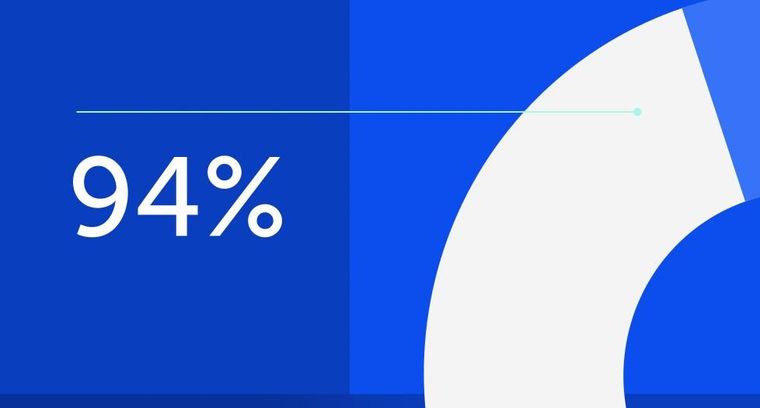
94% of researchers rate our articles as excellent or good
Learn more about the work of our research integrity team to safeguard the quality of each article we publish.
Find out more
REVIEW article
Front. Hematol., 02 February 2024
Sec. Blood Cancer
Volume 3 - 2024 | https://doi.org/10.3389/frhem.2024.1331109
This article is part of the Research TopicArtificial Intelligence in Hematology: Applications from Drug Design to Precision MedicineView all 4 articles
Multiple myeloma is the second most frequent hematologic malignancy worldwide with high morbidity and mortality. Although it is considered an incurable disease, the enhanced understanding of this neoplasm has led to new treatments, which have improved patients’ life expectancy. Large amounts of data have been generated through different studies in the settings of clinical trials, prospective registries, and real-world cohorts, which have incorporated laboratory tests, flow cytometry, molecular markers, cytogenetics, diagnostic images, and therapy into routine clinical practice. In this review, we described how these data can be processed and analyzed using different models of artificial intelligence, aiming to improve accuracy and translate into clinical benefit, allow a substantial improvement in early diagnosis and response evaluation, speed up analyses, reduce labor-intensive process prone to operator bias, and evaluate a greater number of parameters that provide more precise information. Furthermore, we identified how artificial intelligence has allowed the development of integrated models that predict response to therapy and the probability of achieving undetectable measurable residual disease, progression-free survival, and overall survival leading to better clinical decisions, with the potential to inform on personalized therapy, which could improve patients’ outcomes. Overall, artificial intelligence has the potential to revolutionize multiple myeloma care, being necessary to validate in prospective clinical cohorts and develop models to incorporate into routine daily clinical practice.
Multiple myeloma (MM) is the second most frequent hematologic malignancy (1) worldwide with high morbidity and mortality. It presents with bone lytic lesions, anemia, impaired renal function, and hypercalcemia. Although there have been significant advances in the knowledge (2) and treatment (3–5) of this neoplasm, it is still an incurable disease (6).
Large amounts of data have been generated in clinical trials, registries, and real-world cohorts, which can be processed and analyzed using artificial intelligence (AI) algorithms (3). These are integrative approaches that use individual data from datasets and can suggest the optimal therapy for a specific patient, as has been demonstrated in other models of neoplastic (7–9) or non-neoplastic diseases (10, 11).
In this review, we describe how AI models are changing the landscape of MM care, with potential applications of AI during the diagnosis, prognosis, prediction response, and optimal therapy selection. These applications leverage the different types of data often already available as part of the standard of care, such as from laboratory tests, hematopathology [including flow cytometry (FC)], diagnostic images, genomics, and clinical data. Overall, AI has the potential to impact the MM field both directly through improvement in diagnosis or treatment and indirectly through workflow optimization and potentially lower cost (12).
However, although AI is a promising evolving field, it is imperative to ensure that AI applications are effective and have appropriate validation and implementation in the daily clinical care of patients with MM, as the use of AI applications is still at an early stage despite its great potential (12).
AI can be systems, tools, or algorithms that can be trained from data to think, learn, and imitate human intelligence to solve specific problems and perform functions in the real world (13). AI uses various algorithms derived from machine learning (ML) or deep learning (DL).
ML functions by training algorithms on relevant data. It gathers data, interprets them, and makes decisions based on previous and present experiences (14). To measure its performance, different metrics are used, with area under the curve (AUC) being one of the most common. AUC represents the performance of the model at separating classes and accuracy under the receiver operating characteristics (AUROC) related to the model’s overall correctness (13). There are different learning methods in ML: supervised (SL), unsupervised (UL), and reinforcement learning (RL) (Figure 1).
Figure 1 Schematic representation of the subtypes of machine learning (ML) and deep learning (DL). Supervised ML models rely on labeled data and are useful for classification problems, as they are relatively easy to train using data labeling. In contrast, unsupervised ML models do not require labeled data and rely on methods to extract characteristics without requiring direct guidance. Reinforcement ML models rely on a system of reward/punishment feedback based on a series of inputs and outcomes. Several DL structures exist, and each can be leveraged for different purposes and rely on different data structures. Figure created in BioRender.com.
SL learns from data that have been labeled previously, assuming a correct classification. As part of the training process, the model compares its prediction to the ground truth and adjusts to increase the likelihood of a correct prediction in future iterations. Once trained, the system can infer labels on similar (unlabeled) input data. SL includes multi-instance, transductive, active, meta, and multi-task learning styles where the input data are labeled and classified, followed by regression and prediction (14) on these labeled datasets. SL provides more accurate results when used for classification. SL models are simple to train and test using labeled datasets (14).
UL includes self-supervised, constructive, and association rule learning styles. It works on unlabeled data objects. Anomaly detection, clustering, density estimation, feature learning, dimensionality reduction, and association rule discovery are some of the UL tasks (14). It is used for feature extraction, spotting important patterns and structures, and matching together related objects (14).
RL consists of an agent that is trained in a dynamic environment and learns autonomously by trial and error. The agent decides what to do to perform a task and assesses the outcome of such input based on a set endpoint. RL uses no training dataset, so it learns based on the feedback from its experiences (output), minimizing inappropriate actions and maximizing appropriate ones for receiving rewards for expected returns and punishment for unsuccessful attempts (14). The reward and punishment are mathematical functions that assign a numerical value to each state or action of the agent. They measure the goodness of a particular action in a particular state. The reward reflects the goal and provides positive feedback that directs the agent toward the desired outcomes.
The hybrid styles are semi-supervised, feature, and robot learning. The advanced styles are transfer, federated, self-taught, multi-view, online, co-learning, few-shot learning, and ensemble learning (14).
DL is based on the structure and function of the human brain. It builds algorithms into layers, composed of millions of computing neurons, to create an artificial neural network (ANN) (Figure 1). DL uses structured and unstructured data to train a model and performs pre-processing, feature extraction, and recognition. It determines the accuracy of a prediction based on its own ANN (15). It also can exponentially scale up with the rising amount of data, being useful for solving complicated computational problems (15). Furthermore, DL is capable of learning and making intelligent decisions for itself, overtaking other ML methods.
There are different DL-based techniques, including multi-layer perceptrons, in which neurons are in sequential layers, leading to unidirectional movement of information; recurrent neural networks, which analyze an input sequence one item at a time while storing all previous components; and convolutional neural network (CNN), which can learn invariant features to represent spatial correlations from image date (3).
Some of the DL models are CNN, Visual Geometry Group Network (VGGNet), Residual Network (ResNet), Fully Convolutional Networks (FCNs), U-Net, Deep Feed-Forward Networks, Siamese Neural Networks, and Graph Neural Networks (13).
The major clinical manifestations of MM at diagnosis include lytic bone disease, presenting with pain and fractures, anemia, hypercalcemia, renal failure, and an increased risk of infections (14). MM is often confused with other diseases, delaying its diagnosis. It has been reported that 49% of MM patients are diagnosed in emergency services or other secondary care specialties different from hematology, often leading to diagnostic and therapeutic delays (15). The timely diagnosis of MM is crucial for achieving the best patient outcomes and a better quality of life. It is based on the demonstration of ≥10% clonal bone marrow (BM) plasma cells or a biopsy-proven plasmacytoma plus evidence of one or more MM-defining events attributable to the plasma cell disorder or the presence of biomarkers of malignancy (16).
However, in routine clinical practice of physicians different from hematologists, there is no immediate access to BM biopsy, relying mostly on laboratory and imaging data. There is a large amount of clinical and readily accessible laboratory data available that can be processed by AI algorithms to achieve faster and more accurate diagnosis of MM. Although screening tests have been reserved for widely prevalent diseases such as colon and breast cancers, the possibility of developing highly specific models for early detection and intervention of MM patients would be useful for early diagnosis of MM (6).
Different laboratory tests are now collected routinely during clinical practice in most of the patients who present with or without concern for MM. Therefore, analysis of these readily accessible data represents an opportunity for early identification of MM. A retrospective study including a total of 4,187 routine blood and biochemical tests obtained over 10 years from 1,741 MM and 2,446 non-MM patients (infectious, hepatic, renal, and rheumatic immune system diseases) examined the use of routine tests in the diagnosis of MM. Laboratory data included hemoglobin, serum creatinine, serum calcium, quantitative immunoglobulins (IgA, IgG, and IgM), serum albumin, total proteins, and albumin/globulin ratio. The data were analyzed using a Gradient Boosting Decision Tree (GBDT), Support Vector Machine (SVM), Deep Neural Network (DNN), and Random Forest (RF), and an early assistant diagnostic model of MM was created. Among the four ML algorithms, GBDT yielded the highest precision with AUC of 0.929 and 0.899 for MM and non-MM patients, respectively (17).
As immunoglobulin assays are not part of the routine laboratory tests, six variables (hemoglobin, serum creatinine, serum calcium, albumin, total protein, and albumin/globulin ratio) were used to train the model. If the immunoglobulins were considered unavailable, the model would have a 0.79 precision, 0.726 recall, and 0.760 F1 score, which are lower than those of the nine-variable model fit with immunoglobulin based on the GBDT model, with the potential of being more extensively applicable.
It is important to note that although these applications rely on laboratory tests that are routinely part of myeloma assessment, the analysis of these data is performed in an unbiased approach (independent of clinical scenario or indication) and does not rely on dichotomization or pattern approach that may be used by human interpreters. Furthermore, as these models rely solely on results, they can analyze large amounts of data without initial human supervision.
Based on these results, a workflow could be incorporated depending on the available clinical information. For the patients where quantitative immunoglobulins are available, the nine-variable model should be used to achieve higher accuracy. For health centers where immunoglobulin examination is not commonly ordered, the six-variable model should be used as a precautionary measure should indications of further immunoglobulin investigations appear.
Serum protein and serum immunofixation are commonly used for diagnosis and evaluation of response in MM patients. In some laboratories, interpretation is performed manually, which is a time-consuming process that may take up to 3–5 days, delaying decision-making. These processes would benefit from automatization, and ML approaches are useful for this analysis. Evaluation of the presence of M protein and the concentration of albumin, alpha1, alpha2, beta1, beta2, and gamma regions from the gel strips and densitometer graphs has been performed using artificial neural networks, VGG-16, RF, and SVM to automate this process (18). CNN, specifically VGG-16, exhibited the best performance, with reasonable processing times, followed by artificial neural networks and RF, and SVM exhibited the worst performance (18).
Cardiac amyloidosis (CA) arises from the deposition of misfolded proteins in the cardiac muscle. The major types are light chain amyloidosis, caused by the accumulation of immunoglobulin light chains, and transthyretin amyloidosis among the most common etiologies. CA is largely underdiagnosed, as its clinical manifestations can be subtle and often difficult to differentiate from changes observed in hypertension or other cardiovascular diseases, making diagnosis challenging and often delaying the treatment. One approach to overcome this difficulty is the use of automated diagnostic algorithms, which enable the extraction of salient information from “raw data” without requiring pre-processing based on the a priori knowledge of the human operator (15).
Different AI models have been shown to facilitate the detection of rare diseases such as CA (15). Considering that CA patients are likely to present to non-experts with their initial symptoms, and the extensive availability of electrocardiograms in general medicine settings, an AI model was constructed to facilitate the detection of CA, which was evaluated in different medical centers (18). The approach exhibited good predictive accuracy as measured by C-statistics of 0.85–0.91 (95% CI 0.90–0.93), followed by analyses of echocardiograms (ECGs), which exhibited C-statistics of 0.89–1.00, highlighting the necessity of a second set of confirmatory diagnostic testing such as free light chain test, scintigraphy, and possibly tissue biopsy. Other AI algorithms for evaluating magnetic resonance, echocardiography, and mass spectrometry have also been used for the diagnosis of CA (15).
BM aspirate differential cell counts are critical for diagnosis and evaluation of complete response after treatment. Manual counting is the gold standard but has significant limitations, including using a small number of counted cells and being labor-intensive and time-consuming, with high inter- and intra-observer variability (19). In peripheral blood (PB), automated cell analyses are well-established and extensively used. However, few reliable automated counters of BM have been developed because of the complexity of these samples. Diversity in cell morphologies and the high density of touching cells in BM smears hinder the segmentation of single cells, which is required for image processing.
ML approaches have emerged as the principal model for analyzing histology images. Digital pathology imaging coupled with ML had been used to detect and classify BM aspirate cells. ML algorithms that use neural networks are adaptive and can learn from data in an unbiased manner. They exhibit superior performance in detection and classification, and they can require thousands of labeled examples for training algorithms to recognize variations in staining and morphology and to reach diagnostically meaningful accuracy.
ML digital pathology systems that allow differential cell counts of normal BM by analyzing the whole slide have been described (19–22), and one of them included a small set of MM (19). This software achieved a high degree of accuracy in cell detection (0.98 precision-recall AUC) and classification (0.97 ROC AUC) for MM using a two-stage system based on CNN. These algorithms represent an important initial step for MM diagnosis and require validation in larger clinical cohorts.
FC is used in clinical practice to quickly confirm an accurate diagnosis of MM, characterizing, quantifying, and demonstrating the presence of clonal plasma cells in BM or any tissue. Next-generation FC (23) paired with AI algorithms and clinical databases automatically identifies cell populations and significantly reduces the variability of analysis compared with manual approaches, as demonstrated by the FlowCAP (Flow Cytometry: Critical Assessment of Population Identification Methods) competition. Next-generation FC evaluates, with high sensitivity and specificity, the response of treatment and detects measurable residual disease (MRD), becoming the most important prognostic factor of MM patients (24) independent of cytogenetic risk in recent years and is currently being explored as a potential guide to indicate intensification or de-escalation of therapy (25, 26).
Currently, newer instruments can measure 40 or more parameters, enhancing the ability of FC to profile patients with cancer, making FC one of the most important high-throughput tools for single-cell analysis. High-dimensional data visualization requires multiple biaxial plots, which increase quadratically with the number of measured parameters (phenomena described as dimensionality explosion). Therefore, the interpretation of this large amount of data is challenging. It is not easy to identify reproducibly relevant subpopulations or perform large-scale immune monitoring that could be a useful marker of treatment response, particularly to immunotherapies. Furthermore, it depends highly on the operator’s knowledge, adding potential bias and variability of results (27).
AI is an attractive approach to solving these problems. Different types of AI algorithms are being applied to clinical FC data. Supervised and unsupervised clustering algorithms for cell population identification, various dimensionality reduction and ML pipelines, and SL approaches for classifying entire cytometry samples improve diagnostic sensitivity and accuracy as well as prognostic scores (28, 29).
Many programs have been used to accomplish this goal. These are relatively simple workspaces to process, analyze, and visualize large datasets. It includes pre-processing, normalization, multiple dimensionality reduction techniques, automated clustering, and predictive modeling tools, using several ML algorithms to build predictive models of survival or to learn classification rules. Using FlowCT (30), the T-cell compartment has been compared in BM with PB from smoldering MM patients identifying immune biomarkers of progression from smoldering to active MM in a minimally invasive approach, as well as prognostic T-cell subsets in BM of active MM after treatment intensification.
Biomarkers to personalize treatment are continually searched to improve MM outcomes. Currently, undetectable MRD is considered a new endpoint of MM therapy, an intermediate surrogate of prolonged survival (31). Treatment individualization based on the probability of an individual patient attaining undetectable MRD with a singular regimen and confirmation of predicted MRD outcomes represent a new concept of personalized treatment. The classical prognostic factors have a limited ability to predict MRD outcomes when used individually. By contrast, an ML model (32) including tumor burden, cytogenetic (del(17p13) and/or t(4;14)), and immune-related biomarkers predict MRD outcomes in up to 72% of newly diagnosed MM (NDMM) patients treated with different regimens and who have different survival rates, with significant accuracy. This model is available online (www.MRDpredictor.com) (32).
BM biopsies have a single-site bias. Thus, new diagnostic tests and early detection strategies are required in MM. Matrix-assisted laser desorption/ionization time-of-flight mass spectrometry (MALDI-TOF MS) coupled to artificial neural networks can analyze non-linear data and predict and classify variables in multidimensional datasets. PB plasma evaluated using MALDI-TOF MS from MM patients and healthy donors had generated patterns in mass spectra that had served as inputs for artificial neural networks that specifically predicted MM samples with high sensitivity (100%), specificity (95%), and accuracy (98%) (33).
Because of the limitations of conventional diagnostic approaches, such as BM biopsy with inadequate representation of tumor due to the patchy BM or extramedullary infiltration of MM (34) and being an invasive procedure, other methods of diagnosis and prognosis are of significant interest. In this setting, 18F-fluorodeoxyglucose (FDG) positron emission tomography (PET)/computed tomography (CT) plays a crucial role. This diagnostic imaging method has reached a significant level of evidence for clinical decision-making and for establishing prognosis and treatment response. Currently, there is an increased interest in using multimodal applications of ML coupled to PET/CT, as this method facilitates examination of the entire intra-patient heterogeneity of tumor involvement, rather than using limited information obtained from unimodal images (35). In addition, its capacity to predict patient prognosis based on radiomics signatures (36) could have great potential in stratifying MM patients as either low- or high-risk, which is a promising application of AI in this scenario.
Image data of PET, CT, and clinical parameters were analyzed using machine learning algorithms [linear gradient boosting models based on Cox’s partial likelihood (GB-Cox), Cox model by likelihood-based boosting (CoxBoost), generalized boosted regression modeling (GBM), RF for survival model (RSF), and support vector regression for censored data model (SVCR)]. Then, a prognostic model was constructed, which included five PET-based features, four CT-based features, and six clinical data that were significantly related to progression-free survival. Among the algorithms, RSF and GBM had the greatest prognostic accuracy (36).
During the last few years, different prognostic models have been used to stratify NDMM patients, among which the International Staging System (ISS) (37) and Revised-ISS (R-ISS) (38) are the most common. Both prognostic indexes were derived from clinical trials, and these have been validated in real-world MM cohorts treated with different drug combinations. However, there are knowledge gaps, as most patients are categorized into low or intermediate groups. Furthermore, some patients who are classified as low risk have a short survival, and the majority of patients stratified by R2-ISS have very heterogeneous outcomes. Recently, the R2-ISS (39) staging system has been recommended, which added the presence of 1q21 gain/amplification (1q21) to the R-ISS and allowed a better stratification within the intermediate-risk NDMM. New prognostic models based on genetic factors using high-throughput genomic analysis could be very useful, but these are not easily applicable in real life because of cost and technological complexity.
Several studies have shown how AI can significantly improve risk stratification. Using clinical, biochemical, and cytogenetic variables from three different trials of NDMM patients aged 65 years and older and UL clusterization (40), two novel subgroups of patients with significantly different survival were identified. The prognostic precision of this clusterization was superior to that of ISS and R-ISS scores and appeared to be particularly useful in improving risk stratification among R2-ISS patients. The model re-classified the R2-ISS score into clusters with significantly divergent overall survival (OS). Of note, all patients with high lactate dehydrogenase (LDH) or high-risk cytogenetics were assigned to the high-risk cluster. The model retained its predictive power independent of induction type, transplant, conditioning, and the different maintenance schemes. Despite that the model shares some variables with ISS or R-ISS, it provides additional discriminative value to the former two.
Another model used ML, creating a 50-variable RF (IAC-50) that included clinical (patient age and ISS stage), biochemical (serum beta-2 microglobulin), and RNA-seq (expression of 46 genes) data collected by the CoMMpass consortium. The model predicted OS with high concordance between both training and validation sets (C-indexes, 0.818 and 0.780, respectively) (41). Survival predictions for each patient considering the first line of treatment verified that those individuals treated with the best-predicted drug combination had significantly lower mortality rates than patients treated with other schemes. This was particularly important in patients treated with a triple combination therapy including bortezomib, an immunomodulator, and dexamethasone. Notably, the model exhibited a trend of retaining its predictive value in patients with high-risk cytogenetics.
Another model has been developed for OS prediction. Four algorithms were used (Cox proportional hazards, DeepSurv, DeepHit, and RSF), and 30 parameters were incorporated (baseline data, genetic abnormalities, and treatment options) of patients older than 65 years. The RSF model yielded the best score, and OS predictions were largely affected by the maintenance schema variable with the immunomodulator group having the best survival rate (42).
Over the last 15 years, there have been a considerable number of new medications approved for treating MM, with more than 15 new Food and Drug Administration (FDA)-approved medications over the last 10 years. Furthermore, these therapies are often combined with preexisting medications, with the current standard of care including four- or three-drug combinations. Although approval of medications is largely driven by randomized clinical trials (RCTs), where a new medication or combination is compared with a standard of care, the large number of newly approved treatments leads to a lack of head-to-head comparison of interventions. Moreover, although RCTs are fundamental for the assessment of the efficacy of new interventions, clinical trials may not be representative of a specific patient characteristic. In addition, RCTs are often under-represented in patients with high-risk MM (defined by staging classification, clinical features such as extramedullary disease, or genomic/cytogenetic characteristics).
AI represents an attractive approach to leverage the large volume of clinical data that exist in MM, for both patients included in clinical trials and those included in registries or other real-world cohorts. Baseline characteristics including clinical presentation, genomic characteristics, and other variables could be incorporated to either stratify the patients’ prognosis as indicated previously or suggest optimal therapy or combination therapies.
Early examples of this include the model IAC-50, which was created and validated based on the aforementioned CoMMpass registry. This model evaluated the combination and number of agents used in the first line and identified that only 41% of cases received the optimal first-line regimen. Although many of the suggestions would be consistent with our current understanding of MM treatment, such as higher activity, progression-free survival, and OS, with three- vs. two-agent combinations, the IAC-50 model may inform of optimal agents within a therapeutic class, which can identify patients who may benefit from using bortezomib instead of carfilzomib and vice versa. Furthermore, the IAC-50 also informs on the specific triple combinations of different classes of therapy, with patients receiving “optimal” therapy according to the IAC-50 and retaining the predictive value regardless of age (>65 years older at diagnosis), autologous stem cell transplant consolidation, or maintenance use (41).
Similarly, data from several large clinical registries and prospective trials with available whole genome sequencing were pooled. A neural Cox with non-proportional hazards (NCNPH)-based model was created and trained in a discovery cohort of 1,933 patients followed by validation in a cohort of 256 patients originating from the same cohort. The model, named Individualized Risk Model Myeloma (IRMMa), was constructed following a multistate architecture that allowed controlling for time-dependent covariates such as autologous stem cell transplant (ASCT) and maintenance, with a total of two phases: phase 1, induction; and phase 2, post-induction (43).
IRMMa incorporated demographic, clinical, therapeutic, and genomic characteristics to evaluate the prognosis of each patient, with an overall higher C-index for event-free survival (0.69) and OS (0.73) compared with clinical staging systems such as ISS, R-ISS, and R2-ISS. Furthermore, genomic data improved the performance of the model with well-described high-risk alterations such as t (4;14) and deletion 17p; other alterations such as elevated APOBEC mutational signatures were also identified as prognostic.
Lack of access to genomic information is typical during routine clinical practice, which limits the applicability of models that incorporate these types of data. Approaches to inhibit this include the creation of flexible models that can accommodate missing information. IRMMa allows for missing data, and even in the absence of genomic data, it still outperforms ISS, R-ISS, and R2-ISS. Of note, the model also allowed the identification of patients who may benefit from specific interventions, such as ASCT (43). Park et al. developed a prognostic ML model based on data available as part of the current standard of care, using baseline demographic, laboratory, and cytogenetic information for patients treated with lenalidomide and dexamethasone (Rd) or bortezomib, melphalan, and prednisone (VMP), and based on the individual expected OS, a decision on the most appropriate regimen could be informed (44). Importantly, different variables were predictive for outcomes of VMP or Rd treatment, and data on early response were incorporated to further guide potential optimal therapy.
Both IAC-50 and IRMMa are examples of the use of artificial intelligence to leverage existing clinical data, both originating from clinical trials and registries, and they highlight the utility of these models to incorporate many variables to identify potential candidates for a specific intervention. However, with a large number of new therapy options and combinations, longitudinal integration of data will be paramount, as the models would only be able to suggest combinations for interventions included in their training cohorts.
Although some AI applications that once seemed like science fiction are now used in routine clinical medicine, such as interpreting ECG and identifying white blood cell subsets, AI has inherent limitations that need to be acknowledged.
First, as mentioned previously, AI models rely on preexisting data, which leads to an inherent bias for recent/novel interventions, such as a new medication or new diagnostic procedure. It is not likely, therefore, that preexisting AI models could predict the outcomes or response to a novel therapy, especially if they rely on an alternative mechanism of action. For example, in the recently introduced bispecific T-cell engagers in MM, AI will not replace prospective clinical trials and first-in-human drug development. However, AI can enhance drug development through the identification of complex protein–protein interactions or targets, which are applications already being developed. Furthermore, AI could enhance the creation of synthetic controls for RCTs of uncommon disease subgroups, an approach that was already incorporated by the OPTIMUMM trial (reference) using previous trials. However, the impact of this approach would need to be validated, as it may introduce inherent bias.
Second, although not specific to MM, the development of generative AI platforms that can output text, summaries, or other information sets based on unstructured data (such as reports, imaging data, or encounters with patients) may ultimately enhance patient care, limiting the toll on clinicians, reducing hospital costs, and contributing to accurate representation in the electronic medical record and/or trial data generation.
Although there are several limitations mentioned previously, the capability of AI to integrate a large number of variables surpasses the capacity of any human, representing a new approach that otherwise would not be accessible without this technology. With the large amount of data generated in routine clinical practice, exploiting the use of these results, even before considering newer and more expensive techniques (e.g., generalized whole genome/exome sequencing), is a logical next step.
Consequently, although there are innumerable applications for AI, prospective validation of the efficacy of these interventions is paramount and should be treated in the same pragmatic approach as any new intervention in the field, ensuring there is a new benefit for patients or the system.
Lastly, the question of whether AI will replace physicians naturally arises. However, although the capability of AI to support clinicians in decision-making is promising, AI will likely represent a tool to facilitate and enhance the role of physicians in patient care, with longitudinal interactions and patient–physician interactions, and the resulting relationships are invaluable for patients. As both AI and humans aim for the same endpoint, which is to provide the best care and outcomes for multiple myeloma patients, AI represents another improvement to our toolbox, such as the use of fluorescence in situ hybridization (FISH) cytogenetics, therapeutic developments, and advances in imaging. Finally, it is important to note that all the applications of AI described are likely to be beneficial in parallel and not a substitute for human input.
The prognosis of MM patients has changed radically over the last 20 years owing to therapeutic developments, which have enhanced our understanding of plasma cell biology and have resulted in the incorporation of cytogenetic data in clinical and laboratory data with refinements in prognostic models used in clinical practice. Despite this, MM is still a heterogeneous disease, with the current clinical staging system classifying approximately one-third of patients in the intermediate-risk stage, often with heterogeneous clinical outcomes within the same risk stratification.
AI has the potential to revolutionize MM care at all stages, from screening large populations with widely available clinical laboratories (such as hemoglobin, renal function, and albumin) to expediting diagnosis, potentially preventing MM-related complications caused by therapeutic delays. Furthermore, automatization in the processing of different diagnostic methodologies, such as the analysis of serum protein electrophoresis and FC, still relies heavily on human input and represents an area where AI applications could allow automatization, resulting in lower variability and time to result. In addition, with the large volume of data produced by multiplex methods, such as next-generation flow (NGF) and next-generation sequencing, AI applications represent the most attractive approach to facilitate the application of these results to clinical practice, as human analysis is often limited and results in high interpersonal variability.
Similarly, the use of AI in facilitating the diagnosis of amyloidosis represents an attractive approach, as pre-defined clinical criteria based on imaging or ECG are not sensitive enough to identify these patients. However, given the extensive access to ECG and other imaging modalities such as echocardiogram, an AI application that takes the raw data from these modalities could alert non-hematologist healthcare providers regarding the potential diagnosis of amyloidosis, potentially facilitating timely referral and diagnosis.
Although the management of MM has relied heavily on prospective data from clinical trials, the large number of therapeutic options, combined with the paradigm of combination therapy, results in many possible multi-agent regimens. As the direct comparison of these regimens is often not practical and would require many patient follow-ups, leveraging existing clinical and genomic data from randomized trials, registries, and real-world cohorts represented with AI models represents an attractive option that could inform on the optimal therapeutic approach. As mentioned previously, the success of this application relies on the integration of AI models with clinical practice, as predictive models will be required to be relevant and accessible. Longitudinal updates of these models are imperative given the rapid emergence of new therapeutic options, in addition to being flexible enough to work with only clinically available data and also potentially use genomic data that may be available in some scenarios.
To conclude, AI has many applications in the MM space amenable to scalable and systematic implementation in clinical practice. However, although it represents a promising field, validation of the effect of these applications on the intended outcome (time to diagnosis, efficacy, accuracy, prognosis, or therapeutic effect) is required to ensure that AI provides significant improvements to patient care or the system. Furthermore, given the importance of the integration of newly available data in AI models, new challenges arise, such as the longitudinal integration of data, which need to be considered to ensure that AI models are representative, updated, and clinically relevant for daily patient care. In addition, the use of AI as a tool to enhance both clinician and patient experience will likely be part of MM care and medicine in general, and critical use of these emerging tools is required to ensure a meaningful application.
MR: Writing – original draft, Writing – review & editing. AM: Writing – review & editing. MM: Writing – original draft, Writing – review & editing.
The author(s) declare that no financial support was received for the research, authorship, and/or publication of this article.
The authors declare that the research was conducted in the absence of any commercial or financial relationships that could be construed as a potential conflict of interest.
All claims expressed in this article are solely those of the authors and do not necessarily represent those of their affiliated organizations, or those of the publisher, the editors and the reviewers. Any product that may be evaluated in this article, or claim that may be made by its manufacturer, is not guaranteed or endorsed by the publisher.
1. Siegel RL, Miller KD, Wagle NS, Jemal A. Cancer statistics, 2023. CA: A Cancer J Clin (2023) 73(1):17–48. doi: 10.3322/caac.21763
2. Mejia Saldarriaga M, Darwiche W, Jayabalan D, Monge J, Rosenbaum C, Pearse RN, et al. Advances in the molecular characterization of multiple myeloma and mechanism of therapeutic resistance. Front Oncol (2022) 12:1020011. doi: 10.3389/fonc.2022.1020011
3. Allegra A, Tonacci A, Sciaccotta R, Genovese S, Musolino C, Pioggia G, et al. Machine learning and deep learning applications in multiple myeloma diagnosis, prognosis, and treatment selection. Cancers (2022) 14(3):606. doi: 10.3390/cancers14030606
4. Cappell KM, Kochenderfer JN. Long-term outcomes following car T cell therapy: What we know so far. Nat Rev Clin Oncol (2023) 20(6):359–71. doi: 10.1038/s41571-023-00754-1
5. Moreau P, Garfall AL, van de Donk NWCJ, Nahi H, San-Miguel JF, Oriol A, et al. Teclistamab in relapsed or refractory multiple myeloma. N Engl J Med (2022) 387(6):495–505. doi: 10.1056/nejmoa2203478
6. Rodriguez-Otero P, Paiva B, San-Miguel JF. Roadmap to cure multiple myeloma. Cancer Treat Rev (2021) 100:102284. doi: 10.1016/j.ctrv.2021.102284
7. Duminuco A, Mosquera-Orgueira A, Nardo A, Di Raimondo F, Palumbo GA. AIPSS-MF machine learning prognostic score validation in a cohort of myelofibrosis patients treated with ruxolitinib. Cancer Rep (2023) 6(10). doi: 10.1002/cnr2.1881
8. Mosquera-Orgueira A, Pérez-Encinas M, Hernández-Sánchez A, González-Martínez T, Arellano-Rodrigo E, Martínez-Elicegui J, et al. Machine learning improves risk stratification in myelofibrosis: An analysis of the spanish registry of myelofibrosis. Hemasphere (2022) 7(1). doi: 10.1097/hs9.0000000000000818
9. Mosquera Orgueira A, Cid López M, Peleteiro Raíndo A, Abuín Blanco A, Díaz Arias JÁ, González Pérez MS, et al. Personally tailored survival prediction of patients with follicular lymphoma using machine learning transcriptome-based models. Front Oncol (2022) 11:705010. doi: 10.3389/fonc.2021.705010
10. Dinh A, Miertschin S, Young A, Mohanty SD. A data-driven approach to predicting diabetes and cardiovascular disease with machine learning. BMC Med Inf Decision Making (2019) 19(1). doi: 10.1186/s12911-019-0918-5
11. Fleuren LM, Klausch TL, Zwager CL, Schoonmade LJ, Guo T, Roggeveen LF, et al. Machine learning for the prediction of sepsis: A systematic review and meta-analysis of diagnostic test accuracy. Intensive Care Med (2020) 46(3):383–400. doi: 10.1007/s00134-019-05872-y
12. Yin J, Ngiam KY, Teo HH. Role of artificial intelligence applications in real-life clinical practice: Systematic review. J Med Internet Res (2021) 23(4). doi: 10.2196/25759
13. Saranya A, Subhashini R. A systematic review of explainable artificial intelligence models and applications: Recent developments and future trends. Decis Anal J (2023) 7:100230. doi: 10.1016/j.dajour.2023.100230
14. Mahadevkar SV, Khemani B, Patil S, Kotecha K, Vora DR, Abraham A, et al. A review on machine learning styles in Computer Vision—techniques and future directions. IEEE Access (2022) 10:107293–329. doi: 10.1109/access.2022.3209825
15. Allegra A, Mirabile G, Tonacci A, Genovese S, Pioggia G, Gangemi S. Machine learning approaches in diagnosis, prognosis and treatment selection of cardiac amyloidosis. Int J Mol Sci (2023) 24(6):5680. doi: 10.3390/ijms24065680
16. Rajkumar SV, Dimopoulos MA, Palumbo A, Blade J, Merlini G, Mateos M-V, et al. International Myeloma Working Group updated criteria for the diagnosis of multiple myeloma. Lancet Oncol (2014) 15(12). doi: 10.1016/s1470-2045(14)70442-5
17. Yan W, Shi H, He T, Chen J, Wang C, Liao A, et al. Employment of artificial intelligence based on routine laboratory results for the early diagnosis of multiple myeloma. Front Oncol (2021) 11:608191. doi: 10.3389/fonc.2021.608191
18. Rutkowski L. Artificial Intelligence and soft computing: 22nd International Conference, ICAISC 2023, Zakopane, Poland, June 18-22, 2023, Proceedings. Cham: Springer (2023).
19. Chandradevan R, Aljudi AA, Drumheller BR, Kunananthaseelan N, Amgad M, Gutman DA, et al. Machine-based detection and classification for bone marrow aspirate differential counts: Initial development focusing on nonneoplastic cells. Lab Invest (2020) 100(1):98–109. doi: 10.1038/s41374-019-0325-7
20. Lewis JE, Shebelut CW, Drumheller BR, Zhang X, Shanmugam N, Attieh M, et al. An automated pipeline for differential cell counts on whole-slide bone marrow aspirate smears. Mod Pathol (2023) 36(2):100003. doi: 10.1016/j.modpat.2022.100003
21. Choi JW, Ku Y, Yoo BW, Kim J-A, Lee DS, Chai YJ, et al. White blood cell differential count of maturation stages in bone marrow smear using dual-stage convolutional Neural Networks. PloS One (2017) 12(12). doi: 10.1371/journal.pone.0189259
22. Fu X, Fu M, Li Q, Peng X, Lu J, Fang F, et al. Morphogo: An automatic bone marrow cell classification system on digital images analyzed by Artificial Intelligence. Acta Cytologica (2020) 64(6):588–96. doi: 10.1159/000509524
23. Flores-Montero J, Sanoja-Flores L, Paiva B, Puig N, García-Sánchez O, Böttcher S, et al. Next generation flow for highly sensitive and standardized detection of minimal residual disease in multiple myeloma. Leukemia (2017) 31(10):2094–103. doi: 10.1038/leu.2017.29
24. Paiva B, Puig N, Cedena M-T, Rosiñol L, Cordón L, Vidriales M-B, et al. Measurable residual disease by next-generation flow cytometry in multiple myeloma. J Clin Oncol (2020) 38(8):784–92. doi: 10.1200/jco.19.01231
25. Rosiñol L, Oriol A, Ríos-Tamayo R, Blanchard M-J, Jarque I, Bargay J, et al. Lenalidomide and dexamethasone with or without IXAZOMIB maintenance tailored by residual disease status in myeloma. Blood (2023) 142(18):1518–28. doi: 10.1182/blood.2022019531
26. Costa LJ, Chhabra S, Medvedova E, Dholaria BR, Schmidt TM, Godby KN, et al. Minimal residual disease response-adapted therapy in newly diagnosed multiple myeloma (master): Final report of the multicentre, single-arm, phase 2 trial. Lancet Haematol (2023) 10(11):e890–901. doi: 10.1016/s2352-3026(23)00236-3
27. Palit S, Heuser C, de Almeida GP, Theis FJ, Zielinski CE. Meeting the challenges of high-dimensional single-cell data analysis in Immunology. Front Immunol (2019) 10:1515. doi: 10.3389/fimmu.2019.01515
28. Seifert RP, Gorlin DA, Borkowski AA. Artificial Intelligence for clinical flow cytometry. Clinics Lab Med (2023) 43(3):485–505. doi: 10.1016/j.cll.2023.04.009
29. Fuda F, Chen M, Chen W, Cox A. Artificial Intelligence in clinical multiparameter flow cytometry and mass cytometry–key tools and progress. Semin Diagn Pathol (2023) 40(2):120–8. doi: 10.1053/j.semdp.2023.02.004
30. Botta C, Maia C, Garcés J-J, Termini R, Perez C, Manrique I, et al. FLOWCT for the analysis of large immunophenotypic data sets and biomarker discovery in cancer immunology. Blood Adv (2022) 6(2):690–703. doi: 10.1182/bloodadvances.2021005198
31. Harousseau J-L, Avet-Loiseau H. Minimal residual disease negativity is a new end point of myeloma therapy. J Clin Oncol (2017) 35(25):2863–5. doi: 10.1200/jco.2017.73.1331
32. Guerrero C, Puig N, Cedena M-T, Goicoechea I, Perez C, Garcés J-J, et al. A machine learning model based on tumor and immune biomarkers to predict undetectable MRD and survival outcomes in multiple myeloma. Clin Cancer Res (2022) 28(12):2598–609. doi: 10.1158/1078-0432.ccr-21-3430
33. Deulofeu M, Kolářová L, Salvadó V, María Peña-Méndez E, Almáši M, Štork M, et al. Rapid discrimination of multiple myeloma patients by artificial neural networks coupled with mass spectrometry of peripheral blood plasma. Sci Rep (2019) 9(1). doi: 10.1038/s41598-019-44215-1
34. Zamagni E, Nanni C, Dozza L, Carlier T, Bailly C, Tacchetti P, et al. Standardization of 18F-FDG–PET/CT according to Deauville criteria for metabolic complete response definition in newly diagnosed multiple myeloma. J Clin Oncol (2021) 39(2):116–25. doi: 10.1200/jco.20.00386
35. Zhang L, Wang Y, Peng Z, Weng Y, Fang Z, Xiao F, et al. The progress of multimodal imaging combination and subregion based radiomics research of cancers. Int J Biol Sci (2022) 18(8):3458–69. doi: 10.7150/ijbs.71046
36. Zhong H, Huang D, Wu J, Chen X, Chen Y, Huang C. 18F−FDG PET/CT based radiomics features improve prediction of prognosis: Multiple machine learning algorithms and multimodality applications for multiple myeloma. BMC Med Imaging (2023) 23(1). doi: 10.1186/s12880-023-01033-2
37. Greipp PR, Miguel JS, Durie BGM, Crowley JJ, Barlogie B, Bladé J, et al. International staging system for multiple myeloma. J Clin Oncol (2005) 23(15):3412–20. doi: 10.1200/jco.2005.04.242
38. Palumbo A, Avet-Loiseau H, Oliva S, Lokhorst HM, Goldschmidt H, Rosinol L, et al. Revised international staging system for multiple myeloma: A report from international myeloma working group. J Clin Oncol (2015) 33(26):2863–9. doi: 10.1200/jco.2015.61.2267
39. D’Agostino M, Cairns DA, Lahuerta JJ, Wester R, Bertsch U, Waage A, et al. Second revision of the international staging system (R2-ISS) for overall survival in multiple myeloma: A European myeloma network (EMN) report within the harmony project. J Clin Oncol (2022) 40(29):3406–18. doi: 10.1200/jco.21.02614
40. Mosquera Orgueira A, González Pérez MS, Diaz Arias J, Rosiñol L, Oriol A, Teruel AI, et al. Unsupervised machine learning improves risk stratification in newly diagnosed multiple myeloma: An analysis of the Spanish Myeloma Group. Blood Cancer J (2022) 12:76. doi: 10.1038/s41408-022-00647-z
41. Mosquera Orgueira A, González Pérez MS, Díaz Arias JÁ, Antelo Rodríguez B, Mateos M-V. Prognostic stratification of multiple myeloma using clinicogenomic models: Validation and performance analysis of the IAC-50 model. HemaSphere (2022) 6(8). doi: 10.1097/hs9.0000000000000760
42. Bao L, Wang Y, Zhuang J, Liu A, Dong Y, Chu B, et al. Machine learning–based overall survival prediction of elderly patients with multiple myeloma from multicentre real-life data. Front Oncol (2022) 12:922039. doi: 10.3389/fonc.2022.922039
43. Maura F, Rajanna A, Ziccheddu B, Derkach A, Poos A, Maclachlan K, et al. P-357 individualized risk in newly diagnosed multiple myeloma. Clin Lymphoma Myeloma Leukemia (2023) 23. doi: 10.1016/s2152-2650(23)01975-4
Keywords: multiple myeloma, machine learning, prognosis model, early diagnosis, target therapy
Citation: Romero M, Mosquera Orgueira A and Mejía Saldarriaga M (2024) How artificial intelligence revolutionizes the world of multiple myeloma. Front. Hematol. 3:1331109. doi: 10.3389/frhem.2024.1331109
Received: 31 October 2023; Accepted: 09 January 2024;
Published: 02 February 2024.
Edited by:
Jing Ma, University of Illinois Chicago, United StatesReviewed by:
Jakub Radocha, University Hospital Hradec Kralove, CzechiaCopyright © 2024 Romero, Mosquera Orgueira and Mejía Saldarriaga. This is an open-access article distributed under the terms of the Creative Commons Attribution License (CC BY). The use, distribution or reproduction in other forums is permitted, provided the original author(s) and the copyright owner(s) are credited and that the original publication in this journal is cited, in accordance with accepted academic practice. No use, distribution or reproduction is permitted which does not comply with these terms.
*Correspondence: Martha Romero, bWFydGhhLnJvbWVyb0Bmc2ZiLm9yZy5jbw==
†These authors have contributed equally to this work
Disclaimer: All claims expressed in this article are solely those of the authors and do not necessarily represent those of their affiliated organizations, or those of the publisher, the editors and the reviewers. Any product that may be evaluated in this article or claim that may be made by its manufacturer is not guaranteed or endorsed by the publisher.
Research integrity at Frontiers
Learn more about the work of our research integrity team to safeguard the quality of each article we publish.