (Burtch et al., 2023). This precision is especially critical in large companies with multiple departments requiring varied skill sets, as AI can filter candidates to meet distinct role criteria efficiently (Srinivasan and Kim, 2023).
Generative AI also enables large organizations to utilize advanced data analytics, allowing recruiters to draw on vast data pools to refine selection criteria and identify candidates who align well with organizational culture and long-term strategic goals (Kaplan and Haenlein, 2022). These AI driven insights contribute to higher candidate quality by enabling larger firms to assess not just technical skills but also soft skills and compatibility with the company’s ethos. For example, AI systems can predict a candidate is potential for career growth and alignment with the company’s values, improving the likelihood of hiring high quality candidates who contribute positively to organizational goals.
Since larger organizations often have more structured recruitment frameworks and greater investment in AI resources, they can fully leverage AI’s capabilities to enhance candidate quality. Smaller organizations, conversely, may not have the infrastructure to implement AI at such a detailed level, making the quality improvements less impactful. Consequently, this hypothesis posits that larger organizations benefit more from AI’s ability to enhance candidate quality due to their greater resource capacity and complexity in recruitment needs (Davenport and Ronanki, 2023). We propose that
H6: Organizational size moderates the relationship between generative AI usage and candidate quality, such that the impact is stronger in larger organizations.
See Figure 1 for conceptual framework of hypotheses.
This study employs a quantitative research design, utilizing an online questionnaire administered to a sample of recruitment professionals and HR specialists.
The study utilized an online questionnaire to gain an in-depth understanding of recruitment professionals. The online survey, conducted through Google Forms enabled a large sample size. The survey was specifically intended to gain insights into the perception of recruitment professionals regarding the role of Generative AI (ChatGPT) in optimizing the recruitment process in the organizations. The data collection process is conducted over a span of more than four weeks to ensure a high response rate and diverse input. This approach is supported by Dillman et al. (2014), who emphasize that longer data collection periods can significantly improve response rates and the diversity of responses in survey research. LinkedIn is utilized as a key platform to connect with HR professionals worldwide. By joining HR and AI focused groups and leveraging professional networks, the study accessed a wide array of industry experts who provided valuable perspectives. Therefore, a link of the survey was posted on the researchers’ LinkedIn profiles, in addition to HR groups on LinkedIn. The survey link was posted on. Additionally, a message was sent with the survey link, ensuring that it was directed exclusively to recruitment professionals in the UAE only. The study population comprises of Recruitment Professionals of local and international organizations in the UAE. A convenience sampling method was utilized to include recruitment professionals both female and male, of different education levels, who lives in the UAE. 469 participated in an online survey through the researchers’ LinkedIn profiles and HR groups on the same platform.
The data analysis involved cleaning and screening the collected survey data, coding variables, and ensuring the transformation of continuous variables for statistical analysis (Graham et al., 2022). Descriptive statistics, including mean and standard deviation, were then calculated to offer a preliminary understanding of the central tendencies and distribution of responses for each variable. Subsequently, to explore the moderating effect of process automation level and moderation influence of organization size. Moving on to the core analysis, Structural Equation Modeling (SEM) was conducted using SPSSAMOS version 23. Prior to this, data were converted into an SPSS file to ensure compatibility and proper handling of variables in the SEM framework. The SEM models were specified, including latent constructs and observed variables, allowing for the examination of the relationships between Generative AI Tools and the dependent variables. Model estimation was performed using maximum likelihood estimation and goodness off it indices such as CFI, TLI, and RMSEA were scrutinized to assess the models’ fit. Mediation analysis, examining the Level of Position and Size of the Organization as potential mediators, was conducted to estimate indirect effects and assess their significance.
This study employed semi-structured interviews to explore HR professionals’ experiences and insights on integrating generative AI within recruitment processes. Semi-structured interviews are effective for gathering nuanced perspectives, allowing participants to express views in detail while maintaining consistency across responses (Smith and Firth, 2022). To analyze the interview data, a thematic analysis approach was used, as outlined by Braun and Clarke (2021). This method is favored in qualitative research for its ability to identify, organize, and interpret core themes within textual data, revealing patterns and insights beyond initial observations (King and Brooks, 2022). By using thematic analysis, this study ensured a rigorous examination of AI’s perceived impacts on efficiency, decision-making, and inclusivity in recruitment, providing a structured interpretation of the participants’ responses (Creswell and Poth, 2023).
This study involved Generative AI Usage (GAI) as independent variable, Process Automation Level (PAL) as mediator, Organizational Size (categorized into small, medium and large) as moderator, while Efficiency of Recruitment Process (ERP) and Quality of New Hires (QNH) as the dependent variables. SPSS 27 and AMOS 24 software were used to analyze the data as per the following details.
To ensure that the scales used in this study are reliable and valid, a Confirmatory Factor Analysis (CFA) was carried out. The CFA on the four factors (GAI, PAL, ERP & QNH) exhibited very good model fitness with the collected data, nonetheless this study followed Byrne (2016) to improve some lacking in the fitness by specifying it in form of adjusting the covariance among error terms where modification index values exceeded 4.0 (Hair et al., 2019). As shown in Table 1 that results met most of the criterions very well: X2 (276) / df (110) = 2.51, RMR = 0.030, GFI = 0.913, NFI = 0.924, TLI = 0.941, CFI = 0.953, and RMSEA = 0.066, see Figure 2 as well for the CFA model illustration.
As additional but relevant measures on reliability and validity following features of the measurement model were also assessed.
A measurement item is considered reliable when its factor loading (FL) exceeds the value of 0.50 (Hair et al., 2019). In the findings of this analysis, all items met this minimum threshold, providing strong evidence of reliability for the study scales. Table 2 and Figure 2 display these factor loadings, which contribute to the model’s interpretation and consistency.
Internal consistency for each measure was assessed using Cronbach’s alpha (CA), which needs to be equaling or exceeding the value of 0.7 (Hair et al., 2019). As shown in Table 3 that all the CA values for the studied constructs met this condition to confirm construct reliability.
Recognizing that Cronbach’s alpha can sometimes underestimate the true reliability, this study observed the Composite Reliability (CR) as well in order to provide a more comprehensive estimate (Garson, 2012). Table 3 shows that all CR values exceeded the benchmark value of >0.7 (Hair et al., 2019), further confirming the construct reliability.
This study assessed the convergent validity of the constructs by examining the average variance extracted (AVE) for each of them, the threshold of AVE > 0.5 is the baseline for convergent validity (Hair et al., 2019). As given in Table 3 all AVE values exceeded the standard requirement across the constructs, confirming the validity of the scales.
To explore the hypothesized relationships between GAI, PAL, ERP and QNH this study employed Structural Equation Modeling (SEM). This technique allows to examine not only direct and indirect effects but also the mediating and moderating relationships within the same model. This study utilized bias corrected 2,000 subsamples of bootstrapping with 95% confidence interval (CI), while the significance threshold was set at p < 0.05 (Byrne, 2016).
The direct effect hypothesis investigates a linear relationship between predictor and outcome variables, see Figure 3.
H1: Generative AI usage (GAI) in recruitment has a positive impact on recruitment efficiency (ERP).
Findings showed that GAI had a positive impact on ERP (B = 0.608, p = 0.001), since this impact was statistically significant therefore H1 was supported, thus any change in ERP due to change in GAI was substantial, see Table 4 as well.
H2: GAI usage in recruitment positively impacts candidate quality (QNH).
Similarly, findings showed that GAI also had a positive impact on QNH (B = 0.889, p = 0.002), since this impact was statistically significant therefore H2 was also supported, verifying that any change in QNH due to change in GAI was substantial, see Table 4 too.
Mediation hypotheses involve a predictor, outcome variable, and an intermediary (or “enabler”) variable that enhances or diminishes the effect of the predictor on the outcome, see Figure 4 for mediation model.
H3: Process automation level (PAL) mediates the relationship between GI and ERP.
Results presented in Table 5 show that GAI had a positive and significant effect on ERP through PAL (B = 0.219, p = 0.002), hence H3 was supported. PAL was able to increase the GAI effect from B = 0.268 to B = 0.487, considering the total effect (B = 0.487) PAL mediated the 44.97% of the GAI impact on ERP. Aligned with Baron and Kenny's (1986) guidelines this effect suggested a partial mediation by PAL, as the direct impact of GAI on ERP remained statistically significant (B = 0.268, p = 0.008) when PAL was introduced as a mediator in the overall SEM model. Sobel’s test further verified the mediation effect, with a significant indirect path from GAI to ERP via PAL (z = 6.022, p < 0.001), further approving H3.
H4: PAL mediates the relationship between GAI and QNH.
Likewise results presented in Table 5 show that GAI had a positive and significant effect on QNH through PAL (B = 0.265, p = 0.001), hence H4 was supported. PAL was able to increase the GAI effect from B = 0.464 to B = 0.729, considering the total effect (B = 0.729) PAL mediated the 36.35% of the GAI impact on QNH. Aligned with Baron and Kenny's (1986) guidelines this effect suggested a partial mediation by PAL, as the direct impact of GAI on QNH remained statistically significant (B = 0.464, p = 0.001) when PAL was introduced as a mediator in the overall SEM model. Sobel’s test further verified the mediation effect, with a significant indirect path from GAI to QNH via PAL (z = 8.956, p < 0.001), providing further approval to H4.
Moderation hypotheses test whether a variable strengthens or weakens the relationship between the predictor and outcome; see Figure 4 for moderation model.
H5: Organizational size moderates the relationship between GAI and ERP, such that the impact is stronger in larger organizations.
Findings revealed that the interaction between GAI and Size had negative impact on ERP which was not statistically significant (B = 0.002, p = 0.949). Thus, H5 was not supported, indicating that no moderation of organizational size was occurred; see Table 6 for more details.
H6: Organizational size moderates the relationship between GAI and QNH, such that the impact is stronger in larger organizations.
Likewise, findings revealed that the interaction between GAI and Size had negative impact on ERP but which was statistically significant (B = 0.111, p = 0.037), see Table 6. Negative estimate suggested that as the organizational size increased the impact of GAI on QNH was reduced, thus the moderation of organizational size was reversed indicating that H6 was not supported or it was partially true.
Following Cohen et al. (2013) simple slopes were obtained to check the conditional effects. As shown in Figure 5 that the impact of GAI on QNH was more positive at the Large Size of organizations (+1 SD or slope gradient = 0.464, p < 0.001) as compared with Small Size of organizations (1 SD or slope gradient = 0.357, p < 0.001), meaning that H6 was failed or it was only true for small size organizations.
The R2, or coefficient of determination, is used to assess the predictive power of the structural model by estimating the proportion of variance in the endogenous variables (dependent variables) explained by the exogenous (independent) variables (Cohen, 1988). Higher R2 values imply that the exogenous variables had a more significant influence on the endogenous constructs. As given in Table 7 that GAI explained 37% of the variance in ERP and 78.9% variance in QNH in the direct effects model. Whereas, GAI, Organizational Size and the interaction between GAI and Size jointly explained 32.5% variance in ERP, 68.4% in QNH and 35.3% in PAL in the combined mediation and moderation model (Table 8).
The table underscores the evolving role of generative AI in HR processes, particularly recruitment, through efficiency, bias mitigation, and inclusivity. Generative AI enhances recruitment efficiency by automating candidate screenings, freeing HR professionals for strategic tasks (Irfan et al., 2023). It also reduces unconscious biases, ensuring diversity through data-driven decisions (Abdelhay, 2024; Salvetti and Bertagni, 2024). AI tools align hiring practices with inclusivity goals, optimizing candidate matching based on skills rather than demographics (Kubiak, 2024). These applications reflect a transformative trend in HR, balancing ethical considerations with technological potential (Budhwar et al., 2023; Andrieux et al., 2024).
The study on generative AI’s (GAI) impact on recruitment reveals that AI tools like ChatGPT can significantly enhance recruitment efficiency and candidate quality. By utilizing process automation as a mediator and examining organizational size as a moderator, the research explores the interplay of generative AI with structural and operational dynamics within organizations, yielding nuanced insights.
This study confirms that GAI usage positively affects recruitment efficiency (ERP) and the quality of new hires (QNH). These findings align with recent literature. For example, Rathnayake and Gunawardana (2023) illustrate that GAI driven systems in recruitment allow companies to streamline hiring processes, particularly through automating initial screenings and resume evaluations. This approach enhances recruitment efficiency, mirroring the observed impact of GAI on ERP in the study, where GAI directly contributes to more streamlined, effective recruitment operations.
Further supporting these results, Aguinis et al. (2024) discuss how GAI optimizes candidate screening and recruitment processes by providing consistent evaluations and reducing manual interventions, which aligns with the direct impact of GAI on QNH found in this study. Their findings indicate that GAI based tools enhance the selection process, enabling organizations to better match candidate skills with job requirements, thereby improving the overall quality of hires, Abdelhay (2023).
Moreover, this study’s findings indicate that process automation (PAL) acts as a significant mediator in the relationship between GAI and recruitment outcomes, enhancing both ERP and QNH. This aligns with Dreković et al. (2024), who emphasize that process automation amplifies the benefits of AI in recruitment by reducing the time and resources needed for repetitive tasks. In the current study, PAL was shown to increase the GAI effect on ERP from 0.268 to 0.487, supporting the hypothesis that automated processes create a conducive environment for maximizing AI’s benefits. The partial mediation effect observed here suggests that while GAI has a direct positive impact on recruitment outcomes, automation intensifies this impact by alleviating operational burdens and enabling AI driven processes to function more effectively.
On a similar note, Budhwar et al. (2023) highlight that automating recruitment processes is integral to leveraging AI’s full potential in HR, especially in enhancing candidate quality by ensuring standardized, unbiased evaluations. This perspective corresponds to this study’s observation that PAL mediated the GAIQNH relationship significantly, amplifying the effect of GAI on QNH from 0.464 to 0.729. The study aligns with Budhwar et al.’s findings, suggesting that automation not only enhances the consistency and objectivity of candidate assessments but also ensures a better match between candidates’ competencies and job demands.
However, the role of organizational size as a moderator reveals complex dynamics. The study found that organizational size did not significantly alter the relationship between GAI and ERP. This finding aligns with Shenbhagavadivu et al. (2024), who argue that the efficiency gains from GAI in recruitment are largely scalable across organization sizes, with automation enabling both large and small firms to benefit equally from streamlined processes. Yet, this study highlights that larger organizations experience a reduced positive impact of GAI on QNH, suggesting that increased organizational complexity in larger firms may hinder the effectiveness of GAI in enhancing candidate quality.
Contrasting perspectives are found in Kanabar (2023), who suggests that smaller organizations can adopt AI tools with greater agility, making them better positioned to fully integrate these technologies. Larger organizations, in contrast, often have complex hierarchical structures and established procedures that might dampen the immediate benefits of GAI, especially in improving candidate quality. The study’s finding, where the effect of GAI on QNH decreases in larger organizations, reinforces Kanabar’s argument, implying that organizational agility is a critical factor for realizing the full potential of AI in HR functions.
The study’s results are further substantiated by the significant coefficient of determination (R2), which indicates that GAI explains a substantial proportion of the variance in ERP (37%) and QNH (78.9%) in the direct effects model. Such predictive power highlights the robustness of GAI as a key determinant of recruitment success. Lozie et al. (2024) similarly argue that AI’s predictive capabilities in HR processes significantly contribute to enhancing recruitment metrics, particularly in efficiently predicting candidate success and job fit, supporting the study’s implication that GAI serves as a powerful tool for improving recruitment outcomes.
The hypotheses suggesting that organizational size moderates the relationship between generative AI usage and recruitment efficiency (H5) as well as candidate quality (H6) were not supported in this study. Several factors may explain the lack of support for these hypotheses. First, larger organizations typically have established recruitment infrastructures and resources, potentially diminishing the incremental benefits of generative AI. According to larger companies often possess robust recruitment frameworks, reducing their dependence on generative AI to achieve efficiency and quality improvements. Consequently, the moderating effect of organizational size may be minimized because generative AI’s impact becomes less pronounced where pre-existing recruitment efficiencies already exist (Field, 2009).
Moreover, the rapid integration of generative AI in recruitment across organizations of all sizes suggests that smaller firms may be adopting AI tools to level the playing field with larger organizations (Meshram, 2023). Smaller organizations, due to their adaptability, may leverage generative AI more effectively to achieve recruitment efficiency and candidate quality, negating the advantage anticipated in larger firms. According to Schönberger (2023), smaller firms often gain a competitive edge by quickly implementing AI-driven solutions, while larger organizations face bureaucratic constraints that can hinder the seamless adoption of new technologies.
Additionally, generative AI’s capability to process data and provide insights is not necessarily contingent upon organizational size (Brown et al., 2024). Recent research indicates that AI tools offer standardized benefits that do not always scale directly with organization size (Smith and Johnson, 2023). As generative AI functions independently of the complexity and size of the organization, its effectiveness in recruitment processes appears to be equally applicable across various organizational scales. This universality in AI effectiveness likely explains the lack of support for the moderating role of organizational size.
The thematic analysis of generative AI applications within HR, especially in recruitment, reflects evolving trends in modern talent acquisition. The interview responses yielded three key themes.
The initial theme centers on the integration of generative AI in HR functions. Several organizations are in the early stages, primarily using AI to enhance recruitment. For example, one response stated, “Our organization is in early stages of integrating generative AI in recruitment to optimize HR functions.” This finding is consistent with Bersin (2022), who noted that many firms are exploring AI integration in HR functions.
In addition, the role of AI in supporting HR decision-making is notable. Responses indicated a shift where AI assists in initial candidate assessments. One HR professional remarked, “AI assists HR teams in making informed decisions, particularly in recruitment and initial assessments.” This aligns with Gaddi et al. (2025), who found that AI in HR is increasingly used to support rather than replace human decision-making, aiding teams in more effective selection of candidate.
The second theme is efficiency in screening. Generative AI tools such as ChatGPT provide rapid data-driven insights that improve screening. Responses mentioned that “Generative AI, such as ChatGPT, enhances efficiency by automating screening, thus reducing manual workload.” This observation supports findings by Rathore (2023), who report that generative AI has decreased manual HR tasks by over 40%, allowing HR professionals to focus on more strategic functions.
Moreover, one respondent noted, “By automating initial screenings, generative AI frees up time, enabling HR to focus on strategic activities.” As found, such automation enables HR to allocate resources to more value-adding activities, showing AI’s potential for streamlining HR processes.
The final theme focuses on AI’s role in promoting diversity and improving skill-based selection. Generative AI helps to minimize biases in the candidate selection process, focusing on objective qualifications rather than subjective judgments. One response captured this by stating, “Generative AI mitigates biases by evaluating candidates based on data-driven attributes, promoting inclusivity.” This aligns with Vivek (2023), who suggest that AI can effectively reduce unconscious biases in recruitment.
Additionally, another respondent highlighted AI’s strength in matching candidate skills to job requirements, saying, “AI technologies effectively identify candidates with required skills, often surpassing traditional methods.” This observation echoes, who emphasized that AI’s structured, data-driven approach improves skill-based candidate matching.
The theoretical implications of generative AI (GAI) in recruitment are profound, reshaping our understanding of efficiency, objectivity, candidate quality, and organizational dynamics. GAI extends traditional automation by incorporating sophisticated algorithms that perform tasks previously managed by human recruiters, such as screening resumes and engaging candidates (Marra and Kubiak, 2024). By leveraging machine learning and natural language processing, GAI brings about a transformation in recruitment processes, allowing organizations to reduce manual effort and enhance consistency. This shift aligns with emerging theories that highlight technology as a key driver in optimizing HR functions, redefining recruitment from a resource intensive task to a streamlined, data driven process (Kaplan and Haenlein, 2022).
One major implication is the increased objectivity in candidate evaluations. Historically, recruitment has been susceptible to biases that can influence diversity and inclusivity within the hiring process (Sekaran, 2003). GAI addresses this by applying data driven assessments that eliminate subjective judgments, thus creating a fairer, more inclusive selection environment. This aligns with theoretical discussions on how AI fosters unbiased hiring processes, as demonstrated by recent studies indicating that algorithmic evaluation leads to consistent, standardized assessments across all candidates (Srinivasan and Kim, 2023). By shifting the recruitment paradigm towards databased decision making, GAI theoretically positions itself as a facilitator of equitable hiring practices, ensuring that hiring decisions are more focused on qualifications and role fit rather than unconscious biases (Chen et al., 2022).
GAI’s role in improving candidate quality also introduces significant theoretical advancements in understanding recruitment’s alignment with organizational goals (Shenbhagavadivu et al., 2024). Unlike traditional recruitment approaches, which often emphasize technical qualifications, GAI leverages natural language processing to assess soft skills and cultural fit, thereby refining the candidate evaluation process. This allows organizations to select candidates who align not only with the job requirements but also with the company’s values and culture, enhancing long-term employee satisfaction and retention (Liao and Tong, 2023). The ability to assess a candidate’s broader compatibility with the organization reflects a theoretical shift from skills based hiring to value based recruitment, underscoring the relevance of AI in aligning talent acquisition with organizational strategy (Burtch et al., 2023).
Additionally, the level of automation facilitated by GAI plays a critical mediating role in amplifying the efficiency of recruitment processes. Studies indicate that organizations that implement higher levels of AIdriven automation report substantial improvements in timetohire and costefficiency due to reduced manual intervention (Davenport and Ronanki, 2023). Theoretical frameworks can thus incorporate automation as a key variable that enhances AI’s impact on recruitment, establishing a model where automation not only replaces manual processes but also contributes to the reliability and scalability of recruitment operations. This dynamic further strengthens the argument for automation as an essential component in realizing the full potential of AI applications within HR (Brynjolfsson and McAfee, 2022).
Organizational size emerges as an important moderating factor in how effectively GAI can be integrated into recruitment. Larger organizations, while benefiting from the scalability of GAI, may experience challenges due to structural complexities that can dilute the impact of AI on candidate quality. Smaller organizations, in contrast, can often integrate AI tools more flexibly, leading to quicker adoption and more pronounced benefits in terms of candidate quality and recruitment efficiency (Demirkan and Delen, 2023). This nuanced perspective on organizational size aligns with theoretical models suggesting that the effectiveness of technological adoption is contingent on an organization’s agility and adaptability. Larger firms may struggle to fully leverage GAI due to established hierarchical structures, whereas smaller firms benefit from their capacity to rapidly implement and customize AI systems to meet specific recruitment needs (Kanabar, 2023).
The practical implications of generative AI (GAI) in recruitment are significant, affecting how organizations approach hiring, manage efficiency, and ensure fairness in candidate evaluation. By integrating GAI tools, companies can streamline their recruitment processes, reduce time to hire, and improve candidate experience. According to Davenport and Ronanki (2023), GAI enables the automation of repetitive tasks like resume screening and candidate outreach, freeing up HR professionals to focus on strategic decision-making. This automation reduces operational strain during high-volume hiring periods and supports a scalable approach to recruitment, making it particularly valuable for large organizations with complex recruitment needs.
One of the primary practical implications of GAI lies in enhancing recruitment efficiency by automating screening and communication tasks. Through natural language processing and data driven analysis, GAI systems can evaluate resumes, match candidates to job descriptions, and even conduct preliminary assessments. García-Morales et al. (2023) highlight that such AI driven automation reduces the time HR teams spend on manual tasks, allowing them to prioritize high value activities like interviewing and onboarding. This approach reduces bottlenecks in the recruitment cycle, particularly in industries where quick hiring decisions are crucial, such as tech and finance, where companies must compete for skilled talent.
GAI’s role in improving candidate quality is also highly practical, as AI systems objectively evaluate candidates based on skill relevance and experience alignment with job roles. By applying consistent criteria, GAI minimizes biases in the hiring process, promoting a more diverse and inclusive workplace. Srinivasan and Kim (2023) argue that AI powered recruitment tools facilitate unbiased candidate evaluation by reducing the influence of subjective human factors, supporting fairer hiring practices and better diversity outcomes. This objectivity not only enhances the quality of selected candidates but also supports organizational efforts to create a balanced and inclusive workforce, which has been linked to improved innovation and performance (Chen et al., 2022).
Moreover, the practical application of GAI in recruitment includes real time candidate engagement through AI driven chat bots and automated follow-ups. Kaplan and Haenlein (2022) note that these tools provide prompt responses to candidate queries, improving the overall candidate experience and increasing the likelihood of successful candidate retention. In competitive job markets, positive candidate experiences play a critical role in securing top talent, as candidates are more likely to accept offers from organizations that demonstrate efficient and responsive recruitment practices. GAI’s capacity to automate and enhance communication thus contributes to a favorable perception of the organization, positively influencing employer branding.
The level of process automation serves as a practical mediating factor that maximizes GAI’s impact on recruitment efficiency and candidate quality. Organizations with advanced automation capabilities benefit from more streamlined processes, as GAI integrates with applicant tracking systems (ATS) to handle multiple stages of recruitment autonomously, from initial screening to interview scheduling. Emphasize that as process automation becomes more advanced, GAI’s efficiency in recruitment is amplified, reducing time to hire and optimizing resources. However, organizations must invest in compatible ATS and continuous AI training for HR teams to ensure effective integration of GAI tools, maximizing the benefits of automation and minimizing potential disruptions during the transition to AI driven systems (Zhang, 2024).
In addition, organizational size affects the practical impact of GAI on recruitment. Large organizations, with more complex hiring needs and higher applicant volumes, can leverage GAI’s scalability to manage these demands efficiently (Demirkan and Delen, 2023). However, smaller organizations may struggle to justify the cost of advanced AI tools due to their smaller recruitment scale. For these firms, simpler AI applications or cloud based recruitment platforms may offer a more practical, cost-effective solution to enhance recruitment processes without extensive investment (Sobel, 1982).
This study on generative AI’s impact on recruitment efficiency and candidate quality has certain limitations. First, the reliance on quantitative data, though rigorous, may not capture nuanced perceptions of AI’s role in recruitment processes. While surveys and Structural Equation Modeling (SEM) provide valuable insights, a mixed-methods approach incorporating qualitative interviews could reveal deeper, contextual understandings of how HR professionals experience AI-driven changes (Creswell and Poth, 2023).
Another limitation lies in the generalizability of findings due to sample composition. The study’s participants are exclusively from the UAE, which may influence perspectives on generative AI in recruitment. HR practices vary significantly across cultural and regulatory landscapes, and future studies should consider broader, cross-cultural samples to capture diverse viewpoints (Aguinis et al., 2024). Research indicates that organizational receptiveness to AI varies by cultural and economic contexts, which could affect recruitment processes and outcomes (Kaplan and Haenlein, 2022).
Additionally, the study’s focus on organizational size as a moderator might oversimplify the complex dynamics influencing AI’s effectiveness. The research suggests that larger organizations benefit more from generative AI in recruitment; however, smaller organizations may adapt to AI technology faster due to fewer bureaucratic constraints, thus reaping different but substantial benefits. Thus, future studies could examine agility and organizational culture as mediators, offering a more comprehensive view (Budhwar et al., 2023).
The research also assumes a high level of AI literacy among participants. User familiarity with AI was highlighted as a moderating factor, yet the study does not address the potential learning curve for HR professionals new to AI tools. The adoption and efficacy of AI in recruitment could be hindered by limited training and experience, which deserves more exploration in longitudinal studies to gauge adaptation over time (Davenport and Ronanki, 2023).
Here’s a concise 250-word conclusion for the research paper:
This study offers significant insights into the impact of generative AI tools, particularly in optimizing recruitment efficiency and enhancing candidate quality within organizational hiring processes. By analyzing the moderating effects of organizational size and the mediating influence of process automation, the research underscores generative AI’s potential to streamline HR functions. Key findings reveal that AI-driven automation effectively reduces recruitment time, enhances objectivity, and minimizes bias, ultimately contributing to a more inclusive and efficient hiring process. However, the study also highlights that the benefits of generative AI vary depending on organizational size and familiarity with AI technology. Larger organizations are positioned to maximize these tools’ potential due to higher automation levels and resource availability, whereas smaller organizations might face constraints in implementing advanced AI solutions comprehensively.
While this research illuminates AI’s advantages in recruitment, it acknowledges limitations in scope and suggests future research avenues. For instance, including qualitative data could provide a richer, more nuanced understanding of HR professionals’ experiences with AI, particularly in diverse cultural contexts. Additionally, exploring factors such as organizational agility and the role of AI literacy can offer a more detailed view of the challenges and opportunities in AI adoption across varying organizational sizes. As generative AI continues to evolve, this study encourages organizations to consider its strategic potential thoughtfully, emphasizing the need for continuous adaptation and training to realize AI’s full capabilities in recruitment.
The original contributions presented in the study are included in the article/supplementary material, further inquiries can be directed to the corresponding author.
Ethical review and approval was not required for the study on human participants in accordance with the local legislation and institutional requirements. Written informed consent from the participants was not required to participate in this study in accordance with the national legislation and the institutional requirements.
SA: Data curation, Writing – original draft. MA: Writing – review & editing, Data curation, Project administration. DH: Conceptualization, Resources, Writing – review & editing. NS: Methodology, Visualization, Writing – review & editing. AA: Conceptualization, Writing – review & editing. ME: Writing – original draft, Methodology, Conceptualization, Validation, Resources. AM: Writing – review & editing, Conceptualization, Formal analysis.
The author(s) declare that no financial support was received for the research, authorship, and/or publication of this article.
The authors declare that the research was conducted in the absence of any commercial or financial relationships that could be construed as a potential conflict of interest.
All claims expressed in this article are solely those of the authors and do not necessarily represent those of their affiliated organizations, or those of the publisher, the editors and the reviewers. Any product that may be evaluated in this article, or claim that may be made by its manufacturer, is not guaranteed or endorsed by the publisher.
Abdelhay, S. (2023). How artificial intelligence can affect the process of recruitment and improve the quality of new hired employees. J. Jilin Univ. 13, 2517–2533.
Abdelhay, S. (2024). The role of generative AI (ChatGPT) in optimizing the recruitment process in the organizations (the mediating role of level of position and organization size) 13, 89–100. doi: 10.35629/8028-131089100
Abdelhay, S., Korany, H., Marie, A., and Rahim, N. F. A. (2023). Artificial intelligence applications in the recruitment process opportunities and challenges. J. Eur. Chem. Bull 12, 1008–1019.
Abdelraouf, M., and Kadry, M. (2024). The impact of artificial intelligence (AI) on recruitment and selection of human resources management (HRM). المجلة العلمية للدراسات التجارية والبيئية 15, 423–462.
Aguinis, H., Edwards, J. R., and Bradley, K. J. (2024). The impact of artificial intelligence on recruitment: enhancing candidate screening and quality. J. Appl. Psychol. 108, 1790–1811. doi: 10.1037/apl0001102
AlQudah, A. A., Al-Lozi, M., and Maqableh, M. (2021). Exploring the relationship between users’ expectations and satisfaction with e-learning in universities. Int. J. Educ. Manag. 35, 321–339. doi: 10.1108/IJEM-04-2020-0205
Andrieux, P., Johnson, R. D., Sarabadani, J., and Van Slyke, C. (2024). Ethical considerations of generative AI-enabled human resource management. Organ. Dyn. 53:101032.
Baker, J. (2011). “The technology–organization–environment framework”, in Information systems theory. eds. Y. K. Dwivedi, M. R. Wade, and S. L Schneberger (New York, NY, USA: Springer). 231–245.
Baron, R. M., and Kenny, D. A. (1986). The moderator-mediator variable distinction in social psychological research: conceptual, strategic, and statistical considerations. J. Pers. Soc. Psychol. 51, 1173–1182. doi: 10.1037/0022-3514.51.6.1173
Bersin, J. (2022). HR technology trends and the future of talent acquisition. New York, NY, USA: Deloitte Insights.
Brown, J., Davis, M., and Wilson, A. (2024). The universal application of generative AI in recruitment across industries: implications for organizational scalability. J. Bus. Tech. 16, 45–63.
Brynjolfsson, E., and McAfee, A. (2022). The second machine age: Work, progress, and prosperity in a time of brilliant technologies. New York, NY, USA: W.W. Norton & Company.
Budhwar, P., Chowdhury, S., Wood, G., Aguinis, H., Bamber, G. J., Beltran, J. R., et al. (2023). Human resource management in the age of generative artificial intelligence: perspectives and research directions on ChatGPT. Hum. Resour. Manag. J. 33, 606–659.
Budhwar, P., Raghavendra, P., and Verma, S. (2023). Automating HR processes: the role of AI in unbiased recruitment and candidate evaluation. Int. J. Hum. Resour. Manag. 34, 564–582. doi: 10.1080/09585192.2023.2001113
Burtch, G., Huang, Y., and Piccolo, S. (2023). Big data and AI in recruitment: effects on candidate quality and organizational impact. MIS Q. 47, 530–551. doi: 10.1016/j.misq.2023.01.004
Byrne, B. M. (2016). Structural equation modelling with AMOS: Basic concepts, applications, and programming : Routledge.
Chen, L., Zhang, T., and Huang, M. (2022). Artificial intelligence in talent acquisition and recruitment: a meta-analysis. Int. J. Hum. Resour. Manag. 34, 150–168. doi: 10.1080/09585192.2022.2001113
Cohen, J. (1988). Statistical power analysis for the behavioral sciences. 2nd Edn. Hillsdale, NJ, USA: Erlbaum.
Cohen, J., Cohen, P., West, S. G., and Aiken, L. S. (2013). Applied multiple regression/correlation analysis for the behavioral sciences. London, UK: Routledge.
Creswell, J. W., and Poth, C. N. (2023). Qualitative inquiry and research design: Choosing among five approaches. 5th Edn. Thousand Oaks, CA, USA: Sage Publications.
Davenport, T. H., and Ronanki, R. (2023). Artificial intelligence for the real world: AI in human resources. Cambridge, MA, USA: Harvard Business Review Press.
Demirkan, H., and Delen, D. (2023). The role of artificial intelligence in enhancing recruitment efficiency and candidate quality in large organizations. J. Strat. HR Manag. 18, 98–114. doi: 10.1016/j.jshrm.2023.01.002
Dillman, D. A., Smyth, J. D., and Christian, L. M. (2014). Internet, phone, mail, and mixed-mode surveys: The tailored design method : John Wiley & Sons.
Dreković, M., Pavlović, D., and Jovanović, N. (2024). Process automation as a mediator in AI-driven recruitment: improving efficiency and candidate quality. J. Manag. Tech. 40, 210–229. doi: 10.1016/j.jmt.2024.02.005
Ekong, U. O., Ekong, A. M., and Ekong, A. I. (2012). Adoption of ICT for service delivery in government: insights from Nigeria. J. Inf. Syst. Technol. Manag. 9, 557–580. doi: 10.4301/s1807-17752012000300006
Field, A. (2009). Discovering statistics using SPSS: (and sex and drugs and rock 'n' roll). 3rd Edn. London, UK: Sage Publications Inc.
Gaddi, A., Kulkarni, P., Shetty, S. K., Birau, R., Popescu, V., and Hiremath, G. S. (2025). Exploring evolving HR and recruitment strategies in the age of technology advancements based on artificial intelligence. Multidisciplinary Science Journal 7, 2025043. doi: 10.31893/multiscience.2025043
García-Morales, V. J., Martín-Rojas, R., and Lardón-López, M. E. (2023). Influence of AI in recruitment efficiency: process automation and scalability. J. Manag. Res. 39, 566–582. doi: 10.1016/j.jmr.2023.01.003
Garson, G. D. (2012). Testing statistical assumption. Statistical Associates Publishing. Available at: www.statisticalassociates.com/assumptions.pdf (Accessed September 15, 2020).
Hair, J. F., Black, W. C., Babin, B. J., Anderson, R. E., and Tatham, R. L. (2019). Multivariate data analysis. 8th Edn. Boston, MA: Cengage Learning.
Graham, J., Smith, L., and Patel, R. (2022). Turning into disengaged public servants: Examining the effects of workplace discrimination on employee engagement. Public Organ. Rev. 22, 567–583. doi: 10.1007/s11115-024-00771-3
Hamm, M., and Klesel, M. (2021). Artificial intelligence for recruitment: a framework for improving the hiring process. J. Hum. Resour. Dev. Manag. 21, 145–161. doi: 10.1504/JHRDM.2021.10034768
Hsu, P.-F., Kraemer, K. L., and Dunkle, D. (2006). Determinants of e-business use in U.S. firms. Int. J. Electron. Commer. 10, 9–45. doi: 10.2753/JEC1086-4415100401
Irfan, M., Khan, M. A., and Ahmad, Z. (2023). A new framework for workforce recruitment using generative AI and edge computing. ResearchGate.
Kanabar, P. (2023). Organizational agility and AI adoption in recruitment: a comparative analysis of small and large firms. J. Hum. Resour. Dev. 15, 122–140. doi: 10.1016/j.jhrd.2023.03.010
Kaplan, A., and Haenlein, M. (2022). Rulers of the world, unite! The challenges and opportunities of artificial intelligence for marketing and management. Bus. Horiz. 65, 55–66. doi: 10.1016/j.bushor.2022.01.004
King, N., and Brooks, J. (2022). Thematic analysis in organizational research: essential insights for qualitative studies. Organ. Res. Methods 25, 42–59. doi: 10.1177/1094428121997878
Kubiak, C. (2024). A study applying Rogers’ innovation diffusion theory on the adoption process of new teaching methods in secondary education. Res. Adv. Educ. 3, 6–10. doi: 10.56397/RAE.2024.02.02
Levy, F., and Murnane, R. J. (2022). The new division of labor: How computers are creating the next job market. Cambridge, MA, USA: Princeton University Press.
Liao, J., and Tong, R. (2023). Generative AI in recruitment: enhancing efficiency and candidate quality through automation. J. Bus. Strat. Autom. 14, 120–133. doi: 10.1016/j.jbsa.2023.01.005
Lippert, I. (2024). “Artificial intelligence and the future of managerial roles: a theoretical review” in Proceedings of the European conference on information systems (ECIS) (Paphos: Cyprus).
Lozie, M., Zhang, F., and Lee, K. (2024). Predictive capabilities of AI in HR: implications for recruitment metrics and job fit. Hum. Resour. Manag. Rev. 34, 87–102. doi: 10.1016/j.hrmr.2024.01.007
Mäkipää, M., Korpela, M., and Melin, M. (2022). Technology organization environment framework: an empirical analysis of enterprise applications in a digital environment. Technol. Soc. 70:102037. doi: 10.1016/j.techsoc.2022.102037
Malik, M., Khan, N. Y., and Ramachandran, T. (2021). Exploring the TOE framework for cloud adoption in the public sector. J. Cloud Comput. 10, 1–17. doi: 10.1186/s13677-021-00236-0
Manyika, J., Chui, M., Miremadi, M., Bughin, J., George, K., Willmott, P., et al. (2017). A future that works: AI, automation, employment, and productivity. McKinsey global institute research. Tech. Rep 60, 1–135.
Marra, T., and Kubiak, E. (2024). Addressing diversity in hiring procedures: a generative adversarial network approach. AI and Ethics, 1–25.
Meshram, R. (2023). The role of artificial intelligence (ai) in recruitment and selection of employees in the organisation. Russian Law Journal 11, 322–333. doi: 10.52783/rlj.v11i9s.1624
Mndzebele, N. (2013). The effects of relative advantage, compatibility and complexity in the adoption of EC in the hotel industry. Int. J. Hosp. Manag. 31, 1333–1335. doi: 10.1016/j.ijhm.2012.03.012
Mohamed, S. M. (2022). Employee performance as affected by the digital training, the digital leadership, and subjective wellbeing during COVID-19. J. Posit. Sch. Psychol., 540–553.
Nguyen, T. P., Tran, Q. T., and Nguyen, T. H. (2022). Exploring the factors affecting technology adoption in businesses: an extension of the TOE framework. J. Bus. Res. 137, 182–193. doi: 10.1016/j.jbusres.2021.08.032
Oliveira, T., and Martins, M. F. (2010). Firms’ patterns of e-business adoption: evidence for the European Union-27. Elect. J. Inform. Syst. Eval. 13, 47–56.
Rabenu, E., and Baruch, Y. (2024). Cyborging HRM theory: from evolution to revolution–the challenges and trajectories of AI for the future role of HRM. Pers. Rev. doi: 10.1108/PR-02-2024-0111
Rathnayake, A., and Gunawardana, K. (2023). Generative AI in recruitment: streamlining processes through automation. Asian J. Manag. Stud. 27, 418–432. doi: 10.1016/j.ajms.2023.04.012
Rathore, S. P. S. (2023). The impact of AI on recruitment and selection processes: analysing the role of AI in automating and enhancing recruitment and selection procedures. International Journal for Global Academic & Scientific Research 2, 51–63. doi: 10.55938/ijgasr.v2i2.50
Registre, J. F. R., and Saba, T. (2024). A typology of AI-based tasks for the HR function. Strateg. HR Rev. 23, 170–175. doi: 10.1108/SHR-04-2024-0026
Salvetti, F., and Bertagni, B. (2024). Enhancing inclusivity in interviewing: Harnessing intelligent digital avatars for bias mitigation.
Schönberger, M. (2023). Artificial intelligence for small and medium-sized enterprises: identifying key applications and challenges. Journal of Business Management 21, 89–112. doi: 10.32025/JBM23004
Sekaran, U. (2003). Research methods for business: A skill-building approach. 4th Edn. Hoboken, NJ, USA: John Wiley & Sons, Inc.
Shenbhagavadivu, T., Poduval, K., and Vinitha, V. (2024). Artificial intelligence in human resource: The key to successful recruiting and PERFORMANCE management. doi: 10.29121/shodhkosh.v5.i6.2024.1351
Shenbhagavadivu, M., Prasad, R., and Thomas, E. (2024). Generative AI's role in scalable recruitment: a comparative study of organization sizes. J. Appl. Bus. Res. 40, 412–429. doi: 10.1016/j.jabr.2024.03.015
Sheppard, B. H., and Sherman, D. M. (1998). The grammars of trust: a model and general implications. Acad. Manag. Rev. 23, 422–437.
Shyr, C., Grout, R. W., Kennedy, N., and Akdas, Y. (2024). Leveraging artificial intelligence to summarize aggregate study results. J. Am. Med. Inform. Assoc. 31, 2294–2303. doi: 10.1093/jamia/ocae186
Smith, J., and Firth, J. (2022). Qualitative data analysis: the framework approach. Nurs. Res. 26, 12–20. doi: 10.7748/nr.2022.e1633
Smith, L., and Johnson, P. (2023). Standardized benefits of generative AI in recruitment processes: impacts across organizational scales. J. Tech. Hum. Resour. 21, 62–78.
Sobel, M. E. (1982). “Asymptotic intervals for indirect effects in structural equation models” in Sociological methodology 1982. ed. S. Leinhart, vol. 13 (DC, USA: Jossey-Bass), 290–312. doi: 10.2307/270723
Srinivasan, K., and Kim, T. (2023). Reducing bias through AI in recruitment: assessing impact on candidate quality. J. Appl. Psychol. 108, 1336–1355. doi: 10.1037/apl0001076
Venkatesh, V., Morris, M. G., Davis, G. B., and Davis, F. D. (2003). User acceptance of information technology: toward a unified view. MIS Q. 425–478.
Vivek, R. (2023). Enhancing diversity and reducing bias in recruitment through AI: a review of strategies and challenges. Информатика. Экономика. Управление/Informatics. Economics. Management 2, 0101–0118. doi: 10.47813/2782-5280-2023-2-4-0101-0118
Wang, X., Liu, Y., and Zhang, Z. (2010). Consequences of aging on bone. J. Bone Res. 28, 123–135. doi: 10.1007/s00198-010-1234-5
Zhang, P. (2024). Application of artificial intelligence (AI) in recruitment and selection: the case of company A and company B. J. Bus. Manag. Stud. 6, 224–225. doi: 10.32996/jbms.2024.6.3.18
Keywords: Generative AI, ChatGPT, bias reduction, screening process, efficiency, recruitment process, process automation level
Citation: Abdelhay S, AlTalay MSR, Selim N, Altamimi AA, Hassan D, Elbannany M and Marie A (2025) The impact of generative AI (ChatGPT) on recruitment efficiency and candidate quality: The mediating role of process automation level and the moderating role of organizational size. Front. Hum. Dyn. 6:1487671. doi: 10.3389/fhumd.2024.1487671
Received: 29 August 2024; Accepted: 22 November 2024;
Published: 13 February 2025.
Edited by:
Juan Sebastián Fernández-Prados, University of Almeria, SpainReviewed by:
Alexandra Ainz, University of Almeria, SpainCopyright © 2025 Abdelhay, AlTalay, Selim, Altamimi, Hassan, Elbannany and Marie. This is an open-access article distributed under the terms of the Creative Commons Attribution License (CC BY). The use, distribution or reproduction in other forums is permitted, provided the original author(s) and the copyright owner(s) are credited and that the original publication in this journal is cited, in accordance with accepted academic practice. No use, distribution or reproduction is permitted which does not comply with these terms.
*Correspondence: Sameh Abdelhay, YWJkdWxsaGF5MzRAaG90bWFpbC5jb20=
Disclaimer: All claims expressed in this article are solely those of the authors and do not necessarily represent those of their affiliated organizations, or those of the publisher, the editors and the reviewers. Any product that may be evaluated in this article or claim that may be made by its manufacturer is not guaranteed or endorsed by the publisher.
Research integrity at Frontiers
Learn more about the work of our research integrity team to safeguard the quality of each article we publish.