- 1First Street, New York, NY, United States
- 2Mailman School of Public Health, Columbia University, New York, NY, United States
This study examines how population change is associated with changes in sociodemographics and economic outcomes across diverse geographic contexts in the United States from 2000 to 2020. Using Census Tract-level data and generalized additive models (GAMs), we found that communities experiencing population growth showed significant improvements in socioeconomic indicators: for example, a 50% population increase in Northeast metropolitan non-coastal areas was associated with a $10,062 rise [95% confidence interval (CI) = $9,181, $10,944] in median household income. Conversely, areas with population decline faced increasing challenges to community composition: communities experiencing a 50% population decline in West coastal metropolitan areas saw their median age increase by 2.556 years (95% CI = 2.23, 2.89 years), indicating an accelerated aging population. We observed a positive relationship between population growth and local economic growth, with areas experiencing population decline or slow growth showing below-average economic growth. While population change alone explained 10.1% of the variance in county-level GDP growth, incorporating sociodemographic shifts alongside population change using a partial least squares regression (PLSR) more than doubled the explanatory power to 21.4%. Overall, we often found the strength of relationships and sometimes the direction varied by geographic context: coastal areas showed distinct patterns from inland regions, and metropolitan areas responded differently than rural ones. For instance, the percentage of owner-occupied housing was negatively associated with population growth in metropolitan areas, but positively associated in non-metropolitan areas. Our research provides valuable insights for policymakers and planners working to address community changes, particularly in the context of anticipated climate-induced migration. The results suggest that strategies for maintaining economic vitality need to consider not just population retention, but also demographic profiles and socioeconomic opportunities across different geographic contexts.
1 Introduction
Communities are fundamentally shaped by the dynamic interplay between population changes and sociodemographic characteristics within their unique geographic contexts. Population changes trigger distinct patterns of socioeconomic and demographic transformation, generating complex relationships that influence economic outcomes, social structures, and community resilience. For policymakers, urban planners, and community stakeholders, understanding the relationships between population change, demographic composition, and economic outcomes is essential for anticipating future population shifts and their transformative effects on communities.
Migration patterns, a critical driver of population change, have historically exhibited strong demographic selectivity in the U.S. Young adults show the highest mobility rates, often coinciding with educational and career transitions (Bernard et al., 2014; Plane and Jurjevich, 2009). Educational attainment strongly predicts geographic mobility (Kennan and Walker, 2011), contributing to the concentration of young, educated populations in certain areas (Whisler et al., 2008; Abel and Deitz, 2012). Income levels also play a crucial role, with higher-income households showing greater mobility rates and often moving toward areas with stronger economic opportunities and amenities (Chen and Rosenthal, 2008; Molloy et al., 2011). Conversely, lower-income households frequently face mobility constraints and tend to move shorter distances, often within the same metropolitan area (Diamond, 2016). These selective migration patterns can accelerate demographic transitions, leading to faster aging and socioeconomic changes in origin communities while destination areas experience the reverse (Johnson and Winkler, 2015).
Changes in the socioeconomic and demographic fabric of communities in response to changing populations also have implications on community economic outcomes (Kemeny and Storper, 2012). Disparate growths and declines in population across a community’s demographics have differing effects on its labor force, housing markets, and local business environments (Howard, 2020; Zabel, 2012; Glaeser and Gottlieb, 2009). Population inflows can stimulate economic growth through increased consumer spending and tax revenues, while rapid outflows may strain municipal budgets and reduce business activity (Buettner and Holm-Hadulla, 2013; Duranton and Puga, 2014; Adelino et al., 2017).
Notably, sociodemographic transitions are influenced by the geographic context in which they take place, including urban–rural divides, amenity profiles, and regional characteristics. Studies have shown that migration flows of certain demographic groups vary substantially between metropolitan and non-metropolitan areas, leading to distinct demographic compositions of populations in these areas (Ambinakudige and Parisi, 2017; Winkler et al., 2015). In most cases, rural-to-urban migration has historically contributed to the growth and diversification of cities while also exacerbating population decline and aging in many rural communities (Johnson and Lichter, 2019). At the same time, metro versus non-metro migration patterns have been found to vary regionally across the U.S., with the Northeast experiencing more dramatic shifts in population (Johnson and Cromartie, 2006; Rickman and Wang, 2017). Similarly, natural amenities, such as mild climates and coastal proximity, have emerged as important drivers of migration among particular demographic groups (McGranahan, 1999; Gosnell and Abrams, 2011). For example, amenity-driven migration has led to rapid population growth in some rural areas, such as the Rocky Mountain West and the Upper Great Lakes, while other rural regions have continued to experience population decline (Johnson and Beale, 2002).
Climate change has introduced a new dimension to traditional population dynamics experienced in the U.S., as recent years have witnessed the consequences of climate change on people’s lives with instances of property damage, property value impacts, insurance implications, and changing migration patterns unfolding nationwide (McAlpine and Porter, 2018; Keenan et al., 2018; Hauer et al., 2020; Armal et al., 2020; Keys and Mulder, 2024). The geographic dimension of population dynamics comes into play when considering climate change’s regional impacts across the U.S.: coastal areas face tropical cyclones and storm surge, the Midwest and Northeast experience increasing extreme precipitation (First Street, 2023), the central U.S. faces extreme heat exposure (Wilson et al., 2022), and the Western region confronts escalating wildfire risks (Kearns et al., 2022). As these climate-related impacts mount, an increasing number of individuals and communities are considering relocation as an adaptation strategy, giving rise to the phenomenon of climate migration. A growing body of literature has emerged to investigate this phenomenon, in which a combination of exposure, vulnerability, and perceived risk associated with climate hazards has already driven, or is expected to drive, individuals and families to relocate within the United States (Hauer et al., 2016; Hauer et al., 2017; Robinson et al., 2020; Hauer et al., 2021; Shu et al., 2023), Studies have found that climate migration often follows pathways similar to traditional migration factors, as climate impacts compound with existing socioeconomic drivers such as job opportunities, amenities, and affordability (Black et al., 2011; Fan et al., 2018). Other studies suggest that the ability to relocate in response to environmental pressures is often contingent not only on socioeconomic status but also demographic factors (Fothergill et al., 1999; Fussell et al., 2010; Raker, 2020; Kaczan and Orgill-Meyer, 2020).
This suggests that climate change may create distinct migratory patterns that vary across sociodemographic groups, potentially reshaping the composition of populations in both origin and destination communities. For example, coastal areas vulnerable to flooding may experience accelerated population aging as younger residents relocate while older populations remain (Hauer et al., 2024). Research has also found that Black and Hispanic populations face disproportionate risks of isolation from essential services in areas threatened by sea-level rise, potentially lacking resources for relocation (Best et al., 2023). Climate migration’s influence on population and sociodemographic dynamics may also trigger cascading economic effects. Changes in community composition can alter consumption patterns, labor force characteristics, and entrepreneurial activities (Liang et al., 2018; Goetz et al., 2010; Aguiar and Hurst, 2013). The loss of population in climate-vulnerable areas could create feedback loops of further out-migration and economic decline through reduced public services and a shrinking tax base (Hauer et al., 2016; Fan et al., 2018).
Exploring how sociodemographics move with changes in population and the resulting impacts on local economies serves as the next step in a growing body of literature on climate migration. Furthermore, estimating the derivative impacts of climate migration presents critical information for academic researchers, policymakers, industry stakeholders, and others when considering the human dimensions of climate adaptation.
This paper seeks to explore the multi-faceted effects of population change on the composition and economic outcome of communities across the U.S. in geographic context. As such, it also serves as a stepping stone toward understanding the scope of climate migration as it pertains to anticipating the demographic and socioeconomic characteristics of communities experiencing climate-induced population change and how these interact with changes in local Gross Domestic Product (GDP). To achieve this, we derive the historical relationships between population change, sociodemographic shifts, and GDP growth over a 20-year time period as proxies for anticipating the impacts of future climate migration on community profiles and economic viability. Among the sociodemographic characteristics modeled, we examine demographic and socioeconomic attributes from U.S. Census data including age, education, income, housing, race and ethnicity, and occupation. Pairwise cubic spline models are used to flexibly trace how sociodemographic and economic outcomes have historically moved with population growth or decline based on observed trends across the entire universe of population and selected sociodemographic characteristics, and to further serve as predictions for how they may continue to move with future population change. These relationships are also explored in aggregate by estimating composite scores of the general direction and magnitude of how sociodemographic and population interact with each other and against GDP. Our study evaluates these relationships within geographic context by defining a novel set of geographic categories along regional, urban, and coastal divides, characteristic of both the spatial characteristics of population dynamics and climate impacts across the contiguous U.S. Our approach to disaggregating relationships by geographic category leads to more accurate profiles of anticipated changes as population changes across areas of the U.S. We elaborate on our data sources, data and statistical analysis, and results in the sections that follow.
2 Materials and methods
Our two main objectives for this study were to (A) evaluate the relationship between population change and shifts in community sociodemographics, and (B) evaluate the downstream implications of these combined population and sociodemographic changes on economic outcomes (Figure 1). These relationships were evaluated across the contiguous U.S. from 2000 to 2020. Since these relationships likely vary by local context, influenced by regional, urban, and coastal trends, we evaluated these relationships separately by geographic context.
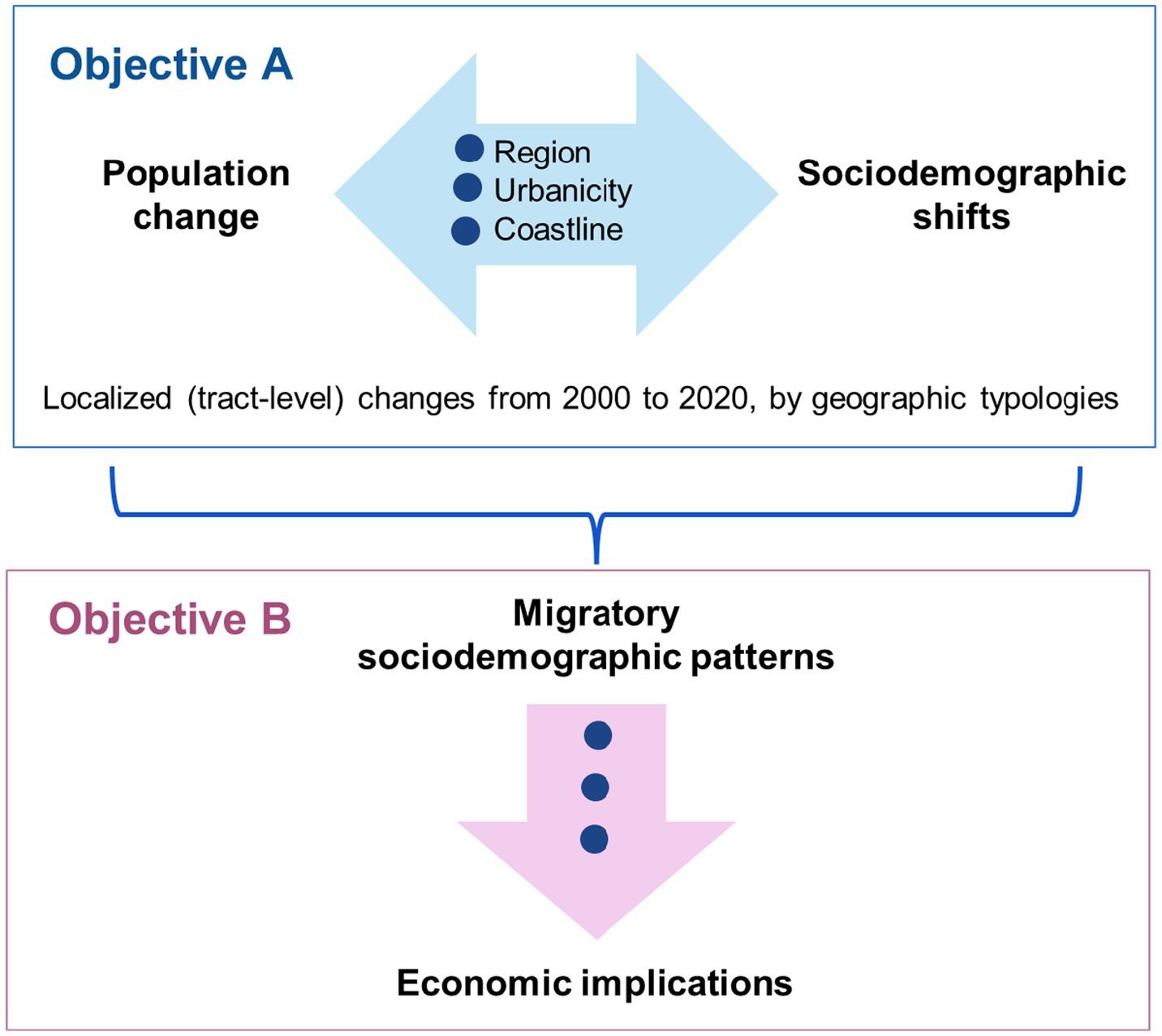
Figure 1. Conceptual framework and objectives for this study. Object A: evaluate the relationship between local changes in population and sociodemographics using historical Census Tract-level data from the U.S. Census Bureau from 2000 to 2020. Object B: investigate how population change, and sociodemographic shifts related to population change, are associated with economic growth, as measured by county-level gross domestic product (GDP) from the U.S. Bureau of Economic Analysis.
2.1 Data sets
2.1.1 Population and sociodemographic information
We collected population and sociodemographic data at the Census Tract-level, the highest resolution available for this information, from the U.S. Census Bureau for decades 2000 and 2020. Data was primarily acquired from the Decennial Census, however, many socioeconomic variables for 2020 were obtained from the 5-year American Community Survey (ACS). Corresponding variables for each time period and specific dataset sources are listed in Supplementary Table 1. Variables evaluated in this study include age, race and ethnicity, sex, income, poverty, education, employment, occupation, housing occupancy and cost (including homeownership and rental costs), and immigration (Table 1). A further table summarizing the percentages of the population and counts for categorical variables (i.e., age group) as well as the mean and standard deviation for continuous variables (i.e., median age) across both 2000 and 2020 are provided in Supplementary Table 2.
For comparability across the two decades, data for 2000 and 2020 were reallocated to the geographic boundaries of 2010 Census Tracts using crosswalks from the Longitudinal Tract Database (LTDB) and the National Historical Geographic Information System (NHGIS). Once data from 2000 and 2020 were reconciled to comparable geographic units of 2010 Census Tracts, we computed changes in population and demographics/socioeconomics over the past two decades. Population change was expressed as a growth rate (%), indicating declines or increases from a “no change” scenario of 0, calculated as:
Changes in sociodemographic variables, either expressed as a percentage or median value (e.g., median age, median income), were computed using a straightforward difference from 2000 to 2020:
Where is either expressed as a percentage or median value.
2.1.2 Economic information
Gross domestic product (GDP) data were collected from the U.S. Bureau of Economic Analysis (BEA)'s Regional Accounts to assess the association of population and sociodemographic changes with local economic viability. Annual county-level data since 2001 were obtained in real 2012 dollars.
To account for variability in annual county GDP estimates, we computed three-year averages. Specifically, average county GDP for 2001–2003 and 2017–2019 were used to calculate the GDP growth rate:
We also tested one-year point estimates and five-year averages, finding similar, but somewhat weaker relationships with population and sociodemographics. We did not include GDP from 2020 to avoid the economic impacts of the COVID-19 pandemic.
2.2 Geographic framework
To explore the relationships under study by geographic context, we divided Census Tracts into Region-Urbanicity-Coastline (RUC) categories, capturing amenity profiles of geographies across the country based on these three dimensions. This approach is grounded in research demonstrating that migration patterns are not uniformly distributed across geographic locations or demographic groups, but depend on various factors such as urbanicity, amenity profile, and regional characteristics (Johnson et al., 2005; Johnson and Winkler, 2015; Johnson et al., 2013; Rappaport, 2007). The three dimensions are:
1. Macro region (Northeast, South, Midwest, and West)
2. Urbanicity (metropolitan and nonmetropolitan) using Rural–Urban Continuum Codes (RUCC) for counties in 2013 (USDA, 2024). In this categorization, counties that are identified as part of a Metropolitan Statistical Area (MSA) are categorized as “metropolitan” and all other counties classified as “nonmetropolitan.”
3. Coastal proximity (coastal and non-coastal) for metropolitan areas, based on whether the county border touches the coastline. There were a limited number of non-metropolitan tracts on the coastline (<1%).
These three dimensions constitute 11 distinct categories that encompass the diverse geographic contexts across the United States (Figure 2).
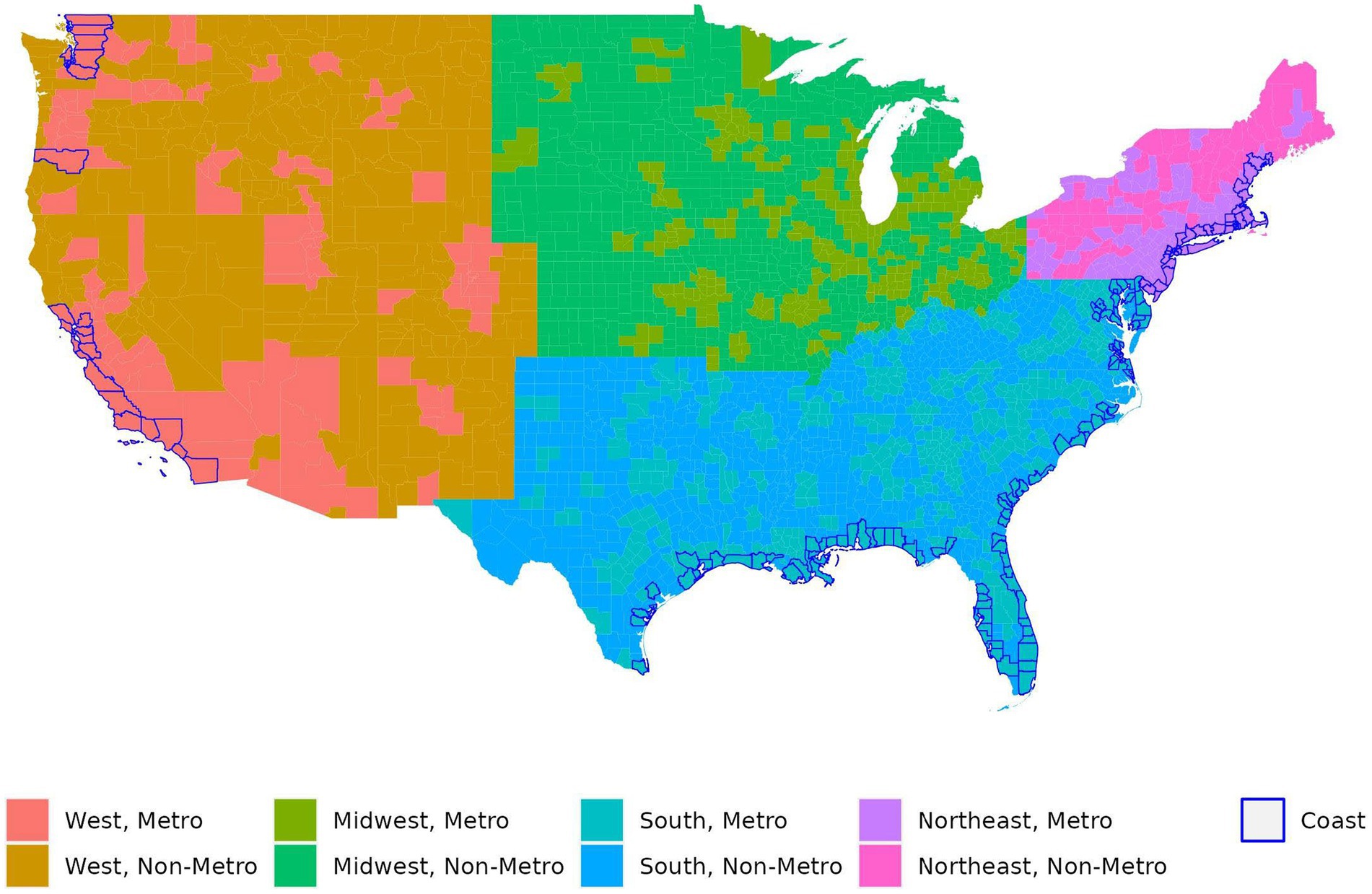
Figure 2. Map of the contiguous U.S. colored by Region-Urbanicity-Coastline categories. Coastline status was distinguished for metropolitan areas only, as the number of non-metropolitan Census Tracts on the coastline were limited. There are no coastal counties in the Midwest.
2.3 Statistical analysis
2.3.1 Population-sociodemographic relationships
We examined how population change is related to various sociodemographic changes across different geographic contexts using two approaches:
First, we evaluated the association between population change and individual sociodemographic factors separately for each RUC classification. To account for potentially non-linear relationships, we modeled population change using a flexible cubic spline with three knots within a generalized additive model (GAM). From each fitted GAM, we derived predicted values of demographic change across the distribution of population change. Predicted sociodemographic changes were centered to a reference point of no population change and plotted along with their 95% confidence intervals (95% CIs). This allows for a clear interpretation of the expected change in a sociodemographic characteristic for a given value of population decline or growth, relative to no growth. Almost all sociodemographic variables had less than 1% missing data; median rent and median value of owner-occupied units was missing about 2% of data.
We generally found relationships between population change and sociodemographics were linear. For ease of interpretation, we also plotted coefficients from simple linear models of population change and individual sociodemographic factors using a heat map. These maps allowed us to compare the magnitude and direction of the relationship between population change and groupings of sociodemographic variables, such as age categories, income brackets, and educational attainment levels, across the RUC continuum.
Our second approach evaluated the collective relationship between multiple sociodemographics and population change using a partial least squares regression (PLSR). We use PLSR to understand patterns among multiple related sociodemographic variables in association with population change. This approach is particularly suited for this analysis due to its ability to handle collinearity between sociodemographic variables that tend to cluster together in communities (e.g., educational attainment and median income are often strongly correlated), and to understand the collective relationship with population change. Sociodemographics in our PLSR model include the difference (2020–2000) in median household income ($), % Bachelor’s degree or higher, median rent ($), median value of owner-occupied housing units ($), % housing units that are owner-occupied, % individuals below the poverty line, % Hispanic, % Black or African American, % Asian, % White, median age (years), % female, % employed, % in a professional occupation, % in a labor occupation, and % who speak limited English. Population change was included as the outcome. A correlation matrix of variables included in our analysis is presented in Supplementary Figure 1. Similar to our GAMs approach, we modeled the relationship between population change and sociodemographic characteristics according to RUC classification. Sociodemographic variables were scaled before analysis to ensure all variables contributed equally to the PLSR model regardless of their original units of measurement (e.g., dollars for income, years for age, percentages for educational attainment). We focused on evaluating relationships within the first component, which explains the maximum variance in sociodemographics and the relationship with population change. The results include a loading, or weight, that is estimated for each sociodemographic variable, indicating the magnitude and direction of the relationship with population change. Sociodemographics with a positive loading are associated with population growth, whereas variables with a negative loading are associated with population decline.
2.3.2 Migratory sociodemographic patterns and economic implications
To meet our second objective, we sought to evaluate the association of (1) population change, and (2) collective population and sociodemographic changes, with local economics, as measured by GDP change. Since GDP is available at the county-level, population and sociodemographic data was aggregated to the county-level from the Census Tract-level.
In this analysis, we also took two approaches, first using a simple linear regression to explore the relationship between population change and GDP alone and second using PLSR, including population change and all sociodemographics listed above, to evaluate the collective impact on GDP. Again, we assessed these relationships separately for each RUC classification. The loadings from the PLSR model were used to produce a composite score for each county, efficiently capturing the collective pattern of population and sociodemographic changes in relation to GDP change. A positive score generally indicates that the county experienced favorable changes in population and sociodemographics associated with GDP growth (i.e., increases in positively-loaded variables, and decreases in negatively-loaded variables). A negative score indicates unfavorable changes in population and sociodemographics associated with GDP decline (i.e., decreases in positively-loaded variables, and increases in negatively-loaded variables).
We compared the R2, or percent of GDP variance explained, from the two models to quantify how much additional GDP variance was explained by sociodemographic variables beyond population change alone. We hypothesized that the combination of both population and sociodemographic changes, as expressed with the composite score, versus population change alone would better explain GDP change.
3 Results
3.1 Relationship between population and sociodemographic change
The results from our GAMs provide flexible predictions about the outcome of each sociodemographic variable along the distribution of possible population changes for each RUC. We provide example interpretations of the relationship between population change and select sociodemographics to highlight the application of our models in anticipating the direction and magnitude of sociodemographic outcomes as populations grow or decline within RUC continuums.
3.2 Socioeconomics
Median household income showed a clear positive relationship with population growth across RUCs, with the weakest relationship observed in Northeast coastal areas as presented in Figure 3. For instance, in Northeast metro coastal areas with a 50% population increase, median household income rose by $3,680 (95% CI: $2,665, $4,695), compared to $10,062 (95% CI: $9,181, $10,944) in Northeast metro non-coastal areas. Further analysis of income brackets using linear regressions presented in Supplementary Figure 2 revealed that the positive relationships across RUCs were primarily associated with households earning $100,000 and above. A tipping point was observed where households earning $100,000 or more showed positive growth across most RUCs, with the strongest association with population change being observed for households earning over $200,000. Conversely, households in the $15,000–$99,000 range exhibited negative relationships with population change.
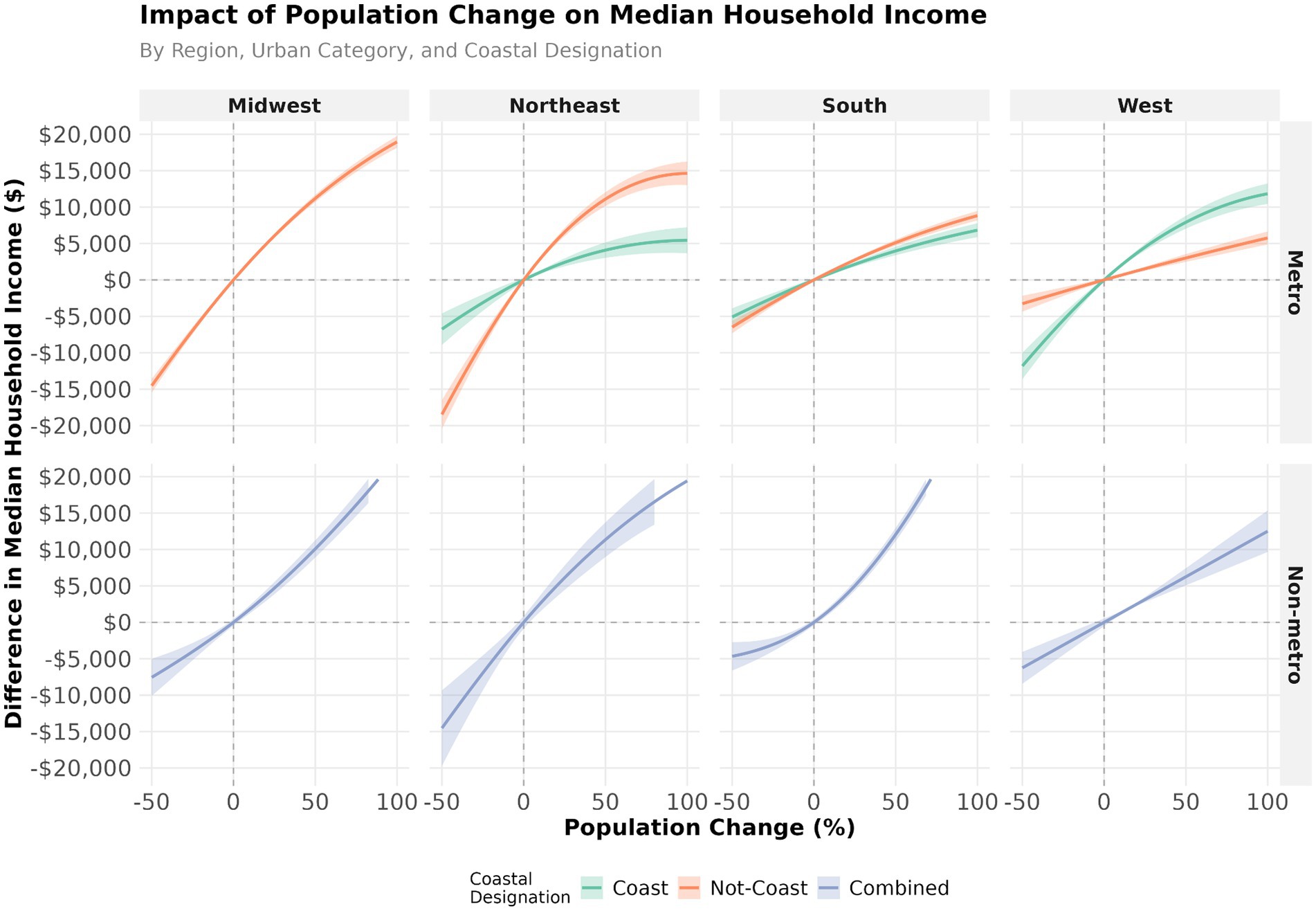
Figure 3. The relationship between population change and median household income for U.S. Census Tracts from 2000 to 2020, analyzed separately across Region-Urbanicity-Coastline categories. Population change modeled using a cubic spline. X-axis is the population growth factor, where values below 0 indicate a population decline and values above 0 indicate population growth (e.g.100 represents a 2-fold increase). Y-axis is the expected difference in median household income from 2000 to 2020 for a given value of population change, relative to no population change.
Other socioeconomic characteristics such as educational attainment, median rent, and median home value of owner-occupied units, showed mostly positive associations with population growth across RUCs, though the magnitude varied by classification. The results for these variables are visualized in Supplementary Figures 3–5.
3.3 Demographics
We observed a clear inverse relationship between population change and median age across all RUCs as presented in Supplementary Figure 6. Census Tracts experiencing population decline showed an increase in median age, while those with population growth exhibited a decrease, at varying rates across regions, urbanicity, and coastal areas. In non-coastal metropolitan areas of the West that experienced a 50% population decline, the median age increased by 0.68 years (95% CI: 0.46, 0.89), relative to no population change. This trend was more pronounced in coastal areas of the metropolitan West, with a median age increase of 2.56 years (95% CI: 2.23, 2.89).
When examining the relationship between population change and specific age groups, distinct patterns emerged as shown in Figure 4. A notable increase in the 55+ years age group was observed in areas with declining populations on the West and Northeast metropolitan coastlines. Specifically, every 1% decrease in population was associated with a 1.38% (SE = 0.0009) increase in the population aged 65–74 in West metro coastlines and a 2.09% (SE = 0.0013) increase in the same group in Northeast metro coastlines. These relationships across coastlines were stronger than their non-coastline counterparts.
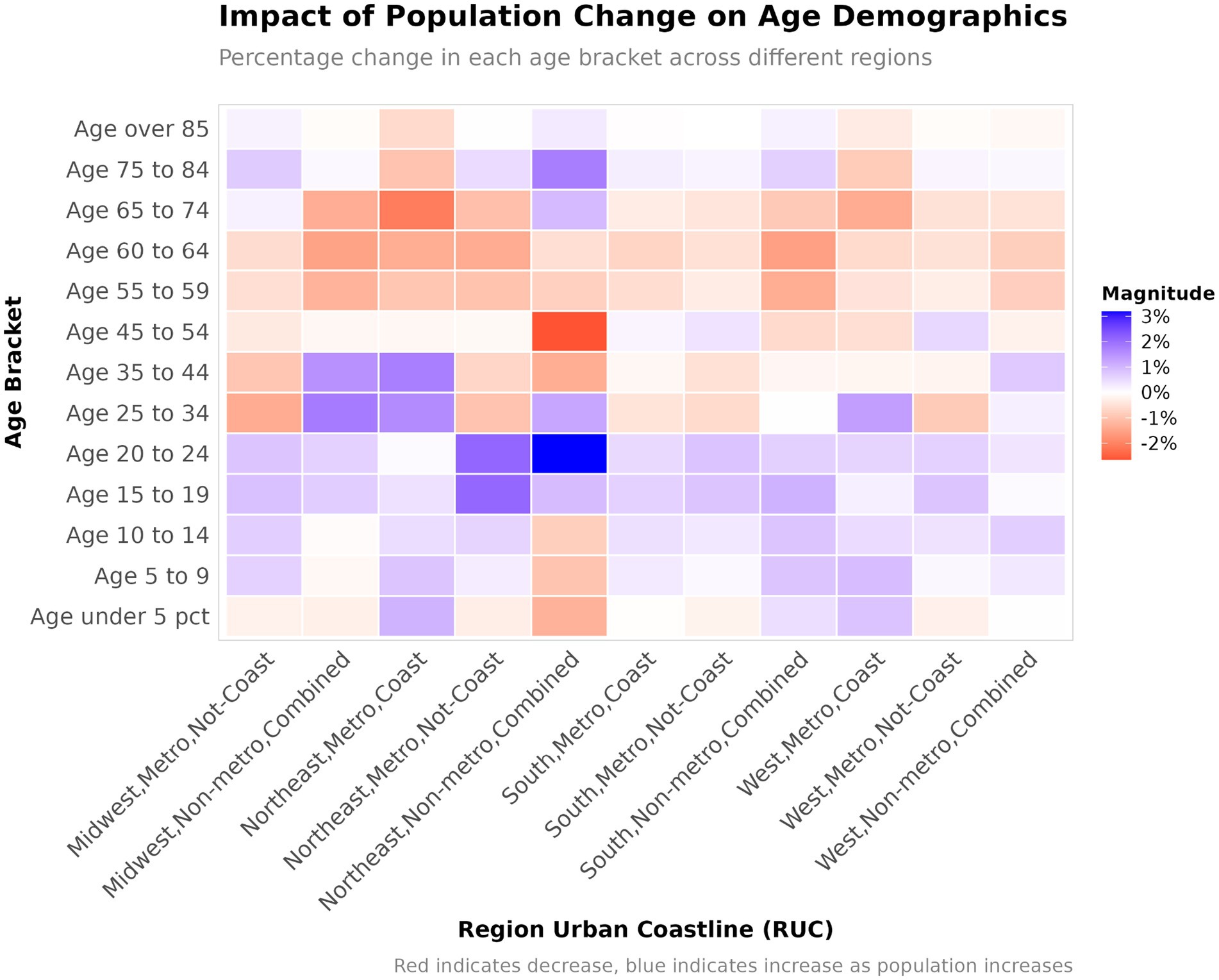
Figure 4. Heatmap of linear relationships between population change and age groups across the Region-Urbanicity-Coastline categories. Positive relationships are color-coded as blue, showing the expected percentage increase of each age group per one-unit increase in population growth. Negative associations are displayed in red. Magnitudes are in percent form and represent the change in each age group relative to a 1% change in population.
As population increased, so did the percentage of younger working age groups aged 15 to 44 years old, with the strongest patterns observed in the Northeast. A tipping point was noted near 45 years and older, where population growth showed a negative relationship with older age groups. Other demographic characteristics exhibited varied trends across RUC classifications. Supplementary Figure 7 demonstrates this in a panel of results visualized across race and ethnicity categories.
3.4 Composite relationships between sociodemographic and population change
3.4.1 Population-sociodemographic relationships
The PLSR analysis between sociodemographic variables and population change revealed patterns that largely corroborated the trends observed in the initial pairwise analyses, while also highlighting some important distinctions. The following section qualitatively summarizes noteworthy deviations from and reinforcements of our pairwise results. The loading chart for the importance of sociodemographic factors on population change is presented in Figure 5. For a closer look of how loadings for each sociodemographic characteristic compare across RUC classifications, see Supplementary Figure 8.
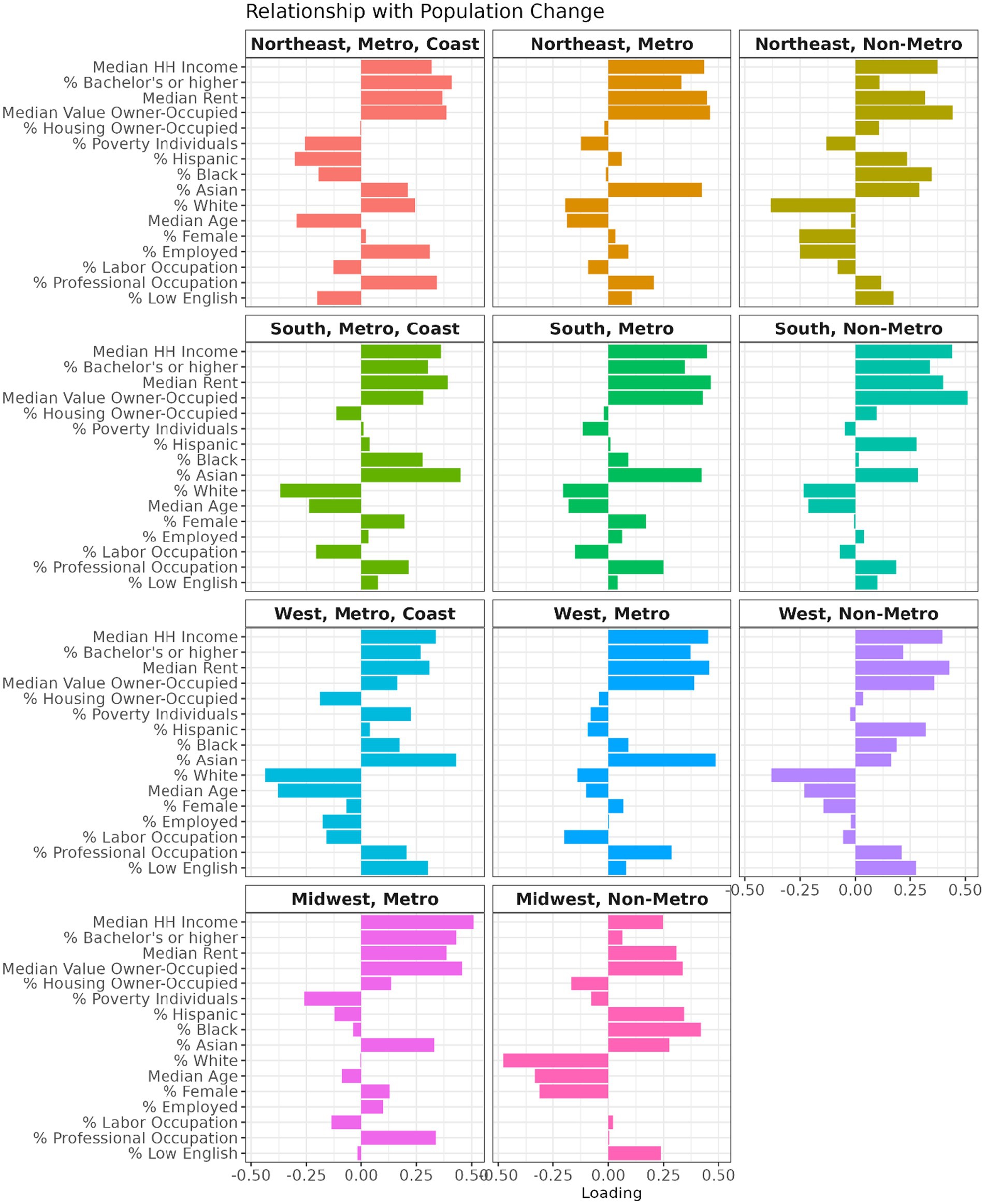
Figure 5. Results from the partial least squares regression (PLSR) showing the relationship between changes in sociodemographics on population change, separately by Region-Urbanicity-Coastline categories. Among 3,024 counties with complete information (96% of 3,143 counties in the contiguous U.S.). A loading, or weight, is shown for each sociodemographic variable, indicating the magnitude and direction of the relationship with population. Variables with a positive loading are associated with increases in population (right-hand side of plots), whereas variables with a negative loading are associated with decreases in population (left-hand side of plots). R2, or % variance explained, for population is shown in the top corner of each plot.
Socioeconomic factors showed the strongest associations with population change compared to demographics. Median household income, median rent, median value of owner-occupied housing, and educational attainment consistently displayed the highest positive loadings across RUC categories, reinforcing their robust positive association with population growth observed in the pairwise models. Some new insights were gleaned from the strength of each characteristic’s association with population growth, as signaled by its loading score. For example, in Northeast metropolitan coastlines, educational attainment emerged as the strongest predictor among all sociodemographic factors, a distinction that is not apparent in pairwise models. We observed a consistently negative association between poverty rates and population change for all RUCs, except West and South metro coastal areas (Supplementary Figure 8).
Housing tenure patterns revealed interesting urban–rural divides. The percentage of owner-occupied housing showed negative loadings in metropolitan RUCs and positive loadings in non-metropolitan RUCs (with the Midwest as an exception), suggesting different dynamics in housing markets and population change between urban and rural areas.
Occupational characteristics demonstrated clearer patterns in the PLSR analysis, with professional occupations consistently showing positive loadings and labor-intensive occupations generally displaying negative loadings across RUCs.
Racial and ethnic composition showed varied relationships across RUCs and categories in the PLSR analysis similar to the associations observed in the pairwise models. While the percentage of Asian population consistently displayed positive loadings, the relationships for other racial/ethnic groups varied more by region in the PLSR results. Percentage Black or Hispanic was generally positively associated with population change, highlighting the diversification of growing communities. However, these same relationships were negative in Northeast metropolitan coastal areas, and to a lesser extent the West and Midwest metropolitan, potentially reflecting social inequities that may affect the ability to relocate. Other demographics, like median age, showed a consistently negative relationship with population change across all RUCs in both analyses, reinforcing this robust finding observed in our pairwise analysis.
3.4.2 Migratory sociodemographic patterns and economic outcomes
The secondary PLSR analysis, which included population change and sociodemographic variables as predictors of GDP change, revealed additional insights about how a combination of these factors are associated with economic output as seen in Figure 6. See Supplementary Figure 9 for a closer comparison across RUC classifications.
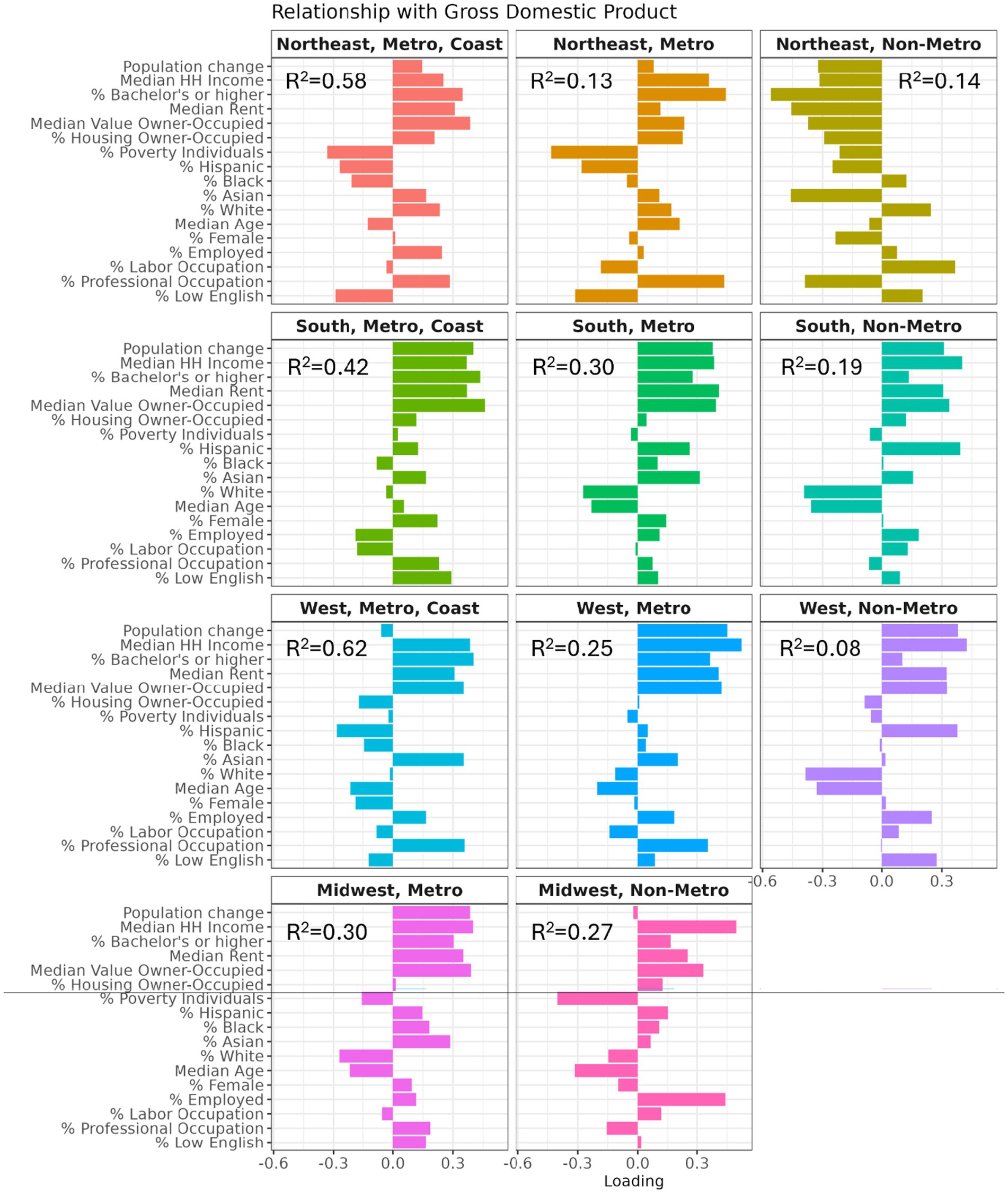
Figure 6. Results from the partial least squares (PLS) regression showing the relationship between collective changes in population and sociodemographics on GDP change (average 2001–2003 to 2017–2019), separately by Region-Urbanicity-Coastline categories. Among 3,024 counties with complete information (96% of 3,143 counties in the contiguous US). A loading, or weight, is shown for population change and each sociodemographic variable, indicating the magnitude and direction of the relationship with GDP. Variables with a positive loading are associated with increases in GDP (right-hand side of plots), whereas variables with a negative loading are associated with decreases in GDP (left-hand side of plots). R2, or % variance explained, for GDP is shown in the top corner of each plot.
Population change itself demonstrated a positive relationship with GDP across most RUCs, with particularly strong loadings in coastal and metropolitan areas. This reinforces the importance of population dynamics in economic growth, but the PLSR analysis allowed for a more nuanced understanding of its relative importance compared to other factors.
Educational attainment showed a consistently strong positive relationship with GDP, that often matched or surpassed population change, highlighting its strong correlation with economic outcomes. The same was observed for other socioeconomic factors that showcased strong relative importance, including median household income, median rent, and median home value.
The relationship between racial and ethnic composition and GDP showed more variation across RUCs compared to the population change analysis, particularly for Hispanic and Black populations. This suggests that associations with GDP are not equitably distributed and may vary depending on access to socioeconomic opportunities at the community level.
The Northeast non-metro RUC stood out in this analysis, showing divergent trends for many variables. Factors typically associated with growth showed weaker or negative relationships with GDP in this region.
Figure 7 displays the comparative results of (A) the linear relationship between population change and GDP change, and (B) the linear relationship between the PLSR composite score, incorporating population and sociodemographic changes, and GDP change.
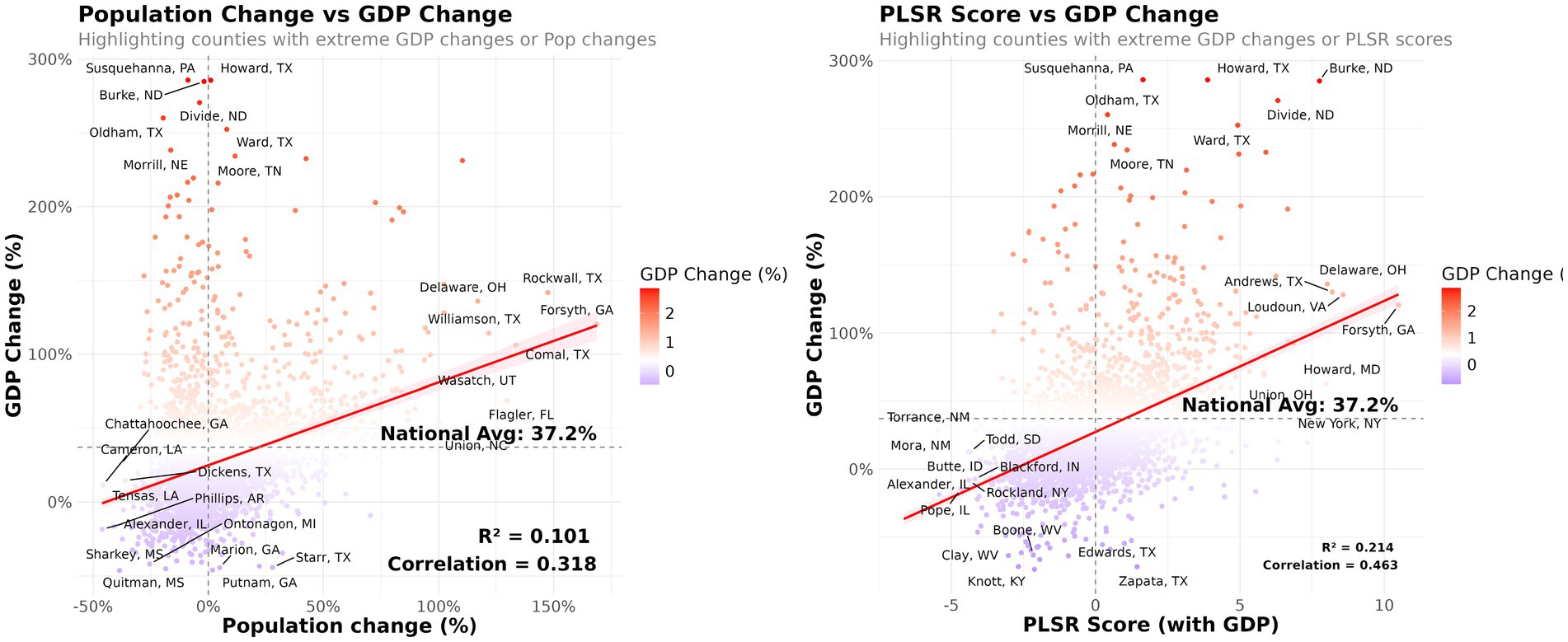
Figure 7. Comparative analysis of GDP change relationships. Left panel shows the linear regression of population change (2000–2020) on GDP change (2001–2003 to 2017–2019 averages). Right panel shows the linear regression of the PLSR composite score (incorporating population and sociodemographic changes from 2000 to 2020) on GDP change (2001–2003 to 2017–2019 averages). Both panels display county-level data points, regression lines, and 95% confidence intervals. R2 values (percentage of variance explained) and Pearson’s correlation coefficients (ρ) are provided in the bottom right corner of each plot. The PLSR score, capturing the collective impact of population and sociodemographic changes, demonstrates greater explanatory power for GDP change compared to population change alone.
When assessing the relationship between population change and GDP change across U.S. counties, a moderate positive correlation (rho = 0.318) is observed. Population change explained approximately 10.1% of the variation in GDP change. When incorporating a broader range of sociodemographic factors via the composite score, the explanatory power of the model increased. The correlation between the composite score and GDP change strengthened to 0.463, with the R-squared value of 0.214. This indicates that the comprehensive sociodemographic model explains 21.4% of the variation in GDP change across counties, more than doubling the explanatory power of population change alone.
4 Discussion
Our study provides a comprehensive analysis of the relationships between population change, sociodemographic shifts, and economic outcomes across diverse geographic contexts in the United States over the past two decades. These findings provide valuable insights that build upon previously identified relationships between sociodemographic characteristics, economic outcomes, and population change, adding to literature on future migration and its potential social and economic impacts. Furthermore, by examining these relationships at the local level across the U.S. and considering how they vary by geography, we offer a more nuanced understanding of expected trends due to climate change. Information from this study will bolster future research, and can be used as a foundation to evaluate sociodemographic and economic changes as a result of observed population responses to climate risk (i.e., Hauer et al., 2017; Shu et al., 2023), and against a larger set of risks (e.g., flood, fire, heat) across various climate scenarios. While our current study contributes a small piece to that larger phenomena, this research provides a holistic understanding of extensive population, sociodemographic, and economic changes that have not been comprehensively examined before.
In regard to the most important results of the research, we found that areas experiencing population growth generally saw favorable increases in socioeconomic indicators such as education levels and income, while areas with population decline tended to see increases in poverty rates and aging populations. Importantly, we observed a positive relationship between population growth and GDP, with areas experiencing population decline or slow growth showing below-average GDP growth. When considering shifts in sociodemographics alongside population change, the relationship with GDP strengthened considerably, suggesting that the composition of a community is important to economic outcomes. These relationships varied by geographic context, highlighting the need for nuanced, location-specific approaches when considering the potential impacts of population change on community profiles and local economies.
The relationship between population change and sociodemographic shifts revealed several notable patterns. Consistent with previous research (e.g., Hauer et al., 2024), we found that areas experiencing population decline tended to see increases in median age, while growing areas saw decreases. This trend was particularly pronounced in coastal areas, suggesting potential implications for climate vulnerability and adaptation capacity. Median household income showed a strong positive relationship with population growth across most geographic categories, aligning with established economic theories of agglomeration effects and human capital concentration (Castells-Quintana and Royuela, 2014; Abel and Deitz, 2012). Past research has also found that low-income individuals and families may be more likely to migrate in search of better economic opportunities or more affordable housing, leading to a concentration of poverty in certain areas (Foulkes and Schafft, 2010; Lichter et al., 2012; Buchholz, 2022). In contrast, high-income individuals and households tend to have greater mobility and more options when it comes to migration decisions, often moving for job opportunities, lifestyle preferences, or amenities, which can lead to a concentration of wealth and human capital in metropolitan regions with strong economies and amenities (Shumway and Otterstrom, 2015; Chen and Pope, 2020).
Our composite score analysis revealed that socioeconomic factors such as median household income, rent, home value, and educational attainment were consistently the strongest predictors of population change. This clustering of influential factors underscores the interconnected nature of socioeconomic characteristics and population dynamics, historically evident in studies over previous decades (Wheeler, 2005; Simon and Nardinelli, 2002). Interestingly, we observed generally positive trends in female population share with overall population growth, particularly in metro areas, which may reflect changing gender dynamics in migration patterns, education, and labor force participation (Falzone, 2017).
Trends in racial and ethnic composition varied by region, revealing complex patterns. While diversity generally increased in areas with population growth, we also observed negative relationships between Black and Hispanic population shares and overall population change in some urban and coastal areas. This suggests potential socioeconomic disparities and environmental justice implications, where disadvantaged subpopulations may be left behind in areas experiencing decline or displacement, including due to impacts from natural disasters (Fothergill et al., 1999; Bullard and Wright, 2009; Raker, 2020). In particular, areas with high climate exposure are found to have higher shares of these racial and ethnic groups (Hunter et al., 2003; Martinich et al., 2013), potentially creating a compounding effect where vulnerable populations face both increased climate risk and limited mobility.
Regarding economic outcomes, our analysis confirmed the expected positive relationship between population change and GDP growth. However, the inclusion of sociodemographic factors in our model doubled the explanatory power in GDP change compared to population change alone. This underscores the importance of considering community composition when assessing economic trajectories. Positive changes in socioeconomic indicators such as income, rent, and education levels were generally associated with GDP growth, as expected. This generally aligns with previous research on the role of human capital in regional economic development and the importance of educational attainment in driving productivity and innovation (Abel and Deitz, 2012). However, we also observed some unexpected trends, such as the positive relationship between labor occupation share and GDP growth in Northeast non-metropolitan areas, suggesting region-specific economic drivers. This could be explained by the presence of niche manufacturing industries or resource-based economies in these areas, as noted by Glasmeier and Salant (2006) in their study of rural economies in the Northeast and Lobao et al. (2016) in their study of rural industrial diversity.
This study has several strengths, including its use of local data at the tract level, evaluation of a multitude of sociodemographic variables, and application of composite scores to collectively assess relationships. The enhanced explanatory power of our PLSR model highlights the complex interplay between various sociodemographic factors and economic growth. While population change was a significant predictor of GDP, the inclusion of additional variables provided a better understanding of the drivers of economic variation across diverse U.S. counties. This multifaceted approach, capturing a broader spectrum of influences on local economic trajectories, reflects the intricate relationship between demographic characteristics and economic outcomes. Further, our novel RUC framework allows for a more nuanced understanding of geographic variation in these relationships. However, limitations include potential errors in Census survey data, possible COVID-19 influences on 2020 sociodemographic and population data, and the assumption that historical relationships will hold for future predictions of sociodemographic and economic change.
5 Conclusion
In conclusion, our research provides a comprehensive understanding of how population change is associated with sociodemographic shifts and economic outcomes across diverse geographic contexts in the United States. By describing these complex relationships, we offer valuable insights about anticipated changes to the characteristics and economic health of communities resulting from population change, particularly in the context of climate-induced migration. Our findings demonstrate that population shifts can trigger cascading effects on community composition and economic trajectories, with important implications for social equity and economic resilience. These results can inform policymakers, planners, and researchers seeking to anticipate and address the multifaceted impacts of population shifts on community profiles and local economies. Understanding these relationships is especially crucial as communities face mounting climate risks that may accelerate population movements. As climate change continues to reshape human geography, this nuanced understanding of population dynamics and their socioeconomic implications will be crucial for developing effective adaptation strategies that promote both community resilience and equitable outcomes across diverse geographic contexts.
Data availability statement
Publicly available datasets were analyzed in this study. This data can be found here: https://data.census.gov/. The BEA data used can be found here: https://www.bea.gov/data/economic-accounts/regional.
Author contributions
JB: Conceptualization, Data curation, Formal analysis, Investigation, Methodology, Validation, Visualization, Writing – original draft, Writing – review & editing. DM: Conceptualization, Data curation, Formal analysis, Investigation, Methodology, Visualization, Writing – original draft, Writing – review & editing. JP: Conceptualization, Investigation, Project administration, Supervision, Writing – original draft, Writing – review & editing. ZH: Conceptualization, Methodology, Writing – original draft, Writing – review & editing.
Funding
The author(s) declare that no financial support was received for the research, authorship, and/or publication of this article.
Conflict of interest
The authors declare that the research was conducted in the absence of any commercial or financial relationships that could be construed as a potential conflict of interest.
Publisher’s note
All claims expressed in this article are solely those of the authors and do not necessarily represent those of their affiliated organizations, or those of the publisher, the editors and the reviewers. Any product that may be evaluated in this article, or claim that may be made by its manufacturer, is not guaranteed or endorsed by the publisher.
Supplementary material
The Supplementary material for this article can be found online at: https://www.frontiersin.org/articles/10.3389/fhumd.2024.1465218/full#supplementary-material
References
Abel, J. R., and Deitz, R. (2012). Do colleges and universities increase their region’s human capital? J. Econ. Geogr. 12, 667–691. doi: 10.1093/jeg/lbr020
Adelino, M., Ma, S., and Robinson, D. (2017). Firm age, investment opportunities, and job creation. J. Financ. 72, 999–1038. doi: 10.1111/jofi.12495
Aguiar, M., and Hurst, E. (2013). Deconstructing life cycle expenditure. J. Polit. Econ. 121, 437–492. doi: 10.1086/670740
Ambinakudige, S., and Parisi, D. (2017). A spatiotemporal analysis of Inter-County migration patterns in the United States. Appl. Spat. Anal. Policy 10, 121–137. doi: 10.1007/s12061-015-9171-1
Armal, S., Porter, J., Lingle, B., Chu, Z., Marston, M., and Wing, O. (2020). Assessing property level economic impacts of climate in the US, new insights and evidence from a comprehensive flood risk assessment tool. Climate, 8:116. doi: 10.3390/cli8100116
Bernard, A., Bell, M., and Charles-Edwards, E. (2014). Life-course transitions and the age profile of internal migration. Popul. Dev. Rev. 40, 213–239. doi: 10.1111/j.1728-4457.2014.00671.x
Best, K., He, Q., Reilly, A. C., Niemeier, D. A., Anderson, M., and Logan, T. (2023). Demographics and risk of isolation due to sea level rise in the United States. Nat. Commun. 14:7904. doi: 10.1038/s41467-023-43835-6
Black, R., Bennett, S. R. G., Thomas, S. M., and Beddington, J. R. (2011). Climate change: migration as adaptation. Nature 478, 447–449. doi: 10.1038/478477a
Buchholz, M. (2022). Priced out? Household migration out of “superstar” US city-regions. Environ. Plan. A 54, 1623–1640. doi: 10.1177/0308518X221118912
Buettner, T., and Holm-Hadulla, F. (2013). City size and the demand for local public goods. Reg. Sci. Urban Econ. 43, 16–21. doi: 10.1016/j.regsciurbeco.2012.10.003
Bullard, R. D., and Wright, B. (2009). “Race, place, and the environment in post-Katrina new Orleans” in Race, place, and environmental justice after hurricane Katrina (Routledge).
Castells-Quintana, D., and Royuela, V. (2014). Agglomeration, inequality and economic growth. Ann. Reg. Sci. 52, 343–366. doi: 10.1007/s00168-014-0589-1
Chen, M. K., and Pope, D. (2020). Geographic mobility in America: Evidence from cell phone data. w27072. Cambridge, MA: National Bureau of Economic Research, w27072.
Chen, Y., and Rosenthal, S. S. (2008). Local amenities and life-cycle migration: do people move for jobs or fun? J. Urban Econ. 64, 519–537. doi: 10.1016/j.jue.2008.05.005
Diamond, R. (2016). The determinants and welfare implications of US Workers’ diverging location choices by skill: 1980-2000. Am. Econ. Rev. 106, 479–524. doi: 10.1257/aer.20131706
Duranton, G., and Puga, D. (2014). “Chapter 5 - the growth of cities” in Handbook of economic growth. eds. P. Aghion and S. N. Durlauf (Elsevier (Handbook of Economic Growth)), 781–853.
Falzone, J. S. (2017). Labor force participation and educational attainment in the United States. Int. Adv. Econ. Res. 23, 321–332. doi: 10.1007/s11294-017-9646-8
Fan, Q., Fisher-Vanden, K., and Klaiber, H. A. (2018). Climate change, migration, and regional economic impacts in the United States. J. Assoc. Environ. Resour. Econ. 5, 643–671. doi: 10.1086/697168
First Street. (2023). The Precipitation Problem. Available at: https://firststreet.org/research-library/the-precipitation-problem (Accessed November 25, 2024).
Fothergill, A., Maestas, E. G. M., and Darlington, J. D. (1999). Race, ethnicity and disasters in the United States: a review of the literature. Disasters 23, 156–173. doi: 10.1111/1467-7717.00111
Foulkes, M., and Schafft, K. A. (2010). The impact of migration on poverty concentrations in the United States, 1995–2000. Rural. Sociol. 75, 90–110. doi: 10.1111/j.1549-0831.2009.00002.x
Fussell, E., Sastry, N., and VanLandingham, M. (2010). Race, socioeconomic status, and return migration to New Orleans after hurricane Katrina. Popul. Environ. 31, 20–42. doi: 10.1007/s11111-009-0092-2
Glaeser, E. L., and Gottlieb, J. D. (2009). The wealth of cities: agglomeration economies and spatial equilibrium in the United States. J. Econ. Lit. 47, 983–1028. doi: 10.1257/jel.47.4.983
Glasmeier, A., and Salant, P. (2006). ‘Low-skill workers in rural America face permanent job loss’, Carsey School of Public Policy. 6. doi: 10.34051/p/2020.7
GOETZ, S. J., HAN, Y., FINDEIS, J. L., and BRASIER, K. J. (2010). U.S. commuting networks and economic growth: measurement and implications for spatial policy. Growth Chang. 41, 276–302. doi: 10.1111/j.1468-2257.2010.00527.x
Gosnell, H., and Abrams, J. (2011). Amenity migration: diverse conceptualizations of drivers, socioeconomic dimensions, and emerging challenges. GeoJournal 76, 303–322. doi: 10.1007/s10708-009-9295-4
Hauer, M. E., White, M. J., Little, J. S., and Sutton, J. (2017). Migration induced by sea-level rise could reshape the US population landscape. Nat. Clim. Chang. 7, 321–325. doi: 10.1038/nclimate3271
Hauer, M. E., Evans, J. M., and Mishra, D. R. (2016). Millions projected to be at risk from sea-level rise in the continental United States. Nat. Clim. Chang. 6, 691–695. doi: 10.1038/nclimate2961
Hauer, M. E., Fussell, E., Mueller, V., Burkett, M., Call, M., Abel, K., et al. (2020). Sea-level rise and human migration. Nature Rev. Earth Environ. 1, 28–39. doi: 10.1038/s43017-019-0002-9
Hauer, M. E., Hardy, D., Kulp, S. A., Mueller, V., Wrathall, D. J., and Clark, P. U. (2021). Assessing population exposure to coastal flooding due to sea level rise. Nat. Commun. 12:6900. doi: 10.1038/s41467-021-27260-1
Hauer, M. E., Jacobs, S. A., and Kulp, S. A. (2024). Climate migration amplifies demographic change and population aging. Proc. Natl. Acad. Sci. USA 121:e2206192119. doi: 10.1073/pnas.2206192119
Howard, G. (2020). The migration accelerator: labor mobility, housing, and demand. Am. Econ. J. Macroecon. 12, 147–179. doi: 10.1257/mac.20180363
Hunter, L. M., White, M. J., Little, J. S., and Sutton, J. (2003). Environmental hazards, migration, and race. Popul. Environ. 25, 23–39. doi: 10.1023/A:1025595505532
Johnson, K.M., and Beale, C.L. (2002) ‘Nonmetro recreation counties their identification and rapid growth’, United States Department of Agriculture, Economic Research Service, 17(4). Available at: https://ideas.repec.org//a/ags/uersra/289572.html (Accessed: 5 November 2024).
Johnson, K., and Cromartie, J. (2006). The rural rebound and its aftermath: changing demographic dynamics and regional contrasts. The Springer Series on Demographic Methods and Population Analysis, 84, 25–49.
Johnson, K. M., and Lichter, D. T. (2019). Rural depopulation: growth and decline processes over the past century. Rural. Sociol. 84, 3–27. doi: 10.1111/ruso.12266
Johnson, K. M., Voss, P. R., Hammer, R. B., Fuguitt, G. V., and Mcniven, S. (2005). Temporal and spatial variation in age-specific net migration in the United States. Demography 42, 791–812. doi: 10.1353/dem.2005.0033
Johnson, K. M., and Winkler, R. L. (2015). Migration signatures across the decades: net migration by age in U.S. counties, 1950-2010. Demogr. Res. 32, 1065–1080.
Johnson, K., Winkler, R., and Rogers, L. (2013). Age and lifecycle patterns driving U.S. migration shifts. Carsey School Public Policy. 192, doi: 10.34051/p/2020.192
Kaczan, D. J., and Orgill-Meyer, J. (2020). The impact of climate change on migration: a synthesis of recent empirical insights. Clim. Chang. 158, 281–300. doi: 10.1007/s10584-019-02560-0
Kearns, E. J., Saah, D., Levine, C. R., Lautenberger, C., Doherty, O. M., Porter, J. R., et al. (2022). The construction of probabilistic wildfire risk estimates for individual real estate parcels for the contiguous United States. Fire 5:117. doi: 10.3390/fire5040117
Keenan, J. M., Hill, T., and Gumber, A. (2018). Climate gentrification: from theory to empiricism in Miami-Dade County, Florida. Environ. Res. Lett. 13:054001. doi: 10.1088/1748-9326/aabb32
Kemeny, T., and Storper, M. (2012). The sources of urban development: wages, housing, and amenity gaps across American cities. J. Reg. Sci. 52, 85–108. doi: 10.1111/j.1467-9787.2011.00754.x
Kennan, J., and Walker, J. R. (2011). The effect of expected income on individual migration decisions. Econometrica 79, 211–251. doi: 10.3982/ECTA4657
Keys, B., and Mulder, P. (2024). Property insurance and disaster risk: New evidence from mortgage escrow data. w32579. Cambridge, MA: National Bureau of Economic Research, w32579.
Liang, J., Wang, H., and Lazear, E. P. (2018). Demographics and entrepreneurship. J. Polit. Econ. 126, S140–S196. doi: 10.1086/698750
Lichter, D. T., Parisi, D., and Taquino, M. C. (2012). The geography of exclusion: race, segregation, and concentrated poverty. Soc. Probl. 59, 364–388. doi: 10.1525/sp.2012.59.3.364
Lobao, L., Zhou, M., Partridge, M., and Betz, M. (2016). Poverty, place, and coal employment across Appalachia and the United States in a new economic era. Rural. Sociol. 81, 343–386. doi: 10.1111/ruso.12098
Martinich, J., Neumann, J., Ludwig, L., and Jantarasami, L. (2013). Risks of sea level rise to disadvantaged communities in the United States. Mitig. Adapt. Strateg. Glob. Chang. 18, 169–185. doi: 10.1007/s11027-011-9356-0
McAlpine, S. A., and Porter, J. R. (2018). Estimating recent local impacts of sea-level rise on current real-estate losses: a housing market case study in Miami-Dade, Florida. Popul. Res. Policy Rev. 37, 871–895. doi: 10.1007/s11113-018-9473-5
McGranahan, D. (1999) Natural amenities drive rural population change. Agricultural economic report no. (AER-781). USDA ERS, p. 27. Available at: http://www.ers.usda.gov/publications/pub-details/?pubid=41048 (Accessed 14 July 2024).
Molloy, R., Smith, C. L., and Wozniak, A. (2011). Internal migration in the United States. J. Econ. Perspect. 25, 173–196. doi: 10.1257/jep.25.3.173
Plane, D. A., and Jurjevich, J. R. (2009). Ties that no longer bind? The patterns and repercussions of age-articulated migration. Prof. Geogr. 61, 4–20. doi: 10.1080/00330120802577558
Raker, E. J. (2020). Natural hazards, disasters, and demographic change: the case of severe tornadoes in the United States, 1980–2010. Demography 57, 653–674. doi: 10.1007/s13524-020-00862-y
Rappaport, J. (2007). Moving to nice weather. Reg. Sci. Urban Econ. 37, 375–398. doi: 10.1016/j.regsciurbeco.2006.11.004
Rickman, D. S., and Wang, H. (2017). US regional population growth 2000–2010: natural amenities or urban agglomeration? Pap. Reg. Sci. 96, S69–S91. doi: 10.1111/pirs.12177
Robinson, C., Dilkina, B., and Moreno-Cruz, J. (2020). Modeling migration patterns in the USA under sea level rise. PLoS One 15:e0227436. doi: 10.1371/journal.pone.0227436
Shu, E. G., Porter, J. R., Hauer, M. E., Sandoval Olascoaga, S., Gourevitch, J., Wilson, B., et al. (2023). Integrating climate change induced flood risk into future population projections. Nat. Commun. 14:7870. doi: 10.1038/s41467-023-43493-8
Shumway, J. M., and Otterstrom, S. M. (2015). Income migration and income convergence across U.S. states, 1995–2010. Growth Chang. 46, 593–610. doi: 10.1111/grow.12104
Simon, C. J., and Nardinelli, C. (2002). Human capital and the rise of American cities, 1900–1990. Reg. Sci. Urban Econ. 32, 59–96. doi: 10.1016/S0166-0462(00)00069-7
USDA (2024). ‘Rural-Urban Continuum Codes’. Available at: https://www.ers.usda.gov/data-products/rural-urban-continuum-codes/ (Accessed: 14 July 2024).
Wheeler, C. H. (2005). Human capital growth in a cross section of us metropolitan areas. FRB of St. Louis Working Paper No. 2005-065A.
WHISLER, R. L., WALDORF, B. S., MULLIGAN, G. F., and PLANE, D. A. (2008). Quality of life and the migration of the college-educated: a life-course approach. Growth Chang. 39, 58–94. doi: 10.1111/j.1468-2257.2007.00405.x
Wilson, B., Porter, J. R., Kearns, E. J., Hoffman, J. S., Shu, E., Lai, K., et al. (2022). High-resolution estimation of monthly air temperature from joint modeling of in situ measurements and gridded temperature data. Climate 10:47. doi: 10.3390/cli10030047
Winkler, R. L., and Johnson, K. M. (2015). Migration signatures across the decades: net migration by age in U.S. counties, 1950-2010. Demogr. Res. 32, 1065–1080. doi: 10.4054/DemRes.2015.32.38
Keywords: population dynamics, demographic composition, socioeconomic composition, climate migration, local GDP, economic growth, climate change
Citation: Buresch JM, Medgyesi D, Porter JR and Hirsch ZM (2024) Understanding how population change is associated with community sociodemographics and economic outcomes across the United States. Front. Hum. Dyn. 6:1465218. doi: 10.3389/fhumd.2024.1465218
Edited by:
Khandaker Jafor Ahmed, University of Michigan, United StatesReviewed by:
Shah Md Atiqul Haq, Shahjalal University of Science and Technology, BangladeshDylan Shane Connor, Arizona State University, United States
Copyright © 2024 Buresch, Medgyesi, Porter and Hirsch. This is an open-access article distributed under the terms of the Creative Commons Attribution License (CC BY). The use, distribution or reproduction in other forums is permitted, provided the original author(s) and the copyright owner(s) are credited and that the original publication in this journal is cited, in accordance with accepted academic practice. No use, distribution or reproduction is permitted which does not comply with these terms.
*Correspondence:Jeremy R. Porter, amVyZW15QGZpcnN0c3RyZWV0Lm9yZw==