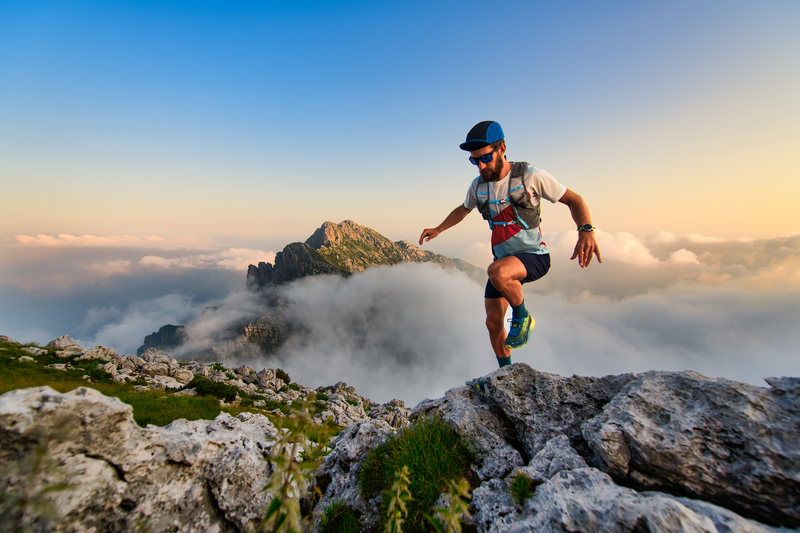
95% of researchers rate our articles as excellent or good
Learn more about the work of our research integrity team to safeguard the quality of each article we publish.
Find out more
PERSPECTIVE article
Front. Hum. Dyn. , 23 June 2021
Sec. Digital Impacts
Volume 3 - 2021 | https://doi.org/10.3389/fhumd.2021.626299
This article is part of the Research Topic Hybrid Collective Intelligence View all 5 articles
Discussions around Covid-19 apps and models demonstrated that primary challenges for AI and data science focused on governance and ethics. Personal information was involved in building data sets. It was unclear how this information could be utilized in large scale models to provide predictions and insights while observing privacy requirements. Most people expected a lot from technology but were unwilling to sacrifice part of their privacy for building it. Conversely, regulators and policy makers require AI and data science practitioners to ensure optimal public health, national security while avoiding these privacy-related struggles. Their choices vary largely from country to country and are driven more by cultural aspects, and less by machine learning capabilities. The question is whether current ways to design technology and work with data sets are sustainable and lead to a good outcome for individuals and their communities. At the same time Covid-19 made it obvious that economies and societies cannot succeed without far-reaching digital policies, touching every aspect of how we provide and receive education, live, and work. Most regions, businesses and individuals struggled to benefit from competitive capabilities modern data technologies could bring. This opinion paper suggests how Germany and Europe can rethink their digital policy while recognizing the value of data, introducing Data IDs for consumers and businesses, committing to support innovation in decentralized data technologies, introducing concepts of Data Trusts and compulsory education around data starting from the early school age. Besides, it discusses advantages of data-tokens to shape a new ecosystem for decentralized data exchange. Furthermore, it emphasizes the necessity to develop and promote technologies to work with small data sets and handle data in compliance with privacy regulations, keeping in mind costs for the environment while bidding on big data and large-scale machine learning models. Finally, innovation as an integral part of any data scientist's job will be called for.
At the beginning of the millennium, the 10 largest German DAX companies—Telekom, Allianz, Siemens, Daimler, SAP etc.—were each significantly more valuable than the largest global digital companies Amazon, Google, Tencent, or Facebook. Almost 20 years later, the relationship has been turned upside down: Alphabet alone is now worth more than the largest DAX companies put together1.
Data is an energy source for the platform economy, with the largest players based on the US West or the Asian East Coasts. The Platform Index2 (operated by Hamidreza Hosseini) shows the performance of 15 platform operators (from Alibaba, Etsy, from Netflix to Weibo) compared to Dow Jones, Nasdaq Composite, and DAX-30 since 2016. This shows how big data players progressed, or were even enriched by aspects of the COVID19 downturn, while the Dax is barely back to its—already more or less stagnant−2017 level. Since the disastrous demise of Wirecard, the causes of which will presumably be dealt with by the judiciary for years to come (and no doubt in the media and politics), SAP is now the only DAX company putting Germany on an international digital map.
Although the DAX newcomer Delivery Hero tries to claim a place in the platform league, hope has so far been stronger than balance sheets. In the 10 years of its existence, the company has not yet been able to report a single profit.
Looking at the digital economy throughout Europe, things are hardly getting any better. Even the success of Spotify and Adyen—which broke through $50 billion market cap barrier—cannot hide the fact that the US and Chinese companies dominate the global data market. European Venture capital investments make only one quarter of the US. Germany, the fourth largest economy in the world, and Europe as a whole are not succeeding in truly getting value out of data. Nevertheless, 500 million people in Europe—in a large ecosystem of businesses, academic institutions, communities, and private consumers—permanently feed the engines of Alphabet, Amazon, and Facebook. In the new Cold War of hot data, which is becoming more and more prevalent between China and the USA, Europe supplies at most the cannon fodder. Europe is not sitting at the negotiating table. It is an item on the menu.
Instead of developing their own ideas for how businesses in Europe might contribute to sustainable data economies, policy makers and corporate CEOs regret not having established a European data value proposition that allows the continent to be an equal player in the global datasphere. One points to the great market segmentation and language diversity, which—coupled with strict regulation of privacy—has counteracted the emergence and spread of European IT giants.
The once so proud continent finds no way out of the impasse of digital marginalization. Where is the exit from this (self-inflicted) digital immaturity?
Is there any hope for a new European renaissance to regain digital relevance? Interest in data and digitization is growing on the continent, party facilitated by global pandemics. The development of Covid apps for Germany, France, or Portugal alone will however not guarantee a sustainable and positive future. This innovation may have been a single effort, but it has already brought certain questions and aspects to the table, to which common answers need to be found:
What does a web look like that contributes to solutions to the problems of our society, including pandemic? How do we cope with legacy IT to solve modern, complex problems? How do we make data accessible in a secure and democratic way? How does a trustworthy Internet work, from which large and small businesses, schools, communities, and every single citizen can profit sustainably and fairly? Can individuals benefit from data which they create? Do we have instruments in place for use of beneficial data technologies?
Sustainable data economy can be practically achieved while simultaneously working on 10 areas across different fields—law and policy, technology, education, and communication to invite wide groups of society into the discussion around digital agenda.
#1: Data value
Data is valuable and absolutely worth protecting. They are the property of the (private or legal) person who creates them.
Data is often referred to as a commodity. To determine their value today is an art. This value changes over time and depends on who controls it. What is data dust and noise for one person can be digital gold for another. Data often begets data (e.g., metadata, synthetic data). A discussion about the value of data is therefore as complex and frustrating as a debate about the value of a work of art.
Much more important, however, are questions of ownership and derived value. Who owns data? Discussions about the regulation of “private property” in the sixteenth and seventeenth centuries were followed by an expansion of the concept of ownership beyond “object ownership” to include “intellectual property” as early as the eighteenth century. Since then, copyright laws have regulated the extent to which the creator also remains the owner of the products of his intellectual work. According to German law, copyright is inalienable, only the rights of use and reproduction can be traded. We urgently need to regulate similar issues with regard to data if we want to maintain long-term trust in digital life and work.
Data—like private assets and intellectual creations—must belong to the people, companies, and organizations that create them.
Certainly, no single contemporary Internet platform giant will formulate such a premise on its own initiative; after all, the business models of all web giants are based in large part on the assumption of this structured legal vacuum. The current fabulous business margins of Facebook, Tencent & Co are only possible because the data creation itself does not cost these companies anything. Of course, the data curation, infrastructure, i.e., data processing and the generation of knowledge, involves considerable expenditure. But the more data is available for the monetizable products, the greater is the benefit, the economies of scale.
The previous legal provisions are solely aimed at individual data protection, in the sense of using the data for purposes other than the actual purpose of the company. This does not do justice to the value of the data itself.
Admittedly, the corporations argue that the individual data is worthless for the people themselves, which is why in practice they accept the terms and conditions with the greatest of ease—regardless of how long the terms and conditions or how detailed the data protection regulations are. But by doing so, they do not consciously accept a waiver of value or a claim to ownership. Just as a sketch by Picasso is far from worthless just because he threw it crumpled up in the trash, and just as I cannot pass off and sell a composition by Fanny Mendelssohn as mine, just because the composer herself never performed it- neither should corporations be allowed to use my posts or other “discarded” data, no matter what for, that I have posted on Twitter, Facebook, or Tiktok, nor should they use the meta-data that I leave unsuspectingly as a data trail, just because I have my cell phone in my pocket and might unintentionally create data simply by being connected to an app or piece of technology.
In the Renaissance we learned that the value of human work is not only created by the sweat of his brow in the field or in the workshop, but that we can also add value at our desk, at the piano or in the studio. Today we must understand that value creation can be created solely through our being and interacting with our digital environment. To do this, we need a legal system that finally extends the concept of ownership to the digital world and technologies that make it easier for us to be in control of our own data.
#2: Digital property rights
Data ownership is identified by an individual data passport/data ID and is linked to a sovereign power of disposal and use.
We live in a world that can be duplicated many times over: every person has a digital shadow. As early as 1991, the US-American computer scientist David Gelernter spoke of “mirror worlds,” a new dimension of human life based on and driven by data—in his optimistic vision of a world of freedom and equality. Today, some argue that Google and Facebook know us better than our own parents because of our search and communication behavior. They may claim to know which color makes us happy, which party we vote for, what we will eat next month, what disease might get us in 2 years, and which university will give our children a chance. Top athletes communicate with their fans via chat bots or simulate their performance under various conditions and draw conclusions for their training programs. At the same time, companies are building gigantic virtual walls around their databases because without appropriate cyber security programs, they run the risk of being robbed of their entire business substance or finding themselves in complex legal situations due to data breach, lack of compliance, or other risks.
Data plays a large part in influencing our present and even has impact on what our future might like. Anyone who creates data—whether private individuals, companies, communities, or foundations—should be able to maintain sustainable control over this data because of the significant impact on the individuals basic rights, prosperity, and other aspects that are normally protected by law. The general terms and conditions or the prohibition of social networks does not give back this control.
The expectation that every single person individually spends the necessary time and effort to control their own digital shadow is unrealistic. To make the process more tractable, People, companies, and organizations must have proof of their digital identity. These data IDs could function like identity cards or commercial register entries and make (all) data transactions identifiable and traceable.
Traditional methods of identity authentication are completely obsolete in the digital world. Currently, identity documents such as passports and driver's licenses are usually issued in physical form and stored digitally in centralized databases. This practice restricts user control and causes security challenges due to considerations of privacy and regulatory compliance. The high number of reported data breaches associated with centralized identity models shows that it is no longer a question of whether an account or database is compromised, but when. We urgently need a decentralized identity model that addresses such security problems while giving users flexible control over the use of their personal data and fast access to the products and services they want.
Today many people in Europe carry an organ donation card with them. Organ donations save lives. There may be a digital equivalent that could be helpful in times of crisis. Many scientists are convinced that in pandemics the most precise data can possibly save lives. It could make sense for people, organizations and companies to donate their data for scientific purposes in times of crisis or by personal choice, either as a complete package in the event of their death or as a monthly data donation in a kind of “standing order.” The handling of such a donation should be as simple as possible. A data ID could, for example, bear a corresponding note stating whether people want to pass on their digital lives and under what conditions this should happen.
Over the next 5 years, developments in digital identity will profoundly change our lives and our economy. Europe and Germany are no worse positioned for these approaches than the USA. What is critical is to develop such models theoretically and test them in practice, but also to invest in them. Instruments and methods for the secure management of data identities could become a valuable product in the democratic world.
#3: Digital separation of powers and decentralized data markets
The states of the EU commit themselves to create decentralized web technologies to prevent any concentration of power in the data network. Decentralized data markets with clear ownership and access rules prevent asymmetries of power in traditional Web.
Precisely because data have a value—as yet unknown for the future—there is a great danger that a high concentration of data will lead to power asymmetries. With regard to intellectual property, affordability of payment channels or stability of access to data, it is contrary to the principles of data democratization that monopolies arise, either in private or public hands, which could become the gatekeepers of data of any kind. Existing literature on valuation of data assets refers to the fact, that individual-level data can be underpriced, as the market economy generate too much of it. Citizens tend to overshare their data, either by waving on their own privacy rights in favor of Big Tech or revealing and compromising information of individuals linked to them (Acemoglu et al., 2019). The implications are very concerning. Even today, the question arises whether some companies are not already in a position to undermine the sovereignty of individual states because of their power over data.
Data markets on decentralized architecture could be a practical response to invite more businesses, non-profits, communities, and individuals into the data economy. They connect buyers and sellers of datasets with one another. Innovation around such markets is key as machine learning and further data technologies continue to embed themselves at the heart of every industry and national economies. They can provide opportunities to innovate around Small Data, proprietary or alternative data sets that are germane to a particular problem or context, to fundamentally shift the distribution of power in current web and facilitate the broad adoption of benefits from data capabilities.
Successful implementation of decentralized data markets depends on the creation and support of new data sharing protocols and other technologies, but above all the willingness to create a new ecosystem—far more data-centric and democratic than the traditional models of American or Chinese companies like Alphabet, Facebook, or Tencent. Traditional businesses in logistics and transportation, healthcare, media and entertainment, consumer goods, and automotive would benefit from getting access to software and data talent while streamlining and reorganizing their data assets in a decentralized model.
The creation of a decentralized data exchange ecosystem won't happen overnight as multiple considerations must be addressed, e.g., seamless and correct storage and transfer of data, security, and ease-of use. Luckily, the advent of blockchain and other trust-based systems with associated smart contract platforms has already triggered significant research into the design of multi-agent systems designed to perform this important work. For example, prediction markets, decentralized token exchanges, curation markets, token curated registries, storage markets, and computational markets provide various examples of systems designed to perform useful work by coordinating and aligning individual pparticipants within an ecosystem. All this can serve as a foundation for decentralized data markets which would benefit businesses and communities of all sizes and varieties.
The token curated registry (TCR) in particular might provide a powerful abstraction for how different parties could work together to implement and benefit from a data market3.
Especially in times of global crisis such as Covid, such a data market could have been invaluable. The challenge of resolving the contradiction between epidemiologically necessary evaluation of mass data and the high sensitivity in handling health data became apparent in Covid apps that contains integrated data protection guarantees as a basic design principle.
Technologies for decentralized data markets and data processing that guarantees data protection show potential, but they are not widely known.
For example, a few years ago, researchers from the Estonian company Cybernetica4, with the help of Multiparty Computing (MPC), were able to determine correlations between graduation chances for working and non-working students without combining confidential data from two different ministries in a central database or even looking at it. This outcome proved that AI systems can be trained without huge data collection efforts and without violating data privacy restrictions even when the use case relates to personal outcomes.
The Ocean Protocol5, developed in Berlin, creates a kind of decentralized orchestration layer between the various participants in the ecosystem, whether data owners, data agents, software developers, data providers, or governance officers. The actors control their “togetherness” via blockchain smart contracts, which can document and evaluate all steps and remunerate these system's participants in tokens. This work provides a valuable example of how a value-based data infrastructure could be created with trust and permissions built into the ecosystem. The Ocean Marketplace currently offers the most convenient tool to value data assets of a for-profit or non-profit organization, or an individual data set.
DECODE6, a pilot project financed by the European Union over 3 years in Barcelona and Amsterdam, which tested a combination of decentralized technologies, was also forward-looking. The aim was for people to collect data, such as noise levels or air quality in their homes, and to decide for themselves which data to use for which purposes.
Oasis Labs7, a startup in San Francisco, has created something similar for health data. Here users can donate genetic information for research projects.
Decentralized web with a multitude of technologies still require a capable technologist like Marc Andreessen –Cofounder of Netscape and creator of the Mosaic Browser—to harmonize existing solutions with an individual consumer in mind, while offering easy-to-use interfaces to experiment with solutions currently attractive only to very technical people.
Last but not least, tokenization of data assets provides a practical approach to scale data valuation for businesses and non-profits (Voshmgir, 2020).
#4: Internet of the free
The states are committed to a common free digital ecosystem that belongs to no one.
Almost unnoticed by the public, we are heading for a kind of silent coup on the Internet, which comes with the harmless name “New IP” and sneaks through the technological back door into the power centers of the whole world. What is it all about? Well, even if there are companies that have built up a dangerous market dominance by the unchecked collection of data, the Internet itself is currently still a place of freedom. Today's Internet belongs to everyone and no one. Only by law can individual states prevent certain companies from offering certain tech services in their country, for example when nations block certain internet traffic in the context of pending military operations. There was no technical way to prevent this from happening.
But now the idea of an alternative network technology is emerging and growing that could put power back into the hands of nation-states instead of individual Internet corporations, companies from traditional economies and individuals. The main argument is that after 50 years of existence, the Web now needs to be “modernized”. It is only “made for computers and telephones” and is not up to the demands of IoT, air cabs or autonomous driving. There is simply not enough IP space to support all of this traffic. This was an argument used by the Chinese company Huawei, which has developed a new Internet protocol called “New IP”8 to replace the technological architecture that has underpinned the Web for half a century.
The states of Saudi Arabia, Iran and Russia support the initiatives presented by China for such a new infrastructure and have already taken legislative measures to implement this brave new world. In November 2019, for example, Russia passed a “Sovereign Internet Law,” which was described by Western media as a “digital ironclad process”9—and rightly so: because with Huawei's help, the Russians are developing tools that can be used to separate the Russian Internet from the global web. Officially, the aim is to protect against hostile cyberattacks and to guarantee national security. However, a desired side-effect, or presumably the main effect, is likely to be that the Russian population will be much better monitored and that critics of the regime will be isolated in their own country. Further examples will follow.
Beijing is systematically expanding its capabilities for technically controlled mass surveillance—and not only domestically. With 16 other countries10—from Egypt to the United Arab Emirates and Serbia—the Chinese government has signed declarations of intent to build a “Digital Silk Road”—or a system of advanced IT infrastructure.
Together, the Chinese government, Chinese telecommunications companies and the Chinese network equipment supplier Huawei continued to press ahead and officially submitted the topic of “New IP” to the International Telecommunication Infrastructure Standardization Organization (ITU) as a topic for its World Telecommunication Standardization Assembly, which is held every 4 years. At WTSA2011, which will be held next in India, the topic is now on the agenda and will be presented in detail at the Global Standards Symposium there. There, the member countries—excluding civil society, traditional business or independent Internet governance experts—could reach an agreement in favor of New IP by simple majority vote, even against the will of individual democratic states.
The fact that the meeting was postponed—probably due to COVID-19—from November 2020 to February 2021 gives only slightly more time. This is because the new standardization of the Internet infrastructure is being pushed forward by interested parties to such an extent that the search for alternatives can hardly keep up—such as the establishment of a decentralized anti-authoritarian free Internet protocol in the interest of all democratic societies. Such ideas are only marginally pursued at the ITU and lead a similar niche existence as the AI4Good conference, which deals with “Artificial Intelligence for Good,” i.e., applications of artificial intelligence and machine learning that are oriented toward the common good. Democracy and IT nerds do not have the financial resources of large countries. Yet they have the answers and instruments needed to let the European value system—and I don't mean just financial values—survive in the new digital world.
If we do not act fast, we might see a scenario predicted by Harvard economist Shoshana Zuboff. In her book “The Age of Surveillance Capitalism” she outlines two versions of the Internet, a market-driven capitalist and an authoritarian version. Both are based on total surveillance.
The introduction of New IP would make the authoritarian version globally standardized and scalable before our very eyes. Anyone who wants to become active on the Internet, whether it be downloading an app or accessing a website, would first need the permission of their Internet provider. Administrators would be able to deny access arbitrarily. Those who are afraid of the ubiquity of companies such as Apple, Google, Facebook and Co. should be even more afraid of New IP. We can still make the digital world democratic. To do this, we just have to take the reins of action.
The solution could be for the EU states to take a loud stand against the “New IP” and form a strong alliance with all other democratic states in the world. Even if a fundamentally new version of the Internet's core protocols is needed, on which there is no consensus at all, democratic governments should advocate that all aspects of the Internet protocol be left to the Internet Engineering Task Force (IETF). The IETF is an international association that is open to everyone and also involves non-governmental organizations in the discussion process. Besides, we require more experiments around new privacy-led decentralized technologies on the local level, linked to more transparency around how data is used.
#5: Data-Trusts
The states of the EU undertake to form one or more joint data trusts from which—anonymized and with the consent of the data owners concerned—all institutions may draw data according to agreed rules.
Decentralized technologies alone will not suffice to create a more sustainable digital world. We need institutions like data trusts, a kind of data cooperative, to provide a governance structure that organizes access to data in a way that takes into account the interests of those who create and use a particular set of data.
Such data cooperatives already exist. MIDATA12 is a Swiss cooperative that collects and manages health data of its members. In Taiwan, the digital minister Audrey Tang has launched an ongoing “presidential hackathon” to establish “data collaborations”13 In Finland, Sitra14, a political enterprise, has launched a similar competition to understand how data exchange can be made fair. The City of San Diego has been hosting hackatons for 4 years in an already well-integrated ecosystem that is geared to the needs of the city15 The city administration discloses data on traffic, cleaning, infrastructure repairs, weather, and the like. Industry giants like Qualcomm provide technology to process this data. Resident startups are building mini-solutions with maximum practical benefits, such as sensor-controlled irrigation of melon fields or collection of pollution data for the Navy using waste collection. Whether such projects increase and are successful is a question of pure political will, a well-mediated vision, and some organizational skills to bring together acting actors.
Recent literature describes requirements for establishing and operating data trusts, and introducing compelling governance mechanisms for different categories of data, e.g., medical, genetic, social media, or financial (Delacroix and Lawrence, 2019; Mills, 2019; Paprica et al., 2020).
#6: Increasing Data Literacy
Everyone has a basic right to digital education and free access to digital knowledge.
The obsession with data has permeated every part of our lives, from our work life to our personal lives and every interaction in between. Political and economic efforts regarding Internet technologies will be of little use if our children know nothing about data and Internet technologies.
It is therefore difficult to understand why the digital offensives of German education policy are limited to equipping schools with digital terminals, laptops, tablets, or smartboards. Much more urgently, we need a data customer offensive for German schools, universities and companies, i.e., the teaching of basic knowledge about data, its significance in our lives and in the economy, and also about programming approaches and terms such as user experience and experience design and problem formulation. This focus would lead to children and young people being aware of their digital shadows and mirrored worlds at a very early age. Knowledge brings responsibility and is a prerequisite for the growing generations to be able to actively decide what future digital life and work may look like, how much leeway data monopolies may have, and perhaps even how small data sets—whether about their own eating habits or donations to a local aid organization—can be used to solve the problems.
So far, European countries have not yet developed an approach to teach data and AI technologies to school children. There are some few efforts to democratize the knowledge on AI. As an example, Finland is rolling out a free online course covering the basics of AI to all European Union citizens16 The country hopes the nearly $2 million project—which will make its “civics course in AI” available in all E.U. official languages—will reach 1 percent of all union citizens by late 2021. The country is working with the University of Helsinki and tech consultancy Reactor to roll out the program, which is based on “The Elements of AI”—the most popular course ever offered by the university.
Today, China is clearly committing itself to more technology knowledge with nationwide educational programs on data and AI. Even the youngest children in kindergarten learn simple programming. Alibaba and Baidu are organizing vacation courses to teach students the approaches of Deep Learning, the AI technology that is now making spectacular breakthroughs in autonomous driving, precision diagnostics and smart speakers. Machine learning is a compulsory subject from the sixth grade onwards. Chinese schools around the world are leading the way in the use of robots in teaching and getting children used to working with machines as early as possible (Lauterbach, 2019).
Children love to ask “why” questions. When adults highlight data as part of the answers and find illustrative material to visualize data, they subtly teaching critical skills for data literacy. Technological progress requires innovation in pedagogy. The impetus for this does not necessarily come from Berlin, Brussels or Paris. Local study institutions and companies can also prioritize the task and get started. Or, in the words of John F. Kennedy: We chose to do these things, “not because they are easy, but because they are hard, because that goal will serve to organize and measure the best of our energies and skills, because that challenge is one that we are willing to accept, one we are unwilling to postpone, and one which we intend to win.”
#7: Making Environmental Considerations Part of the Digital Agenda
The question on what data we collect and analyze is an ecological one. Energy consumption of digital technologies should be made transparent and sustainable.
Rethinking the approach to handling of data, platform monopolies, competition, and enabling traditional businesses to utilize data technologies in a safe and beneficial way would not be complete without highlighting challenges in current status of Machine learning.
When Cristiano Ronaldo posts a photo for his 199.2 million Instagram followers, he uses 30 megawatt hours of energy17 This usage corresponds to the energy consumption of six German large family households for 1 year.
By extension, Data scientists involved in complex computations with massive corpora of data consume even larger amounts of energy. In 2019, researchers at OpenAI developed an algorithm to manipulate parts of a Rubik's cube with a robotic hand. A thousand desktop computers and a dozen computers with GPUs were used to compute the task, driving the energy consumption to around 2.8 gigawatt hours, which roughly corresponded to the output of three nuclear power plants for 1 hour, according to an estimate by Evan Sparks, CEO of Determined AI.18
According to an estimate by the US Department of Energy, data centers around the world consume around 200 terawatt hours of electricity per year19 Consider that this demand was near zero only a generation or so ago. Some predictions assume that data and communications technologies will consume between eight and 20 percent of global electricity by 2030. A third of this consumption is in data centers. Businesses and governments require a dialogue with leading data scientists and research facilities within and outside of Internet companies to address the challenge today. According to forecasts, there will be 25 billion connected devices in the world by 2025. Data is not a new oil. Oil is a finite natural resource that is consumed when it is used. Data lives on. It increases at a rate that is itself increasing. If we are to make use of this ever-expanding resource, we need to be mindful stewards while data gets bigger and bigger.
A first step should be transparency: companies should urgently introduce standards for sustainability and environmental compatibility—analogous to accounting standards. Big counterparties like Google have made considerable commentary focused on only getting electricity from renewable energies and increasing energy efficiency with the help of machine learning tools and applications. Nevertheless, Google does not disclose its energy consumption. Today businesses of all sizes are seriously thinking on how to introduce measures and technologies to be more sustainable. Regulators around the world are taking a tougher stand on ESG (Environmental, Social, Governance) disclosure. The most regulated topics are business ethics and climate change in financial services, energy use, and consumer rights in the US utilities, and product and service safety in healthcare and pharmaceuticals. It is only a matter of time when data governance and safe and environmental-friendly data technologies will be considered within ESG and even be made mandatory in a number of industries, e.g., in financial services.
With the help of tools such as the Machine Learning Emissions Calculator20, the CO2 footprints of algorithms can be roughly calculated. Chip manufacturers such as Nvidia and Qualcomm are investing in the production of energy-efficient chipset architectures. This focus contributes to the energy efficiency of the whole technology stack and should therefore be supported.
Addressing data centers in national policies should become widespread. Switzerland, as an example, assumes that data centers could even account for up to 50 percent of the country's total energy consumption by 203521 This is why the country is working hard on efficiency standards and on powering the servers primarily with renewable energies.
The World Economic Forum lists in “Fourth Industrial Revolution for the Earth”22 more than 80 ways in which AI can be used sustainably. A number of companies put strategic bids on linking Internet of Things and machine learning technologies, to sell sensors for manufacturing, health and agricultural facilities. Technology can help protect our climate, but it can collect a lot of data that nobody really needs—and that nonetheless consume valuable resources.
The question of which data we really want to produce and collect is also an ecological one.
#8: Increasing innovation around Small Data and Data Privacy.
Democratization of data technologies is unthinkable without innovation on small data and data privacy.
There are countless situations where it is difficult for humans to understand the intricate relationship among a large number of features. Computers however can easily capture it by exploring large amounts of data. Since Peter Norvig and his colleges at Google found that for a given problem, with large enough data, very different algorithms perform virtually the same, the hunger to collect, store and process large quantities of data powered technology stacks of companies such as Facebook, Alphabet, Amazon, and Baidu. Collecting and processing as much data as possible can't be a sustainable approach for the future, if we want a multitude of businesses and individuals to benefit from data. Solutions focused on very large data sets often don't have appropriate treatments for bias and variance problems. The noise in these large data sets can often overwhelm the important signals that relate to the problem at hand (imagine trying to hear a very important conversation in a crowded restaurant). In some cases, e.g., detecting rare diseases, there isn't enough data in the first place, so the “missingness” in these large corpora present a sort of confirmation bias that can be not only misleading, but wasteful of resources that might otherwise address the problem with more proper analytic solutions.
Use of small data in today's successful ML technologies such as deep learning is not discussed as much as it should be.
Encouraging innovation around small data should be an integral piece of every digital agenda. Working with small data requires skills in statistics and data science (including data cleaning/preparation), as there are multiple problems to address, e.g., outliers, over-fitting in modeling, or creation of realistic samples while working with time series. There are some techniques deserving further exploration such as:
• Including domain-specific knowledge to guide the learning process (e.g., Human-level concept learning through probabilistic program induction and heuristics),
• Pre-train a network with more optimal starting weights to avoid local optimums and other pitfalls of bad initialization while using stacked autoencoders, possibly enhanced with cognitive methods to converge on the proper weights,
• Implement ensemble mechanisms to neural networks, and choreograph so called week learners to produce a prediction while using algorithms such as Support Vector Machine or Decision Trees, or other unsupervised or ensemble methods,
• Use techniques such as Cosine Loss to increase in accuracy for small datasets when switching the loss function from categorical cross-entropy loss to a cosine loss for classification problems,
• Augment data or make slight changes to the data to produce more data points while experimenting with GANs to generate new data (note, such methods can be confounding to anomaly detection).
If companies, non-profits, educational, and medical facilities, and communities gain in capabilities to leverage their data ownership via distributed data markets, they can provide access to its data to more data scientists. Data science will become far more federated than in the large-company-centric models of today.
Innovation on data privacy happens in large companies, even as they get increasingly hit by fines for negligent management of user data.
Google invested considerable efforts in federated learning, which is about training a centralized model on decentralized data and computation of encrypted data from multiple devices.
Big data breaches, challenges to safely exchange large amount of data for training purposes among research institutions and adverse consequences of exposing personal information are driving a number of private and public organizations to use privacy models like Differential Privacy. In these models, one data set is systematically replaced by another that contains different information but has the same statistical patterns. In this way, conclusions about the identity of the data provider can be avoided, while supporting the necessary analytic rigor for important use cases.
Another attractive technology is “Homomorphic Cryptography,” in which algorithms can process data without decrypting it. This approach enables the intensive use of valuable data including a high level of data protection. Such methods are indeed promising, although there are still challenges to certain use cases such as advanced anomaly detection and synthetic data.
#9: Openness and transparency over development of data-technologies should be funded and protected.
Independent researchers should be allowed to access work of large Internet companies to contribute to risk mgmt. around how AI is getting created.
Today there is not enough openness or transparency in how data technologies are being developed. As an example, machine/deep learning research has become increasingly concentrated in the wealthiest US and Chinese companies. These private companies are building and controlling the algorithms that shape our lives and workplace. It is not clear how Risk Management practices are balanced against necessity to drive commercial objectives such as profit and growth. Lack of diversity in gender, race, age, and experience in different fields of disciplines (including Humanities) among Internet pioneers and their followers in executive management and corporate boards has been already lamented in a number of podcasts, movies and publications. A “move fast and break things” mentality might not be the best fit while designing AI based and automation systems, which can have far-reaching impact for people and society in general. Besides, the few remaining independent research facilities with some scale inevitably get under the sphere of influence of major commercial players, while limiting projects to provide AI technologies to small businesses, research centers, and non-profits. Academia, which 20 years ago was able to retain the brightest minds, cannot compete with Big Tech for AI talent (Lauterbach and Bonime-Blanc, 2018).
For example, OpenAI provided Microsoft with an exclusive access to GPT-323, the world's largest language model and one of the most important innovations in NLP. OpenAI was originally founded as a non-profit and raised its initial billion dollars on the premise that it would pursue AI for the benefit of humanity. It asserted that it would be independent from for-profit financial incentives and thus uniquely positioned to shepherd the technology with society's best interests in mind. Over the years, however, the pressures to fund research made this independence unsustainable.
In 2018 Microsoft acquired GitHub, a move which permanently changed the culture of developers and—according to many users—limited their freedom.
Outside researchers are kept at arm's length even in companies where management claims to work on transparency and optimizing technology for social good. In 2018, for example, Twitter launched a study designed to promote civility and improve behavior on the platform24, and collaborated with Susan Benesch and Cornell's J. Nathan Matias, founder of the Citizens and Technology Lab. The company ended up abandoning the project, citing coding errors.
Securing public and supporting private funding of AI institutes and initiatives should be a priority for years to come as it contributes to a better risk management around how data technologies get created and deployed. Mariana Mazzucato, Director of the UCL Institute for Innovation & Public Purpose in the UK, has been emphasizing for years the importance of governmental investments which drive technology progress in private corporations, e.g., Apple, and calling for mission-oriented industrial policies to direct research to the most urgent economical and societal problems. Her latest work for the European Commission was focused on approaches in public sector to respond to Covid-19 pandemics. Though she claimed the necessity to rethink the data governance, experiment with new forms of ownership over internet platforms and data, no concrete solutions were offered (Mazzucato and Kattel, 2020). Technologists need to step in to suggest solutions beyond tracking apps for mobile devices.
#10: Participatory Platforms around Data Technologies
Municipalities, businesses and non-profits of all sizes should be capable to participate in increasing digital capabilities of their communities.
Building a diversified portfolio of approaches to evolve understanding, competence, and influence in the most relevant domains of AI is not an easy task.
Some of success can be achieved top-down, with advances in governmental policies and funding as it was mentioned in the previous section of this article. International cooperation is helpful too. As an example, in 2020 US and UK signed an agreement to jointly support trustworthy AI (Declaration on Cooperation in Artificial Intelligence Research and Development)25 in interdisciplinary R&D, innovations on regulations, and workforce development.
Good initiatives occur through organizations coordinating industry, academia and non-profits, e.g., The Partnership on AI workshop at NeurIPS26 in 2020 about publication norms in AI research.
Still, society can more fully benefit from AI and data only, if local organizations open up to explore new technologies in order to adopt them to their needs. Albert Einstein is said to have remarked: “The World cannot be changed without changing our thinking.” Local leadership is needed to explore the full potential of data and AI for the benefits of many. Starting simple with a few basic business questions is more likely to be effective than calling out a massive reform that is likely to fail over time. Being transparent (sometimes referred to as explainability) is key. Amsterdam and Helsinki launched in September 2020 AI registries to explain how each city government uses algorithms to deliver services as part of the Next Generation Internet Policy Summit of the European Union. This is a step into the right direction, as it communicates data technology as part of daily problem-solving approaches.
Municipalities of all sizes should create centers focused on the digital capabilities of their communities and enable coordination of local industries, start-ups and non-profits to indemnify areas of overlapping issues that are common to all participants of the local ecosystem. Involved businesses should ensure their APIs are well-documented, communicate about issues of bias and fairness and security directly, and develop better systems for preserving developer privacy. Machine learning practitioners should carefully analyze these APIs prior to using them, test against benchmark datasets that relate to potentially discriminatory outcomes of ML projects, share ethical issues about the API via opening pull requests on the dev's GitHub page (if available), and be clear about the usage of the API in documentation about services it is used within. All of these steps will work not only toward enhancing explainability, but also help avoid unintentional misuse. With a creation of a local data market around municipalities, concrete questions can be addressed to encourage entrepreneurship and self-employment, education around data, and making better calls on public spending.
Progress in such endeavors is a direct function of leadership. Successful companies in AI have spent considerable number of efforts to bust silos, increase learning across the whole organization, and utilize network effects to get the best outcome from the underlying data and data technologies. These best practices on execution can be applied in private and public sector.
We are indeed at a crossroads with respect to data and AI. It is not a guarantee that all progress will take us in a positive direction. By focusing on key guiding principles, we can be not only reflective of how we can use the amazing abundance of tools and technology, but why. This reflection would be a powerful step in the right direction.
The original contributions presented in the study are included in the article/supplementary material, further inquiries can be directed to the corresponding author/s.
This article was a response to practical shortcoming of current digital agendas in Germany and Europe and offers a comprehensive view on critical components of policies from technology questions (e.g., protocols for decentralized data markets, handling of small data) to societal issues (e.g., digital literacy, data trusts), and geopolitical aspects (US and China efforts in digital and AI policies).
The author confirms being the sole contributor of this work and has approved it for publication.
The author declares that the research was conducted in the absence of any commercial or financial relationships that could be construed as a potential conflict of interest.
1. ^https://hy.co/2019/06/10/hy-infographik-dax-unternehmen-vs-plattformen/
2. ^https://www.plattform-index.com
3. ^https://medium.com/@tokencuratedregistry/a-simple-overview-of-token-curated-registries-84e2b7b19a06
4. ^https://cyber.ee/about-us/our-story/
8. ^https://www.huawei.com/de/deu/magazin/aktuelles/new-ip
9. ^https://netzpolitik.org/2019/digitaler-eiserner-vorhang/
10. ^http://frankfurt.china-consulate.org/det/zt/ydyl2/P020190715630556064579.pdf
11. ^https://www.itu.int/en/ITU-T/wtsa20/Pages/default.aspx
12. ^https://www.midata.coop/en/home/
13. ^https://presidential-hackathon.taiwan.gov.tw/en/
14. ^https://www.sitra.fi/en/themes/about-sitra/
15. ^https://www.iotsmartcitiessummit.com/daniel-obodovski
16. ^https://www.elementsofai.com
17. ^https://www.linkedin.com/pulse/climate-risk-20-selfie-cristiano-ronaldo-dancing-despacito-assab/.
18. ^https://www.wired.com/story/ai-great-things-burn-planet/
19. ^https://www.osti.gov/servlets/purl/1372902
20. ^https://mlco2.github.io/impact/
21. ^https://www.nsenergybusiness.com/features/data-centre-energy-efficiency/
22. ^http://www3.weforum.org/docs/WEF_Harnessing_the_4IR_for_the_Earth.pdf
23. ^https://blogs.microsoft.com/blog/2020/09/22/microsoft-teams-up-with-openai-to-exclusively-license-gpt-3-language-model/
24. ^https://medium.com/@susanbenesch/launching-today-new-collaborative-study-to-diminish-abuse-on-twitter-2b91837668cc
25. ^https://www.state.gov/declaration-of-the-united-states-of-america-and-the-united-kingdom-of-great-britain-and-northern-ireland-on-cooperation-in-artificial-intelligence-research-and-development-a-shared-vision-for-driving/
Acemoglu, D., Makhdoumi, A., Malekian, A., and Ozdaglar, A. (2019). Too Much Data: Prices and Inefficiencies in Data Markets. NBER Working Paper No. 26296, September 2019, JEL No. D62,D83,L86. Available online at: https://www.nber.org/papers/w26296 (accessed January 04, 2021).
Delacroix, S., and Lawrence, N. D. (2019). Bottom-up data trusts: disturbing the ‘one size fits all' approach to data governance. Int. Data Privacy Law 9, 236–252. doi: 10.1093/idpl/ipz014
Lauterbach, A. (2019). “Trojanische Verhältnisse?” in Tobias Loitsch, China im Blickpunkt des 21. Jahrhunderts: Impulsgeber für Wirtschaft, Wissenschaft und Gesellschaft (Berlin: Springer), 1–17.
Lauterbach, A., and Bonime-Blanc, A. (2018). The Artificial Intelligence Imperative: A Practical Roadmap for Business. Transl. by I. Bremmer. Praeger (Santa Barbara, CA), 99–100.
Mazzucato, M., and Kattel, R. (2020). Covid-19 and public sector capacity. Oxford Rev. Econ. Policy 36(Suppl. 1), S256–S259. doi: 10.1093/oxrep/graa031
Mills, S. (2019). Who owns the future? Data trusts, data commons, and the future of data ownership. Working Draft. doi: 10.2139/ssrn.3437936
Paprica, P. A., Sutherland, E., Smith, A., Brudno, M., Cartagena, R. G., Crichlow, M., et al. (2020). Essential requirements for establishing and operating data trusts: practical guidance co-developed by representatives from fifteen Canadian organizations and initiatives. Int. J. Popul. Data Sci. 5:1353. doi: 10.23889/ijpds.v5ii.1353
Keywords: #Data, #DataPrivacy, #AI, #DataTrusts, #DataGovernance, #DecentralizedWeb, #DataLiteracy, #SmallData
Citation: Lauterbach A (2021) Unitarism vs. Individuality and a New Digital Agenda: The Power of Decentralized Web. Front. Hum. Dyn. 3:626299. doi: 10.3389/fhumd.2021.626299
Received: 05 November 2020; Accepted: 13 April 2021;
Published: 23 June 2021.
Edited by:
Alexandra K. Przegalinska, Kozminski University, PolandReviewed by:
Le Yu, Hong Kong Polytechnic University, Hong KongCopyright © 2021 Lauterbach. This is an open-access article distributed under the terms of the Creative Commons Attribution License (CC BY). The use, distribution or reproduction in other forums is permitted, provided the original author(s) and the copyright owner(s) are credited and that the original publication in this journal is cited, in accordance with accepted academic practice. No use, distribution or reproduction is permitted which does not comply with these terms.
*Correspondence: Anastassia Lauterbach, YWxhdXRlcmJhY2hAbWUuY29t
Disclaimer: All claims expressed in this article are solely those of the authors and do not necessarily represent those of their affiliated organizations, or those of the publisher, the editors and the reviewers. Any product that may be evaluated in this article or claim that may be made by its manufacturer is not guaranteed or endorsed by the publisher.
Research integrity at Frontiers
Learn more about the work of our research integrity team to safeguard the quality of each article we publish.