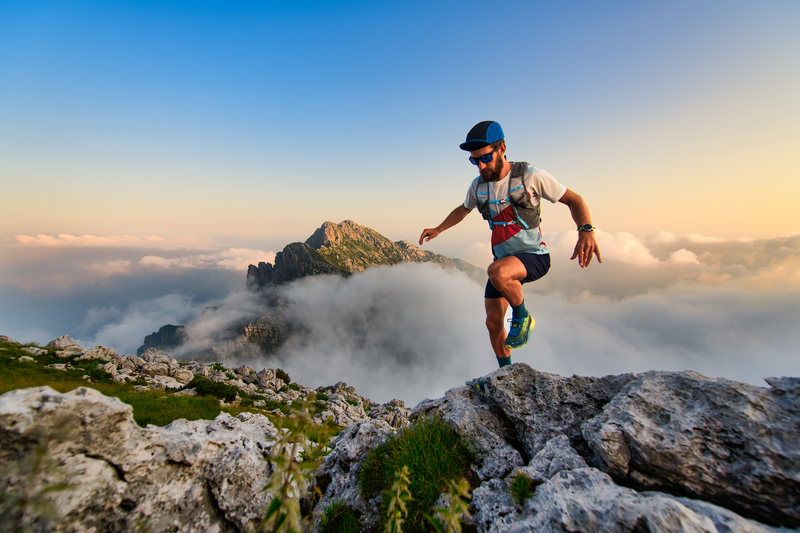
94% of researchers rate our articles as excellent or good
Learn more about the work of our research integrity team to safeguard the quality of each article we publish.
Find out more
EDITORIAL article
Front. Hum. Dyn. , 28 March 2023
Sec. Social Networks
Volume 5 - 2023 | https://doi.org/10.3389/fhumd.2023.1178455
This article is part of the Research Topic Hybrid Collective Intelligence View all 5 articles
Editorial on the Research Topic
Hybrid collective intelligence
This special issue tackles the topic of Hybrid Collective Intelligence—a system of collaboration and decision-making that combines the strengths of both human and artificial intelligence (AI). In such a system, the contributions and expertise of individuals are combined with the speed and accuracy of AI algorithms, resulting in a more robust and effective decision-making process.
Today, with the rapid rise of AI in general, and generative AI in particular, we can safely say that hybrid collective intelligence leverages the ability of AI to process large amounts of data and make predictions based on that data, while also considering the expertise and experience of human individuals who bring unique perspectives and context to the decision-making process.
However, there are also various challenges associated with hybrid collective intelligence, including those related to governance, trust, bias, accountability, as well as societal and economic issues. In the collection of articles presented in this Special Issue, the authors make their best efforts to address and analyze these challenges with scientific rigor.
For instance, to effectively implement hybrid collective intelligence, it is important to consider these challenges and implement systems and processes that ensure transparency and fairness in the decision-making process. Moreover, decentralization combined with application of artificial intelligence can have a significant impact on businesses and organizations. In a decentralized organization, the decision-making power and control is distributed among multiple actors, as opposed to being centralized in a single authority. AI, on the other hand, has the potential to automate and optimize various processes within organizations, leading to improved efficiency and productivity.
The combination of these two technologies can lead to a shift in the traditional centralized structures of organizations, as AI can facilitate decentralized decision-making and enhance the transparency and accountability of organizational processes. This already palpable shift is addressed by some of the papers provided in this Special Issue.
Hazy's paper (Hazy) refers to organizational leadership and collective agency in the era of emerging technologies. The article examines the relationship between individual-led leadership and collective intelligence and agency. Hazy draws on complex network theory and studies of social biology to show that collective agency forms around three key parameters: expectancy alignment, instrumentality within the group, and belief in the trustworthiness of others. When these metrics are stable, information is shared among individuals and can enhance leadership activities. The article is very actionable: it makes 15 testable assertions that suggest new technology could improve organizational effectiveness. Moreover, Hazy introduces a new model of emergent collective intelligent agency (CIA), which states that collective agency is enabled by collective intelligence and actualized through specific interactions called “leading events.” These interactions form a shared representation of stable properties that define the collective as an organization. The model is based on three numerical models that study phase transitions and decision making in complex networks and further the understanding of leadership as a necessary mechanism for human organizing.
The article by Lauterbach shows that the discussions around COVID-19 apps and models have highlighted the challenges of governance and ethics in AI and data science. The use of personal information to build data sets raises concerns about privacy and raises questions about the sustainability of current approaches to technology and data handling. COVID-19 has made it clear that digital policies are needed to ensure economies and societies can succeed in a digital world, but they need to balance privacy and innovation. Lauterbach's paper suggests that Germany and Europe can improve their digital policy by introducing Data IDs, supporting innovation in decentralized data technologies, and promoting education around data. It also discusses the benefits of data-tokens and the importance of working with small data sets while ensuring privacy and considering environmental costs. It calls for innovation to be an integral part of data science.
The article by Przegalinska and Wright devoted to the topic of Universal Basic Income (UBI) claims that AI will or should result in a universal basic income policy are not well-supported by evidence and therefore not worthy of serious consideration. Trends in individual/familial income adjustment to business, economic, and technological change suggest incremental changes, not a radical shift requiring new policy. If AI proves to be a more disruptive force, which—in terms of rapid acceleration—we are in fact observing today, UBI-like payments can be made quickly, as seen with recent fiscal stimulus.
Walter argues that the increasing use of AI systems in the digital world raises concerns about the trustworthiness of information available online. The three main challenges in this domain include algorithmic manipulation, deliberate disinformation campaigns, and the veracity of the medium. The question of how to ensure AI aligns with ethical norms and human values has become a key discussion point. This paper outlines two case reports and proposes solutions for addressing the problems associated with AI information processing. It builds upon previous publications that have dealt with issues of trust and AI information processing, and acknowledges the fast pace of digital and AI developments. The discussion aims to highlight the unresolved issues of information transparency in AI systems and to guide a societal discussion about the difficulties in AI alignment and safety engineering.
As we can see, there is no lack of challenges and open questions regarding hybrid collective intelligence, as well as its future development and impact. The use of AI in decentralized organizations can raise concerns about privacy and data security, as well as issues related to accountability and control. Additionally, there is the need for standardization and governance of AI systems, which can be more difficult in decentralized organizations of the future.
In summary, the link between decentralization and AI in organizations lies in the potential for AI to enhance the efficiency and decision-making capabilities of decentralized structures, while also raising important questions and challenges that this Special Issue aims at addressing.
The author confirms being the sole contributor of this work and has approved it for publication.
The author declares that the research was conducted in the absence of any commercial or financial relationships that could be construed as a potential conflict of interest.
All claims expressed in this article are solely those of the authors and do not necessarily represent those of their affiliated organizations, or those of the publisher, the editors and the reviewers. Any product that may be evaluated in this article, or claim that may be made by its manufacturer, is not guaranteed or endorsed by the publisher.
Keywords: artificial intelligence, hybrid intelligence, decentralization, collective intelligence (CI), emerging technologies
Citation: Przegalińska A (2023) Editorial: Hybrid collective intelligence. Front. Hum. Dyn. 5:1178455. doi: 10.3389/fhumd.2023.1178455
Received: 02 March 2023; Accepted: 20 March 2023;
Published: 28 March 2023.
Edited and reviewed by: Wojciech Marek Kulesza, University of Social Sciences and Humanities, Poland
Copyright © 2023 Przegalińska. This is an open-access article distributed under the terms of the Creative Commons Attribution License (CC BY). The use, distribution or reproduction in other forums is permitted, provided the original author(s) and the copyright owner(s) are credited and that the original publication in this journal is cited, in accordance with accepted academic practice. No use, distribution or reproduction is permitted which does not comply with these terms.
*Correspondence: Aleksandra Przegalińska, YXByemVnYWxpbnNrYUBrb3ptaW5za2kuZWR1LnBs
Disclaimer: All claims expressed in this article are solely those of the authors and do not necessarily represent those of their affiliated organizations, or those of the publisher, the editors and the reviewers. Any product that may be evaluated in this article or claim that may be made by its manufacturer is not guaranteed or endorsed by the publisher.
Research integrity at Frontiers
Learn more about the work of our research integrity team to safeguard the quality of each article we publish.