- VTT Technical Research Centre of Finland Ltd., Espoo, Finland
Recent advances in AI raise questions about its social impacts and implementation. In response, governments and public administrations seek to develop adequate governance frameworks to mitigate risks and maximize the potential of AI development and use. Such work largely deals with questions of how challenges and risks should be managed, which values and goals should be pursued, and through which institutional mechanisms and principles these goals could be achieved. In this paper, we conduct a systematic review of the existing literature on the development of AI governance for public administration. The article describes principles and means by which public administrations could guide and steer AI developers and users in adopting ethical and responsible practices. The reviewed literature indicates a need for public administrations to move away from top-down hierarchical governance principles and adopt forms of inclusive policy-making to ensure the actionability of ethical and responsibility principles in the successful governance of AI development and use. By combining the results, we propose a CIIA (Comprehensive, Inclusive, Institutionalized, and Actionable) framework that integrates the key aspects of the proposed development solutions into an ideal typical and comprehensive model for AI governance.
Introduction
Over the past few years, advances in machine learning, major increases in available data, databases and databanks, and the increasing power of processors have significantly boosted the potential for using artificial intelligence (AI). As a result, AI technologies are increasingly being applied across all sectors of society, and expectations for the continued development and deployment of AI are high. While expectations of significant beneficial effects for society and individuals drive technology and application development, AI may cause risks and problems (Floridi et al., 2018; Tsamados et al., 2021). For example, using AI for efficiency, optimization, and profit maximization might lead to increased inequality and power asymmetries, loss of human autonomy, loss of trust in AI systems, and environmental degradation (Zuboff, 2019; Crawford, 2021; Stahl, 2021). To support the realization of the social and business potential of AI, governments, various organizations, and researchers are developing new ways to govern and guide the development and use of AI. Many contributors think that the current forms of governance should be further developed to better steer the development and use of AI in a socially and environmentally sustainable way, opening up the question of how public governing institutions can respond effectively to emerging challenges to ensure that AI benefits individuals, businesses, and society. For example, researchers have suggested that a new regulatory agency should be developed to support the operationalization of good governance and ethical principles, the assessment of ethical issues and social impacts should be an indispensable part of AI development, and governance should utilize more people-centered and inclusive policy-making (e.g., Floridi et al., 2018; de Almeida et al., 2021; Ireni-Saban and Sherman, 2021; Stahl, 2021; Taeihagh, 2021; Ulnicane et al., 2021).
Current AI governance practices include regulations, industry standards, ethical codes and guidelines, policy strategies, and procedures for coordination and collaboration between stakeholders. While regulation and technical standards form some of the tools for governing AI, they are not by themselves sufficient to steer AI in a socially purposeful and beneficial direction, which is why ethical consideration is needed (Floridi, 2018). To address this challenge, ethical governance of AI aims to minimize the risks of AI and support the use of technology for the common good, as well as social and economic sustainability (Taddeo and Floridi, 2018; Winfield and Jirotka, 2018; Ireni-Saban and Sherman, 2021; Stahl, 2021). However, as a basis for AI governance, ethical principles face problems of weak operationalization and implementation through governmental policies and organizational practices (Mittelstadt, 2019; Hagendorff, 2020; Larsson, 2020; Morley et al., 2020; Stix, 2021). The issue seems not to be the lack of proposed tools and principles as such, but rather their formulation into an applicable approach in different contexts and at all levels of society (Yeung et al., 2019; Stahl et al., 2021; Stix, 2021).
The quickly evolving research in this field addresses topics ranging from governing and using AI for the common good (Floridi et al., 2020; Tomašev et al., 2020; Stahl, 2021; Wamba et al., 2021) and sustainable social and environmental development (Truby, 2020; Vinuesa et al., 2020) to ideas of universal, human rights–based value frameworks for the governance of AI (Donahoe and Metzger, 2019; Yeung et al., 2019; Smuha, 2020). Suggestions addressing ethical issues of AI governance can be perceived at three levels (Stahl, 2021): policy and legislation (e.g., Jobin et al., 2019; European Commission, 2022), organizations (Shneiderman, 2020; Tsamados et al., 2021), and guidance mechanisms for individuals (e.g., Morley et al., 2020). The governance of AI has also been investigated through the lens of AI use in the government (Zuiderwijk et al., 2021) and public sectors (Ireni-Saban and Sherman, 2021).
The policy-level suggestions for developing both policy implementation procedures and the roles and tasks of public administrations in AI policy are the major interest of this article.
These AI governance studies are often influenced by insights of emerging technology governance (e.g., Taeihagh, 2021; Taeihagh et al., 2021; Ulnicane et al., 2021), highlighting the fact that practices of good public governance should also include taking a facilitating role in the coordination and cooperation between state and non-state actors (Borrás and Edler, 2020). Coordination mechanisms and soft-law approaches are potential ways to improve collective decision-making and increase the flexibility and adaptability of the ways AI challenges are addressed in public administration. Such approaches call for a move away from top-down and formal regulation toward procedural improvements in decision-making and governance through various coordinated, anticipatory, and participatory processes (Kuhlmann et al., 2019; Taeihagh et al., 2021). These calls for more flexible (i.e., tentative, adaptive, anticipatory) technology governance modalities and procedures seem to intertwine with each other (Winfield and Jirotka, 2018; Lehoux et al., 2020; Ireni-Saban and Sherman, 2021). Whereas flexible forms of governance provide procedural answers to emerging technology challenges, ethical governance seeks to formulate and apply ethical guidelines to such challenges. Both approaches seek, in turn, to support the development of public governance by referring to principles of responsible research and innovation (RRI) (Winfield and Jirotka, 2018; Lehoux et al., 2020; Ireni-Saban and Sherman, 2021). According to the RRI approach, stakeholder involvement, dialogue, and consideration of different perspectives are fundamental in ensuring that various societal values and interests are accounted for in decision-making.
Thus, the literature on the governance of AI offers a range of suggestions to help public administrations foster ethical AI, but the suggestions remain scattered, and more work is needed in making it applicable to public administration. Therefore, this study aimed to compile and integrate these suggestions into a comprehensive and applicable framework. In this article, we ask what the means are by which public administrations could foster ethical and responsible development, implementation, and use of AI in society? To answer this question, we conducted a systematic literature review on development proposals for the public governance of AI. We focus on the means by which governments could ensure that the organizations responsible for the development, implementation, and use of AI follow ethical requirements. These development suggestions are not restricted to mechanisms of implementing governance; they also apply to the renewal of governance modalities themselves—i.e., the way governance should be practiced by public governing institutions.
While scholars have put forward a number of various suggestions to advance the governance of AI, few studies have attempted to review, compile, and integrate these various ideas into a coherent framework (see Wirtz et al., 2020; de Almeida et al., 2021; Stahl, 2021). To the best of our knowledge, as of writing this article, there is only one other systematic review on the subject. This study by de Almeida et al. (2021) summarizes 21 research and policy papers and presents a rather formal and “top down” AI regulatory framework as a synthesis. The resulting framework is based on a traditional model of public administration and roles of public authorities (i.e., legislative, executive, juridical) and does not discuss needs to reform public administration beyond that paradigm. Furthermore, while their framework involves various forms of cooperation between public operators and stakeholders, the model pays little attention to “bottom up” stakeholders or citizen engagement beyond that of industry members.
In contrast, the results of our review call for paying specific attention to the principles and forms of flexible public governance and RRI, including strong claims for broad stakeholder and citizen collaboration and engagement in articulating common goals, ethical principles, and means for governing AI. A number of studies hold that integrating these perspectives is of special importance in implementation of ethical reasoning in public policy and organizational practices (Winfield and Jirotka, 2018; Kuhlmann et al., 2019; Lehoux et al., 2020; Ireni-Saban and Sherman, 2021; Taeihagh, 2021; Ulnicane et al., 2021). Unlike the study by de Almeida et al. (2021), our study is based on a detailed thematic analysis that enables the validation of the resulting governance framework elements by explicitly linking them to the results of this review. Our review updates the previous contributions by integrating recent works published from the beginning of 2020 to April 2021.
The paper is structured as follows. The Introduction section describes and defines the governance of AI and provides an overview of the challenges of ethical AI governance. The aim in this section is not to conduct a “deep dive” into general governance literature but to define the framework of the review. The Ethical AI governance by public administration section presents the methodology and phases of our literature review. In the Methods section, we analyze the solutions suggested for developing the governance of AI in public administration. A discussion and compilation of suggested solutions for an integrated governance framework follow in the concluding section.
Ethical AI Governance By Public Administration
Definitions of AI and its risks, potential, and objectives steer discussions on governance policy. The potential problems and ethical questions regarding AI are complex and affect society at large (e.g., Floridi et al., 2018; Zuboff, 2019; Coeckelbergh, 2020; Crawford, 2021; Tsamados et al., 2021). Proposed responses range from technical design solutions to organizational management and strategy and government policy to research on and responsiveness to various direct and indirect short- and long-term impacts, externalities, and future trajectories of AI (Stahl, 2021). The perspective in this paper is that AI should be interpreted not only as stand-alone software (or algorithms) but also as a general-purpose technology embedded in wider socio-technical systems. Harnessing the capability of AI in different sectors of a society transforms the way that society works, bringing about socio-technical change. Focusing on AI only as a technical and computational system, separate from its social context and history, disproportionately narrows the debate surrounding its ethical implications, societal preconditions, and potential for social change (Coeckelbergh, 2020; Crawford, 2021) in public governance.
As a concept, governance is highly multi-dimensional, and there are different definitions and approaches to it (Frederickson, 2007). Broadly speaking, for our purposes, governance refers to processes related to decision-making and its implementation. It refers to the organized interaction between different actors—i.e., formal and informal regulation or control that guides action or behavior toward set objectives (Asaduzzaman and Virtanen, 2016). Usually, public governance literature describes forms of governance in terms of paradigm shifts (e.g., Torfing et al., 2020) according to which governance in the public administration over the past decades has evolved to better respond to the increased complexity of society and the resulting “wicked problems”. This change can be described as a shift in governance from hierarchical, regulatory-centered governance toward networks and participation, as well as interactive and democratic governance (Lähteenmäki-Smith et al., 2021).
Technology governance generally refers to the application of norms, regulations, and coordination mechanisms in the innovation and use of technology. For instance, Floridi (2018, p. 3) defines digital governance as “the practice of establishing and implementing policies, procedures, and standards for the proper development, use and management of the infosphere,” which includes good coordination and is complemented by the normative approaches of digital ethics and regulation. There is, however, no clear definition of the governance of AI, and the use of the term varies both in research papers (Zuiderwijk et al., 2021) and in policy documents (Ulnicane et al., 2021, p. 78). Typically, the term refers to the harnessing of the societal potential of AI while concurrently minimizing risks through various coordination, regulatory, and other guiding mechanisms. Such mechanisms include ethical principles, industry standards, information and resource related steering, and oversight to ensure compliance and enforcement (Morley et al., 2020; Stahl, 2021). In addition, Gahnberg (2021) proposed a more specific and technologically oriented definition of AI governance. He proposes that governance should be based on key generalizable elements of AI agency, defined as performance measurements, operating environment, actuators (i.e., effect on environment), and sensors. This could help narrow down the complexity of AI phenomena, as different AI technological components might create unique challenges and thus may require unique governance mechanisms. Accordingly, governance can be defined as “intersubjectively recognized rules that define, constrain, and shape expectations about the fundamental properties of an artificial agent” (Gahnberg, 2021, p. 201).
By governance of AI, this paper refers to complementary normative approaches of governance, regulation, and ethics. Here, public governance refers to coordination and policy implementation practices initiated by public authorities and policy-makers to form policy and steer private and public AI users and stakeholders; ethics refers to considerations to what ought to be done, including “over and above” requirements of the law (i.e., soft ethics), and regulation to the ways in which legal compliance is part of governance practices (Floridi, 2018). Governance of AI may include various frameworks, processes, and tools designed to maintain and promote cooperative possibilities to formulate shared values for AI, as well as to make and implement decisions regarding desirable directions in the development and use of AI (see Dafoe, 2018).
AI governance mechanisms can roughly be divided into two distinct categories: soft and hard law (Wallach and Marchant, 2018; Gutierrez et al., 2021). Hard law refers to formal laws and other sanctioned rules that are developed and implemented through the formal legislative processes. Soft laws, in turn, refer to various ethical principles, recommendations, and codes of conduct, as well as to various technical systems or infrastructure frameworks and related standards and protocols often used as forms of self-regulation within organizations and industries. In the governance of emerging technologies, technology developers and users are typically guided by soft-law instruments for self-regulation in the industrial sectors; legislation is retroactively developed when needed (Taeihagh et al., 2021). However, we consider soft governance to also include a broader array of means, such as coordination, as well as resource- and information-based steering to guide the development and uptake of emergent technologies. This division between soft and hard law and between self-regulation and formal hierarchical regulation reflects the continuous tension between the need for the public administration to regulate processes to avoid risks and advance societal objectives and the autonomy of the entities subject to regulation.
The governance of AI faces problems similar to that of any other emerging technology, which include information asymmetries, policy uncertainty, structural power dynamics, and policy errors (Taeihagh, 2021). These challenges are crystallized in the well-known Collingridge dilemma, in which regulators must choose a way to control technology development without sufficient information regarding the impacts, which cannot be predicted until the technology is in wide use. Thus, the regulator may face a situation in which they need to choose between proactive regulation, protecting citizens from risks, and a less regulated approach that could support innovation.
Currently, there are several examples of soft and hard governance approaches to AI. International standards developed by the International Organization for Standardization (ISO) and the Institute of Electrical and Electronics Engineers (IEEE) guide AI development at the technical level and among AI developers (Cihon, 2019). Both the European Union (European Commission, 2021a) and the Organization for Economic Co-operation and Development OECD (OECDAI Policy Observatory., 2021) have provided policy recommendations to support the safe and beneficial development of AI. Other principles and recommendations include the Asilomar AI Principles, Ethically Aligned Design by the IEEE, Charlevoix Common Vision for the Future of Artificial Intelligence, DeepMind Ethics & Society Principles, Google AI Principles, and the Information Technology Industry AI Policy Principles (Future of Life Institute, 2021). While there have been various proposals in the EU and US for AI-specific regulation [e.g., the current EU Artificial Intelligence Act proposal (European Commission, 2021b); see the High-Level Expert Group on AI (European Commission, 2022)], there are as yet no wide AI-specific regulations in effect. Thus, the existing legislation for AI consists mostly of various more general relevant regulations. These include human rights in the EU, the Charter of Fundamental Rights, the General Data Protection Regulation (GDPR) for privacy, the Product Liability Directive, anti-discrimination directives, and consumer protection. The European Commission (2021c) has recently agreed upon two legislative initiatives, the Digital Services Act (DSA) and the Digital Markets Act (DMA), to update EU-wide rules for digital services. The aim of these initiatives is to both protect the fundamental rights of digital service users and to foster “innovation, growth, and competitiveness”.
While regulation and standards can be efficient tools for governing AI, they are not necessarily sufficient to steer AI in a socially purposeful and beneficial direction, which is why ethical considerations and a capability to apply ethics in various contexts are needed (Floridi, 2018; Delacroix and Wagner, 2021). As a result, ethical principles, codes, and guidelines have emerged as a key soft governance solution to AI (Floridi et al., 2018; Jobin et al., 2019; Stix, 2021). Ethical AI governance is not meticulously defined in the AI governance literature. In general, it refers to a form of governance that minimizes the risks of AI, supports the use of technology for the common good, as well as social and economic sustainability (Ireni-Saban and Sherman, 2021; Stahl, 2021). Principles of good governance, such as effectiveness, transparency, participation, responsiveness, and legitimacy, are also closely related to ethical governance (Winfield and Jirotka, 2018, p. 2). In addition, ethical AI governance has been linked to RRI (Winfield and Jirotka, 2018; Ireni-Saban and Sherman, 2021), which aims to ensure that innovation activities are in the public interest by taking a broad range of stakeholders' perspectives into account at early stages in the innovation process. However, many authors criticize ethical principles and guidelines as insufficient for guaranteeing the ethical development of AI (Mittelstadt, 2019; Stix, 2021), in particular as such self-regulatory guidelines may be used among industry for ethics washing and as a means to avoid further regulation (Hagendorff, 2020; Delacroix and Wagner, 2021). The challenge for ethical AI governance is not necessarily a lack of shared values or ethical and responsible governance tools and principles (Morley et al., 2020). It is rather their compilation into a manageable and applicable approach that is needed for their operationalization in governance practices, policy-making, and AI application and service development (Donahoe and Metzger, 2019; Stahl et al., 2021).
Methods
To answer our research question, we conducted a systematic integrative literature review. An integrative literature review critically examines research data and integrates it to generate new models or frameworks for examining the selected perspective or literature (Torraco, 2016; Cronin and George, 2020). While a systematic literature review may have several different objectives and audiences (Okoli, 2015), this review aims to contribute to research that supports public governance for the ethical and responsible use of AI. In the following, we describe the data sources and collection methods, search terms, and criteria for selecting the literature.
We identified articles related to the governance of AI using the search terms described in Table 1. The search criterion was that the terms must be included in the abstract, title, or keywords of the article. To focus on international contributions that are not behind a language-barrier, we limited the search to English-language peer-reviewed scientific articles, conference papers, and book chapters published between 2010 and 2021. The timeframe was selected for two reasons: first, the general interest in AI research (Wamba et al., 2021) and especially in governance (indicated in our searches) has increased significantly only in the 2010s. Second, we wanted to focus on AI governance literature that accounts for the recent advances in AI ethics and general governance-related studies.
In addition, when looking at academic literature on AI governance development, we consciously excluded papers on the regulation and governance of robotics, digitalization, or similar technologies to keep the review focused. In addition, we excluded papers exclusively considering global coordination efforts for governance. There are a number of national and international AI policy papers (see, e.g., de Almeida et al., 2021) that we did not consider in this study, as the number of available papers was deemed too great for the scope of this review. Moreover, including policy papers would require a separate study with a different methodological approach taking into account their nature as texts carrying various political aspirations.
The literature search was conducted in three phases; the original search in 2019 was complemented with the most recent literature searches from the beginning of 2020 to April 2021:
• The first search was done in October 2019 for articles published between 2010 and 2019. The results included 1,821 articles before removing duplicates (Web of Science [773], Scopus [411], Proquest [157], ScienceDirect [46], Wiley [57], IEEE [159], EBSCO [174], Sage [43], Emerald [1]).
• The second search was done during December 2020 and included papers published during 2019–2020. This resulted in 947 articles before removing duplicates (Web of Science [220], Scopus [511], ScienceDirect [90], IEEE [126]). Wiley was removed from the search bases as it resulted in 3,261 articles, which was deemed too broad for the search.
• The third search was conducted on April 9, 2021, covering articles from 2020 to 2021. There were 624 articles before removing duplicates (Web of Science [23], Scopus [458], ScienceDirect [104], Wiley [6], IEEE [33].
The search results (n = 3,392) were transferred to the Mendeley reference manager program, which removed the remaining duplicates among the search phases. This resulted in 2,240 papers. A three-stage selection process was used to further limit the number of articles (Figure 1). The first phase involved assessing the relevance of the research papers to our topic. Due to the large number of papers, one researcher narrowed down the papers based on their title and abstract into those articles that were clearly not suitable for inclusion and those that were potentially suitable. The selection was validated by discussion with the research team of four people, after which the process was repeated to minimize bias. Most of the papers were clearly not relevant, indicating that the keyword search was too broad. For example, articles covered issues ranging from land development and water governance to animal tracking, block chain technology, and AI in learning and education. In addition, the sample included articles dealing with AI for enhancing information-processing capacities in public-sector decision-making, Internet of Things and smart cities, legal automation, and various AI applications in crime prevention, policing, security, and surveillance. During this phase, we found that papers related to AI governance could be roughly grouped into the following subject categories:
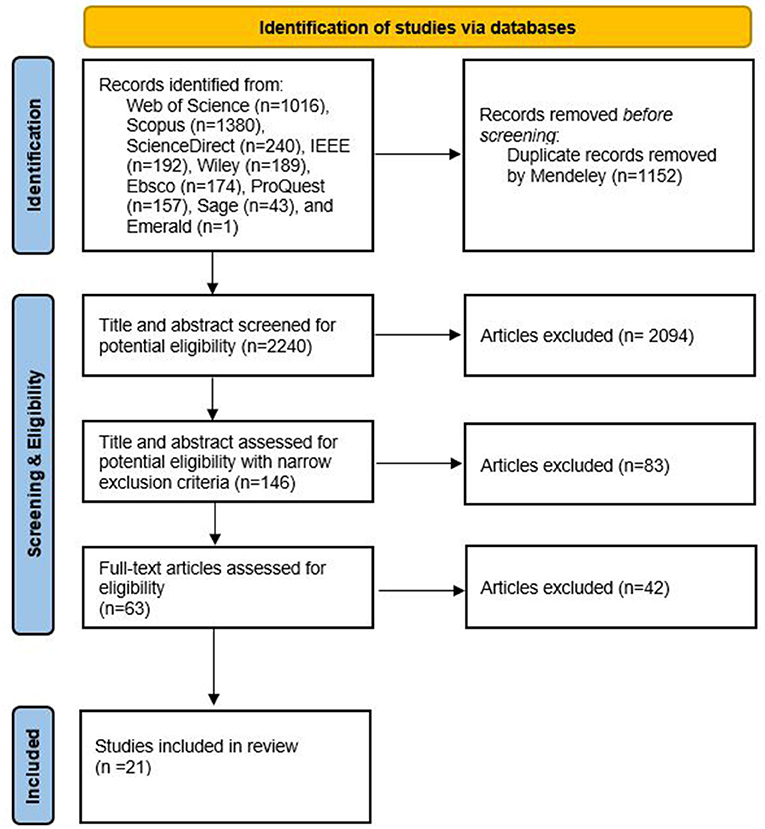
Figure 1. Process of selecting papers. Adapted Prisma flow diagram (Page et al., 2021).
a) Governance of AI, broadly referring to governmental actors using governance mechanisms to steer the development and implementation of AI, or the improvement of public AI governance modes and practices themselves;
b) Governance with AI, also known as algorithmic governance or governance by algorithms, referring to the use of AI as a tool for governance or regulation embedded in public-sector decision-making or organizational practices;
c) AI in public-sector services, i.e., e-governance, seeking to provide and to improve public sector services through IT;
d) Governance of AI in technical terms and/or within organizations, such as data governance, IT governance, and corporate governance.
To keep the study dedicated to our specific research question, we focused on papers examining the governance of AI (category a, above). To ensure that the potential overlapping of categories would not cause the exclusion of relevant articles, articles at this phase were screened using the following criteria:
• Article deals with AI;
• Article covers governance, ethics, or responsibility in the context of public administration or without specifying a context;
• Article has a clear societal perspective and is not only or excessively technical.
After two rounds of critical reading and the exclusion of clearly unsuitable articles, 146 articles remained. In the second phase, two researchers independently reviewed the abstracts according to the exclusion criteria listed below. Differences in opinion were discussed in separate meetings. This phase left 63 papers to be analyzed. Researchers aimed to select papers dealing with the public governance of AI, which included development suggestions. The inclusion criteria were as follows:
• AI should have a major role in the article. Studies in which AI plays only a minor role were removed.
• The article should mainly be about governance and ethics or responsibility in the context of public administration or without specifying a context. Studies in which governance, ethics, or responsibility plays only a minor role were removed, as were studies that are primarily concerned with organizational or data governance. Studies relating to algorithmic governance, e-governance, data governance, corporate governance, or public-sector organization-level governance were removed.
• The articles should suggest ways to improve the governance of AI. Studies in which AI governance was a major theme but not AI development, were removed.
In the third phase, two researchers independently studied the contents of the selected articles to assess their eligibility and quality. This resulted in 21 papers for analysis, as most of the papers did not meet the inclusion criteria after the researchers read the full text. The quality assessment was based on the assumption that the concepts of governance and AI are used loosely in the literature and may appear in the title or abstract, although their contribution to the content is minor or insignificant (Asaduzzaman and Virtanen, 2016; see Zuiderwijk et al., 2021). The quality of the studies was further assessed on the basis of the relevance of the concepts “governance” and “artificial intelligence” to the overall study and publication format. The selection was validated in a discussion with the whole research group.
The selected articles were then analyzed using qualitative thematic analysis, following the steps suggested by Nowell et al. (2017). The aim was to identify and analyze all the themes and dimensions that were defined as important for AI governance. The analysis proceeded in several iterative readings of the extracted data and full-length articles to form an understanding of the themes. In the first phases of analysis, the researchers familiarized themselves with the data and created tentative categories for further analysis. For each article, two researchers read the whole article while taking notes according to pre-defined categories. These categories included basic information, such as the full citation and abstract; the technology considered in the article; the objectives, challenges, and development suggestions; and other remarks. The data extracts were then compiled into tentative themes, which were tested by rereading articles iteratively in the next phases and validated in a discussion among the entire research group. During the rereading, special attention was given to the following: (1) problem definition, i.e., how AI governance is framed as a development topic—typically descriptions of the types of AI-related issues identified as requiring governance, regardless of existing practices and laws, thus setting the raisons d'être for the development proposal; (2) descriptions indicating how the principles and objectives of AI governance should be developed; and (3) descriptions of the means and tools needed to improve AI governance. In the last phases of the analysis, the themes were named and further integrated into a model consisting of the key takeaways of the governance proposals in the literature.
From Integrative Governance Frameworks To Tools Of Governance
On the basis of the analysis and interpretation of the data, we classified the development proposals into four themes (Table 2). The first of the identified themes presents governance frameworks as a means for providing a comprehensive approach to the development of governance of AI in the public administration. Contributions to this theme called for an integrated view on the governance and coordination of AI, which should cover highly context-specific issues and impacts of AI in the regulation processes, policy implementation, and coordination forms of collective decision-making (Gasser and Almeida, 2017; Wirtz et al., 2020; de Almeida et al., 2021).
The second theme deals with changing process requirements of the governance principles to increase the flexibility and effectiveness of policy development. Contributions to the theme considered the rationale for developing AI governance tools as a paradigmatic problem of public governance in the context of technology governance. The predominant view in these articles was that AI as a societal phenomenon is complex, wide-ranging, and rapidly evolving, as well as that traditional state-centric hierarchical governance should be supplemented with agile or adaptive governance processes, including forms of co-regulation and long-term policy strategizing. The contributions to this theme called for more participatory and bottom-up forms of decision-making (Cath et al., 2018; Wallach and Marchant, 2018; Winfield and Jirotka, 2018; Clarke, 2019; Sun and Medaglia, 2019; Buhmann and Fieseler, 2021; Liu and Maas, 2021; Ulnicane et al., 2021).
The third theme deals with the normative aspects and values of governance, as well as with the problem of implementing them in policy-making and organizational practices. The contributions in this theme proposed that ethical guidelines and principles are partially unsuccessful governance tools that do not sufficiently guide AI developers and users; they also sought to provide ways to address the related problems. Contributions suggested ways to enhance the practical implementation of ethical principles and human rights in policy-making (Floridi et al., 2018; Rahwan, 2018; Wallach and Marchant, 2018; Donahoe and Metzger, 2019; Sun and Medaglia, 2019; Truby, 2020; de Almeida et al., 2021; Delacroix and Wagner, 2021; Stix, 2021; Tsamados et al., 2021).
The fourth theme consists of concrete proposals for actions to be implemented by public administrations in the form of a regulatory agency or a set of public governing institutions to support the realization of normative principles and risk management. The suggestions deal with the steps that governing institutions should take to improve the oversight and enforcement of hard and soft governance principles, such as auditing, standards, forms of supervision, and facilitating collaboration (Floridi et al., 2018; Bannister and Connolly, 2020; Dignam, 2020; de Almeida et al., 2021). In the following sub-sections, we discuss each of these themes more in detail.
Comprehensive Frameworks on Governance
In the reviewed literature, governance frameworks function as heuristic descriptions of how to respond to the challenges and opportunities of AI at different levels of society. These frameworks link various perspectives, actors, processes, and mechanisms to form adequate solutions. According to Gasser and Almeida (2017), governance models or frameworks help researchers and public administrators to think about structural and institutional contexts in which AI can be conceptualized as governable. Rather than focusing on specific ethical challenges of AI (such as privacy, security, or power structures), comprehensive frameworks aim to capture the structural challenges of governance from the perspective of public decision-making. They provide “a conceptual lens for societies to think collectively and make informed policy decisions related to what, when, and how the uses and applications of AI should be regulated” (de Almeida et al., 2021, p. 505).
In the reviewed literature, governance is a mechanism to protect the public interest, minimize risks, and balance the interests of different stakeholders in a society (Baldwin et al., 2012) while concurrently ensuring human values and ethics as the foundation of governance (Gasser and Almeida, 2017; Cath et al., 2018; Donahoe and Metzger, 2019). Successful policy development should be based on the views of relevant stakeholders and an understanding of the societal benefits of AI (Cath et al., 2018; Wirtz et al., 2020). The AI governance frameworks should support the increased collective understanding of AI in different contexts and facilitate consensus-building between various stakeholders, as well as support cost–benefit analyses between values and interests (Gasser and Almeida, 2017; Rahwan, 2018; Wallach and Marchant, 2018; Wirtz et al., 2020). Such frameworks should also support the development of holistic governance, in which multiple levels of AI governance from legislation to ethics and technical solutions are seen as a systemic whole (Gasser and Almeida, 2017), and ensure that the developers of AI are subject to statutory oversight by an independent regulator (Rahwan, 2018; Wallach and Marchant, 2018; de Almeida et al., 2021).
While there is an abundance of overlapping elements in the frameworks, the approaches differ to some extent from one another. For instance, the framework suggested by de Almeida et al. (2021) aims for the implementation of ethical principles through a process-oriented and regulative approach that defines the responsibilities and tasks for public institutions and their interactions with industries and service providers, including auditing, certification, standards, and legislation. In turn, by integrating arguments made by Rahwan (2018) and Gasser and Almeida (2017), Wirtz et al. (2020) proposed an integrated, layered AI governance framework. The framework proposes that AI technology and related services present an object of regulation, as they can potentially cause harmful effects and risks. In responding to such negative effects, the regulatory process should use the participatory framing of issues, including assessing costs, benefits, and risks to various stakeholders, evaluating the dynamic impact of regulation, and employing regulatory action for risk management.
The modular frameworks proposed by Wirtz et al. (2020) and Gasser and Almeida (2017) compress the key perspectives on the governance of AI into governance layers as follows: (1) AI technology and services layer, in which we should seek to understand the diversity of AI technologies and their different contexts, leading to context-specific governance and governance mechanisms for different technologies and levels of intervention (technical, organizational, policy); (2) AI challenges layer, in which the variety of societal, ethical, and regulatory impacts and risks of AI are considered and which helps guide policy mechanisms by providing an understanding of the wider societal, ethical, and legal challenges; (3) regulation layer, in which the challenges and responsibilities that should be addressed by AI regulation and coordination are defined; (4) public policy layer, including the implementation of hard and soft governance mechanisms, which can be social and legal norms, regulation and legislation, or ethical principles and codes of conduct, as well as technical and organizational practices such as data management tools, standards, and certifications; policy implementation should take into account the various contexts and levels of implementation in the technical layer and involve various forms of cooperation between different actors and stakeholders; (5) collaborative layer, in which stakeholder goals and conflicting interests are balanced; in this layer, it is important to build trust, shared values, and motivation among different stakeholders (Wirtz et al., 2020, p. 825). The operations should facilitate consensus-building between among the stakeholders and support cost–benefit analyses of values and interests.
As yet, these models are all theoretical and have not been empirically tested or systematically co-created with stakeholders. They are tentative suggestions of aspects that could be considered in the public governance of AI, aiming to support public policy-makers in the development of AI governance.
Processes for Improving Public Governance and Coordination of AI
The second identified theme deals with the question of how to improve public governance and decision-making by considering the contextual and changing nature of AI use and impacts, while avoiding challenges associated with emerging technology governance. The proposed solutions emphasize the following three approaches: (a) use of agile and adaptive forms of governance processes, based on stakeholder inclusion and RRI principles, (b) integration of the notion of constant technological change into governance practices, and (c) use of the co-regulation process for developing regulations (see Table 3).
The agile and adaptive governance approaches are based on empowering stakeholders in decision-making and flexibly reconciling various interests and views. The governance is characterized by constant adaptation as technology evolves—general principles are needed to guide action, but with enough flexibility to respond to constant changes (Gasser and Almeida, 2017; Wallach and Marchant, 2018; Winfield and Jirotka, 2018; Sun and Medaglia, 2019; Ulnicane et al., 2021). Policy-makers have a crucial role in steering policy to tackle societal challenges. In this context, the role of the state should be understood more broadly than as a market corrector. It has different roles from managing risks to supporting inclusion and mediating different needs and interests (Ulnicane et al., 2021). Adaptive governance is based on decentralized, bottom-up decision-making, the use of internal and external expertise, broad participation, and the continuous adaptation of governance to uncertainty (Sun and Medaglia, 2019, paraphrasing Janssen and van der Voort, 2016). Such processes are intended to support an up-to date understanding of AI and its developments in public governance, as well as to ensure that the governance mode is based on a set of values and principles that allow for changes and adaptation in response to changing circumstances. Winfield and Jirotka (2018) and Wallach and Marchant (2018), in turn, proposed agile and responsive forms of governance, in which the role of public administration is seen as an enforcer of soft governance mechanisms. Agile, ethical governance aims to ensure that innovation activities are in the public interest by considering a broad range of stakeholder perspectives, following responsible and ethical principles in innovation processes, and adapting agilely to new situations. From this viewpoint, soft governance mechanisms and their enforcement become key governance tools for public administration.
Notions of agile forms of governance are linked to RRI, which, according to Ulnicane et al. (2021) and Winfield and Jirotka (2018), should underline AI governance development. RRI aims to better align both the process and outcomes of R&I with the values, needs, and expectations of society. RRI principles include anticipation (analysis of the social, economic, and environmental impacts of innovation activity), reflexivity (considering underlying motivations and purposes for participating in the innovation activity openly), inclusiveness (bringing into the common discussion various stakeholder and citizen interests, values, and perspectives), and responsiveness (learning and changing of target-setting and operative practices) (Owen et al., 2013). RRI may complement ethical governance, especially by dealing with the ethical issues in an anticipatory and reflexive manner. As a precondition for RRI in AI governance, Buhmann and Fieseler (2021) have argued that AI policies should be the subject of a critical public debate, reflecting the empowered voice and perspective of the “ordinary citizen”. However, the dialogue on AI ethics and responsibility is complicated by asymmetries of information. This requires outlining practical ways in which the AI debate might become more accessible to citizens. For this purpose, they proposed communicative principles as enablers of meaningful discourse. These include (a) open forums, where every actor can participate in the debate; (b) the maximization of actors' knowledge on the topic at hand; (c) the inclusion of all arguments so that the issue can be assessed from all possible angles; and (d) the principle that various proposals and concerns should be able to influence recommendations and decision-making.
A critical notion on soft governance tools was put forward by Clarke (2019), who stated that soft governance tools in self-regulation are important but ineffective in guiding action toward common goals. Instead, they suggested a co-regulatory framework in their comparative analysis of the regulatory alternatives for AI. Co-regulation refers to a model in which industry, stakeholders, and public authorities jointly negotiate on legal obligations. The result is an enforceable set of rules, wherein the process must take into account the needs of all parties and not be distorted by institutional or market forces. Clarke (2019) provided a concrete framework for designing such a regulatory regime. Based on an earlier paper (Clarke and Moses, 2014), they put forward a framework for assessing the transparency of the regulation process, the consideration of stakeholders' interests, the articulation and enforcement of regulatory mechanisms, and accountability.
The idea of co-regulation and stakeholder coordination is also present in the Wallach and Marchant (2018) suggestion of a governance coordinating committee for the implementation of responsive and agile governance at the national and global levels. The committee should represent all stakeholders from industry and civil society to governments and international standards bodies, as well as individuals or communities that are usually underrepresented. The committee would undertake various tasks related to the involvement of different stakeholders in the provision of a common forum for discussions and mediation between conflicting interests. It would disseminate and evaluate information, as well as analyze and develop soft and hard policy instruments. Additionally, de Almeida et al. (2021), Cath et al. (2018), and Floridi et al. (2018) have proposed coordinating organizations that would bring stakeholders together. In addition to Wallach and Marchant's description, they proposed that the coordinating organization should support collecting and analyzing data, assist and advise different stakeholders in the development of socially and environmentally sustainable AI, conduct foresight analysis to define the envisioned and desired future, and provide recommendations and guidelines for action.
Liu and Maas (2021) have taken a different approach to AI governance, accounting for the long-term AI challenges and changing conditions of its governance and related policy adaptation. They emphasize that current governance processes and policies lack the capacity to adapt to changes induced by fast-paced technology innovation and thus to secure long-term strategies on governing AI. This makes governance approaches insufficient in the face of problems created by AI. While existing governance focuses on the application and development of policies and governance mechanisms, bridging concrete policies and governance solutions with a long-term governance strategy requires a proactive, anticipatory, and future-oriented perspective, which Liu and Maas (2021) call a “problem finding” approach to governance. Such an approach should be based on research on and responsiveness to knowledge on what the potential long-term problems and challenges of AI and its governance will be, and how the conditions and possibilities for the desired governance and its operationalization may change.
Ethics and Human Rights in Policy-Making
The crux of the third theme is the way ethics principles and human values can be used to foster normative ethical governance. A major challenge for ethical and human rights principles is their weak implementation and adoption in AI policy-making and organizational practices. The reviewed literature mostly considered the lack of operationalizable ethics principles, tools, and processes (Floridi et al., 2018; Rahwan, 2018; Wallach and Marchant, 2018; de Almeida et al., 2021; Delacroix and Wagner, 2021; Stix, 2021; Tsamados et al., 2021), as well as the implementation of human rights as a value basis for governance (Donahoe and Metzger, 2019). Although in practice there can be considerable variation in the way human rights are interpreted and implemented in different cultural, organizational, and administrative contexts, and there can be a normative bias toward western values, Donahoe and Metzger (2019) have argued governance based on internationally accepted UN human rights standards should be a normative starting point for the design, development, and use of AI systems. Their approach rests on the idea that a globally accepted set of values is needed as a basis for the governance of AI. First, they claim ethical guidelines drafted by companies or other organizations can be issue- or organization-specific and not designed for governmental policy-making or to form a comprehensive framework for governance. Second, they claim human rights provide a more established and universal value-based approach than the ethical principles of AI. Human rights are already used in the existing regulatory structures and instruments. Accordingly, the question is only how a human rights–based perspective can be put into practice in the governance of AI. Third, they claim that the Universal Declaration of Human Rights is already able to do what ethical frameworks only try to achieve—i.e., taking into account the impact of AI on people. Stix (2021, p. 15), in turn, has argued that the suggested elements for the actionability of ethics principles and human rights are overlapping and complementary. Human rights can serve as the basis for ethical guidelines, as demonstrated by the High-Level Expert Group on AI (European Commission, 2022). Following Stix's arguments, the summary in Table 4 integrates tools and operationalizable principles of ethical principles and human rights.
The suggested concrete operations to support the actionability of normative principles can be categorized as follows: assessment, stakeholder participation, principles of operationalization, and ensuring enforcement. For instance, Stix (2021, p. 7–13) set out three propositions to guide the implementation of ethical principles in policy-making. These include “preliminary landscape assessment” to understand the contextual environment for implementation; “multi-stakeholder participation and cross-sectoral feedback” to address the questions of participation and ways in which principles are drafted; and “mechanisms to support implementation and operationalizability” of the principles to define how to implement them and by whom they should be implemented. Other issues in the literature include the raising of ethical and impact awareness, as well as accountability related mechanisms including calls for oversight and enforcement, auditing, traceability, and transparency (Floridi et al., 2018; Sun and Medaglia, 2019; de Almeida et al., 2021; Tsamados et al., 2021).
Various researchers have claimed that assessment supports the development of principles and guidelines and their application in different contexts. This helps in forming a picture of the current state and potential of an AI system or service, its ethical and human-rights risks, and the extent to which existing regulation and institutional capacities can address ethical issues. By assessing the ability of existing governance and institutional structures to prevent AI risks and support the implementation of ethical principles, it is possible to form an opinion on the need for new regulation and implementation mechanisms. Stakeholder engagement and public debate are key elements in assessing the technical, organizational, legislative, and institutional environment in which AI systems operate and within which their use is governed (Floridi et al., 2018; de Almeida et al., 2021; Stix, 2021).
Stakeholder engagement should be used both in the definition, implementation, and post-implementation stages of ethical or human-rights principles (Delacroix and Wagner, 2021; Stix, 2021). The early involvement of a wide range of stakeholders and citizens, as well as cross-sectoral dialogue among experts, might help ensure that the system works legitimately in terms of democratic and human-rights values (Nemitz, 2018; Donahoe and Metzger, 2019; Stix, 2021). Delacroix and Wagner (2021) even put forward that the legitimacy of ethical frameworks must be questioned if the process is largely driven and managed by the private sector. Public administrations or any organizations aiming to devise AI policies without wider stakeholder consultation risk basing decision-making on one-sided information (Sun and Medaglia, 2019). As a solution, Delacroix and Wagner (2021) have suggested that public administrations should urge professional organizations to contribute to the development of ethical principles. Other authors have argued that such stakeholder involvement could be supported by coordinator organizations (Floridi et al., 2018; Wallach and Marchant, 2018; de Almeida et al., 2021).
The operationalization of principles can be perceived, in turn, as concrete “guidance in the form of a toolbox, or method to operationalize the recommendations” (Stix, 2021, p. 12). In the reviewed literature, such suggestions included both technical and non-technical solutions. Stix (2021) suggested that solutions should include methods and mechanisms to enable civil debate and empower civil society to influence decisions on AI activities. Floridi et al. (2018) provided a list of 20 action points to help policy-makers steer AI for the good of society. The broad range of recommendations include the assessment of current regulations and institutional capabilities; development of legal and coordination procedures, instruments, and institutions; financial incentivization of AI research, principles, and procedures, as well as applications aligning with socially preferable objectives; and support for self-regulation and ethical capacity and awareness-building among the public. The latter includes the idea that education and cross-disciplinary dialogue is needed to ensure that decision makers, AI developers, businesses, and the general public are aware of societal, ethical, and legal implications of AI systems and of concrete recommendations for action (Floridi et al., 2018; Donahoe and Metzger, 2019; Truby, 2020). This includes investing in educating policy-makers, students, and practitioners of relevant fields in subjects of computer science, human rights, and ethics. Other authors have recommended prerequisites for operationalization, such as oversight structures, accountability, traceability, sanction mechanisms, and design to ensure civil debate and influence (Wallach and Marchant, 2018; Truby, 2020; Tsamados et al., 2021), including proposals for the tasks different governing institutions should adopt (de Almeida et al., 2021). Authors have also suggested various technical means and processes for software engineers to address ethical challenges, which are not considered here (Truby, 2020; Tsamados et al., 2021).
Rahwan (2018) has suggested that ethical values and the involved trade-offs should be articulated in a way that engineers and designers can operationalize. Specifically, values should be codified, and the social impacts of algorithms should be quantified to allow for machine-mediated value negotiation between different stakeholders and to help in monitoring compliance with the agreed-upon rules and standards. However, Clarke (2019) has countered such views by stating the following: “No means exists to encode into artifacts human values, nor to embed within them means to reflect differing values among various stakeholders, nor to mediate conflicts among values and objectives […]” (p. 403). The extent to which procedural means to negotiate values and principles can be supported by technical solutions is still an open question in the literature, although various software tools designed to address, minimize, or avoid the ethical risks of AI also exist (Tsamados et al., 2021).
Tools and Tasks for Governing Institutions
The implementation challenge of ethics principles was a crosscutting issue to which several concrete tools and institutional arrangements were proposed in the reviewed papers (Table 5 below). Concrete recommendations for public governing institutions such as oversight, monitoring, and enforcement emerged as a part of a broader set of suggestions to improve implementability of ethics principles (e.g., Floridi et al., 2018; Wallach and Marchant, 2018; Truby, 2020; Tsamados et al., 2021). As observed by Tsamados et al. (2021), a focal ethical concern is that of assigning moral responsibility to someone in the case of wrongdoings and enabling the traceability of causes of wrongdoings in AI systems. Traceability, oversight, and auditing emerge as tools to enable the legally mandated oversight and evaluation of AI systems and to increase compliance with ethics or human-rights standards (e.g., Bannister and Connolly, 2020; de Almeida et al., 2021) and the Sustainable Development Goals (Truby, 2020). In Wallach and Marchant (2018) view, public authorities should also be able to enforce soft-governance rules (or soft laws). For instance, they could require industrial actors to follow standards, like ISO 9000 for quality management. Companies that can consistently demonstrate meeting the criteria could then apply for certification.
Some authors argued that the governance for the common good should include independent institutions for oversight, enforcement, and compliance to minimize the risks of AI and ensure the actionability of ethics or human-rights principles (Floridi et al., 2018; Bannister and Connolly, 2020; Dignam, 2020; de Almeida et al., 2021). For instance, de Almeida et al. (2021) proposed a regulatory agency with a broad array of tasks ranging from assessing data governance and risk-mitigation measures to auditing, certification, and standardization. Floridi et al. (2018), Dignam (2020), and Bannister and Connolly (2020) have offered analogous suggestions by comparing the needed agency to supervisory agencies found in the medical sector, i.e., an agency that would monitor and approve the use of algorithms through a process of evaluation and supervision. The executor of these tasks need not necessarily be a separate institution—instead, a group of agencies or ministries could also perform similar tasks (Clarke, 2019; de Almeida et al., 2021).
Furthermore, there are a number of various concrete tasks that have been suggested for governance institutions, from the monitoring and approval of algorithms to the development of risk-management mechanisms. In the following table, we summarize the major tasks suggested in the literature.
Discussion: Toward A Comprehensive, Inclusive, Institutionalized, And Actionable Model Of AI Governance (CIIA)
The governance procedures and normative principles adopted by public administrations are key factors in promoting ethical and responsible technology development. Our review suggests that such principles and governance practices cannot be meaningfully separated from each other. Thus, based on the review, we suggest four general dimensions to be considered and integrated into AI governance frameworks. By following the core observations of the thematic analysis, we outline these dimensions as follows: (1) Comprehensiveness (a need for a comprehensive governance approach that acknowledges the systemic nature of AI and its governance—including a need for a horizontal and cross-sectoral governance—and works to provide public governing institutions and other relevant actors reference models for the governance of AI). (2) Inclusiveness (a need for engaging various stakeholder views and values in a dialogic process to form adaptive and acceptable governance models in complex and rapidly changing social and technological contexts). (3) Institutionalization (a need for public institutions and governance tools to ensure lasting arrangements for oversight and compliance with ethical standards). (4) Actionability (a need for concrete and actionable ethical principles and human rights in policy-making).
Comprehensiveness
AI governance frameworks should be all-encompassing, enough so to account for the systemic and multi-dimensional nature of the AI phenomenon and its governance challenges (Gasser and Almeida, 2017; Cath et al., 2018; Donahoe and Metzger, 2019; Wirtz et al., 2020; de Almeida et al., 2021). For this purpose, frameworks can be, as suggested, multi-layered or modular (Gasser and Almeida, 2017; Wirtz et al., 2020) in a way that connects multiple levels of AI governance from legislation to ethics and technical solutions with an understanding of the short- and long-term effects of AI implementation. It is important that the framework accounts for the complexity and interconnectedness of various governance and application contexts. Wide horizontal views of governance and collaboration are important in tackling cross-sectoral and multi-disciplinary governance challenges of AI. This may also require a mission-oriented public administration approach, which starts “from the societal challenge and task at hand and working one's way from there, rather seeking to find the solutions through variable and flexible pathways than of basing the activity to a planning-based structure of steps and milestones” (Lähteenmäki-Smith, 2020, p. 6).
Inclusiveness
Being a generic technology, which can be applied in a number of contexts and connected to various other technologies, AI needs to be understood as a contextual phenomenon, requiring context-specific governance mechanisms to address societal, ethical, and legal challenges. Contextuality emphasizes the inclusion of various stakeholder and citizen views, knowledge, and values in governance processes. Complexity requires granular and flexible responses, which are fostered by collective understanding, consensus building, and deliberation regarding potential challenges, impacts, and values in every situation. Due to the constant change of the technology and social contexts, governance should be adaptive and agile to both short- and long-term challenges (Wallach and Marchant, 2018; Winfield and Jirotka, 2018; Sun and Medaglia, 2019; Liu and Maas, 2021; Ulnicane et al., 2021). Such forms of governance and coordination need to address AI-related social and technological complexity, especially through broad stakeholder participation and dialogue that can be used as an informational basis for decision-making and value formulation. Understanding the needs and interests of stakeholders and including their unique knowledge of various technological contexts and social situations can help to reduce information asymmetries and policy uncertainty. Inclusiveness can be supported through specific organizations or organizational arrangements aiming to coordinate and bring together information and stakeholders in mutual dialogue and learning.
Institutionalization
To avoid ad hoc, scattered, and non-coordinated governance initiatives and arrangements, the policy and governance should be clearly coordinated and institutionalized either by embedding it in existing administrative and regulatory structures or by establishing a separate agency (or agencies) for the purpose (Floridi et al., 2018; Bannister and Connolly, 2020; Dignam, 2020; de Almeida et al., 2021). Tasks for a public governing structure would include AI-related decision-making, oversight and approval, auditing, risk management, certification, standardization, and legislation development. The structure would develop, test, and stabilize appropriate governance instruments and approaches in the short and long term. As suggested in the reviewed literature, the tasks could also include providing insights on general ethical issues related to AI and impact assessments to be used in legislation and policy-making. The institutional procedures for decision-making would include interaction with stakeholders and responsiveness accordingly.
Actionability
A focal challenge in integrating ethical principles and human rights in public AI governance is how they can be operationalized in practice. Operationalizability or actionability of principles and rights may include various mechanisms and tools to help in their implementation. The literature includes a broad range of recommendations from mechanisms of civil debate to various assessment and auditing procedures, financial incentivization, and support for self-regulation (Floridi et al., 2018; Rahwan, 2018; Wallach and Marchant, 2018; Donahoe and Metzger, 2019; Sun and Medaglia, 2019; de Almeida et al., 2021; Delacroix and Wagner, 2021; Stix, 2021; Tsamados et al., 2021). To be effective, however, the implementation of such mechanisms should become a part of responsibility and accountability schemes, which are linked directly to various oversight, enforcement, and sanction mechanisms.
In an attempt to merge and integrate the CIIA principles (comprehensive, inclusive, institutionalized, and actionable) and the major ideas behind them in the review, we propose the following comprehensive approach for the governance of AI (Figure 2). The presented CIIA model is intended as an ideal typical model that can be used as a reference point for governance developers, as well as further research on the issue. We used the ideal type (Stanford Encyclopedia of Philosophy., 2017; Swedberg, 2018) to refer to a combination of the most essential characters of a phenomenon. It is an analytical construct that cannot be found empirically; however, its validity is ascertained by adequacy. In this case, the model integrates and visualizes the most important features of the reviewed governance mechanisms and principles. It does not correspond to particular cases but functions as a general comparative point for the development or assessment of governance practices.
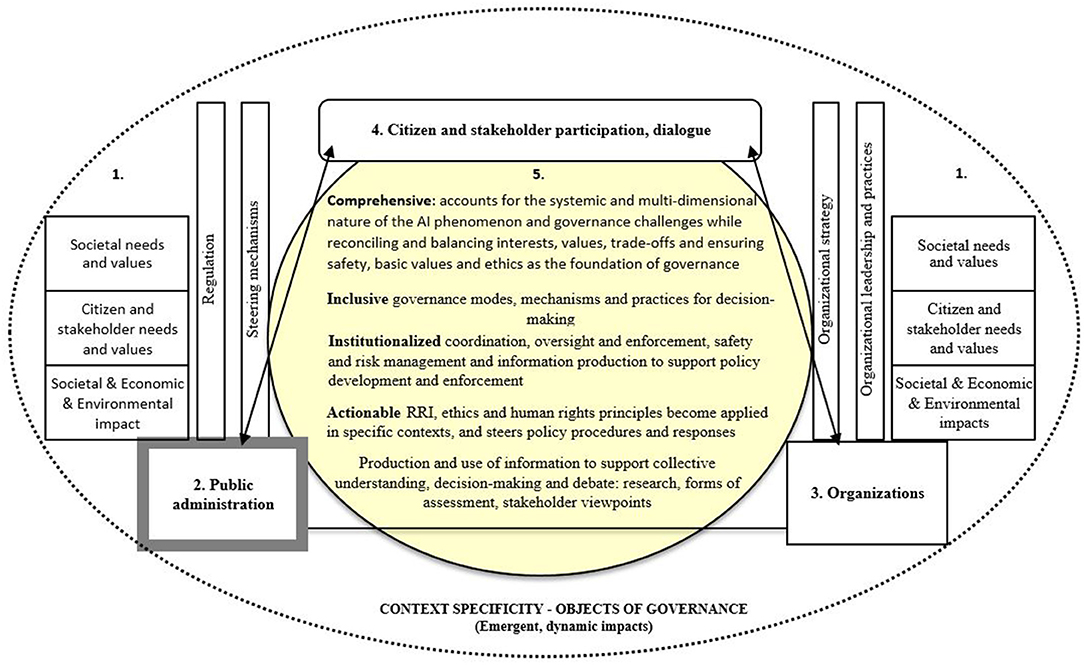
Figure 2. Comprehensive, inclusive, institutionalized, and actionable framework of AI governance (CIIA).
While AI governance mechanisms can be studied, inter alia, from technical tools to organizational leadership, external oversight, and policies (Shneiderman, 2020; Stahl, 2021), our ideal typical model focuses especially on the governance interface between public administrations and organizations using or developing AI. A key assumption in the model is that the informational and value basis of the procedures and substantive rules determine the possibility for ethical and responsible outcomes. To move toward the ethical development and use of AI, the principles for good governance, human rights and ethics, and the procedures of RRI should be integrated in the governance approach alongside procedures for adaptation to short- and long-term challenges and strategic objectives (e.g., Winfield and Jirotka, 2018; Yeung et al., 2019; Buhmann and Fieseler, 2021; Liu and Maas, 2021; Ulnicane et al., 2021).
In the model, the starting point for the need for and target-setting and legitimacy of public governance are the societal and citizen needs and values, as well as the impacts of AI (number 1 in Figure 2). The needs relate to the capacities and possibilities of AI applications in societal use—e.g., their potential to improve services and safety or increase efficiency. While, for instance, efficiency and safety are also societal values, we refer more widely to human-rights values and ethical values. As there can be tensions or even contradictions between various needs and values, governance is needed as a mechanism to normatively define the public interest by balancing the interests of different stakeholders and ensuring an inclusive process of integrating various human values and ethical standpoints as the foundation of technology development and implementation (e.g., Baldwin et al., 2012; Cath et al., 2018; Yeung et al., 2019). In balancing various interest and values, governing institutions must be critically aware of, and account for, the various short- and long-term social, economic and environmental effects of AI implementation both locally and globally (e.g., Liu and Maas, 2021). To manage this, there needs to be a comprehensive model that enables furthering the collective understanding of the phenomena, sustainable policy responses and their oversight, and the enforcement mechanisms as part of a holistic governance system.
Governance is a multidimensional phenomenon, which we earlier defined broadly as referring to processes related to decision-making, implementation, and organized interaction between different actors. Numbers 2–3 in Figure 2 refer to the key stakeholder dimensions. Public administration is seen as the key public governance institution coordinating, overseeing, and enforcing various hard- and soft-governance forms. The public, private, and semi-public organizations' governance indicates the legal compliance, self-organization, and implementation (or non-implementation) of ethical standards and practical means for actionability. In addition, the interactions among the public administration, public and private organizations, stakeholders, and citizens are an essential dimension of ethical and responsible governance in highly complex environments.
Public administrations (number 2 in Figure 2) have a specific responsibility of sensitivity to various societal needs and values and ensuring that various forms of governance, norm-setting, steering, and sanctions are compatible and balanced. They also must take into account the application of AI in various settings, environments, and for different objectives and potential uses with varying impacts. In this role, public administrations function as “brokers” between various social interests and values, while having their own interest in defining and enforcing the public good (e.g., Nemitz, 2018; Donahoe and Metzger, 2019; Yeung et al., 2019; Delacroix and Wagner, 2021; Stix, 2021).
Organizations (number 3 in Figure 2) self-organize their actions strategically and operationally to manage internal and external expectations and create practices to pursue their goals. Depending on the organization, they may involve themselves in various internal and external activities in AI governance–related questions. Aside from complying with legal norms, organizations (like industries) are also stakeholders, whose representatives put forward their interests to affect the framing and norm-setting in various dialogues and interactions with the public administration, other stakeholders, and citizens. Organizations may also need public and other support attempting to ethically implement and govern AI. This support may include, for example, information sharing, providing ethical guidelines that are based on human rights and broadly shared norms, structures supporting AI impact assessment, certification, and financial support for innovative and responsible socio-technical solutions (e.g., Floridi et al., 2018; Rahwan, 2018; Wallach and Marchant, 2018; Sun and Medaglia, 2019; de Almeida et al., 2021). Depending on the organizations and their goals, they may also put varying emphasis on different internal and external interests. It is expected that industries primarily respond to shareholder interests, promoting a logic of profit accumulation in AI development and use (Crawford, 2021), while public or semi-public organizations might function according to other objectives. A common industry response to observations about the ethical challenges of AI and subsequent calls for regulation are self-regulatory ethical guidelines. Some observers have been critical of self-regulatory approaches to ethical AI and have called for compliance with general principles of deliberation, testing and evaluation, oversight, and sanctions (Yeung et al., 2019).
Besides organizations and industries, citizens are important stakeholders in democratic societies (number 4 in Figure 2). It is important that citizens' values and interests are integrated into governance processes, as it strengthens the democratic basis, functionality, and legitimacy of governance (e.g., Delacroix and Wagner, 2021; Stix, 2021). Furthermore, governing AI in complex and varied social contexts (context specificity in Figure 2) increases informational asymmetries and policy uncertainty (Taeihagh, 2021). Addressing the complexity requires a firm informational basis that is linked to grass-roots understandings of situational and societal phenomena with a value base. Wicked or so-called systemic problems (Rittel and Webber, 1973) further emphasize the importance of gaining systemic knowledge and building mutual understanding through the wide inclusion of and deliberation among stakeholders with local and situational information (Sun and Medaglia, 2019; Liu and Maas, 2021).
Governance needs to consider both the context specificity and the emergent and dynamic long-term effects of AI and the policies aimed at its regulation and coordination (number 5 in Figure 2). The core of Figure 2 covers the general CIIA principles, as defined above, to be integrated into AI governance. By following these principles, the governance should be context-specific and agile in relation to emergent AI-related questions, reconcile and balance various interests and values, and be able to legitimate trade-offs while ensuring safety and risk management. Governance uses widely diverse information to support collective understanding, decision-making and debate including academic research, various evaluation and assessment forms, and stakeholder and citizen perspectives. Ethical AI governance uses and incorporates actionable ethics, human-rights, and RRI principles (anticipation of impacts, engagement of stakeholders in a dialogue, reflexivity on motivations, and responsiveness to information) in policy-making. It also uses inclusive and flexible governance mechanisms and practices to support decision-making adaptability to short- and long-term governance challenges. In addition, socially sustainable and long-term development of AI governance requires institutionalized coordination, oversight and enforcement, safety and risk management, and information production. However, defining AI as an object of governance in terms of different contexts and dynamic long-term effects and ethical risks can be problematic. One potential way to reduce complexity is to adhere to Gahnberg's (2021) proposal to recognize AI through the fundamental properties of its immediate agency, defined as the way its performance is measured, its operating environment, the technology through which it makes an effect, and sensors for collecting data.
A crucial aspect of the model is that the public administration aims to correct power imbalances between stakeholders influencing policy-making by requiring private stakeholders to follow some substantive and procedural normative principles. Awareness of the power dynamics involved in the framing and use of AI is a fundamental concern for the balanced deliberation of ethical AI. Crawford (2021) has expressed concern over the extent to which industry narratives have successfully managed to frame AI and its ethical considerations as separate from the harmful material and social conditions of its value production. These conditions may include the extraction of minerals and other environmental resources, bad labor conditions, and highly questionable collection and use of data. Framing is central to how AI problems are understood in policy papers and, consequently, what kinds of solutions are developed. Framing is also performative in the sense that it shapes our thinking on what kind of governance is relevant, urgent, possible, or necessary (Konrad and Böhle, 2019; Mager and Katzenbach, 2021). Such framing partially guides the funding of AI, objectives of AI development, and AI governance principles (Ulnicane et al., 2020; Bareis and Katzenbach, 2021). In essence, the framing of AI depends on the extent to which various stakeholder views are taken into consideration in the forming of AI strategies, collective sociotechnical imaginaries, and the anticipation of impacts (Radu, 2021). This is why, according to Crawford (2021, p. 255), there is an apparent need to understand the “lived experience of those who are disempowered, discriminated against, and harmed by AI systems” to shed light on the potential (and often ignored) harms caused by the systemic conditions for AI value production.
Ethical governance of AI intertwines with the general paradigmatic, political, and ideological considerations of governance and the role of the state. Many of the suggested AI governance solutions seem to be congruent with the key characteristics of the so-called New Public Governance (NPG) paradigm that refers roughly to horizontal inter-organizational coordination and collaboration, as well as the strong involvement of citizens and other societal actors in public governance (Torfing et al., 2020). Similar governance features can also variably be found in notions of agile, adaptive, tentative, and anticipatory governance (Kuhlmann et al., 2019; Lehoux et al., 2020; Taeihagh et al., 2021). However, along with NPG congruence, various researchers have expressed the strong need for vertical control in enforcing and overseeing the implementation of substantive and procedural norms. This suggests an actual overlap of NPG-related approaches with other governance paradigms like classical hierarchical bureaucracy. In fact, it might be counter-productive to restrict proposed solutions to any single governance paradigm, and policy-makers should instead aim to adapt and mix different governance modes to find the best solutions for particular contexts (Rhodes, 2016). The CIIA model suggests that both types of approaches are important and that governance should be flexible and adaptive in this sense, as contexts of application vary considerably. Thus, in practice, ethical governance incorporates various governance mechanisms that may hinder unethical and harmful operations and value production. Furthermore, in line with ethical governance (Winfield and Jirotka, 2018), the CIIA model assumes that public administration should adhere to the norms and principles of democracy and good governance.
While the existing studies on public governance of AI focus on defining governance targets and mechanisms, there is an apparent and urgent need for empirical experimentations and studies on how new forms of governance and the operationalization of major principles of good AI governance may be successfully implemented. To our knowledge, there is currently little empirical basis for the understanding of AI ethics challenges and potential responses to the application of AI in different contexts and places (Sun and Medaglia, 2019; Stahl et al., 2021) or real-world cases of stakeholder engagement and public deliberation in devising the governance of AI. Empirical studies and experimentations would serve as practical benchmarks in functional and non-functional practices for policy-makers and public administrations in ethical AI governance in varying technological, administrative, and social contexts.
Conclusions
Increasing the utilization of AI in society challenges public governance institutions to respond in a way that supports the possibility of beneficial effects to individuals, businesses, and society. Calls to leverage AI for the common good and perceived insufficiency of current practices in fostering such processes are pressuring public administrations to rethink the rationale for applying traditional regulatory governance mechanisms and adopt new modes of governance.
Current literature on how public administrations should develop the governance of AI for the common good is relatively scant. There has been a need for an updated and integrated perspective on how public administration could facilitate the formulation, adoption, and implementation of ethics and human rights in policy-making and organizational practices. To further the discussion on the governance of AI for the common good, our review explored means for its public governance. The review integrates the various approaches, principles, and tools suggested for developing governance and offers an updated, thematically argued, and broad perspective compared to previous integrative models (Wirtz et al., 2020; de Almeida et al., 2021).
As a summary of the review, we propose a CIIA framework that integrates the key aspects of the proposed development solutions into an ideal typical and comprehensive, general model for AI governance. The four dimensions sum up the following principles: (a) AI governance frameworks should be comprehensive enough to account for the systemic and multi-dimensional nature of the AI phenomenon and horizontal, cross-sectoral governance challenges; (b) governance needs to address AI-related social and technological complexity through broad stakeholder participation and dialogue that can be used as an informational basis for decision-making; (c) policy and governance should be coordinated and institutionalized either by embedding it in the existing administrational and regulative structures or by establishing a separate organ(s) for the purpose; (d) and general ethical and good governance principles of AI must be actionable via concrete mechanisms and tools to help in the implementation and be connected to various oversight, enforcement, and sanction mechanisms.
Besides functional governance mechanisms that are designed especially to solve societal challenges caused by the implementation of AI, there is also a need to consider the influence of political ideologies and institutionalized policies. The proposed solutions may presuppose an ideological and paradigmatic shift in the way public governance is organized in general. The implementation of principles summarized in the CIIA model imply moving toward public administration that emphasizes increasingly inclusive collaboration and horizontal coordination while retaining some forms of traditional bureaucratic hierarchy for enforcement and oversight. At present, it seems that we do not have any systematic real-life experimentation or empirical research on governance for ethical AI. Thus, there is an apparent need for empirical experimentations and studies on how new forms of AI governance may be implemented.
Data Availability Statement
The original contributions presented in the study are included in the article/supplementary material, further inquiries can be directed to the corresponding author.
Author Contributions
AS is the main author of the text and the main person who conducted the literature selection and analysis. MN participated in the writing and provided key input in the revisions of the analysis and in the suggested dimensions and model. JL contributed to the model construction and manuscript revision by commenting and suggesting revisions. PP contributed to the literature selection process and review, the methods development, and citations and reference entries in the final version. The order of authorship reflects the relative extent of contribution. All authors contributed to the design of the review, manuscript writing or revision, model construction, and approval of the submitted version.
Funding
The article was written in the research project Ethical AI for the Governance of the Society (ETAIROS, Grant Number 327356), funded by the Strategic Research Council at the Academy of Finland.
Conflict of Interest
The authors declare that the research was conducted in the absence of any commercial or financial relationships that could be construed as a potential conflict of interest.
Publisher's Note
All claims expressed in this article are solely those of the authors and do not necessarily represent those of their affiliated organizations, or those of the publisher, the editors and the reviewers. Any product that may be evaluated in this article, or claim that may be made by its manufacturer, is not guaranteed or endorsed by the publisher.
Acknowledgments
The authors wish to acknowledge the project Ethical AI for the Governance of the Society (ETAIROS), funded by the Strategic Research Council at the Academy of Finland. Any opinions, findings, and conclusions or recommendations expressed in this material are those of the authors and do not necessarily reflect the views of the funding agencies.
References
Asaduzzaman, M., and Virtanen, P. (2016). “Governance theories and models,” in Global Encyclopedia of Public Administration, Public Policy, and Governance, ed. Farazmand, A, . New York, USA: Springer. doi: 10.1007/978-3-319-31816-5_2612-1
Baldwin, R., Cave, M., and Lodge, M. (2012). Understanding Regulation: Theory, Strategy, and Practice (2nd ed.). Oxford; New York, NY: Oxford University Press. doi: 10.1093/acprof:osobl/9780199576081.001.0001
Bannister, F., and Connolly, R. (2020). Administration by algorithm: a risk management framework. Informat. Polity. 25, 471–490. doi: 10.3233/IP-200249
Bareis, J., and Katzenbach, C. (2021). Talking AI into being: the narratives and imaginaries of national AI strategies and their performative politics. Sci. Technol. Human Values. 01622439211030007
Borrás, S., and Edler, J. (2020). The roles of the state in the governance of socio-technical systems' transformation. Res. Policy. 49, 103971. doi: 10.1016/j.respol.2020.103971
Buhmann, A., and Fieseler., C. (2021). Towards a deliberative framework for responsible innovation in artificial intelligence. Technol. Soc. 64. doi: 10.1016/j.techsoc.2020.101475
Cath, C., Wachter, S., Mittelstadt, B., Taddeo, M., and Floridi, L. (2018). Artificial intelligence and the “good society”: the US, EU, and UK approach. Sci. Eng. Ethics. 24, 505–528. doi: 10.1016/j.techsoc.2020.101475
Cihon, P. (2019). Standards for AI Governance: International Standards to Enable Global Coordination in AI Research & Development. Future of Humanity Institute. University of Oxford.
Clarke, R. (2019). Regulatory alternatives for AI. Comput. Law Secur. Rev. 35, 398–409. doi: 10.1016/j.clsr.2019.04.008
Clarke, R., and Moses, L. B. (2014). The regulation of civilian drones' impacts on public safety. Comput. Law Secur. Rev. 30, 263–285. doi: 10.1016/j.clsr.2014.03.007
Cronin, M. A., and George, E. (2020). The why and how of the integrative review. Organ. Res. Methods. doi: 10.1177/1094428120935507
Dafoe, A. (2018). AI Governance: A Research Agenda. Governance of AI Program. Future of Humanity Institute. University of Oxford. Available online at: https://www.fhi.ox.ac.uk/wp-content/uploads/GovAI-Agenda.pdf (accessed December 1, 2021).
de Almeida, P. G. R., dos Santos, C. D., and Farias, J. S. (2021). Artificial intelligence regulation: a framework for governance. Ethics Inf. Technol. 23, 505–525. /10.1007/s10676-021-09593-z
Delacroix, S., and Wagner, B. (2021). Constructing a mutually supportive interface between ethics and regulation. Comput. Law Secur. Rev. 40. doi: 10.1016/j.clsr.2020.105520
Dignam, A. (2020). Artificial intelligence, tech corporate governance and the public interest regulatory response. Camb. J. Reg. Econ. Soc. 13, 37–54. doi: 10.1093/cjres/rsaa002
Donahoe, E., and Metzger, M. M. (2019). Artificial intelligence and human rights. J. Democracy. 30, 115–126. doi: 10.1353/jod.2019.0029
European Commission. (2021a). A European Approach to Artificial Intelligence. Available online at: https://digital-strategy.ec.europa.eu/en/policies/european-approach-artificial-intelligence (accessed December 29, 2021).
European Commission. (2021b). Proposal for a Regulation of the European Parliament and of the Council Laying Down Harmonised Rules on Artificial Intelligence (Artificial Intelligence Act) and Amending Certain Union Legislative Acts. Available online at: https://eur-lex.europa.eu/legal-content/EN/TXT/?uri=CELEX%3A52021PC0206 (accessed December 29, 2021).
European Commission. (2021c). The Digital Services Act package. Available online at: https://digital-strategy.ec.europa.eu/en/policies/digital-services-act-package (accessed January 7, 2022).
European Commission. (2022). High-Level Expert Group on Artificial Intelligence. Available online at: https://digital-strategy.ec.europa.eu/en/policies/expert-group-ai (accessed January 14, 2022).
Floridi, L. (2018). Soft ethics and the governance of the digital. Philos. Technol. 31, 1–8. doi: 10.1007/s13347-018-0303-9
Floridi, L., Cowls, J., Beltrametti, M., Chatila, R., Chazerand, P., Dignum, V., et al. (2018). AI4People—an ethical framework for a good AI society: opportunities, risks, principles, and recommendations. Minds Mach. 28, 689–707. doi: 10.1007/s11023-018-9482-5
Floridi, L., Cowls, J., King, T. C., and Taddeo, M. (2020). How to design AI for social good: seven essential factors. Sci. Eng. Ethics. 26, 1771–1796. doi: 10.1007/s11948-020-00213-5
Frederickson, H. G. (2007). “Whatever happened to public administration? Governance, governance everywhere” in The Oxford Handbook of Public Management, ed, Ferlie, E., Lynn Jr. L. E., and Pollitt, C. New York, NY: Oxford University Press. doi: 10.1093/oxfordhb/9780199226443.003.0013
Future of Life Institute. (2021). AI Policy Challenges and Recommendations. Availabe online at: https://futureoflife.org/ai-policy-challenges-and-recommendations/ (accessed December 29, 2021).
Gahnberg, C. (2021). What rules? Framing the governance of artificial agency. Policy Soc. 40, 194–210.
Gasser, U., and Almeida, V. A. (2017). A layered model for AI governance. IEEE Int. Comput. 21, 58–62. doi: 10.1109/MIC.2017.4180835
Gutierrez, C. I., Marchant, G. E., and Michael, K. (2021). Effective and trustworthy implementation of AI soft law governance. IEEE Technol. Soc. Mag. 2, 168–170. doi: 10.1109/TTS.2021.3121959
Hagendorff, T. (2020). The ethics of AI ethics: an evaluation of guidelines. Minds Mach. 30, 99–120. doi: 10.1007/s11023-020-09517-8
Ireni-Saban, L., and Sherman, M. (2021). Ethical Governance of Artificial Intelligence in the Public Sector. London: Routledge.
Janssen, M., and van der Voort, H. (2016). Adaptive governance: towards a stable, accountable and responsive government. Gov. Inf. Q. 33, 1–5. https://doi.org/10.1016/j.giq.2016.02.003
Jobin, A., Ienca, M., and Vayena, E. (2019). The global landscape of AI ethics guidelines. Nat. Mach. Intell. 1, 389–399. doi: 10.1038/s42256-019-0088-2
Konrad, K., and Böhle, K. (2019). Socio-technical futures and the governance of innovation processes: An introduction to the special issue. Futures. 109, 101–107. doi: 10.1016/j.futures.2019.03.003
Kuhlmann, S., Stegmaier, P., and Konrad, K. (2019). The tentative governance of emerging science and technology: a conceptual introduction. Res. Policy. 48, 1091–1097. doi: 10.1016/j.respol.2019.01.006
Lähteenmäki-Smith, K., Samuli, M., Vartiainen, P., Uusikylä, P., Jalonen, H., Kotiranta, S., et al. (2021). Valtion ohjaus 2020—luvulla. Säädös—ja resurssiohjauksesta järjestelmänavigointiin. Valtioneuvoston Selvitys—ja Tutkimustoiminnan Julkaisusarja. 2021, 17.
Lähteenmäki-Smith, K., and Virtanen, P. (2020). ”Mission-oriented public policy and the new evaluation culture,” in Society as an Interaction Space: A Systemic Approach, ed. Lehtimäki, H., Uusikylä, P., and Smedlund, A., (eds). Singapore: Springer.
Larsson, S. (2020). On the governance of artificial intelligence through ethics guidelines. Asian J. Law Soc. 7, 437–451. doi: 10.1017/als.2020.19
Lehoux, P., Miller, F. A., and Williams-Jones, B. (2020). Anticipatory governance and moral imagination: methodological insights from a scenario-based public deliberation study. Technol. Forecast. Soc. Change. 151, 119800. doi: 10.1016/j.techfore.2019.119800
Liu, H. Y., and Maas, M. M. (2021). “Solving for X?” Towards a problem-finding framework to ground long-term governance strategies for artificial intelligence. Futures. 126, 102672. doi: 10.1016/j.futures.2020.102672
Mager, A., and Katzenbach, C. (2021). Future imaginaries in the making and governing of digital technology: multiple, contested, commodified. New Media Soc. 23, 223–236. doi: 10.1177/1461444820929321
Mittelstadt, B. (2019). Principles alone cannot guarantee ethical AI? Nat. Mach. Intell. 1, 501–507. doi: 10.1038/s42256-019-0114-4
Morley, J., Floridi, L., Kinsey, L., and Elhalal, A. (2020). From what to how: An initial review of publicly available AI ethics tools, methods and research to translate principles into practices. Sci. Eng. Ethics. 26, 2141–2168. doi: 10.1007/s11948-019-00165-5
Nemitz, P. (2018). Constitutional democracy and technology in the age of artificial intelligence. Philos. Trans. Royal Soc. 376, 20180089. doi: 10.1098/rsta.2018.0089
Nowell, L. S., Norris, J. M., White, D. E., and Moules, N. J. (2017). Thematic analysis: Striving to meet the trustworthiness criteria. Int. J. Qual. Methods. doi: 10.1177/1609406917733847
OECDAI Policy Observatory. (2021). OECD AI Principles Overview. Available online at: https://oecd.ai/en/ai-principles (accessed August 26, 2021).
Okoli, C. (2015). A guide to conducting a standalone systematic literature review. Commun. Assoc. Inf. Syst. 37, 43. doi: 10.17705/1CAIS.03743
Page, M. J., McKenzie, J. E., Bossuyt, P. M., Boutron, I., Hoffmann, T. C., Mulrow, C. D., et al. (2021). The PRISMA 2020 statement: an updated guideline for reporting systematic reviews. Br. Medical J. 372, n71. doi: 10.1136/bmj.n71
Radu, R. (2021). Steering the governance of artificial intelligence: national strategies in perspective. Policy Soc. 40, 178–193. doi: 10.1080/14494035.2021.1929728
Rahwan, I. (2018). Society-in-the-loop: programming the algorithmic social contract. Ethics Inf. Technol. 20, 5–14. doi: 10.1007/s10676-017-9430-8
Rittel, H. W. J., and Webber, M. M. (1973). Dilemmas in a general theory of planning. Policy Sci. 4, 155–169. doi: 10.1007/bf01405730
Shneiderman, B. (2020). Bridging the gap between ethics and practice: Guidelines for reliable, safe, and trustworthy human-centered AI systems. ACM Trans. Interact. Intell. Syst. 10, 1–31. doi: 10.1145/3419764
Smuha, N. A. (2020). Beyond a human rights-based approach to AI governance: promise. Philos. Technol. 34, 1–14. doi: 10.1007/s13347-020-00403-w
Stahl, B. (2021). Artificial intelligence for a better future. An ecosystem perspective on the ethics of AI and emerging digital technologies. Springer Briefs in Research and Innovation Governance. doi: 10.1007/978-3-030-69978-9
Stahl, B. C., Andreou, A., Brey, P., Hatzakis, T., Kirichenko, A., Macnish, K., et al. (2021). Artificial intelligence for human flourishing: Beyond principles for machine learning. J. Busi. Res. 124, 374–388. doi: 10.1016/j.jbusres.2020.11.030
Stanford Encyclopedia of Philosophy. (2017). Max Weber. Available online at: https://plato.stanford.edu/entries/weber/#IdeTyp (accessed January 3, 2022).
Stix, C. (2021). Actionable principles for artificial intelligence policy: three pathways. Sci. Eng. Ethics. 27, 1–17. doi: 10.1007/s11948-020-00277-3
Sun, T. Q., and Medaglia, R. (2019). Mapping the challenges of artificial intelligence in the public sector: evidence from public healthcare. Gov. Inf. Q. 36, 368–383. doi: 10.1016/j.giq.2018.09.008
Swedberg, R. (2018). How to use Max Weber's ideal type in sociological analysis. J. Class. Soc. 18, 181–196. doi: 10.1177/1468795X17743643
Taddeo, M., and Floridi, L. (2018). How AI can be a force for good. Science. 361, 751–752. doi: 10.1126/science.aat5991
Taeihagh, A. (2021). Governance of artificial intelligence. Policy Soc. 40, 137–157. doi: 10.1080/14494035.2021.1928377
Taeihagh, A., Ramesh, M., and Howlett, M. (2021). Assessing the regulatory challenges of emerging disruptive technologies. Regul. Gov. 15, 1009–1010. doi: 10.1111/rego.12392
Tomašev, N., Cornebise, J., Hutter, F., Mohamed, S., Picciariello, A., Connelly, B., et al. (2020). AI for social good: unlocking the opportunity for positive impact. Nature Commun. 11, 1–6.
Torfing, J., Andersen, L. B., Greve, C., and Klausen, K. K. (2020). Public Governance Paradigms: Competing and Co-existing. Cheltenham: Edward Elgar Publishing. doi: 10.4337/9781788971225
Torraco, R. J. (2016). Writing integrative literature reviews: Using the past and present to explore the future. Hum. Resour. Dev. Rev. 15, 404–428.
Truby, J. (2020). Governing artificial intelligence to benefit the UN sustainable development goals. Sustain. Dev. 28, 946–959. doi: 10.1002/sd.2048
Tsamados, A., Aggarwal, N., Cowls, J., Morley, J., Roberts, H., Taddeo, M., et al. (2021). The ethics of algorithms: key problems and solutions. AI & Society. doi: 10.1007/s00146-021-01154-8
Ulnicane, I., Eke, D. O., Knight, W., Ogoh, G., and Stahl, B. C. (2021). Good governance as a response to discontents? Déjà vu, or lessons for AI from other emerging technologies. Interdiscip. Sci. Rev. 46, 71–93. doi: 10.1080/03080188.2020.1840220
Ulnicane, I., Knight, W., Leach, T., Stahl, B. C., and Wanjiku, W. G. (2020). Framing governance for a contested emerging technology: insights from AI policy. Policy Soc. 40, 158–177. doi: 10.1080/14494035.2020.1855800
Vinuesa, R., Azizpour, H., Leite, I., Balaam, M., Dignum, V., Domisch, S., et al. (2020). The role of artificial intelligence in achieving the sustainable development goals. Natural Commun. 11. doi: 10.1038/s41467-019-14108-y
Wallach, W., and Marchant, G. E. (2018). An Agile Ethical/Legal Model for the International and National Governance of AI and Robotics. Association for the Advancement of Artificial Intelligence.
Wamba, S. F., Bawack, E. R., Guthrie, C., Queiroz, M. M., and Carillo, K. D. A. (2021). Are we preparing for a good AI society? A bibliometric review and research agenda. Technol. Forecast. Soc. Change. 164, 120482. doi: 10.1016/j.techfore.2020.120482
Winfield, A. F., and Jirotka, M. (2018). Ethical governance is essential to building trust in robotics and artificial intelligence systems. Philos. Trans. Royal Soc. 376, 20180085. /10.1098/rsta.2018.0085
Wirtz, B. W., Weyerer, J. C., and Sturm, B. J. (2020). The dark sides of artificial intelligence: An integrated AI governance framework for public administration. Int. J. Public Adm. 43, 818–829. doi: 10.1080/01900692.2020.1749851
Yeung, K., Howes, A., and Pogrebna, G. (2019). “AI governance by human rights-centred design, deliberation and oversight: An end to ethics sashing,” in The Oxford Handbook of AI Ethics, ed. Dubber, M., and Pasquale, F., New York: Oxford University Press.
Zuboff, S. (2019). The Age of Surveillance Capitalism: The Fight for a Human Future at the New Frontier of Power. New York: Public Affairs.
Keywords: artificial intelligence, governance, ethics, responsibility, collaboration, AI for the common good
Citation: Sigfrids A, Nieminen M, Leikas J and Pikkuaho P (2022) How Should Public Administrations Foster the Ethical Development and Use of Artificial Intelligence? A Review of Proposals for Developing Governance of AI. Front. Hum. Dyn. 4:858108. doi: 10.3389/fhumd.2022.858108
Received: 19 January 2022; Accepted: 26 April 2022;
Published: 19 May 2022.
Edited by:
David Duenas-Cid, Kozminski University, PolandReviewed by:
Stefano Calzati, Delft University of Technology, NetherlandsColin Van Noordt, Tallinn University of Technology, Estonia
Copyright © 2022 Sigfrids, Nieminen, Leikas and Pikkuaho. This is an open-access article distributed under the terms of the Creative Commons Attribution License (CC BY). The use, distribution or reproduction in other forums is permitted, provided the original author(s) and the copyright owner(s) are credited and that the original publication in this journal is cited, in accordance with accepted academic practice. No use, distribution or reproduction is permitted which does not comply with these terms.
*Correspondence: Anton Sigfrids, YW50b24uc2lnZnJpZHMmI3gwMDA0MDt2dHQuZmk=