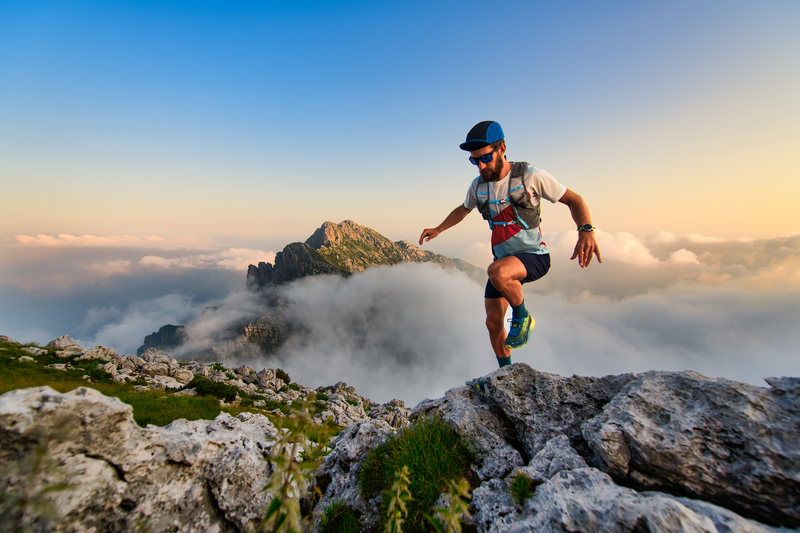
94% of researchers rate our articles as excellent or good
Learn more about the work of our research integrity team to safeguard the quality of each article we publish.
Find out more
ORIGINAL RESEARCH article
Front. Hum. Dyn. , 03 October 2024
Sec. Digital Impacts
Volume 6 - 2024 | https://doi.org/10.3389/fhumd.2024.1473893
This article is part of the Research Topic Socio-Legal, Ethical, Technical and Medical Considerations on Neuroprivacy and Brain-Machine Interaction Technologies in the era of A.I. View all 10 articles
This paper explores the emerging practice of workplace surveillance by using neurotechnologies, particularly wearable neurodevices, to monitor employees’ cognitive abilities, concentration levels, and emotional responses. It aims to assess the legality of such practices within the framework of EU law, focusing on the General Data Protection Regulation (GDPR) and the EU Artificial Intelligence Act (AI Act) by providing a detailed analysis of recent EU legislation in the context of the implementation of neurosurveillance at the workplace. Furthermore, the paper discusses whether current regulations adequately address the use of neurotechnologies in the workplace or are overly restrictive. It raises the question of ensuring sufficient flexibility in the regulations to allow for legitimate implementations of neurotechnologies in the labour field for workers’ safety while protecting workers’ rights. Overall, the paper offers insights into the intersection of neurotechnology advancements and labour relations and stimulates critical discussion about the fair balance between innovation and workers’ rights.
In 1921, the Russian author Yevgeny Zamyatin wrote the dystopian novel “We,” which depicts a fictional totalitarian society where individuality and personal freedom are suppressed in favour of societal harmony and order (Zamiatin, 1924). The novel takes place in the 26th century and is set in a state where individuals are subject to mass surveillance methods and expected to act, think, and feel according to predefined rational values. The novel’s protagonist, D-503, is a mathematician and engineer who works on constructing a spaceship called the Integral. As the story progresses, D-503’s beliefs are challenged, leading to a conflict between his loyalty to the state and his desire for personal freedom. In Zamyatin’s dystopian society, most people are presented as sincerely driven by the principles of rational social order and world harmony. Conversely, modern-day surveillance methods are primarily driven by profit interests (Zuboff, 2019). One variant of modern surveillance methods is implemented in the workplace to increase the profit and efficiency of the business. Unfortunately, this frequently leads to the dehumanization of individuals, treating them as mere machines rather than beings with autonomy and free will.
Today, algorithmic management has transformed workplace monitoring, giving rise to the so-called Amazonian Era (Gilbert and Thomas, 2021). The name is inspired by the technology company Amazon, which often pioneers the invasive collection of personal information at work (Gurley, 2022). It has been reported that this company implements location tracking, biometric analysis, face and image recognition, wearable devices, advanced algorithms, and big data to collect employees’ productivity data. This information is then used to assess, discipline, rate, and reward workers, creating new power dynamics in the workplace (De Stefano, 2019).
As neurotechnology advances, there is a growing concern that employers may be able to intrusively monitor their workers’ brains through modern surveillance methods known as neurosurveillance (Muhl and Andorno, 2023). These neurotechnologies are becoming more accessible due to the progress and the convergence of neuroscience, AI, machine learning and big data. By using neurodevices, employers can monitor employees’ mental workload, emotional states, concentration levels, and degree of alertness or fatigue in the workplace (Maior et al., 2018; Wexler and Reiner, 2019; Niso et al., 2023).
One of the most accessible ways to record and analyse brain activity is through an EEG wearable device, such as a headband or earbuds, which can collect raw brain data. Through algorithmic analysis, this brain data may be separated from noise and used to gain access to information aboet alut an employee’s concentration level, emotions, and mental workload; it constitutes the first-order data. It can also integrate additional data sources to generate second-order inferences (ICO, 2023). For example, using brain data, the employer can make broader predictions about the workers’ future performance, cognitive abilities or behavioral trends.
The recent report of the UK’s independent data regulatory body, the Information Commissioner’s Officer, predicts a significant expansion in the use of non-invasive neurotechnology in the workplace for safety, wellness, and employee recruitment reasons over the next five years (ICO, 2023). For example, neurosurveillance can be integrated into health and safety programs to measure and enhance employee attention and focus. It is possible now to identify neurocognitive states like mind wandering, effort withdrawal, and inattentional phenomena (Dehais et al., 2020). In this context, neurodevices can be particularly useful for high-risk environments, such as those that involve heavy machinery or long working hours shifts. Additionally, wearable neurotechnologies that promote well-being are now available in the consumer market as a self-check tool to gain awareness of concentration and stress levels, the degree of alertness or fatigue, and the emotional states in the workplace (Wexler and Reiner, 2019; Niso et al., 2023). As a recruitment tool, neurotechnologies could be used to identify individuals with desired behavioral traits, estimate cognitive abilities and classify participants’ levels of executive functions and intelligence scores (Zazon et al., 2023). According to Nita Farahany’s opinion, EEG-based systems will become a gold standard in workplace fatigue monitoring, but how much we ultimately gain from workplace brain wearables depends mainly on how employers leverage the technology (Farahany, 2023).
It is important to point out that mental data is generated subconsciously, and individuals have no control over the information it reveals. For example, EEG brain data can provide valuable insights into various cognitive and neurological processes and emotional responses to stimuli. Employers can use these data to determine the cognitive abilities and level of concentration of their workers. Combined with other technologies like algorithmic control of task duration, performance monitoring, and real-time location tracking, it could create an environment of unprecedented work efficiency, where every minute of the employee’s work time is well counted and directed towards efficiency. However, this over-intrusive practice can severely affect employees’ human agency, privacy rights, and psychological well-being.
There are concerns that algorithm-based neurotechnologies could become more invasive and intrusive with the progress and collection of Big Data. This could lead to a blurring of work-life balance as workers may feel they are constantly being watched, even during their off-time. This constant surveillance may cause workers to feel uncomfortable and act unnaturally, such as forcing them to smile or suppress their true emotions and personalities to please the algorithm. As we move towards a future where machines influence work requirements, workers may feel pressured to adopt the high standards of maximising effectiveness, displaying unwavering concentration, remaining devoid of emotional responses, and achieving 100% efficiency in managing workloads. As argued by N. Farahany, using brain wearables at work has implications beyond employee safety, productivity, and stress levels; it also affects workers’ dignity and the future of work itself (Farahany, 2023).
Over the past few years, the use of AI and digital tools in workplace management and evaluation have been a matter of attention for scientists and lawmakers. Still, the application of neurodevices for employer management and worker surveillance has yet to be thoroughly examined. While neurotechnologies offer employers new tools, there is a lack of legal assessment regarding the acceptability of neurosurveillance in the workplace. This issue has been little discussed in mainstream debates about the future of work (Muhl and Andorno, 2023).
In addition, it is essential to acknowledge that AI and digitalisation pose not only quantitative risks in terms of job availability in the market but also qualitative risks for employees (De Stefano, 2019). Instead of simply replacing humans with machines, certain technological advancements allow employers to treat humans as though they were machines (Gilbert and Thomas, 2021). This can be seen in the changing business models associated with the rise of the “gig economy,” where emerging technologies significantly impact the quality of working conditions. For example, digital platforms that utilize algorithmic management have created a new form of employment known as the “non-standard form of employment” (Vallas and Schor, 2020). These platforms, such as Uber, Bolt, and Deliveroo, connect independent subcontractors with paid tasks from clients, providing services on demand (De Groen et al., 2018). The distribution of tasks, work intensity, and work prices are determined directly by algorithms, while worker ratings are based on customer feedback. The Platform Work Directive, currently undergoing final approval by the Council of the EU, aims to obliges EU countries to establish a rebuttable legal presumption of employment at national level (European Commission, 2021). Contrary to initial expectations, the EU will not establish standardized criteria for classifying platform workers as employees. As a result, member states may implement the presumption differently (McKenzie, 2024).
Further research is required because platform work is diverse in nature. Initially, it is crucial to establish the criteria for differentiating platform workers from self-employed individuals as outlined in the Platform Work Directive and upcoming state legislation. This article focuses on determining the rules for implementing neurotechnologies in traditional employment. However, some articles from the Platform Work Directive will be referenced as examples of relevant regulatory solutions.
As neurotechnology has the potential to impact workplaces significantly, it is crucial to coordinate regulatory policies properly (Gonfalonieri, 2020). In particular, this article aims to explore how the existing EU privacy legislation, mainly the General Data Protection Regulation (hereafter “the GDPR”) (European Union, 2016) and the Artificial Intelligence Act (hereafter “the AI Act”) (European Union, 2024), are equipped to balance neurotechnological advances and the employees’ privacy and right to good and fair working conditions (Riso, 2023). It is beyond the scope of this paper to conduct an in-depth comparative analysis of EU member states legislation.
It is widely agreed that the European Union has the world’s strictest legislation for protecting private data. The recently enacted AI Act is the first of its kind in the world. In this article, I would like to analyse how the EU prepares for new emerging trends in employee neurosurveillance with AI-based neurotechnologies. As this trend continues to develop, it raises concerns about the future of work and the role of technology in shaping our professional lives. It prompts a reasonable question: how can we prevent the creation of a dystopian and paternalistic workplace environment? This naturally leads to another question: does the current legal framework of the EU adequately cover such technologies, and are there any gaps?
More specifically, this article mainly aims to assess the EU’s legal frameworks regarding the legitimacy of implementing non-invasive wearable neurodevices for employees monitoring. For methodological purposes, this task will be performed using a two-step strategy. Firstly, analyse the GDPR concerning the legitimacy of the collection of employees’ brain data. Secondly, examine the AI Act in relation to the use of specific brain data and other employees’ information to draw inferences about workers.
The GDPR, known as the most influential data protection regulation worldwide, regulates all stages of data processing and is applicable to any organisation that deals with the personal data of natural persons within the EU. Recital 4 of the GDPR recognises that the right to personal data protection is not absolute, but “it must be considered in relation to its function in society and be balanced against other fundamental rights, in accordance with the principle of proportionality”.
Article 6 of the GDPR defines the lawfulness of processing personal data. According to it, personal data should be processed on the basis of the consent of the data subject or some other legitimate basis laid down by law, either in the GDPR or in other Union or Member State law. This may include the necessity to comply with the legal obligation to which the controller is subject or the necessity for the performance of a contract to which the data subject is party or in order to take steps at the request of the data subject prior to entering into a contract.
Article 88 of the GDPR provides general rules for processing employees’ data by employers across EU member states. First, each member-state of the European Union has the freedom to choose how to regulate the processing of employees’ data, including sensitive data, through national laws and collective agreements, with a focus on safeguarding the data subject’s dignity, legitimate interests and fundamental rights, with particular regard to the transparency of processing and the transfer of personal data. Second, the employers are allowed to process employee data for work-related purposes and must obtain consent for any additional processing activities. According to the article 88 (1) of the GDPR, this means that the employer can process employees’ data for: recruitment, execution of employment contracts, diversity and equality in the workplace, planning and organization of work, management of the company, safety and health in the workplace, protection of employer’s or customers’ property, or any other obligation the employer may have under the applicable laws and collective agreements. This also includes, in some circumstances, processing of sensitive personal data, such as health data, race, ethnic origin and others.
In labour relationships, the GDPR regulates all data processing and ensures a balance between an employer’s legitimate interest in developing their business while providing safe working conditions and an employee’s reasonable expectation of privacy. Employers must comply with strict data protection rules when processing an employee’s data. Therefore, although continuous monitoring and tracking of worker behavior is technically possible, the legislation sets boundaries for intrusive surveillance that respects employees’ privacy.
When assessing whether an employer is allowed to collect and process their employees’ brain data under the GDPR, it is crucial to consider some essential factors: (2.1) Firstly, the legal status of brain data must be defined. (2.2) Secondly, consent as a legal basis for processing sensitive data must be examined. (2.3) Thirdly, the conditions for processing employees’ sensitive data to assess their working capacity should be analysed. (2.4) Additionally, it is important to highlight that employers have an obligation to consult with their workers prior to deploying any workplace surveillance systems. (2.5) Lastly, we need to identify the potential weaknesses of the GDPR.
It is beyond dispute that the measurements of brain activity obtained by an employer can be labelled as “personal data” as they can be linked to a specific individual (employee). According to Article 4(1) of the GDPR, personal data can be defined as “any information that pertains to an identified or identifiable natural person”.
It is essential to determine if personal data obtained through the use of neurosurveillance tools falls under a special category of personal data that is called “sensitive data.” Article 9 of the GDPR outlines a list of sensitive data categories, which includes health, medical data, and biometric characteristics of an individual. It also includes information that reveals political opinions, religious or philosophical beliefs, trade union membership, or sexual orientation.
In academic discourse, experts generally agree that brain data should be considered a special category of sensitive data, extending to it the same level of protection as provided for genetic data under the GDPR (Rainey et al., 2020; Ienca et al., 2022; Ienca and Malgieri, 2022). Furthermore, researchers suggest including a new term in legislation for brain data, referring to “quantitative information regarding the structure, activity, and function of the human brain” (Ienca et al., 2022). Until the concept of brain data is explicitly included as a special category of data in the GDPR, the classification of brain data as sensitive would depend on the type of device used for its collection (medical or consumer) and the information it could reveal.
It is important to mention, that the distinction between mental data and brain data has become a crucial topic of discussion in neuroscience, neuroethics, and data protection. Ienca and Malgieri (2022) introduced the concept of “mental data” as information that allows inference of an individual’s mental states, distinguishing it from both neural and behavioral data. They argue that while some neural and behavioral data can be considered mental data when used to infer mental states, not all such data fall into this category. This conceptualization aligns with Ienca et al. (2022) definition of brain data as “quantitative data about human brain structure, activity and function,” which can include direct measurements of brain activity and indirect functional indicators. The distinction is further nuanced by Muñoz et al. (2024), who notes that brain data can be combined with non-neural contextual data to support inferences about mental processes in a broader sense. This perspective highlights the potential for a more comprehensive understanding of mental states through the integration of various data sources. However, there are ongoing debates about the accuracy and reliability of current neurodevices in inferring mental states from neural data, which complicates the distinction between mental and brain data. These discussions underscore the need for a more precise conceptual and regulatory framework to address the unique challenges posed by the collection, analysis, and protection of both mental and brain data in an era of advancing neurotechnology (Muñoz et al., 2024).
There is an opinion that “not all mental data are protected under the strict regime of sensitive data,” because “the list of sensitive data categories in the GDPR (health, biometric, genetic, political opinions, sexual orientations, etc.) is not comprehensive enough to include ‘emotions’ or other ‘thoughts’ not related to health status, sexuality or political/religious beliefs” (Ienca and Malgieri, 2022).
From another perspective, raw EEG brain data that records brain wave frequencies could potentially contain information about neurological disorders such as epilepsy, Alzheimer’s, and Parkinson’s disease. As a result, it falls under the definition of “health data” as a special category of personal data described in Article 4(15) of the GDPR.
This opinion aligns with the clarification provided by the Article 29 Working Party, an independent European organization responsible for enforcing data protection regulations across the EU and fostering collaboration among EU data protection authorities. According to its commentary, personal data is considered health data when “the raw sensor data that can be used in itself or in combination with other data to draw a conclusion about the actual health status or health risk of a person” (Annex—Health Data in Apps and Devices, 2015). In our case, the data itself contains sensitive information about the individual, while the processing algorithm only determines what kind of information can be derived from the raw data. Therefore, treating brain data as sensitive aligns with the fundamental human rights principle of privacy and warrants protection.
Defining data obtained from neurodevices as sensitive would generally prohibit employers from processing it, as per Article 9(1) of the GDPR. However, the law provides exceptions and special conditions that allow for its processing, which will be examined further.
According to Article 9(1)(a) of the GDPR, processing of sensitive data requires freely given, specific, informed, and unambiguous consent. However, the legal validity of the employee’s consent becomes problematic.
According to the Guidelines 05/2020 on Consent under the Regulation of the European Data Protection Board, “consent can only be an appropriate lawful basis if a data subject is offered control and is offered a genuine choice with regard to accepting or declining the terms offered or declining them without detriment” (European Commission, 2018). The validity of the consent at the workplace was also a matter of clarification of Article 29 Working Party (EDPB, 2018), an independent EU advisory body in its Opinion 2/2017 on Data Processing at Work (Falque-Pierrotin, 2017). It defines the general presumption: “Employees are almost never in a position to freely give, refuse or revoke consent, given the dependency that results from the employer/employee relationship.” This Opinion 2/2017 also addressed specifically the use of wearable devices by employers to track and monitor employee health and activity. According to Opinion 2/2017, “Given the sensitive nature of health data and the unequal relationship between employers and employees, it is highly unlikely that legally valid explicit consent can be obtained for the tracking or monitoring of such data.” Indeed, as employees are inherently dependent on their employer in many respects, their consent could be given under the fear of job loss or any other disadvantage. In this regard, the consent for neuromonitoring can be considered as a non-valid form to express free will.
Moreover, neither the employer nor the employee can be certain about the type of information that might be revealed through neurosurveillance. The employee may have difficulty predicting their own biological and mental processes prior to giving permission to use this information. This is because the data obtained from an employee’s brain signals may uncover unconscious or implicit information that the employee is unaware of and cannot control. For example, the use of neurotechnology could reveal the personal opinions of employees, detect their irritation and hidden stress, or other strong emotions towards their colleagues and superiors. In this regard, the consent could not be defined as specific. Additionally, as research advances, previously obtained data may be rediscovered with greater precision in the future, without the data subject’s permission and awareness. Some experts consider brain scans to be comparable to unique fingerprints, as they provide a distinct depiction of an individual’s brain (Finn et al., 2015).
If we assume that, hypothetically, employees may provide consent for the use of their information for a specific purpose, such as monitoring of their fatigue level for safety reasons, there is a possibility that this data could be intentionally or unintentionally used for additional purposes without the employee’s awareness or permission and despite the obligation for purpose limitation of personal data under article 5.1.b GDPR. Furthermore, when combined with other data resources such as wearable devices for physical conditions, monitoring of voice, face images and text writing patterns, the information could be even more broad, and reveal complex characteristics of the personality. This problem is also addressed in the academic discussion by the concept of “digital phenotyping” (Insel, 2017; Loi, 2019).
All these arguments make it difficult to define such consent as informed, specific, and unambiguous under Article 9(1)(a) of the GDPR. This leads to the conclusion that, according to the GDPR, it is not legal for an employer to use tools for neurosurveillance at the workplace based solely on employee consent. Consent can be necessary but not in itself sufficient (Aloisi and Gramano, 2019). However, the GDPR has another provision that could potentially allow the employer to collect and process employees’ brain data.
In accordance with Article 9(2)(h) of the GDPR, special categories of sensitive data can be processed when it is necessary for assessing the working capacity of an employeeor for preventive or occupational medicine. In addition, any processing of such data must adhere to the obligations of professional secrecy.
Moreover, employers have a legal duty to provide a safe working environment, and the aforementioned provisions were established to ensure that they adhere to this obligation. This responsibility is outlined in the EU Framework Directive on Safety and Health at Work, commonly known as the EU Safety Directive OSH (European Union, 2008). Additionally, this directive emphasizes that “when entrusting tasks to a worker, the employer must consider their capabilities with regards to health and safety and adapt to technical progress” (European Union, 1989).
As fatigue and drowsiness are major contributors to workplace injuries, implementing neurotechnology could be considered a measure to enhance safety in tasks where the lack of attention poses a significant risk of accidents (Mitler et al., 1988). By analyzing EEG signal patterns, it becomes possible to detect fatigue and to take preventive measures to avoid accidents caused by human errors. An example of the implementation of neurotechnology for safety reasons can be observed in the mining industry. For instance, SmartCaps, an EEG-based technology integrated into helmets, is currently utilized as safety equipment (Wenco, 2023). Similar neurodevices can assist transport operations such as road, aviation, rail and maritime, as well as other occupational settings (e.g., hospitals, emergency operations, law enforcement) (Ramos et al., 2022) or rescue operations (Dell’Agnola et al., 2022), particularly when irregular work hours are involved. It is important to emphasize that the potential implementation of neurotechnology in such cases might align with EU legislation.
Before taking the decision to use neuromonitoring for workers’ safety, the employers should conduct a Data Protection Impact Assessment (hereinafter, DPIA) as required by Article 35 of the GDPR. This assessment evaluates the potential impact of data processing on the rights and freedoms of individuals, especially when sensitive data is involved and new technologies with unforeseen consequences for fundamental human rights are utilized. Recital 75 of the GDPR also emphasizes the need for DPIA in cases where personal aspects, particularly those related to analyzing or predicting work performance are evaluated. Article 35.7 of the GDPR establishes the minimum requirements for a DPIA. Firstly, the employers conducting a DPIA for the processing of personal data must explain the purpose and legitimate interest behind this processing, such in this case preventive and occupational health purposes.
Secondly, they must assess the necessity and proportionality of the processing, demonstrating that alternative, less intrusive measures are insufficient. In this case, the employer needs to prove that they are adapting to technological advancements to enhance safety, and other existing safety methods are considered less effective and more intrusive. Therefore, the use of the neurodevice could in principle be justified.
There are arguments supporting the proportionality of such measures. For instance, it can be argued that constant video surveillance of drivers operating high-risk machines may be more intrusive than the use of EEG neurodevices, which specifically monitor alpha brain waves to detect fatigue levels and prevent accidents by alerting the driver (Farahany, 2023). This perspective suggests that monitoring brain activity with neurodevices can provide a targeted approach to ensuring safety without the need for constant video surveillance, which may be perceived as more invasive. However, there are differing opinions regarding the effectiveness of EEG-based tools for fatigue monitoring, pointing to a lack of evidence of such neurodevices (Hussein et al., 2023).
Lastly, employers must ensure that the use of the neurodevice does not violate the rights and freedoms of employees and that adequate safeguards are in place to protect their privacy. For instance, the employer should implement data minimization techniques, ensuring that only necessary and relevant data is collected and processed. Additionally, the employer should follow a regime of secrecy, safeguarding the collected data and confirming that access to it is limited to authorized individuals.
The conditions of DPIA are aligned with the same principles as the arguments in the European Court of Human Rights decisions regarding employee privacy (article 8) (European Court of Human Rights, 2022). Yet, the decisions of the European Court of Human Rights (ECHR) also consider another criterion- the employee’s awareness of monitoring as an additional factor when assessing the permissibility of workplace monitoring (Muhl and Andorno, 2023). Implementing neurosurveillance at the workplace may seem challenging at first due to the strict rules of the GDPR. However, there is a possibility that employers can justify such measures for the safety of their workers. To determine the legitimacy of neurosurveillance at the workplace, key principles such as proportionality and subsidiarity are called to play a crucial role.
The European Data Protection Board (EDPB) (which officially replaced Article 29 Working Party) can significantly clarify the situation by publishing recommendations or opinions that explicitly recognise brain data as sensitive, and provide clear guidelines on acceptable conditions for its processing in the workplace. Specifically, the EDPB could define the principle of proportionality as considering both the needs and interests of workers and the company’s interests in such processing. In this regard, a clear criterion of proportionality could be that using neurotechnology in the workplace should benefit the employee and provide tangible safety benefits to the worker compared to other less intrusive tools. Such guidelines would yield positive outcomes for the labour market and offer much-needed clarity for developers intending to introduce neurodevices into the EU market.
In sum, employers should carefully consider implementing neurotechnology for safety purposes, ensuring that the processing of sensitive data adheres to the principles of necessity, proportionality, and data minimisation. It is crucial to protect privacy and maintain confidentiality, and employers should only collect and process the minimum amount of data necessary to achieve fair objectives. In this context, it is essential to establish comprehensive guidelines and best practice standards for the responsible development and deployment of neurotechnologies before their adoption in the European Union labour market.
Specifically, it is suggested to include an explicit provision in the EU level labour legislation that using neurotechnologies in the workplace might be permitted only for safety purposes for exceptional cases, such as monitoring employee fatigue in high-risk jobs. It should also be clear that employers are prohibited from analysing their employees’ emotions and thoughts (De Stefano, 2020).
Scholars suppose that legal protections guaranteeing worker privacy and discretion are blunt instruments without mechanisms that also strengthen worker voices in how these protections are implemented (De Stefano and Doellgast, 2023). In this regard, collective labour rights, especially collective bargaining, are the most effective and proven tools to give workers an authentic voice in distributing benefits or costs from the AI- and data-driven ‘digital revolution’. Labour legislation in the EU offers employers the right to actively participate in the decision to use surveillance tools in the workplace. Employers can express their concerns and, in some cases, even prohibit the implementation of specific technologies for surveillance. Workers frequently overlook this aspect, but active participation allows employees to directly impact changes in working conditions by sharing their perspectives (Moore, 2020).
More concretely, article 11 of the EU Safety Directive OSH (European Union, 1989) requires employers to involve workers in discussions about implementing new technologies at the workplace before conducting a Data Protection Impact Assessment (DPIA). A similar approach is illustrated in Council Directive 2002/14/EC, which sets out a framework for informing and consulting employee representatives “with a view to reaching an agreement on decisions within the scope of the employer’s powers” (European Union, 2002).
These provisions are also in line with domestic labour laws. Depending on the EU country, domestic labour laws could give the employee representatives the right to consultation or, quite often, they even provide a right to co-determination (Aloisi and Gramano, 2019). For example, the right to consultation is realised in France (Respublique Francaise, 2008). It is mandatory for the employer to consult the works council before implementing new technology that may impact the employees’ working conditions, pay, employment, qualifications, or training. If the employer hinders the establishment and free appointment of the works council or obstructs their regular operation, they may be punished with one year’s imprisonment and/or a fine (Respublique Francaise, 2008).
In Germany, for instance, any change in worker policy that affects the pace of work requires co-determination. Co-determination is a decision-making structure within an enterprise whereby employees and their representatives influence decisions, often at a senior level and a relatively early stage (Eurofound, 2020). The implementation of devices, programs, and software must be negotiated in detail with the works councils before implementation (Moore, 2020).
This variety of domestic regulations can result in an international company implementing different policies in different countries. Workers in countries with the right to consultation and co-determination may enjoy better working conditions than those in other countries. This is why it would be important to harmonise labour legislation in the EU to provide co-determination rights in all member countries.
In the EU, employees have the legal right to participate in the selection process of AI-based monitoring tools used in the workplace (European Agency for Safety and Health at Work, 2022). It is crucial to raise awareness among workers about their ability to negotiate and influence their employers’ decisions regarding implementing neuromonitoring tools. For example, the German Confederation of Trade Unions (DGB) has put forward valuable suggestions on AI in the workplace in its Concept Paper on AI for Good Work, which could also apply to neurotechnologies in the workplace (DGB, 2020). By analogy with this suggestion, we can assume that employers should provide workers with advance notice and clear explanations of the workplace neuromonitoring devices they intend to implement. To negotiate effectively, workers must have access to relevant information about new technologies. Therefore, workers’ literacy in technological advancements is crucial for effectively protecting their rights.
An additional example is that the Platform Work Directive also recognizes the importance of consulting workers and their representatives, as outlined in Articles 6, 9, and 12. The proposed Directive aims to encourage social dialogue on algorithmic management systems by establishing collective rights for receiving information and consulting on significant changes related to the use of automated monitoring and decision-making systems. Consequently, individuals working through platforms and their representatives will benefit from improved transparency and understanding of algorithmic management practices, as well as enhanced access to remedies for automated decisions, resulting in better working conditions.
Allowing employers access to the workers’ brain data for safety purposes also sheds light on the potential weakness of GDPR legislation. As some researchers suppose, the GDPR does not address the new risks posed by inferential analytics because “individuals are granted little control and oversight over how their personal data is used to draw inferences about them” (Wachter and Mittelstadt, 2019). Once the data is lawfully obtained, very little control or understanding is reserved for inferential analytics, which remains a “no man’s land” (Wachter et al., 2017).
In our case, it means that the data legally collected for safety purposes, such as monitoring an employee’s level of fatigue and alertness, could be combined for management purposes with other personal information about the worker, such as their gender, age, and health status. This combined data could then be used to create analytics that invade privacy, harm someone’s reputation, provoke discrimination, or make critical decisions based on predictions or subjective opinions that may not be reliable or accurate.
Some scholars reasonably suppose that in cases where new personal information is inferred, such as in the process of profiling, it should be viewed as creating new personal data. This should also apply to the process of re-identifying anonymous or pseudonymous data when it is used to make assessments and decisions (Sartor, 2020). Additionally, the Article 29 Working Party provides valuable commentary on these risks. It states that when repurposing personal data for individualised inferences, it is crucial to assess the legitimacy of the new purpose based on several criteria. These include (1) evaluating the distance between the new purpose and the original purpose, (2) considering the alignment of the new purpose with the expectations of the data subjects, the nature of the data, and their impact on the data subjects’ interests, and (3) ensuring that the data controller has implemented appropriate safeguards to ensure fair processing and prevent any undue negative effects (Article 29, 2013).
Assuming that neurotechnology at the workplace might be permitted only for safety reasons, such as fatigue monitoring for high-risk jobs, it is essential to strictly prohibit any other forms of brain data analysis by developers, employers and other parties. In this regard, privacy law must focus on the use, harm, and risk of data rather than solely on the nature of personal data (Solove, 2023). The legal requirement that personal data must only be used for a clearly defined purpose must be maintained by all means (IndustriAll European Trade Union, 2022). Additionally, it is reasonable to examine the underlying algorithms of neurosurveillance tools thoroughly. In this context, the legislation that regulates the development and use of AI systems plays a significant role.
The European Commission has recently taken steps towards introducing a Regulation that lays down harmonised rules for artificial intelligence, more commonly referred to as the EU AI Act (European Union, 2024). At the end of 2023, the European Parliament, the Council of the European Union and the European Commission had successfully worked in a negotiation process called the “trilogue” to develop a final version of the legislation. The agreed text is finalised and adopted by the EU Parliament and the Council in early 2024, followed by an 18-month transition period before it becomes fully enforced.
The AI Act is a comprehensive framework “for the development, marketing, and use of artificial intelligence in compliance with Union values.” The AI Act does not replace but, in some ways, overlaps with the protections offered by the GDPR, although the former’s scope is more expansive and not restricted to personal data. Its goal is to establish a technology-neutral definition of AI systems and categorise them based on their risk level. To ensure human fundamental rights, health, and safety, the regulation imposes various requirements and obligations based on categories, which include unacceptable, high, limited, and low or minimal risk. AI systems that pose unacceptable risks will be prohibited, while a broad range of high-risk AI systems will be authorised with a set of requirements and obligations to gain access to the EU market. The AI systems with minimal or low risk are allowed, mostly unconditionally.
The AI Act is designed to govern the use of AI systems in various areas, including biometric identification of people, management and operation of critical infrastructure, education, essential private and public services, law enforcement, migration, asylum and border control management, the administration of justice, and democratic processes. According to the AI Act, the use of AI in employment is considered high-risk.
The comprehensive scope of the AI Act may be perceived as a disadvantage. Some scholars suppose that the AI Act risks overgeneralising its regulatory solutions, neglecting to deal with particularities at stake in different sectors (De Stefano and Wouters, 2022). However, in the context of employment regulations, the AI Act does not limit the ability of the Union and Member States to create more specific rules (IndustriAll European Trade Union, 2022). It allows for additional regulations to be put in place to govern the use of AI in the labour sector more precisely.
In general, it is essential to make sure that various provisions of the AI Act are interconnected and coordinated with EU legislation. For instance, the algorithmic management in traditional employment and the rules for platform workers need to be compared and harmonised.
In the AI Act, AI-based neurotechnologies systems for employment are mentioned directly or indirectly in two contexts: (a) addressing unacceptable risk and (b) relating to high-risk AI-based systems.
Firstly, the AI Act proposes specific restrictions to deploy and implement AI that intentionally manipulates individuals. More concretely, Article 5(a) of the AI Act prohibits “the placing on the market, putting into service or the use of AI systems that deploy subliminal techniques beyond a person’s consciousness or purposefully manipulative or deceptive techniques.” The objective of this provision is to prevent “the effect of materially distorting the behavior of a person or a group of persons by appreciably impairing their ability to make an informed decision, thereby causing them to take a decision that they would not have otherwise taken in a manner that causes or is reasonably likely to cause that person, another person or group of persons significant harm.” Additionally, Recital 29 of the AI Act clarifies that this limitation applies to “machine-brain interfaces or virtual reality as they allow for a higher degree of control of what stimuli are presented to persons, insofar as they may be materially distorting their behavior in a significantly harmful manner” (European Union, 2024).
Although the AI Act does not provide an explicit definition for “machine-brain interfaces,” these provisions have a beneficial impact as they restrict the use of neuroscience advancements in an invasive and intrusive manner. According to Recital 29, such use can subvert or impair a person’s autonomy, decision-making, or free choices in ways that people may not consciously be aware of, or even if aware, they might still be deceived or unable to control or resist. It seems that there is a need to clarify the AI Act further around the use of neurotechnology, particularly to prevent its manipulative and deceptive use. This could be achieved by explicitly limiting the collection of brain data for manipulative purposes and prohibiting the use of neurodevices to influence brain activity, such as through neurostimulation, for any purposes except medical.
Surprisingly, the previous version of the AI Act contained in Recital 16 a much more precise prohibition of “neuro-technologies assisted by AI systems that are used to monitor, use, or influence neural data gathered through brain-computer interfaces” (European Union, 2024). Ultimately, this version of the Act did not pass the negotiation process.
Secondly, the AI Act has an important provision that prohibits AI systems designed to detect the emotional state of individuals in the workplace. According to Article 5f, “the placing on the market, putting into service for this specific purpose, or use of AI systems to infer emotions of a natural person in the areas of workplace and education institutions.” According to Recital 44, the legislator explains that “there are serious concerns about the scientific basis of AI systems aiming to identify or infer emotions, particularly as expression of emotions vary considerably across cultures and situations, and even within a single individual.” As a result, such systems have “limited reliability, lack specificity, and limited generalizability”.
This rule is designed as a timely response to existing labour market AI-based tools used to catch a candidate’s emotions during a job interview by analysing facial expressions, movements, pulse frequency or voice (Newman, 2020). The provision could also prevent employers from using highly intrusive EEG-based neurotechnologies to detect workers’ emotions.
Article 5 f includes an exception that prohibits the use of AI systems to infer the emotions of individuals in the workplace, except for medical or safety reasons. Moreover, Recital 18 distinguishes between emotions and physical states, treating them as separate concepts. According to this provision, it is forbidden to identify or make assumptions about the emotions or intentions of an individual based on their biometric data. This includes emotions such as happiness, sadness, anger, surprise, disgust, embarrassment, excitement, shame, contempt, satisfaction, and amusement. However, physical states such as pain or fatigue are exempted from this prohibition. For example, systems that detect the state of fatigue in pilots or drivers can be used to prevent accidents. In light of this, lawmakers have prohibited the use of emotion recognition AI systems in workplace-related situations. However, they have left room for the implementation of safety-based neurodevices to detect fatigue for high-risk professions in compliance with labour and data protection laws.
It may be reasonable to consider expanding the list of unacceptable risks under the AI Act to include the prohibition of using neural data obtained through brain-computer interfaces to make inferences about employees. This means that neurodevices should not be used to estimate the cognitive abilities of workers to compare their efficiency, resilience and other personal characteristics. To prevent neurodiscrimination, it would be beneficial to include provisions that entirely prohibit employers from estimating the cognitive abilities of their employees based on neuro data and AI. Moreover, if the employer, under certain circumstances, could detect the physical state of the employee, it should be legally prohibited to use this data for any reason other than preventing accidents.
The Platform Work Directive identified a similar approach, as stated in Article 6 (5), which prohibits the processing of any personal data regarding the emotional or psychological state of the platform worker. In addition, the article provides that digital labour platforms must not process any personal data concerning platform workers that are not intrinsically connected to and strictly necessary for the performance of their contract. This includes not only the psychological or emotional state of the platform worker, but also data on private conversations, health, and any data while the platform worker is not offering or performing platform work.
In sum, the AI Act contains important provisions that forbid the use of AI systems that are associated with purposefully manipulative or deceptive techniques and emotion detection. These provisions are not limited to cases related to the implementation of neurotechnologies and the use of mental data, but they provide general rules aimed at protecting mental privacy, mental integrity, and cognitive liberty at the secondary law level (De Stefano, 2020).
High-risk AI systems are the main focus of the AI Act. They are allowed on the market but must comply with specific mandatory requirements. Such systems may either be used as safety components within products or, as mentioned in the law, as a type of stand-alone product (Article 6 of the AI Act).
According to the AI Act, AI systems used for employment, workers’ management, and self-employment are considered high-risk AI systems (Article 6 (2), Annex III AI Act). This includes two types of AI systems that are designed for specific purposes related to employment:
1. AI systems intended to be used for recruitment or selection of natural persons, notably to place targeted job advertisements, to analyse and filter job applications, and to evaluate candidates;
2. AI systems intended to be used to make decisions affecting terms of work-related relationships, the promotion or termination of work-related contractual relationships, to allocate tasks based on individual behavior or personal traits or characteristics or to monitor and evaluate the performance and behavior of persons in such relationships.
Recital 57 of the AI Act states that AI systems mentioned above “based on individual behavior, personal traits or biometric data, monitoring or evaluation of persons in work-related contractual relationships, should also be classified as high-risk.” This is because “those systems may appreciably impact future career prospects, livelihoods of these persons and workers’ rights.” Additionally, “such systems may perpetuate historical patterns of discrimination, for example, against women, certain age groups, persons with disabilities, or persons of certain racial or ethnic origins or sexual orientation.” Furthermore, “AI systems used to monitor the performance and behavior of these persons may also undermine the essence of their fundamental rights to data protection and privacy”.
Additionally, the Article 26 (7) of the AI Act states the obligation “to inform workers’ representatives and the affected workers that they will be subject to the use of the high-risk AI system before putting into service or using a high-risk AI system at the workplace.” This obligation is in accordance with Union and national law and practice regarding the information of workers and their representatives. The text also emphasizes that this regulation does not override existing obligations for employers to inform and consult workers or their representatives under other legal instruments.
Overall, the AI Act represents a proactive approach to addressing the ethical and societal implications of AI deployment in the workplace, aiming to foster a more inclusive and equitable work environment for all.
After analysing the AI Act, it can be concluded that the use of AI-powered neurodevices in the field of labour is considered high-risk. Under the AI Act, developers of high-risk AI systems must meet various requirements. These include a comprehensive set of risk management, data governance, monitoring and record-keeping practices, detailed technical documentation, transparency and human oversight obligations, and standards for accuracy, robustness and cybersecurity. High-risk AI systems must also be registered in an EU-wide public database. Miscategorising an AI system and/or failing to comply with the respective provisions is subject to a fine of at least 5 million or 1.5% of global turnover, whichever is higher.
As a result, the AI Act has introduced robust measures to protect employees from any possible misuse of AI-powered neurotechnology by employers. However, it is still uncertain whether neurodevices intended for safety purposes and meant to be introduced into the market as safety equipment will be considered legitimate after the evaluation under the risk management system outlined in Chapter 2 of the AI Act. More research is required to determine how effective the safeguards provided to high-risk systems under the AI Act are. It is worth noting that most of the risk management measures (mentioned in Article 9 of the AI Act) are based on self-assessment by technology developers and implementers. Therefore, it is crucial to wait for practical solutions and see how requirements for high-risk systems will be put into practice in real-life scenarios.
The replacement of workers by AI tools is often the primary concern when discussing the future of work. Machines can make data-based decisions faster and more efficiently than humans. As a result, some jobs may be substituted by technological innovation. However, experts predict that the labour market will slowly adapt to these technological advancements and overcome that problem. According to those predictions, low-skilled workers, who are most at risk of job displacement, will likely shift to tasks less susceptible to computerisation, such as those requiring creativity and social intelligence (Frey and Osborne, 2017).
Although monitoring an employee’s mind may seem far-fetched at first glance, it is possible with the current state of technology. The convergence of emerging technologies, such as AI and neurotechnologies, has brought ubiquitous worker surveillance to a new level. The usage of neurotechnologies and their effects on the world of work will only grow. Pervasive and intrusive employee monitoring poses a risk not only to the rights to privacy and data protection but also to the right to good and fair working conditions – this warrants more attention in policymaking (Riso, 2023).
Therefore, at the EU legislative level, from the perspective of the protection of workers’ rights, it is crucial to anticipate the employers’ attempts to implement intrusive neurosurveillance methods. In this regard, the decision to use new revolutionary methods of workplace monitoring should always be judged from the perspective of the workers’ benefits as a first barrier for considering the implementation of such technology.
A review of the EU legislation shows that, at least in theory, the current EU regulations provide a sufficient level of protection for employees. However, there are still areas of uncertainty that need to be addressed to bring some clarity. Based on the analysis of the EU law presented in this paper, the final thoughts and recommendations can be summarised as follows:
Firstly, it is true that employers may face legal risks if they decide to implement neurosurveillance in the workplace due to the GDPR’s strict regulations on data processing. Nonetheless, it is important to recognize that if employers can justify these measures for the safety of their employees, there is a possibility that they will proceed with them. Therefore, in such circumstances, the legitimacy of neurosurveillance in the workplace will largely depend on adopting effective measures to protect employees’ privacy and respecting principles such as proportionality and subsidiarity of brain data collection. In this regard, as a preliminary measure, the European Data Protection Board (EDPB) could play a clarifying role by adopting recommendations that explicitly recognise brain data as sensitive and provide fair guidelines on the conditions for its processing. Specifically, the EDPB could define the principle of proportionality by considering both the needs and interests of workers and the company’s interests. In this regard, a clear criterion of proportionality could be that using neurotechnology provides tangible benefits not only for an employer but also for the workers’ safety.
Another common suggestion is to define brain data as a special category of sensitive data under GDPR. Although that labelling is helpful, it is insufficient because once the data is collected, it becomes another challenge for workers to control its use. Therefore, it is crucial to focus on how brain data is used and to restrict its intrusive and manipulative use.
Secondly, The AI Act prohibits the AI-based neurotechnologies associated with neuro manipulation, mind reading and emotion detection in the labour field. This is useful, but more measures are needed. It is crucial to expand the list of unacceptable risks under the AI Act, including the prohibition of using neural data obtained through brain-computer interfaces to make inferences about employees. This means that neurodevices should not be used to estimate workers’ cognitive abilities to compare their efficiency, resilience, and other personal characteristics. There is a critical need for extensive normative and legal research and broader public discourse to address the complexities of neurodiscrimination. The evaluation of cognitive abilities in the employment context must be defined as grounds for discrimination.
Thirdly, the AI Act is intended to have broad applicability across multiple sectors, and not only regarding labour relations, which are rather incidentally regulated by the Act. Therefore, it is important to focus further on the labour sector when considering employers possibly use AI-based neurodevices to monitor employees’ mental states. Establishing specific standards for implementing neurotechnology in different industry sectors would be reasonable. For instance, as neurosurveillance becomes more prevalent in high-risk professions such as high-speed train drivers and heavy machinery operators, it is essential to develop responsible use statements tailored to these fields. The first stage in developing such a code of best practices should include a discussion involving trade unions, industry representatives and technology developers to foster a culture of trust among parties.
The original contributions presented in the study are included in the article/supplementary material, further inquiries can be directed to the corresponding author.
EM: Writing – review & editing, Writing – original draft.
The author(s) declare that no financial support was received for the research, authorship, and/or publication of this article.
The author declares that the research was conducted in the absence of any commercial or financial relationships that could be construed as a potential conflict of interest.
All claims expressed in this article are solely those of the authors and do not necessarily represent those of their affiliated organizations, or those of the publisher, the editors and the reviewers. Any product that may be evaluated in this article, or claim that may be made by its manufacturer, is not guaranteed or endorsed by the publisher.
Aloisi, A., and Gramano, E. (2019). Artificial intelligence is watching you at work: digital surveillance, employee monitoring, and regulatory issues in the EU context. Comp. Lab. L. Poly J. 41, 95–121.
Annex—Health Data in Apps and Devices. (2015). Concept of “health data” in directive 95/46/EC [preprint]. Available at: https://ec.europa.eu/justice/article-29/documentation/other-document/files/2015/20150205_letter_art29wp_ec_health_data_after_plenary_annex_en.pdf (Accessed May 30, 2023).
Article 29. (2013). Data protection working party opinion 03/2013 on purpose limitation [preprint]. Available at: https://iapp.org/media/pdf/resource_center/wp203_purpose-limitation_04-2013.pdf (Accessed November 10, 2023).
De Groen, W. P., Kilhoffer, Z., Lenaerts, K., and Mandl, I. (2018). Employment and working conditions of selected types of platform work [preprint]. Available at: https://core.ac.uk/download/pdf/219377081.pdf (Accessed September 23, 2023)
De Stefano, V. (2019). “negotiating the algorithm”: automation, artificial intelligence, and labor protection. Comp. Lab. L. and Pol'y J. 41:15. doi: 10.2139/ssrn.3178233
De Stefano, V. (2020). ‘Masters and servers’: collective labour rights and private government in the contemporary world of work. Int. J. Comparat. Labour Law Indust. Relat. 36, 425–444. doi: 10.54648/IJCL2020022
De Stefano, V., and Doellgast, V. (2023). Introduction to the transfer special issue. Regulating AI at work: labour relations, automation, and algorithmic management. Transfer 29, 9–20. doi: 10.1177/10242589231157656
De Stefano, V., and Wouters, M. (2022). AI and digital tools in workplace management and evaluation: an assessment of the EU's legal framework. Osgoode Legal Studies Research Paper. Available at: https://www.europarl.europa.eu/thinktank/en/document/EPRS_STU(2022)729516 (Accessed November 23, 2023).
Dehais, F., Lafont, A., Roy, R., and Fairclough, S. (2020). A neuroergonomics approach to mental workload, engagement and human performance. Front. Neurosci. 14:268. doi: 10.3389/fnins.2020.00268
Dell’Agnola, F., Jao, P. K., Arza, A., Chavarriaga, R., Millán, J. D. R., Floreano, D., et al. (2022). Machine-learning based monitoring of cognitive workload in rescue missions with drones. IEEE J. Biomed. Health Inform. 26, 4751–4762. doi: 10.1109/JBHI.2022.3186625
DGB. (2020). Artificial Intelligence (AI) for Good Work. Available at: https://www.dgb.de/downloadcenter/++co++b794879a-9f2e-11ea-a8e8-52540088cada (Accessed November 10, 2023).
EDPB. (2018). Legacy: art. 29 working party. Available at: https://www.edpb.europa.eu/about-edpb/who-we-are/legacy-art-29-working-party_en (Accessed November 26, 2023).
Eurofound. (2020). Co-determination. Available at: https://www.eurofound.europa.eu/en/european-industrial-relations-dictionary/co-determination (Accessed November 15, 2023).
European Agency for Safety and Health at Work. (2022). Summary—artificial intelligence for worker management: an overview. Available at: https://osha.europa.eu/en/publications/summary-artificial-intelligence-worker-management-overview (Accessed November 10, 2023).
European Commission. (2018). Guidelines on consent under regulation 2016/679 (wp259rev.01). Available at: https://ec.europa.eu/newsroom/article29/items/623051/en (Accessed December 10, 2023).
European Commission. (2021). Proposal for a directive of the European Parliament and of the council on improving working conditions in platform work. Available at: https://state-of-the-union.ec.europa.eu/state-union-2021_en (Accessed December 12, 2023).
European Court of Human Rights. (2022). Factsheet—surveillance at workplace. Available at: https://www.echr.coe.int/d/fs_workplace_surveillance_eng?p_l_back_url=%2Fsearch%3Fq%3Dprivacy%2Bcases%26folder%3D839313 (Accessed September 10, 2024).
European Union. (1989). Consolidated text: council directive of 12 June 1989 on the introduction of measures to encourage improvements in the safety and health of workers at work (89/391/EEC). Available at: https://eur-lex.europa.eu/legal-content/EN/TXT/?uri=CELEX%3A01989L0391-20081211&qid=1684159750691 (Accessed December 10, 2023).
European Union. (2002). Directive 2002/14/EC of the European Parliament and of the council of 11 march 2002 establishing a general framework for informing and consulting employees in the European Community—joint declaration of the European Parliament, the council and the commission on employee representation. Available at: https://eur-lex.europa.eu/legal-content/EN/ALL/?uri=CELEX%3A32002L0014 (Accessed December 18, 2023).
European Union. (2008). Consolidated text: council directive of 12 June 1989 on the introduction of measures to encourage improvements in the safety and health of workers at work (89/391/EEC). Available at: https://eur-lex.europa.eu/legal-content/EN/TXT/?uri=CELEX%3A01989L0391-20081211&qid=1684159750691 (Accessed December 10, 2023).
European Union. (2016). Regulation (EU) 2016/679 of the European Parliament and of the council of 27 April 2016 on the protection of natural persons with regard to the processing of personal data and on the free movement of such data, and repealing directive 95/46/EC (general data protection regulation) (text with EEA relevance). Available at: https://eur-lex.europa.eu/legal-content/EN/TXT/?uri=celex%3A32016R0679 (Accessed December 10, 2023).
European Union. (2024). Regulation (EU) 2024/1689 of the European Parliament and of the council of 13 June 2024 laying down harmonised rules on artificial intelligence and amending regulations (EC) no 300/2008, (EU) no 167/2013, (EU) no 168/2013, (EU) 2018/858, (EU) 2018/1139 and (EU) 2019/2144 and directives 2014/90/EU, (EU) 2016/797 and (EU) 2020/1828 (artificial intelligence act) (text with EEA relevance). Available at: http://data.europa.eu/eli/reg/2024/1689/oj (Accessed September 14, 2023).
Falque-Pierrotin, I. (2017). Article 29 data protection working party [preprint]. Available at: https://www.aepd.es/sites/default/files/2023-02/wp249-en-opinion-2-17-teleworking.pdf (Accessed September 27, 2023).
Farahany, N. A. (2023). The battle for your brain: defending the right to think freely in the age of neurotechnology. New York: St. Martin's Press.
Finn, E. S., Shen, X., Scheinost, D., Rosenberg, M. D., Huang, J., Chun, M. M., et al. (2015). Functional connectome fingerprinting: identifying individuals using patterns of brain connectivity. Nat. Neurosci. 18, 1664–1671. doi: 10.1038/nn.4135
Frey, C. B., and Osborne, M. A. (2017). The future of employment: how susceptible are jobs to computerisation? Technol. Forecast. Soc. Chang. 114, 254–280. doi: 10.1016/j.techfore.2016.08.019
Gilbert, A., and Thomas, A. (2021). The Amazonian era. How algorithmic systems are eroding good work [Preprint]. Available at: https://tfl.ams3.cdn.digitaloceanspaces.com/media/documents/The_Amazonian_Era_-_IFOW_report_May_2021pdf.pdf (Accessed November 15, 2023).
Gonfalonieri, A. (2020). What brain-computer interfaces could mean for the future of work. Available at: https://hbr.org/2020/10/what-brain-computer-interfaces-could-mean-for-the-future-of-work (Accessed December 10, 2023).
Gurley, L. K. (2022). Internal documents show Amazon’s dystopian system for tracking workers every minute of their shifts. Available at: https://www.vice.com/en/article/5dgn73/internal-documents-show-amazons-dystopian-system-for-tracking-workers-every-minute-of-their-shifts (Accessed November 15, 2023).
Hussein, R. M., Miften, F. S., and George, L. E. (2023). Driver drowsiness detection methods using EEG signals: a systematic review. Comput. Methods Biomech. Biomed. Engin. 26, 1237–1249. doi: 10.1080/10255842.2022.2112574
ICO. (2023). ICO tech futures: Neurotechnology. Available at: https://ico.org.uk/about-the-ico/research-and-reports/ico-tech-futures-neurotechnology/ (Accessed November 15, 2023).
Ienca, M., Fins, J. J., Jox, R. J., Jotterand, F., Voeneky, S., Andorno, R., et al. (2022). Towards a governance framework for brain data. Neuroethics 15:20. doi: 10.1007/s12152-022-09498-8
Ienca, M., and Malgieri, G. (2022). Mental data protection and the GDPR. J. Law Biosci. 9:lsac006. doi: 10.1093/jlb/lsac006
IndustriAll European Trade Union. (2022). Artificial intelligence as a challenge and an opportunity for workers and their representatives [preprint]. Available at: https://news.industriall-europe.eu/documents/upload/2022/6/637897670199433879_dopted%20-%20All%20eyes%20on%20AI.%20Artificial%20Intelligence%20as%20a%20challenge%20for%20workers%20and%20their%20representatives%20-%20EN.pdf (Accessed November 17, 2023).
Insel, T. R. (2017). Digital phenotyping: technology for a new science of behavior. JAMA 318, 1215–1216. doi: 10.1001/jama.2017.11295
Loi, M. (2019). The digital phenotype: a philosophical and ethical exploration. Philos. Technol. 32, 155–171. doi: 10.1007/s13347-018-0319-1
Maior, H. A., Wilson, M. L., and Sharples, S. (2018). Workload alerts—using physiological measures of mental workload to provide feedback during tasks. ACM Trans. Comput. Hum. Interact. 25, 1–30. doi: 10.1145/3173380
McKenzie, Baker. (2024). European Union: platform workers directive goes ahead—presumption of employment and regulation of algorithmic management in platform work. Available at: https://www.globalcompliancenews.com/2024/04/03/https-insightplus-bakermckenzie-com-bm-investigations-compliance-ethics-european-union-platform-workers-directive-goes-ahead-presumption-of-employment-and-regulation-of-algorithmic-management-in-pla/ (Accessed September 10, 2023).
Mitler, M. M., Carskadon, M. A., Czeisier, C. A., Dement, W. C., Dinges, D. F., and Graeber, R. C. (1988). Catastrophes, sleep, and public policy: consensus report. Sleep 11, 100–109. doi: 10.1093/sleep/11.1.100
Moore, P. V. (2020). Data subjects, digital surveillance, AI and the future of work: study. European Parliament. Available at: https://www.europarl.europa.eu/stoa/en/document/EPRS_STU(2020)656305 (Accessed November 23, 2023).
Muhl, E., and Andorno, R. (2023). Neurosurveillance in the workplace: do employers have the right to monitor employees' minds? Front. Hum. Dyn. 5:1245619. doi: 10.3389/fhumd.2023.1245619
Muñoz, J. M., Borbón, D., and Bezerra, A. M. (2024). Chapter three—computational psychiatry and digital phenotyping: ethical and neurorights implications. Dev. Neuroeth. Bioeth. 7, 49–63. doi: 10.1016/bs.dnb.2024.02.005
Newman, D. (2020). Emotional recognition tech — is it dangerous to the recruitment process? Available at: https://fowmedia.com/emotional-recognition-tech-dangerous-to-recruitment-process/ (Accessed September 10, 2023).
Niso, G., Romero, E., Moreau, J. T., Araujo, A., and Krol, L. R. (2023). Wireless EEG: a survey of systems and studies. NeuroImage 269:119774. doi: 10.1016/j.neuroimage.2022.119774
Rainey, S., McGillivray, K., Akintoye, S., Fothergill, T., Bublitz, C., and Stahl, B. (2020). Is the European data protection regulation sufficient to deal with emerging data concerns relating to neurotechnology? J. Law Biosci. 7:lsaa051. doi: 10.1093/jlb/lsaa051
Ramos, P. M., Maior, C. B., Moura, M. C., and Lins, I. D. (2022). Automatic drowsiness detection for safety-critical operations using ensemble models and EEG signals. Process Saf. Environ. Prot. 164, 566–581. doi: 10.1016/j.psep.2022.06.039
Respublique Francaise. (2008). Code du travail Partie législative (Articles L1 à L8331-1). Available at: https://www.legifrance.gouv.fr/codes/article_lc/LEGIARTI000006900861 (Accessed November 10, 2023).
Riso, S. (2023). Monitoring and surveillance of workers in the digital age. Available at: https://www.eurofound.europa.eu/data/digitalisation/research-digests/monitoring-and-surveillance-of-workers-in-the-digital-age (Accessed December 10, 2023).
Sartor, G. (2020). The impact of the general data protection regulation (GDPR) on artificial intelligence: study. European Parliament. Available at: https://www.europarl.europa.eu/stoa/en/document/EPRS_STU(2020)641530 (Accessed December 12, 2023).
Solove, D. J. (2023). Data is what data does: regulating based on harm and risk instead of sensitive data. Nw. UL Rev. 118:1081. doi: 10.2139/ssrn.4322198
Vallas, S., and Schor, J. B. (2020). What do platforms do? Understanding the gig economy. Annu. Rev. Sociol. 46, 273–294. doi: 10.1146/annurev-soc-121919-054857
Wachter, S., and Mittelstadt, B. (2019). A right to reasonable inferences: re-thinking data protection law in the age of big data and AI [preprint]. Available at: https://papers.ssrn.com/sol3/papers.cfm?abstract_id=3248829# (Accessed November 27, 2023).
Wachter, S., Mittelstadt, B., and Russell, C. (2017). Counterfactual explanations without opening the black box: automated decisions and the GDPR. Harv. JL Tech. 31, 841–887. doi: 10.2139/ssrn.3063289
Wenco. (2023). Wearable technology that eliminates fatigue incidents. Available at: https://www.smartcaptech.com/industries/mining/ (Accessed November 1, 2023).
Wexler, A., and Reiner, P. B. (2019). Oversight of direct-to-consumer neurotechnologies. Science 363, 234–235. doi: 10.1126/science.aav0223
Zazon, D., Fink, L., Gordon, S., and Nissim, N. (2023). Can NeuroIS improve executive employee recruitment? Classifying levels of executive functions using resting state EEG and data science methods. Decis. Support. Syst. 168:113930. doi: 10.1016/j.dss.2023.113930
Keywords: neurosurveillance, brain data, mental data, AI act, GDPR, employers’ rights
Citation: Muhl E (2024) The challenge of wearable neurodevices for workplace monitoring: an EU legal perspective. Front. Hum. Dyn. 6:1473893. doi: 10.3389/fhumd.2024.1473893
Received: 31 July 2024; Accepted: 25 September 2024;
Published: 03 October 2024.
Edited by:
Agusti Solanas, University of Rovira i Virgili, SpainReviewed by:
Diego Borbón, Universidad Externado de Colombia, ColombiaCopyright © 2024 Muhl. This is an open-access article distributed under the terms of the Creative Commons Attribution License (CC BY). The use, distribution or reproduction in other forums is permitted, provided the original author(s) and the copyright owner(s) are credited and that the original publication in this journal is cited, in accordance with accepted academic practice. No use, distribution or reproduction is permitted which does not comply with these terms.
*Correspondence: Ekaterina Muhl, ekaterina.tikhomirova@ibme.uzh.ch
Disclaimer: All claims expressed in this article are solely those of the authors and do not necessarily represent those of their affiliated organizations, or those of the publisher, the editors and the reviewers. Any product that may be evaluated in this article or claim that may be made by its manufacturer is not guaranteed or endorsed by the publisher.
Research integrity at Frontiers
Learn more about the work of our research integrity team to safeguard the quality of each article we publish.