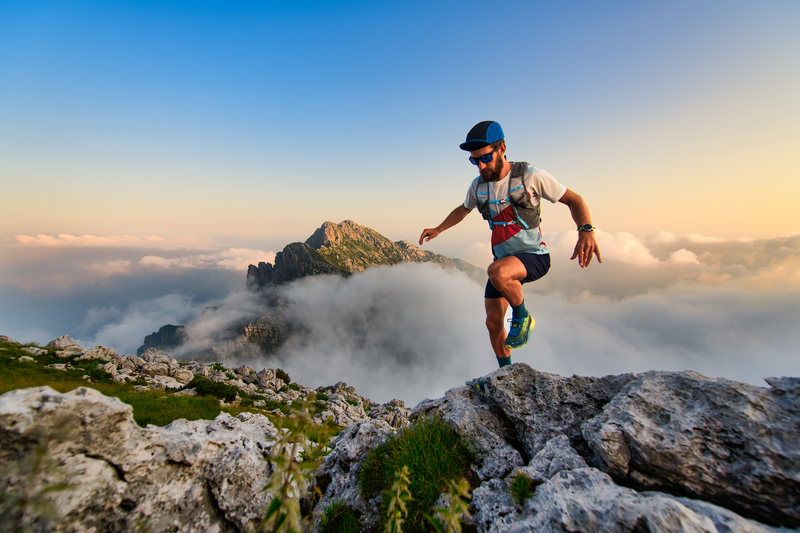
94% of researchers rate our articles as excellent or good
Learn more about the work of our research integrity team to safeguard the quality of each article we publish.
Find out more
ORIGINAL RESEARCH article
Front. Hematol. , 15 December 2022
Sec. Blood Cancer
Volume 1 - 2022 | https://doi.org/10.3389/frhem.2022.1060029
Purpose: Numerous factors may affect the survival outcomes of patients with acute myeloid leukemia (AML), mainly disease-related and treatment-related factors. The impact of other factors, such as the insurance status and the distance to healthcare facilities, are still unclear and may differ between different healthcare systems. We investigated the effects of insurance status and distance to the treatment center on the survival of AML patients.
Materials and methods: This is a single-center, observational, retrospective study of patients diagnosed with AML (2015–2020) and treated at the American University of Beirut Medical Center in Lebanon. Data regarding patient baseline characteristics, disease-related factors, insurance status, and area of residence were collected. Multivariate Cox regression analysis was used to identify main independent predictors of overall survival (OS).
Results: We identified 142 AML patients with a median age of 52 years (range 18–86). Of them, 91 (64%) were males, 77 (54%) had ELN intermediate risk, and 88 (62%) patients received intensive chemotherapy. After a median follow-up of 22.4 months, the median RFS and OS were 37.4 months and not reached, respectively. A Cox regression model for OS was done using the following variables: age, gender, body mass index, comorbidities, smoking status, insurance status, distance from the center, ELN classification, treatment used, and allotransplant. A higher risk of death was seen among the uninsured patients and those living beyond 40 km from the treatment center compared with fully insured patients and those living in proximity to the center (hazard ratio [HR]: 3.65; 95% CI [1.79, 7.45], p-value <0.0001; HR: 4.38; 95% CI [1.75, 10.95], p-value 0.002, respectively).
Conclusions: The outcome of patients with AML does not depend only on disease-related factors, as the insurance status and the distance from the area of residence to the treatment center were found to be independent predictors of survival in AML patients.
Acute myeloid leukemia (AML) is a group of hematologic neoplasms with malignant clonal expansion of myeloid stem cells accounting for around 35% of all leukemias (1, 2). Recent advancements in treatment options and the use of targeted therapies have improved the outcomes of patients diagnosed with AML. The 5-year relative survival in the US has improved from 6.4% in 1975 to 30.5% during the period of 2012 to 2018, as per SEER data analysis (2). In a single center study from Lebanon, until 2017, the 3-year overall survival (OS) was 53% and the 3-year leukemia-free survival (LFS) for patients achieving complete remission was 54% (3).
The OS of patients diagnosed with AML depends on many well-described risk factors; age, performance status, cytogenetics, molecular subtype, the type of treatment received, and socioeconomic factors are predictor factors of survival (4, 5). Access to treatment centers may be affected by insurance status and distance traveled, hence influencing the prognosis of patients diagnosed with AML. Although many studies showed a positive correlation between poor survival and lack of insurance and/or increased distance traveled (6, 7), others reported no correlation (6, 8–10).
In Lebanon, universal healthcare services are not available, leading to many inequities in access to healthcare, the ability to pay for it, and sometimes in health outcomes (11). Lebanon encompasses a small area of land of 10,452 square kilometers along the Mediterranean Sea with a population of 6.8 million and the largest per capita population of refugees in the world (12). Lebanon has both public and private healthcare insurances. More than half of the population is uninsured. The uninsured can benefit from medical care covered by the Ministry of Public Health (MoPH), but this would be in public hospitals or private ones with a predetermined payment ceiling.
In this paper, we used a database from the American University of Beirut Medical Center (AUBMC), a main non- profit private tertiary referral medical center for reviewing all cases treated for AML. Our center is located in Beirut, the capital of Lebanon. After controlling for potential confounding factors, we assessed the impact of healthcare insurance and distance traveled to the treatment center on the survival of patients newly diagnosed with AML. To the best of our knowledge, this paper is the first in Lebanon and the region to assess for potential disparities in the management of patients with AML.
All consecutive adult patients (≥18 years of age) newly diagnosed with AML who received treatment at the AUBMC between January 2015 and January 2020 were eligible for our study. We included cases of acute promyelocytic leukemia (APL). We excluded patients with an AML diagnosis on their charts with no further information about their disease and those who visited for a second opinion and then were lost to follow-up. The study was approved by the American University of Beirut (AUB) institutional review board, and in accordance with the declaration of Helsinki. A waiver of informed consent was granted for this chart review study.
Patients who were candidates for intensive chemotherapy received anthracyclines-based therapy for induction followed by intermediate dose of continuous cytarabine for consolidation and/or allogeneic hematopoietic cell transplant (allo-HCT). Others received hypomethylating agents, low-dose chemotherapy, or supportive care. Patients with APL received retinoic acid and arsenic trioxide. Allo-HCT was considered for patients with adverse-risk disease, selected patients with intermediate-risk disease based on the 2017 European Leukemia Net (ELN) classification (13), and relapsed/refractory AML.
This study used a database from the electronic medical record system (EMR). Our EMR contains baseline demographics, pathological and clinical data on patients diagnosed with AML. An extensive retrospective chart review was performed to collect information on age at diagnosis, gender, comorbidities at presentation, smoking status, body mass index (BMI), cytogenetic/molecular abnormalities, insurance source at diagnosis, distance to the treatment center, information on treatment administration, and allo-HCT. Hospitalizations, clinic follow-ups, and disease evaluations were also gathered from the charts of our patients. Patients were classified into three insurance groups: fully insured, publicly insured and uninsured, and two distance groups from our hospital: <40 kilometers (km) or ≥ 40 km. Distance was estimated based on the straight- line driving distance between patients’ most recent residence and our tertiary center. We used the last address on patients’ charts, which corresponds to patients’ address at last encounter. Forty kilometers was chosen being close to the mean distance traveled by all patients. Comorbidities were defined by the presence at diagnosis of one or more chronic health conditions different by etiology from the primary disease (14).
The primary endpoints of this study included 2-year OS and 2–year relapse-free survival (RFS). We calculated response outcomes as per the definition of the International medical group (15). OS was defined as the time that begins at diagnosis and up to the time of the last follow-up or death. RFS was calculated from the date of attaining the leukemia-free state to the date of relapse or death, whichever occurs first.
Percentages and frequencies were calculated for all the variables. Kaplan-Meier curves were used to estimate OS and RFS, the log-rank was used to check for significant differences between the studied groups. We used the Cox-regression analysis for survival estimates after adjusting for possible confounding factors, using the following: age, gender, body mass index, presence of comorbidities, smoking status, insurance status, distance from the center, ELN classification, and treatment used. We reported odds ratio (OR) and hazard ratio (HR) with 95% confidence intervals (CI). A p-value <0.05 was used to indicate statistical significance. All statistical analysis was performed using the SPSS v.25.0 statistical packages.
Two hundred eighteen patient charts were screened. A total of 142 patients (64% males) were included in our study based on the eligibility criteria. Their baseline characteristics are summarized in Table 1. The median age at diagnosis was 52 years (range, 18-86 years). The majority of patients are non-smokers (n=93, 65%) with no previous comorbidities (n=104, 73%). Most of patients were fully insured (n=65, 46%), a quarter were publicly insured (n=36, 25%) and almost a quarter were uninsured (n=41, 29%). The median BMI range was 26.5 Kg/m2 (16-42).
Forty percent (n=57) of our population had a distance to treatment center less than 40 Km. Thirty-eight (27%) patients had a favorable risk as per ELN 2017 classification, 77 (54%) had an intermediate-risk, and 23 (16%) patients had an adverse-risk. Seven patients had core binding factor (CBF) molecular aberrations on molecular studies. Fifty-three subjects had normal cytogenetic and molecular data, 12 subjects had NPM1 mutations, 10 subjects had FLT3 mutation and 14 had concomitant FLT3 and NPM1 mutations. Only 1 patient had CEBPA biallelic mutation. Among all patients, 21 had translocation between chromosome 15 and 17 [t (15, 16)], 10 had complex karyotype and 7 had inversion of chromosome 16 [inv (17)]. One subject had NPM1 mutation with complex karyotype, one had FLT3 mutation with complex karyotype and one had both CEBPA with cKit mutation. Eight had other derangements and 3 had incomplete cytogenetic and molecular data.
Eighty-eight (62%) patients received intensive chemotherapy, 25 (18%) received hypomethylating agents, 21 (15%) received retinoic acid and arsenic trioxide, with only two patients (1%) receiving low dose chemotherapy and one patient (1%) was managed with supportive care. Five (3%) patients were lost to follow-up and did not receive treatment at our center, thus not included in the final analysis. Sixty-six (46%) patients received an allo-HCT in first remission.
With a median follow-up of 22.4 months (range; 0-76 months), survival outcomes were estimated using the Kaplan- Meier curves. The mean OS was 52 months [46.1- 58] (median non reachable) and the RFS was 39.6 months [33.5- 45.7] with a median RFS of 37.4 months (Figure 1). The rate of complete remission (CR) was 70% (100 of 142 cases). Out of 41 patients where cause of death was recorded, 80.5% died from septic shock.
Figure 2 presents the Kaplan-Meier curves for each of the 4 permutations of risk factors: (A) living at less than 40 km of treatment center and being uninsured, (B) living at 40 km or more and being uninsured, (C) living at less than 40 km and being insured, and (D) living at 40 km or more and being insured.
Figure 2 Overall Survival according to the four permutations of risk factors. (A) <40 km and uninsured (B) ≥ 40 km and uninsured (C) <40 km and insured (D) ≥ 40 km and insured.
Multivariate Cox analysis of OS was used to adjust for potential confounding factors and showed that patients who are uninsured and those living beyond 40 km of the treatment center have worse OS than others who are fully insured and living in proximity to the specialized treatment facility. Results are statistically significant with P values of <0.0001 and 0.001, respectively (Table 2). Similarly, better OS was seen with subjects who received intensive chemotherapy (p-value= 0.08) and those who received allogenic stem cell transplant (p-value <0.0001).
There was no difference in the incidence of death by septic shock between patients living within 40 Km of treatment center and others living beyond this distance (P= 0.73). Similarly, there was no difference in the percentage of deaths in complete remission between the two groups (P= 1).
All patients with APL survived. Thus, the impact of distance traveled on OS in patients with APL cannot be assessed.
This study represents a cohort of patients diagnosed with AML from one of the largest hospitals in Lebanon. We hypothesized that patients without insurance coverage and those living farther away from treatment center would have worse survival estimates. To our knowledge, this study is the first in Lebanon to assess for potential disparities in access to healthcare of AML patients. After adjusting for confounding risk factors, the Cox regression analysis of OS showed that fully insured patients had better OS rates compared to uninsured. Similarly, patients living in proximity to our treatment center had better OS compared to those living farther away.
Ten studies concerning the impact of insurance status on survival outcomes of patients with AML have been identified (6). Our results are consistent with seven of the reported studies. A recent study by Colton et al. (17) investigated the Surveillance, Epidemiologic, and End Results database (SEER) and showed that insurance status was a predictor of death in age groups 15-10 and 25-29, favoring private insurance. Another study by Pulte et al. (16) used the SEER database too and demonstrated lower survival expectations for patients, of all ages, who are uninsured or covered with Medicaid compared to patients with other insurances. Despite the strengths of this study with large sample size, information about prognostic factors like the presence of comorbidities and cytogenetic/molecular derangements did not exist. Master et al. (18) described insurance disparities in more than 60,000 with AML using the National Cancer Data Base (NCDB). They found that uninsured patients with AML had worse outcomes than Medicaid patients, who had worse survival compared to private insurance. Other smaller studies showed similar results. A study of patients from the Virginia Cancer Registry described lower rates of receiving chemotherapy and SCT, as well as lower survival for uninsured patients compared to those with private insurance (19). Ortiz-Ortiz et al. demonstrated disparities related to insurance type in Puerto Rico in 516 cases with leukemia including 159 patients with AML, however, this report did not take into consideration other possible confounding factors (20). Borate et al. (21) utilized the SEER database for 5,541 patients aged between 19 and 64 years and found by multivariate analysis a Hazard ratio (HR) of death of 1.24 for patients with Medicaid coverage compared with private insurance.
There are many differences between the healthcare system in the US and Lebanon. However, similarities reside in the absence of a universal healthcare system. In Lebanon, healthcare coverage is provided by public and private healthcare insurances and almost 50% of the population has no formal healthcare insurance. Our findings are consistent with the mentioned studies for worse survival with lack of insurance.
Selby et al. (22) determined better survival after day 100 of receipt of allo-HCT in patients with a better-insured group. Our study did not show a separate analysis for follow-up after allo-HCT by insurance type because of our small sample size. However, we included allo-HCT in the Cox regression analysis, and the results were statistically significant. Contrary to our study, three small studies, with 2 of them from a single-institution database and one from the New York and California cancer registries, did not show any correlation between insurance type and survival (23–25).
Regarding the effect of distance from the treatment center on the survival of AML patients, the review of the literature revealed two prior studies in the US supporting our results (7, 26). One of the studies analyzed a large allo-HCT cohort from DF/BWCC and showed an apparent worse OS with increased driving distance, one year from transplant. This effect was shown to be independent of other modifiable factors and more prominent on non-relapse mortality (26). The other study from Oklahoma University reported lower rates of complete remission with increased distance. It also showed decreased OS with longer distances; however, this result was not statistically significant (7). In contrast, five other studies from the US, one from Denmark and one from Oman reported a negative association or no effect of distance on survival (8–10, 27–30). Khera et al. (29) evaluated the association between distance and overall mortality in 2849 allo-HCT patients. An association was found in non-myeloablative HCT, however, results did not reach statistical significance. Banerjee et al. (30) evaluated distress and physical function in 1136 patients 2 to 10 years post-transplant, and found no impact of driving distance. Similarly, a single center study from Oman did not show correlation between distance travelled and OS of patients who underwent allo-HCT (28). In a single-center study, Medeiros et al. (8) showed that living within 20 miles of cancer center was associated with worse OS, however, after adjusting for confounding factors, distance lost its impact on survival outcomes. A database study from Nebraska Medical Center demonstrated no effect of travel distance on OS with a non-statistically significant increased mortality in patients living > 100 miles compared to those living <25 miles from the treatment center (31). Rodriguez et al. (27) reported that distance to the cancer center had no impact on OS in those receiving remission induction therapy for AML. Similarly, a Danish study, where healthcare services are universal, showed a lack of association between distance traveled and curative-intent therapy, treatment response, and survival in AML (9).
Many potential explanations exist for the difference between our results and those reported in the literature. First of all, the Lebanese healthcare context is different from healthcare settings in the US or Denmark. In Lebanon, most of the academic tertiary referral centers are located in Beirut, the capital of Lebanon. Previous studies have shown that management of AML at academic or large-volume centers is associated with better survival outcomes (32–35). The reason behind this observation is multifactorial and might be related to earlier diagnosis, decrease time to initiation of chemotherapy, prompt management of emergencies, availability of transplant services, and clinical trials occurring in academic centers (34, 36). Thus, centralization of academic centers in Lebanon leading to increased travel distance for patients living in suburban or rural areas, explains the difficulty in access to resources for the management of AML emergencies and treatment-related complications. Second, traveling longer distances may not appear as a prognostic factor for OS in the reported studies (27, 31) because of potential confounders. In fact, patients in the US traveling to treatment centers have better performance status, educational and financial situation, and compliance to treatment (10, 27, 37, 38).
Compared with prior studies, our study is novel because it shows data from a cohort of patients diagnosed in Lebanon and simultaneously studies the effect of biological and health system factors. We have demonstrated that survival in AML patients was significantly higher in patients with better healthcare coverage and living close to the treatment center.
Our study has several limitations. First, since our study is retrospective, data on known prognostic factors including functional status, socio-economic level, and cytogenetics were unavailable or incomplete. Therefore, we cannot confirm that patients in each group had an equivalent disease. Second, our cohort size is small relatively from a single tertiary center. However, we think our results are representative of Lebanon, given that we included all patients with AML diagnosed at one of the largest hospitals in Lebanon over five years. Third, some of our patients are medical tourists and may return to their home countries after treatment. Thus, their deaths may not be recorded. Given that most medical tourists are uninsured, the effect of insurance status on survival may be underestimated. Fourth, our recorded insurance status is assessed at diagnosis. However, insurance status can change during the course of the disease, and people may lose their insurance. This may cause an underestimation of the effect of insurance.
In summary, our report detected the presence of health disparities in Lebanon according to insurance and geography. Disparities came as a consequence of a weak, privatized, and centralized healthcare system. With Lebanon sinking into one of the most severe global economic crises, we expect worse healthcare disparities affecting the socially disadvantaged groups. A true paradigm shift is urgently needed in policy making to ensure equity in access and utilization of healthcare services.
The raw data supporting the conclusions of this article will be made available by the authors, without undue reservation.
The studies involving human participants were reviewed and approved by American University of Beirut (AUB) institutional review board. Written informed consent for participation was not required for this study in accordance with the national legislation and the institutional requirements.
MM and LM collected the data, reviewed the literature, and drafted the manuscript. MC performed statistical analysis. NM, JC, AB and IA reviewed and edited the final draft. All authors have read and approved the final manuscript.
The authors declare that the research was conducted in the absence of any commercial or financial relationships that could be construed as a potential conflict of interest.
All claims expressed in this article are solely those of the authors and do not necessarily represent those of their affiliated organizations, or those of the publisher, the editors and the reviewers. Any product that may be evaluated in this article, or claim that may be made by its manufacturer, is not guaranteed or endorsed by the publisher.
1. The website of the national cancer institute. Available at: https://www.cancer.gov.
2. National Cancer Institute, Bethesda M. SEER cancer stat facts: Acute myeloid leukemia. Available at: https://seer.cancer.gov/statfacts/html/amyl.html.
3. Mohty R, Massoud R, Chakhachiro Z, Mahfouz R, Nassif S, El-Cheikh J, et al. Risk adapted therapeutic strategy in newly diagnosed acute myeloid leukemia: Refining the outcomes of ELN 2017 intermediate-risk patients. Leuk Res (2021) 105:106568. doi: 10.1016/j.leukres.2021.106568
4. Juliusson G, Antunovic P, Derolf A, Lehmann S, Mollgard L, Stockelberg D, et al. Age and acute myeloid leukemia: Real world data on decision to treat and outcomes from the Swedish acute leukemia registry. Blood (2009) 113(18):4179–87. doi: 10.1182/blood-2008-07-172007
5. O'Donnell MR, Tallman MS, Abboud CN, Altman JK, Appelbaum FR, Arber DA, et al. Acute myeloid leukemia, version 3.2017, NCCN clinical practice guidelines in oncology. J Natl Compr Canc Netw (2017) 15:926–57. doi: 10.6004/jnccn.2017.0116
6. Crotty K, Lee M, Treiman K, Sae-Hau M, Wines C, Viator C, et al. Health insurance, blood cancer, and outcomes: A literature review. Am J Manag Care (2021) 27(6 Spec No.):SP241–SP4. doi: 10.37765/ajmc.2021.88733
7. Lam DW, Maymani H, Machiorlatti MG, Srour SA, Phan M, Selby G, et al. Analysis of impact of distance from residence to treatment center on the outcome of patients with acute myeloid leukemia. Blood (2013) 122:1712. doi: 10.1182/blood.V122.21.1712.1712
8. Medeiros BC, Dunn TJ, Kohrt HE, Coutre S, Gotlib J, Liedtke M, et al. The impact of distance to treatment center on the outcome of AML. Blood (2010) 116:4742. doi: 10.1182/blood.V116.21.4742.4742
9. Tostesen M, Nørgaard M, Nørgaard JM, Medeiros BC, Marcher CW, Overgaard UM, et al. Longer distance to a specialized treatment center does not adversely affect access to treatment or outcome in acute myeloid leukemia: A national population-based cohort study. Blood (2018) 132:613. doi: 10.2147/CLEP.S210456
10. Lamont EB, Hayreh D, Pickett KE, Dignam JJ, List MA, Stenson KM, et al. Is patient travel distance associated with survival on phase II clinical trials in oncology? J Natl Cancer Inst (2003) 95(18):1370–5. doi: 10.1093/jnci/djg035
11. Salti N, Chaaban J, Raad F. Health equity in Lebanon: a microeconomic analysis. Int J Equity Health (2010) 9:11. doi: 10.1186/1475-9276-9-11
12. Worldometers.info (2022). Available at: https://www.worldometers.info/world-population/lebanon-population/.
13. Dohner H, Estey E, Grimwade D, Amadori S, Appelbaum FR, Buchner T, et al. Diagnosis and management of AML in adults: 2017 ELN recommendations from an international expert panel. Blood (2017) 129(4):424–47. doi: 10.1182/blood-2016-08-733196
14. Ording AG, Sorensen HT. Concepts of comorbidities, multiple morbidities, complications, and their clinical epidemiologic analogs. Clin Epidemiol (2013) 5:199–203. doi: 10.2147/CLEP.S45305
15. Cheson BD, Bennett JM, Kopecky KJ, Buchner T, Willman CL, Estey EH, et al. Revised recommendations of the international working group for diagnosis, standardization of response criteria, treatment outcomes, and reporting standards for therapeutic trials in acute myeloid leukemia. J Clin Oncol (2003) 21(24):4642–9. doi: 10.1200/JCO.2003.04.036
16. Pulte D, Castro FA, Brenner H, Jansen L. Outcome disparities by insurance type for patients with acute myeloblastic leukemia. Leuk Res (2017) 56:75–81. doi: 10.1016/j.leukres.2017.02.001
17. Colton MD, Goulding D, Beltrami A, Cost C, Franklin A, Cockburn MG, et al. A U.S. population-based study of insurance disparities in cancer survival among adolescents and young adults. Cancer Med (2019) 8(10):4867–74. doi: 10.1016/j.leukres.2017.02.001
18. Master S, Munker R, Shi Z, Mills G, Shi R. Insurance status and other non-biological factors predict outcomes in acute myelogenous leukemia: Analysis of data from the national cancer database. Anticancer Res (2016) 36(9):4915–21. doi: 10.21873/anticanres.11057
19. Bradley CJ, Dahman B, Jin Y, Shickle LM, Ginder GD. Acute myeloid leukemia: How the uninsured fare. Cancer (2011) 117(20):4772–8. doi: 10.1002/cncr.26095
20. Ortiz-Ortiz KJ, Ortiz-Martinez de Andino JJ, Torres-Cintron CR, Tirado-Gomez M, Gonzalez-Falero A, Caballero-Varona D, et al. Effect of type of health insurance coverage on leukemia survival in adults in Puerto Rico. P R Health Sci J (2014) 33(3):132–5. doi: 10.1371/journal.pone.0194415
21. Borate UM, Mineishi S, Costa LJ. Nonbiological factors affecting survival in younger patients with acute myeloid leukemia. Cancer (2015) 121(21):3877–84. doi: 10.1002/cncr.29436
22. Selby GB, Ali LI, Carter TH, Veseley S, Roy V. The influence of health insurance on outcomes of related-donor hematopoietic stem cell transplantation for AML and CML. Biol Blood Marrow Transplant (2001) 7(10):576. doi: 10.1016/S1083-8791(01)70018-3
23. Al-Ameri A, Anand A, Abdelfatah M, Kanaan Z, Hammonds T, Haller N, et al. Outcome of acute myeloid leukemia and high-risk myelodysplastic syndrome according to health insurance status. Clin Lymphoma Myeloma Leuk (2014) 14(6):509–13. doi: 10.1016/j.clml.2014.03.003
24. Srour SA, Machiorlatti M, Pierson NT, Bhutta UZ, Cherry M, Selby GB, et al. Impact of health care insurance status on treatment outcomes of acute myeloid leukemia. Clin Lymphoma Myeloma Leuk (2017) 17(7):450–6 e2. doi: 10.1016/j.clml.2017.05.011
25. Yung RL, Chen K, Abel GA, Gesten FC, Roohan PJ, Boscoe FP, et al. Cancer disparities in the context of Medicaid insurance: A comparison of survival for acute myeloid leukemia and hodgkin's lymphoma by Medicaid enrollment. Oncologist (2011) 16(8):1082–91. doi: 10.1634/theoncologist.2011-0126
26. Abou-Nassar KE, Kim HT, Blossom J, Ho VT, Soiffer RJ, Cutler CS, et al. The impact of geographic proximity to transplant center on outcomes after allogeneic hematopoietic stem cell transplantation. Biol Blood Marrow Transplant (2012) 18(5):708–15. doi: 10.1016/j.bbmt.2011.08.022
27. Rodriguez CP, Baz R, Jawde RA, Rybicki LA, Kalaycio ME, Advani A, et al. Impact of socioeconomic status and distance from treatment center on survival in patients receiving remission induction therapy for newly diagnosed acute myeloid leukemia. Leuk Res (2008) 32(3):413–20. doi: 10.1016/j.leukres.2007.07.015
28. Al Naabi M, Al Khabori M, Al-Huneini M, Al-Rawas A, Dennison D. Impact of home-to-Centre distance on bone marrow transplantation outcomes. Sultan Qaboos Univ Med J (2019) 19(1):e15–e8. doi: 10.18295/squmj.2019.19.01.004
29. Khera N GT, Flowers MED, Sandmaier BM, Loberiza F, Lee SJ, Appelbaum F. Association of distance from transplantation center and place of residence on outcomes after allogeneic hematopoietic cell transplantation. Biol Blood Marrow Transplant (2016) 22(7):1319–23. doi: 10.1016/jbbmt20160301
30. Banerjee R, Yi JC, Majhail NS, Jim HSL, Uberti J, Whalen V, et al. Driving distance and patient-reported outcomes in hematopoietic cell transplantation survivors. Biol. Blood Marrow Transplant (2020) 26(11):2132–8. doi: 10.1016/j.bbmt.2020.08.002
31. Dhakal P, Lyden E, Muir K-LE, Al-Kadhimi ZS, Maness LJ, Gundabolu K, et al. Effects of distance from academic cancer center on overall survival of acute myeloid leukemia: Retrospective analysis of treated patients. Clin Lymphoma Myeloma Leukemia (2020) 20(10):e685–e90. doi: 10.1016/j.clml.2020.05.016
32. Bhatt VR, Shostrom V, Armitage JO, Gundabolu K. Treatment patterns and outcomes of octogenarian patients with acute myeloid leukemia. Am J Hematol (2019) 94(6):E169–E72. doi: 10.1002/ajh.25464
33. Bhatt VR, Shostrom V, Gundabolu K, Armitage JO. Utilization of initial chemotherapy for newly diagnosed acute myeloid leukemia in the united states. Blood Adv (2018) 2(11):1277–82. doi: 10.1182/bloodadvances.2018019125
34. Hahn AW, Jamy O, Nunnery S, Yaghmour G, Giri S, Pathak R, et al. How center volumes affect early outcomes in acute myeloid leukemia. Clin Lymphoma Myeloma Leuk. (2015) 15(11):646–54. doi: 10.1016/j.clml.2015.07.646
35. Bhatt VR, Chen B, Lee SJ. Use of hematopoietic cell transplantation in younger patients with acute myeloid leukemia: A national cancer database study. Bone Marrow Transplant (2018) 53(7):873–9. doi: 10.1038/s41409-018-0105-9
36. Kelly C, Hulme C, Farragher T, Clarke G. Are differences in travel time or distance to healthcare for adults in global north countries associated with an impact on health outcomes? A systematic review. BMJ Open (2016) 6(11):e013059. doi: 10.1136/bmjopen-2016-013059
37. Pant M, Bhatt VR. Early mortality and survival in older adults with acute myeloid leukemia. Int J Hematol Oncol (2017) 6(3):61–3. doi: 10.2217/ijh-2017-0013
Keywords: acute myeloid leukemia (AML), insurance status, distance, treatment center, survival
Citation: Mahmoud M, Al Mahmasani L, Charafeddine M, Zahreddine A, Moukalled N, El Cheikh J, Bazarbachi A and Abou Dalle I (2022) Impact of insurance status and distance from residence to treatment center on the outcomes of patients diagnosed with acute myeloid leukemia. Front. Hematol. 1:1060029. doi: 10.3389/frhem.2022.1060029
Received: 02 October 2022; Accepted: 28 November 2022;
Published: 15 December 2022.
Edited by:
Francesco Di Raimondo, University of Catania, ItalyReviewed by:
Koji Sasaki, University of Texas MD Anderson Cancer Center, United StatesCopyright © 2022 Mahmoud, Al Mahmasani, Charafeddine, Zahreddine, Moukalled, El Cheikh, Bazarbachi and Abou Dalle. This is an open-access article distributed under the terms of the Creative Commons Attribution License (CC BY). The use, distribution or reproduction in other forums is permitted, provided the original author(s) and the copyright owner(s) are credited and that the original publication in this journal is cited, in accordance with accepted academic practice. No use, distribution or reproduction is permitted which does not comply with these terms.
*Correspondence: Iman Abou Dalle, aWE0MUBhdWIuZWR1Lmxi
Disclaimer: All claims expressed in this article are solely those of the authors and do not necessarily represent those of their affiliated organizations, or those of the publisher, the editors and the reviewers. Any product that may be evaluated in this article or claim that may be made by its manufacturer is not guaranteed or endorsed by the publisher.
Research integrity at Frontiers
Learn more about the work of our research integrity team to safeguard the quality of each article we publish.