- 1School of Economics, Xiamen Univerisity, Xiamen, China
- 2School of Finance, Guangdong University of Foreign Studies, Guangzhou, China
- 3Southern China Institute of Fortune Management Research, Guangzhou, China
This paper investigates whether domestic migration of households in urban China has any impact on their risks of being defrauded. By utilizing the China Household Finance Survey (CHFS) in 2015, we find that domestic migration is significantly positively related to households’ risk of being defrauded. After controlling the corresponding individual and household characteristics, the result indicates that the probability of being defrauded with any losses and the amount of money being defrauded of migrant households in urban areas increased significantly by 0.98 and 7.43% compared to local urban households, respectively. This positive relationship between the risk of being defrauded and migration status of households is mainly concentrated in eastern China and highly educated households. Besides, the baseline results remain robust when we perform robustness checks, including the IV estimation which tackles the potential endogeneity of the migration status of the household head. Furthermore, we find that migrant households with fewer local social networks or less satisfaction of the current level of social security tend to be defrauded more intensively. The findings call for reflections on policies of domestic migration and urbanization and their unintentional consequences of social integration and security.
1 Introduction
In the criminal law of China, fraud refers to the act of defrauding public and private properties by making up facts or concealing the truth for the purpose of illegal possession. Fraud crimes have increased drastically from the early 2010s onwards in China1. This has caused significant losses to investors involved and seriously affected the stability of the financial system and society as a whole2. In 2018, the State Council’s general inspector launched a comprehensive campaign to crack down on illegal and criminal activities such as financial fraud. In 2021, it further issued another notice on preventing and handling fraud activities in illegal fundraising, which aims at strengthening the overall coordination of financial supervision to protect ordinary investors. Thus, research on fraud related issues has important policy implications in China.
Meanwhile, with the continuous advancement of urbanization and industrialization in China, immigrant labor has played a crucial rule during this process. Previous research mainly focuses on social integration problems of immigrant labor in urban areas such as their economic status, social security, and residential rights as compared to local Hukou registered residents (Alba and Nec, 2003; Chen and Deng, 2019; Yin et al., 2020). In addition, some have investigated the contribution of domestic migration on the effectiveness of the allocation of resources and economic growth (Taylor and Williamson, 1997; Batista et al., 2019; Gu and Wan, 2020). Meanwhile, the unprecedented flow of domestic migration has brought pressure to the local government and the public, and many places regard migrants as a social management problem. In particular, as fraud crimes have increased significantly since the 2010s, it is important to investigate whether migration status has any impact on the risk of households being defrauded in urban areas. According to the Report of China Household Finance Survey in 2015, 56.69% of the residents suffered from telephone fraud, SMS fraud, online fraud (QQ, WeChat, Fetion, phishing websites, etc.), and face-to-face fraud, and among those 6.29% suffered economic losses. Apparently, households are more likely to encounter fraud than to suffer losses due to fraud, indicating that Chinese households have a certain sense of financial protection (Gan et al., 2014). At the same time, the relationship between migration and crime has also been widely discussed (Schemer, 2012; Zatz and Smith, 2012). What is the effect of domestic migration on the risk of households being defrauded in urban China? This paper utilizes the China Household Finance Survey (CHFS) in 2015 to answer this question.
A large number of studies have investigated the crime rate of migration from the perspective of offenders (Becker, 1968; Martens, 1997; Lee et al., 2001; Eckert, 2002; Chen et al., 2009). However, migrants are very often victims of crimes as well. Specifically, migrants are generally at the bottom of society, exposed to the complex environment, which makes them vulnerable to crime offenses. They have become the “absolute victim” of the double attacks from a small number of floating and permanent population perpetrators (Brian and Stephen, 2011; Tong, 2013; Luca, 2015; Belli et al., 2019). This paper adds to the literature in that we use micro household survey data to empirically test the effect of domestic migration on the risk of households being defrauded in urban China, and to investigate the potential causes why the migrants are cheated and victimized in fraud crimes. We find that domestic migration status has a significantly positive impact on the risk of households being defrauded in urban China. In particular, this positive effect mainly comes from households that reside in eastern China and with a highly educated household head. Furthermore, we find that migrant households with less local social networks or less satisfaction of the current level of social security tend to be defrauded more intensively. Our result is consistent with the explanation that, because domestic migrants are often excluded from the local social safety net and enjoy fewer social security protections compared with the local residents, and have less social communication with local people3, this makes them more vulnerable to be illegally infringed and increase their risk of being defrauded. The remainder of this paper is organized as follows: Section 2 presents the data. Section 3 presents the model and the estimation results. Section 4 concludes.
2 Data
The data used in this paper are the 2015 wave of China Household Finance survey (CHFS), which was carried out nationwide by Survey and Research Center for China Household Finance in Southwest University of Finance and Economics. The sample covers 29 provinces (autonomous regions and municipalities directly under the central government), 351 counties (districts and county-level cities), and 1,396 village communities. The survey questionnaires include household demographics, assets and liabilities, insurance and security, expenditure and income, and subjective attitudes of family members. The CHFS also provided detailed information on whether the household suffered losses due to being defrauded, and the hukou status in the part of household basic information.
In this paper, domestic migrants (migrant) in urban China are defined as a group of residents that consist of two sub groups: non-local registered residents and local rural registered residents living in urban areas. It takes the value of 1 if an urban resident belongs to one of the above two sub groups, and 0 otherwise. The key dependent variable is the risk of households being defrauded, which corresponds to answers to the questions “Whether you or other family members have suffered losses due to being defrauded?” and “How much is the economic loss due to being defrauded?” in the questionnaire of the 2015 wave of the CHFS data (Gan et al., 2014)4. We measure the probability of the risk of households being defrauded by evaluating whether or not a household has suffered losses due to being defrauded (loss), while the intensity of being defrauded is measured by the logarithm of loss due to being defrauded (ln_loss). After excluding the observations with missing values, we come up with 24,336 observations across 158 cities and 29 provinces.
The descriptive statistics of the variables are shown in Table 1. It shows that the proportion of domestic migrants in the whole sample is 10.04%.5 Meanwhile, roughly 4% of the urban households have suffered losses due to being defrauded, and the maximum economic losses could go as high as 2,000,000 yuan.
Before we enter into empirical investigation, we graph the average probability of being defrauded and the average amount of being defrauded for local urban residents and migrants in Figure 1. It could be observed that both numbers are higher for migrants than for local residents in urban China.
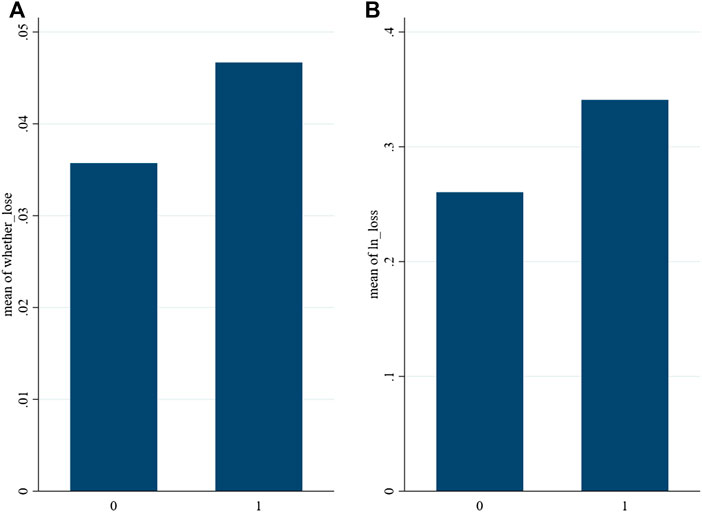
FIGURE 1. The average of the probability of being defrauded (A) and the average log amount of being defrauded (B) for urban residents (Migrant = 0) and migrants (Migrant = 1).
3 Model Specification and Estimation Results
3.1 The Model and the Baseline Results
We employ the following empirical specification to estimate the effect of domestic migrant on the risk of households being defrauded:
where risk_defraudedi represents the risk of household i being defrauded. It refers to either lossi or ln_lossi. Lossi indicates whether or not household i has been defrauded and suffered any losses. Ln_lossi is the logarithm of loss due to being defrauded.
Migranti represents the migration status of household i, which equals one if households are non-local registered residents or local rural registered residents living in urban areas. The parameter
Table 2 presents the baseline results. From Columns (1) to (3) the dependent variable is loss, while from Columns (4) to (6) it is ln_loss. In Columns (1) and (4), only migrant plus the provincial dummies which control for the unobservable effects originating from where the households live are included. In Columns (2) and (5), the individual characteristic control variables are added. In Columns (3) and (6), household characteristic variables are further added. The estimated
For the control variables, we observe that from Column (6), ln_asset has a significantly positive impact on ln_loss, indicating that households with higher assets suffer more losses from being defrauded. The estimated parameters of ln_loan and pro_plloan are also significantly positive at the 1% level, showing that households with higher loans or more involved in an informal financial market borrowing and lending suffer more losses from being defrauded. For the social security and insurance variables, households with a housing provident fund suffer less losses due to being defrauded, as the estimated parameter associated with hous_fud is significantly negative at the 1% level. Meanwhile, Columns (3) and (6) show that an increase of financial literacy measured by training in finance (tra_fin) increases the risk of households being defrauded. This result is very interesting. One possible explanation could be that perhaps people with training in finance are more open to new forms of finance, such as internet finance. But development of internet finance is also positively correlated with fraud crimes such as illegal fundraising (Lai, et al., 2021b), which may lead those with training in finance to suffer more losses. In addition, age has a U-shaped effect on the risk of households being defrauded, with young households and elder households having higher risk of being defrauded compared to those middle-aged households. Besides, married couples have lower risk of being defrauded compared to single households, while poorer self-evaluated health status has a positive impact on the risk of being defrauded. Other control variables do not have a significant effect on the dependent variable.
3.2 Heterogeneity Test
In this section, we perform the heterogeneity test of the effect of domestic migration on households’ risk of being defrauded.
Columns (1) to (4) of Table 3 display the estimation results when households are grouped to subsamples according to the years of education (eduy) of household head. Columns (1) and (3) show the results when eduy of household head is high school or below, while Columns (2) and (4) show the results when eduy is above high school. The estimated coefficient associated with domestic migration is positive and significant for those of above high school education. This result is very interesting. This indicates that migrants that are highly educated are more vulnerable toward being defrauded compared to local residents who are also highly educated. This is probably due to two reasons. First, highly educated people could more easily obtain credit from the formal financial market, such as from banks. As such, if they are being defrauded, they may suffer more losses because of their easier access to credits compared to other households. Second, highly educated migrants may not have an extended social network in the place of residence compared with highly educated local residents, which makes them more vulnerable when they encounter risky events such as frauds. As such, they may be more likely to suffer from fraud events.
In Table 4, we group the 29 provinces in CHFS to three regions: East, Middle, and West. Households are grouped by the region of their residing area. The estimated coefficient associated with migrant in Columns (1) and (4) is positive and significant for the eastern regions, while it is positive but insignificant for the west. However, it becomes slightly negative but insignificant for the middle. This result shows that the vulnerability of migrants in terms of being defrauded is more severe in the east part of China, where economic development is more advanced compared to the other two regions. This may be due to two reasons. First, fraud activities have cost, and those originators may find it more profitable when conducting fraud activities in places where people are more densely populated, such as the east coastal region, which leads to economy of scale for fraud activities. Second, people in the eastern region have a significantly higher per capita income compared to those in other regions; thus, fraud originators may potentially obtain more money from fraud activities in the eastern region compared to other regions.
3.3 Robustness Analysis
We specify the following model to perform the robustness check of the baseline:
where
The estimation results are displayed in Table 5. Column (1) displays the results of estimated coefficients, and Column (2) shows the marginal effect of the Probit Model. The estimated coefficients associated with migrant are significantly positive at the 5% level, which is consistent with the results in Table 2. In addition, the magnitude of the marginal effects of Column (2) is also very close to that of Column (3) of the baseline in Table 2.
In addition, it is possible that there exists reversed causality between the risk of being defrauded and the decision of migration. The migration decision of a migrant may be caused by being defrauded at the home city. In order to address this problem, we first postulate that when people change place of residence, usually they also change jobs. As such, by looking at how long they have worked in their current employment, we can filter out samples that have worked in their current job for less than 1 year, during which they report whether or not they were being defrauded. This can help us deal with the potential endogeneity of migration decisions to some extent. CHFS provided a relevant question that asks, “From which year did you start to do the current job?” A total of 701 respondents replied that they started the current job less than 1 year before. As such, we excluded them and re-estimated the baseline regressions. The estimation results are presented in Table 6. It shows that the estimated coefficients associated with migrant status remain positive in both Columns (1) and (2), while it is significant at the 5% level in Column (2). This shows that migrant status has a significantly positive effect on the amount of losses that households suffer from being defrauded, which is consistent with the baseline results in Table 2.
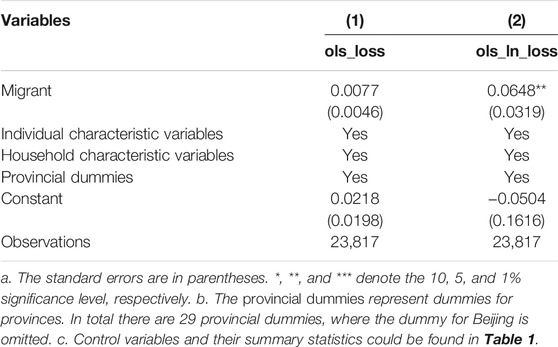
TABLE 6. The robustness check of risk of households being defrauded on migrant status (excluding samples that started their current works in less than 1 year).
3.4 Instrumental Variable Estimation
In the previous section, we have excluded the samples that migrated during the same year as they were being defrauded to tackle the potential endogeneity problem. However, a more systematic way is to use the instrumental variable estimation. As such, we employ an instrumental variable for the variable migrant, which is the local housing price that the household resides in. Local housing price is directly correlated with the number of local migrants, as more migrants lead to higher demand for housing and an increase in housing price. However, local housing price does not have a direct impact on whether a local household is being defrauded. The estimation results are presented in Table 7.
The first column shows that local housing price is significantly correlated with migrant status of household head. Columns (2) and (3) show that the IV regressions yield.
3.5 Impact Channel Analysis
The main difference between domestic migrants and local residents in the urban areas is that the former group has less access to social security, social benefits, and other public resources, almost all of which are operated by the local city governments (Yang, 2012). Table 1 shows that with the household level CHFS data, the average value of pension and medical insurance in urban China is 80.23 and 91.78% respectively, while that of unemployment insurance and housing fund are less than 20%. It is difficult for domestic migrants in urban areas to enjoy the same social welfare as the local residents in terms of housing fund, unemployment insurance, and so on, even if the migrant has worked as and made as great contributions to urban area development as local residents (Deng and Hu, 2007; Du et al., 2005). Here we want to test if this lack of social security or the subjective view of migrants towards these unequal treatments may lead to the greater risk of migrant households being defrauded. Therefore, the satisfaction of the current social security level (ss_satis) and the social or commercial insurance (insur) are considered as additional possible factors affecting the risk of households being defrauded.
At the same time, research has shown that the living conditions of migrants, such as renting or sharing houses, could increase their chances of victimization (Zhang, 2012). The theory of crime mode shows that the weakening of crime defense caused by the weakening of rental mode6 and family structure7 further aggravate the imbalance of the power balance between predators and defenders, and increase the incidence of crime high (Cheng et al., 2016). Therefore, it is assumed that one of the influencing factors of the increase of victimization caused by migration lies in the spatial weakness, namely the reduction of social scope (net_social), as they usually have smaller number of close contacts, such as relatives compared to local residents. Besides, their relatively poor living conditions and lack of security, especially the rental mode (rent_house), may make them more vulnerable to crimes. As such, we specify the following model:
where net_sociali indicates the level of the number of relatives with blood lineage of household i’s living in the same county or city, ss_satisi indicates household i’s satisfaction of the current social security level, and oth_channelsi includes insuri, rent_housei. We test whether these are potential channels through which migrant status exerts its positive effect on households’ risk of being defrauded.
The estimation results are displayed in Table 8, which shows that the estimated parameter of the interactive terms migranti ×net_sociali and migranti ×ss_satisi are significantly negative at the 5 and 10% level in Columns (1) and (2), respectively, whereas the estimated parameter associated with net_social is positively insignificant while that with ss_satis is negatively significant at the 10% level. This indicates that when domestic migration increases in urban China, households that have wider social networks in the local area or are satisfied with the current social security level tend to have lower risk of being defrauded when compared to migrants with less extensive social networks and lower satisfaction with the current social security level. Meanwhile, the estimated parameter of the migrant is still significantly positive at the 5% level in Column (1), but it becomes insignificant in Column (2). In addition, the estimated parameter of ss_satis and its interactive term with migrant has a significantly negative impact on the risk of households being defrauded when we add other channels such as insur and rent_house, while the estimated coefficient in front of migrant is no longer significant. This shows that the effect of domestic migration on the risk of households being defrauded is mainly through the channel of the satisfaction of the current social security level, as shown in Column (3). However, when it comes to the loss due to being defrauded, the above results do not exist.
4 Conclusion
This paper investigates the effect of domestic migration on the risk of households being defrauded. We employ the 2015 wave of China Household Finance Survey data and find that domestic migration has a positive effect on the risk of households being defrauded. In addition, this positive effect mainly comes from households that live in eastern China or are highly educated. Besides, the baseline results remain robust when we perform robustness checks, including the IV estimation which tackles the potential endogeneity of the migration status of the household head. Further tests on the impact channels show that the satisfaction of current social security level is the primary channel through which domestic migration exerts its positive impact on the risk of households being defrauded. Our result provides important policy implications on urban social security provided by city governments. In particular, social security of migrants should be provided in an equal way as to local residents, and the government should pay attention to its potential effect on strengthening migrants against being defrauded.
Data Availability Statement
Publicly available datasets were analyzed in this study. This data can be found here: https://chfs.swufe.edu.cn/.
Author Contributions
MY wrote the first draft and verified the data. NL cleaned up the data and performed the empirical estimation. JL revised the draft, checked the empirical procedure, and prepared the manuscript for submission.
Conflict of Interest
The authors declare that the research was conducted in the absence of any commercial or financial relationships that could be construed as a potential conflict of interest.
Publisher’s Note
All claims expressed in this article are solely those of the authors and do not necessarily represent those of their affiliated organizations, or those of the publisher, the editors, and the reviewers. Any product that may be evaluated in this article, or claim that may be made by its manufacturer, is not guaranteed or endorsed by the publisher.
Footnotes
1Lai et al. (2021a) and Lai et al., (2021b) shows that one category of fraud crimes, the illegal fundraising, has increased from 195 criminal court cases in 2013–4,545 cases in 2019.
2China Judicial Big Data Research Institute shows that the number of cases of the national telecom network fraud exhibited an upward trend from 2015 to 2017, with a year-on-year increase of 51.47 and 70.34% in 2016 and 2017, respectively. And the data show that telecom fraud is still the main way of fraud, but network fraud has increased significantly. The 2019 Research Report on the trend of online fraud shows that financial fraud is the type of fraud with the highest number of reports, and the victims of financial fraud suffer high losses.
3Contacts are generally limited to relatives, villagers, and other acquaintances, and rarely communicate with other outsiders, which refers to Peng, D., 2020, Involution and Inverse Involution: Differences in the Direction of Intergenerational Changes in the Social Communication of the Migrant Population, Journal of Shenzhen University (Humanities and Social Sciences),38(05),112–123.
4The definition of household members in CHFS is those who should be able to jointly determine household income and expenditure (see CHFS 2015 Questionnaire), among whom some may live together with the person surveyed, some may not. But domestic helpers and drivers employed are not included. In addition, adult children are not counted as household members from the perspective parents. As such, the parents are not considered household members of migrant children, and vice versa.
5This number is consistent with data from other nationally representative survey such as China Labor Dynamic Survey, where the proportion of migrants of urban total population is around 10% as well (Lai, Ye and Zhang, 2020).
6The weakening of residential defense, especially the rental mode, is reflected in the lack of physical and symbolic protection facilities.
7The weakening of family structure includes the small number of family members, living habits far away from the family, and so on.
References
Alba, R., and Nee, V. (2003). Remaking the American Mainstream: Assimilation and Contemporary Immigration. Cambridge: Harvard University Press.
Batista, C., Seither, J., and Vicente, P. C. (2019). Do migrant Social Networks Shape Political Attitudes and Behavior at home? World Develop. 117, 328–343. doi:10.1016/j.worlddev.2019.01.019
Becker, G. S. (1968). Crime and Punishment: An Economic Approach. J. Polit. Economy 76 (02), 169–217. doi:10.1086/259394
Belli, R., Freilich, J. D., and Newman, G. (2019). “Migration, Crime, and Victimization,” in Migration, Crime, and Victimization, International And Transnational Crime And Justice. Editor M. Natarajan (Cambridge: Cambridge University Press), 240–244. doi:10.1017/9781108597296.040
Brian, B., and Stephen, M. (2011). The Impact of Migration on Crime and Victimization, A Report for the Migration Advisory Committee. LSE: Centre for Economic Performance.
Chen, G., Li, S., and Chen, Y. (2009). Population Mobility and Crime: An Empirical Analysis Based on China’s Observation. Chin. J. Popul. Sci. 04, 52–61+111112.
Chen, Y., and Deng, Z. (2019). Liquidity Constraint Shock, Job Search and Post Match Quality-Evidence from Rural-To-Urban Migrants in China. J. Labor Res. 40, 332–355. doi:10.1007/s12122-018-9278-7
Cheng, J., liu, J., and Wang, J. (2016). Domestic Immigration, Residential Status and Crime Rates. Sociological Stud. 03, 218–241+246.
Deng, D., and Hu, H. (2007). Mobility, Deprivation, Exclusion and Integration: Social Integration and the Acquirement of Security Right. Chin. J. Popul. Sci. 06, 14–22.
Du, P., Ding, Z., Li, B., and Zhou, F. (2005). Work, Security and Assimilation of Migrants in Beijing. Popul. Res. 04, 53–61.
Eckert, R. (2002). Hostility and Violence against Immigrants in Germany since 1992Migration, Cult. Conflict Crime
Gan, L., Yin, Z., and Tan, J. (2014). Report on the Development of Household Finance in Rural China. Springer.
Gu, C., and Wan, X. (2020). Research on the Transmission Mechanism of Population Migration to Economic Development: Based on the Threshold Effect Model. Northwest Popul. J. 41 (04), 58–71.
Lai, J. T., Ye, M., and Zhang, H. (2020). Home Ownership and Life Satisfaction of Migrants in Urban China. Appl. Econ. Lett. 28 (4), 287–293. doi:10.1080/13504851.2020.1752359
Lai, J., Xie, J., and Cao, S. (2021b). Digital Financial Inclusion and Illegal Fundraising, Working Paper. Guangdong: School of Finance, Guangdong University of Foreign Studies.
Lai, J., Xie, J., Ye, M., and Yi, X. (2021a). Illegal Fundraising and Financial Availability: Evidence from Court Cases Data in China, Working Paper. Guangdong: School of Finance, Guangdong University of Foreign Studies.
Lee, M. T., Martinez, R., and Rosenfeld, R. (2001). Does Immigration Increase Homicide? Negative Evidence from Three Border Cities. Sociol. Q. 42 (04), 559–580. doi:10.1111/j.1533-8525.2001.tb01780.x
Liang, P. H., and Jiang, H. Z. (2020). Financial Availability and Risk Prevention of Internet Finance: An Empirical Analysis Based on Online Pyramid Selling Cases. China Ind. Econ. (04), 116–134.
Luca, N. (2015). Immigration and Crime: Evidence from Victimization Data. J. Popul. Econ. 28, 697–736.
Martens, P. L. (1997). Immigrants, Crime, and Criminal justice in Sweden, Ethnicity, Crime, and Immigration: Comparative and Cross-National Perspectives. Chicago: University of Chicago Press.
Schemer, C. (2012). The Influence of News media on Stereotypic Attitudes toward Immigrants in a Political Campaign. J. Commun. 62 (05). doi:10.1111/j.1460-2466.2012.01672.x
Taylor, A. M., and Williamson, J. G. (1997). Convergence in the Age of Mass Migration. Eur. Rev. Econ. Hist. 1, 27–63. doi:10.1017/s1361491697000038
Tong, M. (2013). Criminal Victims and Victim Prevention of Floating Population. Chin. Criminology Rev. 03, 48–54.
Yang, J. (2012). Urban-rural divide and Inside-Outside Disparity: Unequal Access of Migrants to Social Securities in China. Popul. Res. 35 (05), 8–25.
Yin, Z., Liu, T., and Zhang, C. (2020). The Impact of Rural Labor Mobility on Household Savings Rate. China Ind. economy 382 (01), 26–44.
Zatz, M. S., and Smith, H. (2012). Immigration, Crime, and Victimization: Rhetoric and Reality. Annual Review of Law and Social Science 8. doi:10.1146/annurev-lawsocsci-102811-173923
Keywords: migrant, risk of being defrauded, social security, social exclusion, China
Citation: Ye M, Lian N and Lai J (2022) Domestic Migration and the Risk of Households Being Defrauded in Urban China. Front. Hum. Dyn 3:781139. doi: 10.3389/fhumd.2021.781139
Received: 22 September 2021; Accepted: 13 December 2021;
Published: 03 February 2022.
Edited by:
Mingming Jiang, Shandong University, ChinaReviewed by:
Danqing Shen, Shandong University, ChinaDong Zhou, Shanghai Jiao Tong University, China
Copyright © 2022 Ye, Lian and Lai. This is an open-access article distributed under the terms of the Creative Commons Attribution License (CC BY). The use, distribution or reproduction in other forums is permitted, provided the original author(s) and the copyright owner(s) are credited and that the original publication in this journal is cited, in accordance with accepted academic practice. No use, distribution or reproduction is permitted which does not comply with these terms.
*Correspondence: Jennifer Lai, ZWNvbmxhaUBnbWFpbC5jb20=