- 1School of Bioinnovation and Bio-based Product Intelligence, Faculty of Science, Mahidol University, Bangkok, Thailand
- 2MUSC Centre of Excellence in STEM Education, School of Bioinnovation and Bio-based Product Intelligence, Faculty of Science, Mahidol University, Bangkok, Thailand
- 3Premise Biosystems Co., Ltd., Bangkok, Thailand
- 4Excellent Center for Drug Discovery, Faculty of Science, Mahidol University, Bangkok, Thailand
- 5Department of Surgery, Faculty of Medicine Ramathibodi Hospital, Mahidol University, Bangkok, Thailand
- 6Biophysics Lab, Department of Physics, Faculty of Science, Mahidol University, Bangkok, Thailand
Objectives and design: Low-grade inflammation is a hallmark of chronic diseases. More sensitive tools for chronic low-grade inflammation detection are needed and herein presented as a proof of concept. Heightened sensitivity to inflammation may be achieved by analyzing the compensation mechanisms of hematopoiesis in response to stress. The production of red blood cells and platelets, which are particularly vulnerable to physiological imbalances, are especially important in this context due to their high turnover rates. The compensatory mechanisms involve the production or release of rare immature blood cell types that herein serve as important biomarker targets.
Methods: A cell-based liquid biopsy platform, using negative selection, was used to detect circulating rare cells in comprehension, allowing simultaneous analysis of an immature cell panel from one sample. The concentration ranges under physiological conditions for each cell marker were evaluated on a self-reported healthy control cohort and prospectively tested on three individuals undergoing various interventions: one afflicted with early-stage breast cancer, another with atherosclerosis in follow-up, and a third healthy individual with cardiovascular disease risk.
Results: The approach effectively identified rare cellular abnormalities in asymptomatic individuals who exhibited no abnormalities in their complete blood counts. This condition was designated as silent inflammation (SI). SI was effective in monitoring response to intervention and predicting inflammation state.
Conclusions: The detection of SI proved valuable in aiding inflammation differential diagnosis and for monitoring the response to interventions in all three subjects.
1 Introduction
Low-grade chronic inflammation, often unnoticed clinically, is believed to be the underlying cause of numerous chronic diseases and contributes to accelerated aging, known as inflammaging (1–4). Chronic inflammation involves sustained activation of alternative pathways, tissue damage, ischemia, and necrosis, primarily driven by the prolonged activity and recruitment of immune cells, such as macrophages and neutrophils, to the affected area. These cells release signaling molecules (cytokines and chemokines) that promote vascular changes, enhance blood flow, and increase the permeability of blood vessels leading to the elimination of pathogens, the removal of dead cells, and the initiation of tissue repair (1, 2, 5). While chronic low-grade inflammation in asymptomatic non-diseased individuals may not be classified as a clinical condition or disease, it signifies a disrupted physiological state with the potential to compromise overall health. Analyzing such inflammation states could help identify apparently healthy individuals who are at risk of chronic diseases and improve the sensitivity of measuring therapeutic responses and inflammation dynamics over time. However, we may need more sensitive detection tools that support studying the connection between chronic conditions or anti-inflammatory treatments and specifically low-grade chronic inflammation (6). Blood marker analysis, focusing on metabolism, is practical, including lipid and glucose profiling (2). Advanced laboratory tests may examine intravascular coagulation using D-dimer or plasminogen activator inhibitor-1, or measure inflammatory signaling with serum levels of pro-inflammatory cytokines or acute-phase proteins such as the cardio-reactive protein (CRP). CRP might be more sensitive than the erythrocyte sedimentation rate, but cytokines and acute-phase proteins can have varied baseline levels based on factors like age, ethnicity, and gender, and can respond differently to stressors (3, 5, 7). In this vein, CRP is biased towards interleukin-6 (IL-6)-associated inflammation and relies on liver function, making diagnosing low-grade inflammation challenging due to marker insensitivity, a wide range tolerance, and susceptibility to acute phase changes, leading to unreliable interpretations in the low concentration range.
We propose to assess the blood’s response to inflammatory stress, herein referred to as hematological stress, for predicting low-grade inflammation (8–12). Hematological stress involves stress hematopoiesis that entails responses including stress erythropoiesis (8–10) and thrombopoiesis (13–15), respectively, as highly sensitive mechanisms compensating for inflammation-induced high-throughput cell imbalances. Low-grade inflammation detection was further based on predicting vascular injury and hypoxia denoting typical inflammation indicators (16, 17). Immature blood cell types are crucial for this examination, as they exhibit the highest sensitivity to stress due to shorter lifespans and, in parts, greater functionality (18), although they are rare in low-grade inflammation thus, posing a challenge for detection.
As far as we know, there has not been a comprehensive analysis of rare circulating blood cells to detect hematological stress and predict low-grade inflammation. Despite their rarity, highly immature circulating erythroblasts and platelets are present under normal conditions and can be examined using specialized assays like flow cytometry (19) or cell-based liquid biopsy platforms (20, 21). While cell-based liquid biopsy technology was primarily used for cancer detection (22), it was utilized to analyze immature hematopoietic CD45-negative cells, such as erythroblasts (23), circulating megakaryocytes (24), or circulating endothelial cells (25). We propose a marker panel to probe stress erythropoiesis involving analysis of highly immature large reticulocytes and normoblasts; to probe platelet-related stress involving analysis of circulating platelet aggregates (CPAs), large immature platelets, giant platelets (G.Plt), and naked megakaryocytes (nMkcs) (18, 26, 27); and to probe vascular injury involving analysis of circulating endothelial cells (28). Although these markers are not new, their use in probing ultra-low physiological concentrations represents progress towards more sensitive analysis of low-grade inflammation.
Usefulness relating to treatment response measurement based on low-grade inflammation detection and grading is demonstrated in three example cases, one concerning the monitoring of breast cancer standard care treatment and two others concerning the assessment of lifestyle change in an atherosclerosis patient during follow-up and in a healthy individual with the risk of cardiovascular disease.
2 Material and methodology
2.1 Study samples
A healthy control group consisting of 20 individuals aged 23 to 66 years (average, 39.8 years) was tested aiming at the identification of the concentration ranges of rare hematological markers at true physiological steady state and aiming at developing thresholds for abnormality (see Supplementary Table S1). A cancer cohort comprising 17 treatment-naive individuals afflicted with mamma carcinoma of various types staged 0–2 was included to test significance in difference of CPAs between the cohorts of healthy and cancer-afflicted individuals. Three individuals were used for SI detection and prospective treatment monitoring comprising one individual afflicted with early-stage breast cancer, a healthy individual with sedentary and work-laden lifestyle being at risk of cardiovascular complications, and one individual treated for atherosclerosis by stent placement 2 years prior without cardiological complications. The breast cancer patient aged 37 reported a drop in quality of life (QoL) during and after adjuvant chemotherapy from 90% to 70%. The breast cancer patient’s inflammation status was investigated from pre- over peri- to post-intervention of a total of five tests in a 1-year period comprising two tests, before and 4 weeks after surgery, as well as three tests, during, 2 weeks after adjuvant chemotherapy, and 6 months into follow-up. The patient was diagnosed with ductal invasive carcinoma without a special type having a 17-mm-sized tumor in the left breast without lymphovascular invasion and node involvement. The patient did not report any comorbidity. The histology report suggested ER: 80%, PR: 70%, HER2: 0%, and Ki67: 50%. The patient was treated with breast-conserving surgery and sentinal lymph node biopsy as well as adjuvant chemotherapy as follows: Dexamethasone 10 mg IV stat/Ondansetron 8 mg IV stat/Doxorubicin 60 mg/m2/day IV every 3 weeks/Endoxan 60 mg/m2 IV every 3 weeks/Olanzapine 5 mg PO hs * 5 day/Ondansetron 8 mg PO every 12 h * 5 days. A healthy person with a sedentary work-centric lifestyle aged 54 herein referred to as an “unhealthy” person was selected for SI analysis falling into the risk profile of cardiovascular disease. The person reported a 154% weight gain over a 3-year time span, a sleeping period of less than 7 h, regular but inexcessive alcohol consumption without smoking, no special nutritional awareness, and physical inactivity. The unhealthy person reported subjective wellbeing and normality in basic laboratory tests including complete blood count and no comorbidities. The unhealthy person agreed to lifestyle changes after a first SI test and was followed up 3 months later. The lifestyle changes primarily included 16/8 protocol intermittent fasting with an increase in physical activity (fitness club visitations twice a week) and lowered alcohol consumption. The atherosclerosis-afflicted individual aged 64 was used to measure response to lifestyle change having tested before and after a 2-month self-responsible lifestyle intervention comprising longer sleeping hours from 5 to 6 h to over 7 h, intermittent fasting following the 16/8 rule, an increase in physical activity to over 1 h per day (mostly fast walking), drinking herbal tea (mulberry), and nutritional adaptation towards lower carbohydrates and more fats without snacking.
2.2 Rare cell enrichment
Peripheral blood was taken by venous puncture collecting 10 mL in a green-top BD Vacutainer blood collection tube containing sodium heparin. The blood sample was processed not longer than 3 h after phlebotomy and kept at room temperature until use. Informed consent was sought from the patient at the time of the blood draw. For systemic abnormality analysis, the Rarmax® cell-based liquid biopsy platform (Premise Biosystems Co., Ltd., Version 2.3) was used as previously (Schreier et al., 2024, is under peer review, retrievable on request) described following the principle of negative selection. In brief, whole blood nucleated cells were pre-enriched by bulk red blood cell (RBC) removal based on chemical lysis and desired rare cells were enriched by removing bulk white blood cells (WBCs). The analysis was conducted by automated fluorescence microscopy. In detail, standard chemical lysis buffer (154 mM NH4Cl, 10 mM NaHCO3, and 2 mM EDTA) treatment was applied to remove RBCs from 7 mL of whole blood. The cell suspension was incubated twice at room temperature (RT) for a maximum of 3–5 min following centrifugation at 300×g for another 5 min each. The final cell pellet was resuspended in 0.5 mL of PBS, supplemented with 0.5% bovine serum albumin, and allowed to rest for 15 min at RT. The cell numbers of nucleated cells subsequent to RBC lysis were determined by a hemocytometer (Neubauer) and subjected to enrichment. In brief, peripheral blood rare cell isolation was carried out by automated CD45-positive cell depletion assay (Walderbach II) following the manufacturer’s description (SanoLibio Co. Ltd., Thailand). Subsequent to enrichment, the sample was split into equal halves containing 20–30 µL each and immediately stained for subsequent analysis by fluorescence microscopy adding anti-CD31_FITC (Ebioscience), anti-CD45_PE (Ebioscience), and anti-CD24_PE (Ebioscience) in one panel, and anti-CD71_FITC (Ebioscience), anti-CD24_PE (Ebioscience), and anti-CD45_PercPCy5.5 (Ebioscience) in the second panel, each using 0.5 µL of undiluted dye solution and incubating at RT in the dark for 25 min. A washing step was followed by diluting the cell suspension with 1 mL of PBS, supplemented with 1.5% bovine serum albumin prior to pelleting by centrifugation at 200×g for 3 min and resuspending in 120 µL using PBS for each stained sample. Nucleus staining was performed prior to microscopy using 0.5 µL of Hoechst 33342 DNA staining reagent (1:10 dilution) (ThermoFisher).
2.3 Image acquisition and analysis
The enriched and stained cell suspensions (panels 1 and 2) were added into two flat bottom wells per panel of a 384-well ViewPlate-black plate (Perkin Elmer) and monolayered by centrifugation at 80×g for 2 min, which was followed by high-resolution image acquisition at 40× magnification using the Operetta high-content imaging system (PerkinElmer). A standard acquisition protocol was used for all samples, allowing intra-patient and inter-patient sample comparability. Images were recorded from a total of six channels based on the fluorophores used, with optimized and fixed exposure times. Columbus analysis software served as a screening and image analysis tool. Sample analysis followed two reader assessment with the first person conducting an image screening step according to a standardized reading protocol and the second person conducting rare cell identification. Image records per sample comprised 117 fields (color z-stack) per well. Rare cell marker-positive cells were identified by a cell-like round formation, specific morphologies as detailed in the Results section, positive Hoechst DNA staining, and positive (CD71 and CD31) fluorescence emission in the absence of the typical ring formation or membrane staining as a consequence of positive CD45_PE/CD24_PE or CD45_PerCy5.5 staining. The hematopoietic status was excluded in case of CD45 or CD24 signal at background noise level and low PE-channel autofluorescence as identified by dim fluorescence signal inside the cellular event and the absence of membrane staining (see Supplementary Figure S3). Sample read-out followed the list of cell types as described in the Results section. High median marker concentrations >50 cells per 5 mL (e.g., rare platelet fraction or CPAs) measured in healthy donors sufficed the reading of 2 × 20 fields of the 117 fields per well then extrapolating the concentration to 3.5 mL per panel and finally normalizing to our standard of 5 mL. All other cells were read over the entire collection of fields. Endothelial cells as well as large platelets and G.Plt were identified by CD31-positive staining and distinguished by significance in morphology (see Results). The analysis of circulating endothelial cells involved not only total counts per 5 mL but also an estimate of morphological heterogeneity following size, shape, nucleation status, and clustering. A different cell morphology was implied if at least one of the criterion differed substantially. The sum of cell morphologies was denoted as endothelial pleomorphic factor. The analysis of endothelial cells was used to verify response to treatment as well as the prediction of vascular injury by a subset of markers. Image analysis of CD71 followed descriptions as previously made (23).
2.4 Statistical analysis
Analysis of cohort data sets included the assessment of statistical significance in difference between cohorts and was based on the two-sample unpaired open t-test and calculated by standard formulas provided in Microscoft Excel. A p-value ≤ 0.05 was considered statistically significant. Standard statistical calculation included values of mean, median, and standard deviation that were also calculated by Microsoft Excel.
3 Results
Rare cells occur within specific concentration ranges under normal conditions in adults, typically ranging from one cell to a few hundred per 10 mL. Detecting such low concentrations relies on the functional sensitivity of each detection platform, which often has inadequate limits of detection. As a result, normal and abnormal ranges are relative to the detection platform, making standardization currently unattainable. We utilized the Rarmax platform (Version 2.3), a cell-based liquid biopsy system based on negative selection, to investigate rare cell healthy concentration ranges in a control group of 20 individuals of varying demographics (refer to Material and Methodology). The median concentration served as a cutoff for predicting physiological equilibrium (refer to Table 1). Steady-state hematopoiesis and the absence of inflammation were confidently assumed when cell concentrations fell below this median value. We operated under the premise that elevated levels of rare immature blood cells would likely correlate with increasing severity of inflammation-induced hematological disorder, potentially leading to clinically evident cytopenia or cytosis. Hence, concentrations surpassing the median but within the control group’s range and below the abnormality cutoff were deemed elevated without clinical significance. We chose a 75% cutoff instead of the 95% due to the undefined nature of the control cohort and the lack of a sensitive gold standard for detecting subtle inflammatory imbalances. This decision acknowledges a probability that one-third of healthy donors might be outliers with non-apparent inflammatory imbalances. Notably, our results suggested a 50% positive rate of low-grade inflammation among healthy donors at the specified cutoffs, albeit at a low grade (Supplementary Table S1).
3.1 Markers of silent inflammation
This platform was designed to enable the identification and differentiation of a wider range of rare cell types, facilitating a comprehensive approach to rare cell analysis in realization of what we have referred to as systemic cytology (29). This was achieved by utilizing a minimal set of fluorescent antigen markers (see Material and Methodology) while also incorporating morphological distinctions for further cell-type differentiation. The analysis principle involved distinguishing between hematopoietic and non-hematopoietic cells and was realized by the absence of CD45 marker staining and, thus, excluded all rare hematopoietic cells. Nevertheless, unambiguous identification of the CD45-negative status was hampered by cellular autofluorescence. We would like to refer to Supplementary Figure S3 for the clarification of CD45 status identification.
The marker panel included the analysis of platelets. The platelet (Plt) count was used to distinguish between compensated and uncompensated stress hematopoiesis. Uncompensated stress hematopoiesis was identified when Plt count exceeded 4.5 × 105 cells or fell below 1.5 × 105 cells per µL, while compensated stress hematopoiesis was indicated by platelet levels within this range, referred to as silent inflammation (SI).
The nMkc was included into the panel as a surrogate marker for the prediction of upregulated megakaryopoiesis (MK) herein referred to as accelerated MK (aMK) (30–32). In general, aMK is predictive of peripheral platelet consumption or loss (30, 31). The nMkc is directly associated with the megakaryocyte denoting one of two cellular end products of the megakaryocyte after platelet release (33). The other end product is referred to as micro-megakaryocyte appearing as smaller cells with a cytoplasm and slightly irregular non-lobular or bilobular nuclei with a dense mature chromatin when compared to larger nMkc that appear as large less dense bare nuclei with segmentation or lobes (Figure 1A). Owing to the clear morphological distinction, only the nMkc was used for marker identification. The nMkc physiological steady-state concentration range determined by our platform measured ≤4 cells per 5 mL (median value). Concentrations greater 12 cells per 5 mL and multi-segmentation in at least two cells (>2 cell segments, as a sign of increased polyploidy) were interpreted as being predictive of inflammation-induced aMK. The morphological correlation between increased ploidy and nucleus segmentation may be established (34–36); however, in nMkc, the exact polyploidic state may not be predicted based on morphology. The link between nMkc polyploidy and inflammation is backed by evidence showing that certain pro-inflammatory factors directly affect megakaryocyte-committed progenitors. This interaction promotes enhanced differentiation and accelerated maturation of megakaryocytes, leading to increased polyploidy (15, 31, 37). Moreover, IL-6 was reported to have an indirect influence by stimulating thrombopoietin (TPO) secretion (14) or a direct influence in combination with other pro-inflammatory cytokines, for example, IL-3 (32, 36). These revelations may have been leveraged mostly for acute inflammation detection; however, there is little reason to believe that the mechanism would not apply to low-grade inflammation.
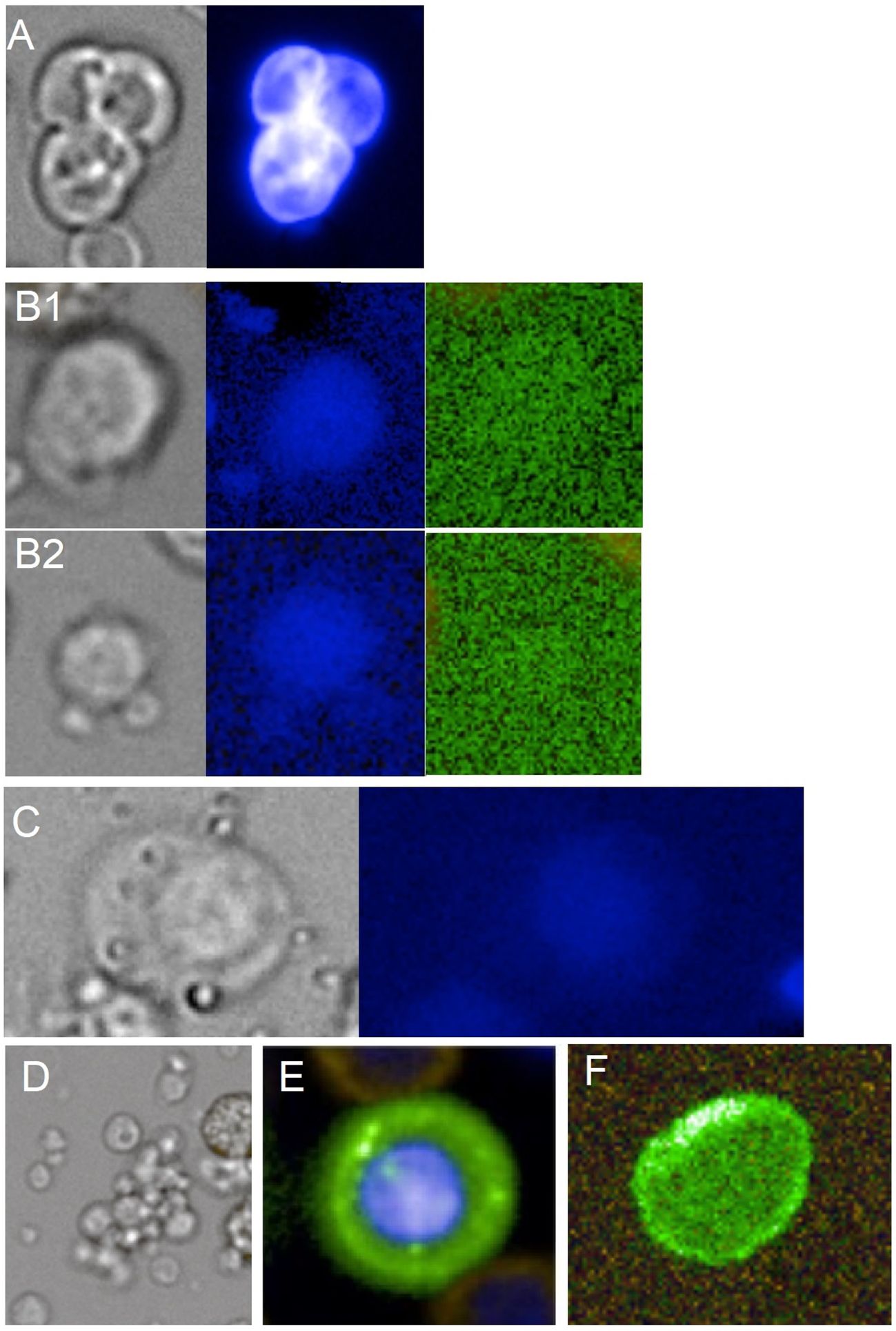
Figure 1. Gallery of SI markers. (A) tri-segmented naked megakaryocyte sized 18.7 µm shown in bright-field appearance (gray) and Hoechst staining (blue). (B1, B2) Large 11.4-µm and smaller 6.8-µm rare immature platelets shown in bright field (gray), Hoechst stain (blue), and near-background dim autofluorescence (green). (C) Giant platelet shown in bright field (gray) and Hoechst stain (blue) sized 16.3 µm. (D) Circulating platelet aggregate of mixed sizes in bright field (gray). (E) Normal circulating erythroblast showing CD71-positive membrane staining (green) and Hoechst DNA stain (blue) sized 10.4 µm. (F) Immature CD71+ large reticulocyte of 8.4 µm in major diameter showing CD71-positive membrane staining (green).
Immature platelets were employed as markers to signify deviations from hematopoietic homeostasis and were identified by their distinct morphology and specific characteristics of rarity including larger size (>5.5 µm), medium to high density (approximately >1,067 g/dL), positive CD31 staining, and autofluorescence in the FITC channel as a sign of increased mitochondrial activity (38–40) (Figure 1B; Supplementary Figure S1). These selection criteria yielded a notably low concentration range in the healthy control cohort, ranging from 130 to 1,180 cells per 5 mL. Hence, this subset of immature platelets was termed the rare platelet fraction (RPF). RPF was interpreted as a platelet subset with metabolically greater capacity relating to multiple functional regulators when compared to mature and commonly encountered reticulated platelets (18, 41, 42). The correlation between increased concentrations of immature platelets and likewise RPF and inflammation is corroborated by multiple studies across various inflammatory conditions (43–45). The suggested pathophysiological mechanism involves increased platelet production or provision of function that is independent of megakaryocytes, referred to as emergency thrombopoiesis (ET) and is most likely driven by pro-inflammatory factors (13, 46).
The inclusion of G.Plt in this panel is predicated on their alleged heightened severity in hematological stress resulting from platelet steady-state imbalances. This is attributed to their reported superior cell functionality in terms of granulation potential and pro-thrombotic state when compared to any other platelet form (18). In this study, the size range was delineated from 12.5 to 25 μm, exhibiting cytological features similar to RPF but possessing distinct morphology and less stringent identification of autofluorescence (18, 26). Under bright-field microscopy, G.Plt can look similar to circulating endothelial progenitor cells, appearing round or WBC-like. However, G.Plt can be distinguished using the Hoechst channel, where G.Plt show dim pixel intensity in the center and a fading or irregular outline. In contrast, endothelial progenitor cells exhibit strong nuclear staining and well-defined outlines (Figure 1C; Supplementary Figure S1). G.Plt are herein considered functionally analogous to RPF, both linked to ET. Elevated or detectable levels of G.Plt are often associated with cardiovascular diseases and, less frequently, with severe hereditary conditions when found together (26, 47).
Furthermore, the CPA was incorporated into the panel to augment the analysis of platelet-related stress. It offers a sensitive “as is” indication of the coagulation status in the blood, signifying either the cause or the consequence of vascular injury. We hypothesized that the elevation of CPA serves as a real-time marker influenced by splenic and hepatic clearance, which must occur within a few hours. Therefore, persistent abnormality even at lower concentrations is suspicious of chronic lesions such as cancer or atherosclerosis or indicates impaired clearance. CPA is a well-documented distinct clot mostly containing platelets in sizes similar to WBCs and larger clots measuring up to 100 µm, which can be ascribed to apoptotic platelets following activation without shape changes, which were reported to induce clotting 50–100 times faster than normal platelets as is ascribed to the increased presence of phosphatidylserine on the platelet surface (48–50). Therefore, it is crucial to analyze CPA distinctly from fibrin microclots or leukocyte-platelet aggregates. A standardized definition for direct CPA identification is not yet established. In our study, CPA was defined as clumps comprising at least six platelets when of larger size (5 µm) or aggregates above 8 µm in diameter if containing smaller platelets. This definition significantly impacts our analysis, revealing the prevalence of CPA in healthy individuals ranging from 0 to 83 (median) or 341 (mean value) events per 5 mL. The relative rarity of CPA is supported by Nishikawa et al. (2021) who reported a mean concentration of approximately 1,200 events per 5 mL in their healthy control cohort albeit including leukocyte platelet aggregation (48).
The markers RPF and nMkc, along with their ratio and CPA, may assist in differentiating between effective aMK, ET, ineffective aMK, and ineffective MK. This has implications on the inflammation-type dominance (see Supplementary Table S4). G.Plt was excluded due to the incomplete understanding of its cell biology. Effective aMK was defined in this context as a heightened yet compensated platelet function need (normal platelet count and no CPA abnormalities) most likely driven by a pro-inflammatory milieu that leads to an elevated steady state of platelets as could be expected during infections. In our analysis, this was indicated by an RPF/nMkc ratio below 100, which signifies abnormalities in both RPF and nMkc, or only in nMkc. In this situation, Plt counts may be normal or even elevated. A platelet function deficit was inferred upon detection of ET (RPF elevation) without the indication of megakaryopoietic upregulation yielding an RPF/nMkc ratio greater than 64 and was graded as 1 for CPA <83 and graded as 2 for CPA >83 events per 5 mL. If chronic, the condition may denote a bone marrow centric pathology of suppressed MK eventually causing thrombocytopenia. Furthermore, ineffective aMK was graded as 1 for an RPF/nMkc ratio below 64 (normal RPF) and CPA >83 events per 5 mL and suggested a pro-thrombotic state with anti-inflammatory dominance preventing RPF upregulation and indicated an unmet platelet function need. Ineffective aMK graded as 2 indicated a scenario with an RPF/nMkc ratio >64 (reflecting abnormalities in both RPF and nMkc) and a CPA >83 events per 5 mL, indicating a platelet function deficit despite the upregulation of RPF and a relatively stronger pro-inflammatory state when compared to grade 1. Ineffective MK was defined here in the context of CPA abnormalities as the sole abnormality. CPA formation and resolution can occur within a few hours, while ET and aMK typically take days. Therefore, finds of ineffective MK may be rather common depending on daily activity and expecting swift resolution. However, if found, chronic immune suppression and a platelet function deficit are indicated. A summary of platelet pathologies is given in Supplementary Table S4. In the healthy cohort, eight (40%) individuals were classified as having a normal condition, five (25%) as having ineffective MK, four (20%) as having ET, two (10%) as having ineffective aMK, and one (5%) as having effective aMK.
Erythroid cell-related pathology was probed analyzing highly immature reticulocytes herein referred to as enucleated erythroblasts (enCEBs) and normoblasts herein referred to as circulating normal erythroblasts (nCEBs) (Figures 1 E–F). nCEB identification was achieved through the observation of elevated Transferrin Receptor Protein 1 (CD71) membrane signal intensity and a cell size range spanning from 6 to 25 µm, which is modulated by the cell’s maturation state and the duration of circulation. Immaturity and, thus, sensitivity to erythropoietic stress increase with cell size and CD71 fluorescence intensity. The association of nCEB with stress erythropoiesis was described elsewhere (51) as well as the association of stress erythropoiesis with inflammation (8–10, 23), thus supporting the herein proposed prediction of low-grade inflammation by the two markers. The measurement of high concentrations of nCEB in the range of several thousand cells per mL was usually applicable in severe disease (52), and low concentrations of a few cells per mL were used for fetal cell analysis in maternal blood referred to as non-invasive prenatal testing (20). A physiological baseline concentration of approximately 1.5 nCEB per 5 mL was suggested previously using a similar platform (21) and is corroborated in this work with a slightly lower median value measuring 0.6 cells per mL (t-test difference, p = 0.09). The concentration range of a few to a few hundred cells per 5 mL delineates latent normoblastemia (lNB) and denotes a measure of the so-called ineffective erythropoiesis (9, 52). Chronic lNB is a rather non-specific find, suggesting uncompensated hypoxia and can be associated with bone marrow-centric inflammation caused by disseminated cancer cells or bone marrow toxicity. It can be further associated with hormonal imbalances (e.g., testosterone insufficiency), with hematopoietic stem cell disorders, or systemic inflammation in general affecting bone marrow erythroblast differentiation and maturation.
The reticulocyte is a well-described biomarker and used in instances of improved RBC disorder monitoring (53). Under physiological conditions, its concentration range within the total RBC population is relatively high, typically comprising 1% to 3% (54). High cell immaturity may constitute 1% to 4% of the reticulocyte population, characterized by heightened CD71-positive membrane staining intensity and an increased size averaging approximately 8 µm (54). The Rarmax platform exhibited selectivity towards high CD71 staining and, additionally, towards enCEB resistant to chemical lysis, which withstands the rigorous RBC lysis procedure, as detailed in the Material and Methodology section and suggests increased cellular immaturity. Consequently, the analysis identified a normal median concentration in the healthy cohort of 6.5 cells per 5 mL. Similar to lNB, enCEB elevation or latent reticulocytosis is not diagnostic of a specific disease or condition (53). However, enCEB abnormality is highly suggestive of either effective accelerated erythropoiesis or iron-restricted ineffective erythropoiesis (11, 55). The latter may be underpinned by activation of the inflammation-related IL-6/hepcidin axis referred to as functional iron deficiency or by nutrition-related iron deficiencies referred to as absolute iron deficiency. Iron-restricted erythropoiesis may be differentiated from effective accelerated erythropoiesis by the Hgb level, with the former showing mostly normal or even higher levels and the latter showing a reduction (55). In addition, absolute iron deficiency may exclusively yield normoblast abnormality represented by erythroid abnormalities such as megaloblasts and macronormoblasts (56). Elevation in both markers nCEB and enCEB allows the prediction of latent anemia of inflammation or anemia of chronic disease (11, 57). In the healthy cohort, 15 (75%) individuals were classified as having a normal condition, 3 (15%) as having latent reticulocytosis, 1 (5%) as having lNB, and 1 (5%) as having latent anemia of inflammation.
3.2 Grading silent inflammation
The usefulness of an inflammation grading system for the herein-presented marker panel followed the idea of hematological stress burden assessment and aimed for the objective measure of response to intervention. In this vein, four grades were sought with grades 1 and 2 inferring mild disorder, without or with minor response to stress. Grade 1 would indicate cellular stress in the absence of stress response (markers: CPA and enCEB). Grades 3 and 4 implied definitive hematopoietic stress and response (markers: RPF, nMkc, and nCEB) and may bare a greater risk of disease development or detection, respectively. For better clarity, we refer to Table 2.
When applied to the healthy cohort, 50% tested negative for SI, while 15% exhibited SI grade 1, 30% exhibited grade 2, and one donor was graded as 4 (donor 7), which was explained by a history of prior illness, suggesting an incomplete recovery phase. No individuals with SI grade 3 were detected. The median and mean grades of the control cohort were 0.5 and 0.9, respectively, underscoring the prevalence of health issues in the general population (58). The SI grade did not correlate with age.
3.3 Case studies
In the following, we demonstrate the usefulness of SI analysis and grading for intervention monitoring, in an early-stage breast cancer patient before and after therapies, in an atherosclerosis patient in follow-up that committed to lifestyle changes, and in a healthy individual undergoing lifestyle changes. Both persons undergoing lifestyle changes were educated about the test results before and after the interventions. Benefits included increased health awareness and commitment to lifestyle changes as is fundamental for efficient lifestyle adaptions/corrections and the measure of objective response to various interventions as sensitive feedback.
The general blood values (Supplementary Table S2), most importantly the Hgb, RBC, and the Plt count, were within normal range in the atherosclerosis patient that reported minor health issues including occasional acid reflux and strong afternoon fatigue. The disease state was clinically characterized as stable with pro-inflammatory dominance. The person scored SI grade 4 prior to the intervention relating to the diagnosis of mild lNB, ET (when using a G.Plt 3.5× above the cutoff), and effective aMK (nMkc 2.1× above the cutoff). Improvement in scoring was sought by the lifestyle changes and demonstrated by a decrease in SI grade from 4 to 3. Profile improvement was evidenced by substantial reductions in G.Plt concentration and reduction within the normal range in reticulocyte count both regressing into physiological steady state. The diagnosis of effective aMK and mild lNB persisted similar to the pre-intervention levels. This result was further supported by the substantial reduction in circulating endothelial cell count and the cell pleomorphic factor (Table 3).
The “unhealthy” individual consented to a lifestyle modification following education on his inflammatory status. Prior health assessments, including complete blood count and lipid profile, showed no abnormalities (data not shown). Before the intervention, the SI profile was graded as 4, exhibiting a pro-inflammatory state relating to effective aMK (including elevated G.Plt), alongside lNB, and having a relatively low but normal platelet count (Table 3). Unexpectedly, the person remained unresponsive to the intervention 3 months later, maintaining an SI grade 4 and a pro-inflammatory state. Post-intervention, mild thrombocytopenia was noted, along with ineffective aMK grade 2 having measured a 3.3-fold increase in megakaryocytes, a 6.3-fold increase in RPF, a 5.2-fold increase in G.Plt, and no significant change in the lNB.
SI dynamics were longitudinally monitored in an early-stage breast cancer patient, as outlined in the Material and Methodology section, through sequential assessments conducted before treatment initiation, at 4 weeks post-surgery, during adjuvant chemotherapy, and at 2 weeks and 6 months post-treatment. Notably, no abnormalities in RBC and Ptl parameters were observed prior to surgery or during chemotherapy. The patient, enrolled in a larger liquid biopsy clinical trial, was unremarkable with respect to stage and histological type and was selected based on a decline in QoL from 90% to 70% during and following adjuvant chemotherapy, prompting further investigation into the patient’s inflammatory status. The SI was graded as 2 at baseline, indicating anemia of inflammation as well as ineffective MK. The pathologies in parallel may indicate unresolved inflammation, which could be anticipated due to cancer-associated inflammation and immune suppression, respectively. In line with the established understanding of cancer-associated inflammation, we expected to see—and did observe—a significant reduction in nCEB and enCEB to nearly normal levels in the post-surgery test (see Table 3). This indicates a reduction in pro-inflammatory cancer-related inflammation. Additionally, the suspected immune suppression prior to surgery appeared to have diminished, as indicated by an increase in RPF and a decrease in CPA, which now shows a profile suggestive of an overall pro-inflammatory dominance with SI rated as 3. The profile in the peri-ACT test graded SI as 3 and indicated a rebound to latent anemia of inflammation this time attributed to chemotherapy side effects, along with ineffective aMK grade 1, both of which are driven by pro-inflammatory activity. The SI profile in the test 2 weeks after chemotherapy was graded as 1 and verifies a good inflammatory response and recovery from the treatment. The profile continued to show latent reticulocytosis at levels comparable to the baseline test and indicated a persistent pro-inflammatory milieu. Surprisingly, the profile deteriorated despite QoL improvements and was graded as 3 after 6 months into follow-up. The profile seemed to have returned to the pre-therapy unresolved inflammatory status linked to inadequate control of platelet aggregation, despite a notable increase in RPF and G.Plt (ET grade 2), along with the reemergence of mild anemia of inflammation.
4 Discussion
Chronic low-grade inflammation signals an unhealthy state linked to faster cell aging and overall weakened health (3, 4). This inflammation is a key factor in the development and progression of diseases, increasing the risk of both latent conditions and complications. Studying inflammation-induced hematological stress through highly sensitive markers like immature platelets and RBC can reveal real-time responses to inflammation, thus predicting inflammation. Detecting these stress indicators not only aids in disease risk assessment but also promotes patient health awareness, crucial for prevention efforts. Additionally, early detection of hematological stress offers the best chance of restoring balance with minimal intervention.
While the cell markers discussed are well-documented in literature, they have not been utilized as predictive markers for low-grade inflammation, likely due to the technical difficulty of accurately detecting rare circulating cells. Our platform, tested with an LOD of one cell per 5.6 mL in spike-in experiments (data not shown), may thus lack the necessary sensitivity for all herein proposed markers. While a range of >130 cells per 5 mL was observed for RPF, G.Plt were absent in 6 of 20 control cases, suggesting their physiological concentration to fall below 0.19 cells per mL. Consequently, only mildly elevated concentrations in some markers are detectable by our assay. The prevalence of blood circulating inflammatory stress-associated rare cells under physiological conditions in concentration ranges from 0.1 to roughly 200 cells per mL (see Table 1) suggests that the physiological steady state and hematological disorder may follow an inverse relationship. In this view, compensation mechanisms are rammed up in direct correlation with increasing inflammatory imbalances rather than being switched on and off.
aMK underpins nMkc abnormality, which we believe has been overlooked as a predictor of low-grade inflammation. Instead, its utility has been demonstrated primarily for severe disease (24, 25, 59). Since the late 1950s, the cell marker has been intriguing in cancer blood analysis (27). Recently, it has drawn attention as a predictive and prognostic biomarker in cancer, particularly in conjunction with analyzing circulating endothelial and tumor cells. Bhakdi et al. (2019) dubbed them giant polyploidic cells, while Xu et al. (2017) referred to them as BigNeg using the Parsortix Liquid biopsy system (24, 25). A common ground on the concentration range under physiological conditions may not be given. Bhakdi and co-workers did not report nMkc findings in the healthy control cohort whereas the median number of nMkc using the Parsortix platform was 1 cell/7.5 mL (range, 0–15) suggesting congruence with our results in general even though our average in the healthy cohort was 1.2 per mL. The biological concentration at physiological levels may even be slightly higher taking into account older reports using simpler filtration methods reporting an average concentration of 3.6 per mL (33). Like nMkc, the elevation of highly immature platelets, labeled RPF, and most likely G.Plt strongly relates to pro-inflammatory conditions (42). However, such cell types were not used as markers to predict low-grade inflammation; thus, their mention here is presented as a new finding and consequently lacking a reference to normal physiological concentration levels.
Platelet-related aggregation encompasses various coagulation phenomena, including platelet–leukocyte aggregates (neutrophil and monocyte aggregates), endothelial microparticle aggregates, and fibrin platelet microclots (60, 61). All of those aggregate types may have different pathological underpinnings. This cell-based liquid biopsy platform facilitated in particular CPA analysis for the prediction of an increasingly pro-thrombotic status and may be superior to a similar marker analysis including D-dimer, β-thromboglobulin, plasminogen-activator inhibitor type 1, factor VII, fibrinogen, and von Willebrand factor given the visual evidence of clotting (13). Because of its relatively small size, elevated CPA levels—especially in asymptomatic health-compromised individuals—may raise the risk of developing diffuse microthrombi in peripheral capillaries and arterioles. Conditions of reduced phagocytic capacity resulting from trauma or chemotherapy would exacerbate this risk, potentially leading to small vessel occlusion, tissue ischemia, and, in the worst case, thrombotic microangiopathy. Direct microscopic measurement of such clots has been challenging and hindered accurate estimation of the concentration at steady state. Nonetheless, studies investigating platelet aggregation directly may support our observation of their relative rarity (48, 62). Certainty regarding the in vivo formation of stable CPA is crucial for the interpretation of chronic coagulopathy, as is perhaps evidenced by the significant difference between control and early-stage cancer cohorts (Supplementary Table S3, t-test difference: p = 0.025). Additionally, in the breast cancer case example BR59, CPA concentration levels displayed consistency. The in vivo formation is further suggested by the role of platelet binding during and after apoptosis, highlighting a clotting mechanism that differs from the disaggregation of clots during wound healing. However, the possibility of unstable platelet aggregates cannot be discounted, and all relevant influences on platelet kinetics, including physical activity (50), should be considered in causal determinations.
Utilizing the CPA, RPF, G.Plt, nMkc, and the ratio of RPF/nMkc can help distinguish between central and peripheral platelet disorders in the context of thrombocytopenia. A bone marrow-centric disorder could be influenced by factors like disseminated tumor cells, drugs, or systemic inflammation leading to MK suppression (nMkc <13) and yielding an RPF/nMkc ratio >33 (RPF >400 cells per 5 mL). Peripheral platelet disorders, on the other hand, may involve excessive platelet consumption leading to effective or ineffective aMK (nMK >12). In application, only the unhealthy patient showed mild thrombocytopenia. The post-intervention test was characteristic of ineffective aMK, hence indicating a potential peripheral platelet disorder.
Paulson and colleagues have provided evidence supporting extramedullary erythropoiesis as a compensatory mechanism for inflammation-induced stress hematopoiesis (8, 9). This suggests that tissues outside the bone marrow, like the spleen, may harbor erythrocyte-committed stem cells and release more erythroblasts in the circulation ascribed to a reduced barrier function when compared to bone marrow endothelium, which is particularly evident in acute or severe chronic inflammation (63). The Rarmax platform targets the rarest known form of reticulocytes, identified as the largest cells measuring approximately 8 µm on average with high CD71 fluorescence staining (54). Concentrations of the absolute reticulocyte count in healthy individuals were reported to range from approximately 0.5% to 1.1% of RBC (54, 64, 65). The most immature fraction of reticulocytes may constitute 3% to 5% of the immature fraction (54), which is still an extremely high amount of CD71+ reticulocytes that would amount to approximately 8×106 cells per 5 mL and renders our discovery of extremely low cell counts perplexing. This anomaly can be attributed to the pre-enrichment process involving an intense protocol for removing RBCs, reducing their numbers by nearly 6 logs. Consequently, our platform exhibits bias, favoring the selection of reticulocytes resistant to ammonium chloride buffered lysis. It is a well-established fact that reticulocytes, particularly when immature, demonstrate greater resistance to osmotic lysis due to their lower osmotic fragility (66).
We expected and demonstrated the capacity of our approach to prospectively measure hematological disorder as could be expected in a cancer patient due to the disease process itself, to the treatment, and to complications of therapy (11, 67–70). The initial baseline inflammatory condition in the patient BR59 may be common and was explained by an upregulation of pro-inflammatory factors like IL-1β, IL-6, TNF-α, and IFN-γ. IL-6 prompts the liver to produce hepcidin, leading to restricted iron availability for erythropoiesis. TNF-α inhibits erythropoiesis indirectly by hindering cell regeneration and directly by impeding EPO Hif activity, reducing EPO signaling and causing ineffective erythropoiesis (55). IL-1 and IFN-γ would contribute to myeloid skewing, resulting in ineffective erythropoiesis but also ET, which was not indicated in the SI profile arguing for IL-6 and TNF-α as major players. Additionally, the cancer patient exhibited a pro-thrombotic state as indicated by CPA elevation and could be explained by the presence of a cancer-associated wound (69) and/or tumor–cell interaction with platelets, potentially via tumor-derived microparticles (71, 72). Elevated CPA levels pose a risk or are a consequence of vascular injury. Indeed, a correlation between CPA and CEC may be exemplified in the patient who revealed predominantly small cells of a similar type, suggesting origin from small vessels and further supporting the risk of microvascular occlusion. Evidence supporting cancer-associated inflammation was confirmed by the remission of SI markers 4 weeks post-surgery. This remission may also predict a reduced risk of anemia during chemotherapy (71, 72. Despite the complete tumor resection, CPA and enCEB remained elevated and suggested a residual pro-inflammatory and pro-thrombotic influence independent of tumor presence. The de novo emergence of ET (grade 2) and the reduction in CPA following surgery suggest a remission of immune suppression and a shift in the inflammatory balance toward a pro-inflammatory state. This may indicate a prolonged recovery from the surgical wound, which was resolved during and shortly after chemotherapy. The patient commenced adjuvant chemotherapy with an SI graded as 3. Delayed initiation of chemotherapy might have allowed for disorder alleviation and a more balanced status after a few weeks, potentially enhancing resilience to chemotherapy. The peak reticulocytosis value, compared to other measurements, is an anticipated consequence of chemotherapy and is often linked to chemotherapy-induced iron-restricted erythropoiesis, especially in the context of the significant drop in hemoglobin from 13.4 to 12.7 mg/dL. However, chemotherapy-induced eryptosis may also play a contributory role (73). Bone marrow suppression of MK was not indicated in the patient as evidenced by the emergence of mild aMK. Increased vascular injury in the peri-adjuvant chemotherapy test was detected, supporting our prediction of an elevated risk of microvessel damage in the presence of CPA elevation and upon heightened splenic and hepatic burdening associated with chemotherapy. The latter finding may correlate with a decrease in QoL. Accordingly, the damage regressed to some extent in the test after chemotherapy completion. The test 2 weeks after chemotherapy showed an overall good response to therapy with respect to residual cancer inflammation taking into account CPA and nCEB as well as an overall amelioration of chemotherapy side effects taking into account regression in CEC and enCEB counts now scoring the SI grade as 1. The profile abnormality worsened in the test 6 months after. In the context of symptom absence, a QoL report scoring 80%, along with platelet levels within normal range and low baseline WBC concentrations, a low probability of acute inflammation was suggested. Therefore, this worsening could be considered as “SI in progression”. In general, this finding might be explained by long-term side effects (74). However, the similarity between pre- and post-therapy abnormalities related to the inflammatory state indicated the presence of residual cancer pathology, particularly concerning a wound that commonly involves thrombotic stress and vascular injury. In this vein, SI detection warrants the investigation as an alternative tool for relapse prediction when compared to the analysis of circulating tumor cells often showing negative results until a few months or weeks before the clinical detectable recurrence (75, 76). It then remains an unresolved issue whether to pursue treatment or adopt a conservative approach for the patient, given the exhaustion of available cancer therapy options. Anti-inflammatory treatment/intervention may offer potential benefits to the patient irrespective of the underlying circumstances.
Atherosclerosis is not considered curable in the conventional sense and managed by preventing the progression. Lifestyle modifications were shown to be beneficial to positively influence inflammation profiles and endothelial dysfunction (77). The SI in the test person afflicted with atherosclerosis prior to the intervention was graded as 4 due to abnormal elevations in G.Plt, nMkc, and mild elevation in nCEB. The markedly abnormal CEC profile could be expected and was used to gauge improvement. From previous reports that investigated lifestyle interventions, we could also expect decreases in IL-6-dominated inflammation and, to a lower extent, in TNF-α levels (46, 77). These expectations were corroborated in the significant reduction of enCEB after the intervention, the persistence of nCEB and aMK, and a substantial amelioration in vascular injury. The significant reduction in G.Plt count was unexpected and may be supported by the alleviation of vascular injury severity. An overall improvement of SI grade 4 to 3 was documented. The lipid profile revealed no significant changes but rather a slight uptick in cholesterol, yet within range of normality that we ascribe to the reduction of statin medication. The relative clump-free blood picture in both tests was ascribed to the aspirin treatment. In line with previous communications to treat atherosclerosis by focusing on inflammation, long-term strict lifestyle adaptations achieving a health benefiting routine may be advisable holding the promise of disease remission (78).
In contrast to the atherosclerosis patient, the unhealthy individual did not respond to the lifestyle intervention. Instead, the profile maintained a high SI grade, was characteristic of mild thrombocytopenia, and experienced a significant increase in stress related to MK and thrombopoiesis. This refractory response suggests the likelihood of an underlying disorder. In the absence of other signs of inflammation and with a decreased platelet steady state, this could be interpreted as a TNF-α imbalance and may indicate the presence of a latent anti-platelet autoimmune disorder (79).
The proposed rare cell panel may represent what could be called holistic rare cell analysis, based on understanding single-cell status within the broader context of abnormalities. However, we acknowledge that our knowledge of individual cell types and patient-specific cells is still in its infancy, which limits pathological interpretations. For instance, the function of G.Plt remains unclear regarding its role in anticoagulation, pro-thrombotic activity, and nitric oxide production and as an inflammatory trigger. Additionally, determining the origins of normoblasts and endothelial cells, as well as assessing ploidy in nMkc, would be highly beneficial.
In conclusion, we have introduced a novel panel of rare cell-based markers aimed at detecting and characterizing low-grade inflammation based on the current knowledge of pathophysiology, which aids in the differential diagnosis of inflammatory conditions in asymptomatic individuals and highly sensitive monitoring of health interventions. The study validated the premise of heightened sensitivity to low-grade inflammation-induced hematopoietic stress by examining highly immature rare RBCs and platelets. Our study showcased advancements in detecting cell immaturity, particularly in relation to circulating megakaryocytes, reticulated platelets, and reticulocytes. Conventional hematological indices would remain normal or inconclusive, hence having used the term silent inflammation for low-grade inflammation. We determined abnormality thresholds through analysis of a healthy control cohort, which, in contrast to complete blood count, effectively differentiated between pre- and post-intervention statuses. Additionally, we found that monitoring CPAs was beneficial in assessing treatment response in an early-stage cancer patient. Considering all these markers collectively, the detection of SI warrants further investigation as a surrogate marker panel for evaluating treatment response objectively and predicting the likelihood of recurrence in cancer survivors.
Data availability statement
The original contributions presented in the study are included in the article/Supplementary Material. Further inquiries can be directed to the corresponding author.
Ethics statement
The study is part the project that titles “Advancing cell-based liquid biopsy” and was approved by the Mahidol University Central IRB, Mahidol University with protocol number 2019/197.3007 as well as by Research Ethics Committee of Ramathibodi Hospital (protocol ID 02-65-01) for testing breast cancer patient blood.The studies involving humans were approved by Research Ethics Committee of Ramathibodi Hospital Mahidol University Central IRB, Mahidol University. The studies were conducted in accordance with the local legislation and institutional requirements. The participants provided their written informed consent to participate in this study.
Author contributions
SS: Conceptualization, Data curation, Formal analysis, Investigation, Methodology, Project administration, Writing – original draft, Writing – review & editing. PB: Data curation, Investigation, Methodology, Writing – original draft. SB: Conceptualization, Data curation, Funding acquisition, Project administration, Writing – review & editing. PL: Data curation, Investigation, Project administration, Resources, Writing – review & editing. WT: Project administration, Supervision, Writing – review & editing.
Funding
The author(s) declare that no financial support was received for the research, authorship, and/or publication of this article.
Conflict of interest
Authors SS, PB, SB were employed by the company Premise Biosystems Co., Ltd. The corresponding author and SB are shareholders of a company involved in the development and manufacturing of cell separation technology and biomarkers as was employed in this work.
The remaining authors declare that the research was conducted in the absence of any commercial or financial relationships that could be construed as a potential conflict of interest.
Publisher’s note
All claims expressed in this article are solely those of the authors and do not necessarily represent those of their affiliated organizations, or those of the publisher, the editors and the reviewers. Any product that may be evaluated in this article, or claim that may be made by its manufacturer, is not guaranteed or endorsed by the publisher.
Supplementary material
The Supplementary Material for this article can be found online at: https://www.frontiersin.org/articles/10.3389/frhem.2024.1472609/full#supplementary-material
References
2. Bengmark S. Acute and “chronic” phase reaction—a mother of disease. Clin Nutr. (2004) 23:1256–66. doi: 10.1016/j.clnu.2004.07.016
3. Franceschi C, Garagnani P, Vitale G, Capri M, Salvioli S. Inflammaging and ‘Garb-aging’. Trends Endocrinol Metab. (2017) 28:199–212. doi: 10.1016/j.tem.2016.09.005
4. Baechle JJ, Chen N, Makhijani P, Winer S, Furman D, Winer DA. Chronic inflammation and the hallmarks of aging. Mol Metab. (2023) 74:101755. doi: 10.1016/j.molmet.2023.101755
5. Osborn O, Olefsky JM. The cellular and signaling networks linking the immune system and metabolism in disease. Nat Med. (2012) 18:363–74. doi: 10.1038/nm.2627
6. Minihane AM, Vinoy S, Russell WR, Baka A, Roche HM, Tuohy KM, et al. Low-grade inflammation, diet composition and health: current research evidence and its translation. Br J Nutr. (2015) 114:999–1012. doi: 10.1017/S0007114515002093
7. Kelley-Hedgepeth A, Lloyd-Jones DM, Colvin A, Matthews KA, Johnston J, Sowers MR, et al. Ethnic differences in C-reactive protein concentrations. Clin Chem. (2008) 54:1027–37. doi: 10.1373/clinchem.2007.098996
8. Paulson RF, Hariharan S, Little JA. Stress erythropoiesis: definitions and models for its study. Exp Hematol. (2020) 89:43–54. doi: 10.1016/j.exphem.2020.07.011
9. Paulson RF, Ruan B, Hao S, Chen Y. Stress erythropoiesis is a key inflammatory response. Cells. (2020) 9:634. doi: 10.3390/cells9030634
10. Bennett LF, Liao C, Quickel MD, Yeoh BS, Vijay-Kumar M, Hankey-Giblin P, et al. Inflammation induces stress erythropoiesis through heme-dependent activation of SPI-C. Sci Signal. (2019) 12:eaap7336. doi: 10.1126/scisignal.aap7336
11. Weiss G, Ganz T, Goodnough LT. Anemia of inflammation. Blood J Am Soc Hematol. (2019) 133:40–50. doi: 10.1182/blood-2018-06-856500
12. Bousounis P, Bergo V, Trompouki E. Inflammation, aging and hematopoiesis: A complex relationship. Cells. (2021) 10:1386. doi: 10.3390/cells10061386
13. Nishimura S, Nagasaki M, Kunishima S, Sawaguchi A, Sakata A, Sakaguchi H, et al. IL-1α induces thrombopoiesis through megakaryocyte rupture in response to acute platelet needs. J Cell Biol. (2015) 209:453–66. doi: 10.1083/jcb.201410052
14. Kaser A, Brandacher G, Steurer W, Kaser S, Offner FA, Zoller H, et al. Interleukin-6 stimulates thrombopoiesis through thrombopoietin: role in inflammatory thrombocytosis. Blood. (2001) 98:2720–5. doi: 10.1182/blood.V98.9.2720
15. Machlus KR, Johnson KE, Kulenthirarajan R, Forward JA, Tippy MD, Soussou TS, et al. CCL5 derived from platelets increases megakaryocyte proplatelet formation. Blood. (2016) 127:921–6. doi: 10.1182/blood-2015-05-644583
16. Nazarov K, Perik-Zavodskii R, Perik-Zavodskaia O, Alrhmoun S, Volynets M, Shevchenko J, et al. Phenotypic alterations in erythroid nucleated cells of spleen and bone marrow in acute hypoxia. Cells. (2023) 12:2810. doi: 10.3390/cells12242810
17. Sprague AH, Khalil RA. Inflammatory cytokines in vascular dysfunction and vascular disease. Biochem Pharmacol. (2009) 78:539–52. doi: 10.1016/j.bcp.2009.04.029
18. Handtke S, Thiele T. Large and small platelets—(When) do they differ? . J Thromb Haemostasis. (2020) 18:1256–67. doi: 10.1111/jth.14788
19. D’Souza E, Ghosh K, Colah R. A comparison of the choice of monoclonal antibodies for recovery of fetal cells from maternal blood using FACS for noninvasive prenatal diagnosis of hemoglobinopathies. Cytometry Part B: Clin Cytometry: J Int Soc Analytical Cytol. (2009) 76:175–80. doi: 10.1002/cyto.b.v76b:3
20. Collarini EJ, Cain CA, Gammon D, Harriman B, Magee K, Du G, et al. Comparison of methods for erythroblast selection: application to selecting fetal erythroblasts from maternal blood. Cytometry: J Int Soc Analytical Cytol. (2001) 45:267–76. doi: 10.1002/1097-0320(20011201)45:4<267::AID-CYTO10023>3.0.CO;2-D
21. Schreier S, Borwornpinyo S, Udomsangpetch R, Triampo W. An update of circulating rare cell types in healthy adult peripheral blood: findings of immature erythroid precursors. Ann Trans Med. (2018) 6(20):406. doi: 10.21037/atm.2018.10.04
22. Allard WJ, Matera J, Miller MC, Repollet M, Connelly MC, Rao C, et al. Tumor cells circulate in the peripheral blood of all major carcinomas but not in healthy subjects or patients with nonmalignant diseases. Clin Cancer Res. (2004) 10:6897–904. doi: 10.1158/1078-0432.CCR-04-0378
23. Schreier S, Budchart P, Borwornpinyo S, Arpornwirat W, Triampo W. Circulating erythroblast abnormality associated with systemic pathologies may indicate bone marrow damage. J Circulating Biomarkers. (2021) 10:145. doi: 10.33393/jcb.2021.2220
24. Xu L, Mao X, Guo T, Chan PY, Shaw G, Hines J, et al. The novel association of circulating tumor cells and circulating megakaryocytes with prostate cancer prognosis. Clin Cancer Res. (2017) 23:5112–22. doi: 10.1158/1078-0432.CCR-16-3081
25. Bhakdi SC, Suriyaphol P, Thaicharoen P, Grote ST, Komoltri C, Chaiyaprasithi B, et al. Accuracy of tumour-associated circulating endothelial cells as a screening biomarker for clinically significant prostate cancer. Cancers. (2019) 11:1064. doi: 10.3390/cancers11081064
27. Melamed MR, Cliton EE, Mercer C, Koss LG. The megakaryocyte blood count. Am J Med Sci. (1966) 252:301. doi: 10.1097/00000441-196609000-00009
28. Erdbruegger U, Dhaygude A, Haubitz M, Woywodt A. Circulating endothelial cells: markers and mediators of vascular damage. Curr Stem Cell Res Ther. (2010) 5:294–302. doi: 10.2174/157488810793351721
29. Schreier S, Wannapong T. Systemic cytology. A novel diagnostic approach for assessment of early systemic disease. Med Hypotheses. (2021) 156:110682. doi: 10.1016/j.mehy.2021.110682
30. Kaushansky K. Determinants of platelet number and regulation of thrombopoiesis. In: ASH Education Program Book, vol. 1. (Washington, DC: American Society of Hematology) (2009). p. 147–52.
31. Couldwell G, Machlus KR. Modulation of megakaryopoiesis and platelet production during inflammation. Thromb Res. (2019) 179:114–20. doi: 10.1016/j.thromres.2019.05.008
32. Behrens K, Alexander WS. Cytokine control of megakaryopoiesis. Growth Factors. (2018) 36:89–103. doi: 10.1080/08977194.2018.1498487
33. Breslow A, Kaufman RM, Lawsky AR. The effect of surgery on the concentration of circulating megakaryocytes and platelets. Blood. (1968) 32:393–401. doi: 10.1182/blood.V32.3.393.393
34. Mattia G, Vulcano F, Milazzo L, Barca A, Macioce G, Giampaolo A, et al. Dierent ploidy levels of megakaryocytes generated from peripheral or cord blood CD34+ cells are correlated with dierent levels of platelet release. Blood. (2002) 99:888–97. doi: 10.1182/blood.V99.3.888
35. Machlus KR, Italiano JE Jr. The incredible journey: From megakaryocyte development to platelet formation. J Cell Biol. (2013) 201:785–96. doi: 10.1083/jcb.201304054
36. Mazzi S, Lordier L, Debili N, Raslova H, Vainchenker W. Megakaryocyte and polyploidization. Exp Hematol. (2018) 57:1–135. doi: 10.1016/j.exphem.2017.10.001
37. Haas S, Hansson J, Klimmeck D, Loeffler D, Velten L, Uckelmann H, et al. Inflammation-induced emergency megakaryopoiesis driven by hematopoietic stem cell-like megakaryocyte progenitors. Cell Stem Cell. (2015) 17:422–34. doi: 10.1016/j.stem.2015.07.007
38. Shrirao AB, Schloss RS, Fritz Z, Shrirao MV, Rosen R, Yarmush ML. Autofluorescence of blood and its application in biomedical and clinical research. Biotechnol Bioengineering. (2021) 118:4550–76. doi: 10.1002/bit.v118.12
39. Kolenc OI, Quinn KP. Evaluating cell metabolism through autofluorescence imaging of NAD (P) H and FAD. Antioxid Redox Signaling. (2019) 30:875–89. doi: 10.1089/ars.2017.7451
40. Bartolomé F, Abramov AY. Measurement of mitochondrial NADH and FAD autofluorescence in live cells. In: Mitochondrial Medicine: Volume I, Probing Mitochondrial Function. (New York, NY: Humana Press) (2015). p. 263–70.
41. Margraf A, Zarbock A. Platelets in inflammation and resolution. J Immunol. (2019) 203:2357–67. doi: 10.4049/jimmunol.1900899
42. Hamad MA, Krauel K, Schanze N, Gauchel N, Stachon P, Nuehrenberg T, et al. Platelet subtypes in inflammatory settings. Front Cardiovasc Med. (2022) 9:8235495. doi: 10.3389/fcvm.2022.823549
43. Cesari F, Marcucci R, Gori AM, Caporale R, Fanelli A, Casola G, et al. Reticulated platelets predict cardiovascular death in acute coronary syndrome patients. Thromb Haemostasis. (2013) 109:846–53. doi: 10.1160/TH12-09-0709
44. Corpataux N, Franke K, Kille A, Valina CM, Neumann FJ, Nührenberg T, et al. Reticulated platelets in medicine: current evidence and further perspectives. J Clin Med. (2020) 9:3737. doi: 10.3390/jcm9113737
45. Liu QH, Song MY, Yang BX, Xia RX. Clinical significance of measuring reticulated platelets in infectious diseases. Medicine. (2017) 96:e9424. doi: 10.1097/MD.0000000000009424
46. Malik A, Kanneganti T-D. Function and regulation of IL-1α in inflammatory diseases and cancer. Immunol Rev. (2018) 281:124–37. doi: 10.1111/imr.2018.281.issue-1
47. Kato K, Martinez C, Russell S, Nurden P, Nurden A, Fiering S, et al. Genetic deletion of mouse platelet glycoprotein Ibbeta pro-duces a Bernard-Soulier phenotype with increased alpha-granule size. Blood. (2004) 104:2339–44. doi: 10.1182/blood-2004-03-1127
48. Nishikawa M, Kanno H, Zhou Y, Xiao TH, Suzuki T, Ibayashi Y, et al. Massive image-based single-cell profiling reveals high levels of circulating platelet aggregates in patients with COVID-19. Nat Commun. (2021) 12:7135. doi: 10.1038/s41467-021-27378-2
49. Maurer-Spurej E, Devine DV. Platelet aggregation is not initiated by platelet shape change. Lab Invest. (2001) 81:1517–25. doi: 10.1038/labinvest.3780365
50. Kumpuris AG, Luchi RJ, Waddell CC, Miller RR. Production of circulating platelet aggregates by exercise in coronary patients. Circulation. (1980) 61:62–5. doi: 10.1161/01.CIR.61.1.62
51. Constantino BT, Cogionis B. Nucleated RBCs—significance in the peripheral blood film. Lab Med. (2000) 31:223–9. doi: 10.1309/D70F-HCC1-XX1T-4ETE
53. Adane T, Asrie FGZ, Getaneh Z. Clinical utility of immature reticulocyte fraction. J Clin Chem Lab Med. (2021) 4(9):1000p172.
54. Malleret B, Xu F, Mohandas N, Suwanarusk R, Chu C, Leite JA, et al. Significant biochemical, biophysical and metabolic diversity in circulating human cord blood reticulocytes. PloS One. (2013) 8:e76062. doi: 10.1371/journal.pone.0076062
55. Tanno T, Miller JL. Iron loading and overloading due to ineffective erythropoiesis. Adv Hematol. (2010) 1:358283. doi: 10.1155/2010/358283
56. Aslinia F, Mazza JJ, Yale SH. Megaloblastic anemia and other causes of macrocytosis. Clin Med Res. (2006) 4:236–41. doi: 10.3121/cmr.4.3.236
57. Camaschella C, Nai A. Ineffective erythropoiesis and regulation of iron status in iron loading anaemias. Br J Haematol. (2016) 172:512–23. doi: 10.1111/bjh.2016.172.issue-4
58. Blair SN. Physical inactivity: the biggest public health problem of the 21st century. Br J Sports Med. (2009) 43:1–2.
59. Briere J, Kiladjian JJ. E Peynaud-Debayle 4 Megakaryocytes and platelets in myeloproliferative disorders. Baillière’s Clin Haematol. (1997) 10:65–88. doi: 10.1016/S0950-3536(97)80051-0
60. Gerrits AJ, Frelinger AL III, Michelson AD. Whole blood analysis of leukocyte-platelet aggregates. Curr Protoc Cytometry. (2016) 78:6–15. doi: 10.1002/0471142956.2016.78.issue-1
61. Héloire F, Weill B, Weber S, Batteux F. Aggregates of endothelial microparticles and platelets circulate in peripheral blood. Variations during stable coronary disease and acute myocardial infarction. Thromb Res. (2003) 110:173–80. doi: 10.1016/S0049-3848(03)00297-4
62. Hermann M, Lisch C, Gerth R, Wick G, Fries D, Wick N. Circulating microaggregates as biomarkers for the Post-COVID syndrome. IDCases. (2024) 24:e02000. doi: 10.1016/j.idcr.2024.e02000
63. Alamo IG, Kannan KB, Loftus TJ, Ramos H, Efron PA, Mohr AM. Severe trauma and chronic stress activates extramedullary erythropoiesis. J Trauma Acute Care Surg. (2017) 83:144–50. doi: 10.1097/TA.0000000000001537
64. Kono M, Kondo T, Takagi Y, Wada A, Fujimoto K. Morphological definition of CD71 positive reticulocytes by various staining techniques and electron microscopy compared to reticulocytes detected by an automated hematology analyzer. Clin Chim Acta. (2009) 404:105–10. doi: 10.1016/j.cca.2009.03.017
65. Serke S, Dieter H. Identification of CD71 (transferrin receptor) expressing erythrocytes by multiparameter-flow-cytometry (MP-FCM): correlation to the quantitation of reticulocytes as determined by conventional microscopy and by MP-FCM using a RNA-staining dye. Br J Haematol. (1992) 81:432–9. doi: 10.1111/j.1365-2141.1992.tb08252.x
66. Marks PA, Johnson AB. Relationship between the age of human erythrocytes and their osmotic resistance: a basis for separating young and old erythrocytes. J Clin Invest. (1958) 37:1542–8. doi: 10.1172/JCI103746
67. Ludwig H, Van Belle S, Barrett-Lee P, Birgegård G, Bokemeyer C, Gascón P, et al. The european cancer anaemia survey (ECAS): a large, multinational, prospective survey defining the prevalence, incidence, and treatment of anaemia in cancer patients. Eur J Cancer. (2004) 40:2293–306. doi: 10.1016/j.ejca.2004.06.019
68. Gaspar BL, Sharma P, Das R. Anemia in Malignancies: pathogenetic and diagnostic considerations. Hematology. (2015) 20:18–25. doi: 10.1179/1607845414Y.0000000161
69. Manjili SH, Isbell M, Ghochaghi N, Perkinson T, Manjili MH. Multifaceted functions of chronic inflammation in regulating tumor dormancy and relapse. In: Seminars in cancer biology, vol. 78. Amsterdam, Netherlands: Academic Press (2022).
70. Porta C, Larghi P, Rimoldi M, Totaro MG, Allavena P, Mantovani A, et al. Cellular and molecular pathways linking inflammation and cancer. Immunobiology. (2009) 214:761–77. doi: 10.1016/j.imbio.2009.06.014
71. van Es N, Sturk A, Middeldorp S, Nieuwland R. Effects of cancer on platelets. In: Seminars in oncology, vol. 41. Amsterdam, Netherlands: WB Saunders (2014).
72. Tesselaar ME, Romijn FP, Van Der Linden IK, Prins FA, Bertina RM, Osanto S. Microparticle-associated tissue factor activity: a link between cancer and thrombosis? J Thromb Haemost. (2007) 5:520–7. doi: 10.1111/j.1538-7836.2007.02369.x
73. Durigova A, Lamy PJ, Thezenas S, Pouderoux S, Montels F, Romieu G, et al. Anemia and iron biomarkers in patients with early breast cancer. Diagnostic value of hepcidin and soluble transferrin receptor quantification. Clin Chem Lab Med. (2013) 51:1833–41. doi: 10.1515/cclm-2013-0031
74. Deleemans JM, Chleilat F, Reimer RA, Henning JW, Baydoun M, Piedalue KA, et al. The chemo-gut study: Investigating the long-term effects of chemotherapy on gut microbiota, metabolic, immune, psychological and cognitive parameters in young adult Cancer survivors; study protocol. BMC Cancer. (2019) 19:1–11. doi: 10.1186/s12885-019-6473-8
75. Radovich M, Jiang G, Hancock BA, Chitambar C, Nanda R, Falkson C, et al. Association of circulating tumor DNA and circulating tumor cells after neoadjuvant chemotherapy with disease recurrence in patients with triple-negative breast cancer: preplanned secondary analysis of the BRE12-158 randomized clinical trial. JAMA Oncol. (2020) 6:1410–5. doi: 10.1001/jamaoncol.2020.2295
76. Si H, Esquivel M, Mendoza Mendoza E, Roarty K. The covert symphony: cellular and molecular accomplices in breast cancer metastasis. Front Cell Dev Biol. (2023) 11:12217845. doi: 10.3389/fcell.2023.1221784
77. Dod HS, Bhardwaj R, Sajja V, Weidner G, Hobbs GR, Konat GW, et al. Effect of intensive lifestyle changes on endothelial function and on inflammatory markers of atherosclerosis. Am J Cardiol. (2010) 105:362–7. doi: 10.1016/j.amjcard.2009.09.038
78. Ridker PM, Everett BM, Thuren T, MacFadyen JG, Chang WH, Ballantyne C, et al. Antiinflammatory therapy with canakinumab for atherosclerotic disease. New Engl J Med. (2017) 377:1119–31. doi: 10.1056/NEJMoa1707914
Keywords: liquid biopsy, rare cells, circulating endothelial cells, normoblast, inflammation, hematopoiesis
Citation: Schreier S, Budchart P, Borwornpinyo S, Lertsithichai P and Triampo W (2024) Rare cell-based liquid biopsy for improved measurement of low-grade chronic inflammation. Front. Hematol. 3:1472609. doi: 10.3389/frhem.2024.1472609
Received: 29 July 2024; Accepted: 28 October 2024;
Published: 02 December 2024.
Edited by:
Robert F. Paulson, The Pennsylvania State University (PSU), United StatesReviewed by:
Tatyana Grinenko, Shanghai Jiao Tong University, ChinaMohamed Essameldin Abdelgawad, Wake Forest University, United States
Copyright © 2024 Schreier, Budchart, Borwornpinyo, Lertsithichai and Triampo. This is an open-access article distributed under the terms of the Creative Commons Attribution License (CC BY). The use, distribution or reproduction in other forums is permitted, provided the original author(s) and the copyright owner(s) are credited and that the original publication in this journal is cited, in accordance with accepted academic practice. No use, distribution or reproduction is permitted which does not comply with these terms.
*Correspondence: Wannapong Triampo, d3RyaWFtcG9AZ21haWwuY29t