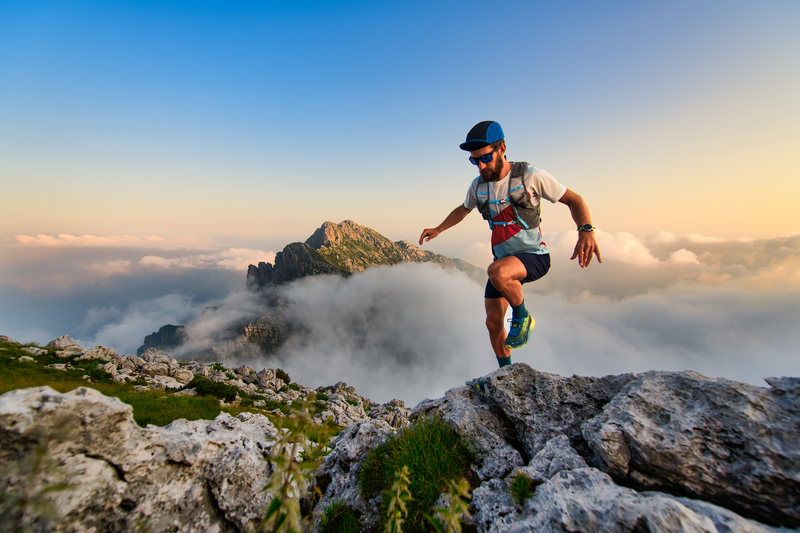
94% of researchers rate our articles as excellent or good
Learn more about the work of our research integrity team to safeguard the quality of each article we publish.
Find out more
SYSTEMATIC REVIEW article
Front. Health Serv. , 24 August 2023
Sec. Implementation Science
Volume 3 - 2023 | https://doi.org/10.3389/frhs.2023.1211150
This article is part of the Research Topic Going Beyond the Traditional Tools of Implementation Science View all 13 articles
Background: The process of translation of AI and its potential benefits into practice in healthcare services has been slow in spite of its rapid development. Trust in AI in relation to implementation processes is an important aspect. Without a clear understanding, the development of effective implementation strategies will not be possible, nor will AI advance despite the significant investments and possibilities.
Objective: This study aimed to explore the scientific literature regarding how trust in AI in relation to implementation in healthcare is conceptualized and what influences trust in AI in relation to implementation in healthcare.
Methods: This scoping review included five scientific databases. These were searched to identify publications related to the study aims. Articles were included if they were published in English, after 2012, and peer-reviewed. Two independent reviewers conducted an abstract and full-text review, as well as carrying out a thematic analysis with an inductive approach to address the study aims. The review was reported in accordance with the PRISMA-ScR guidelines.
Results: A total of eight studies were included in the final review. We found that trust was conceptualized in different ways. Most empirical studies had an individual perspective where trust was directed toward the technology's capability. Two studies focused on trust as relational between people in the context of the AI application rather than as having trust in the technology itself. Trust was also understood by its determinants and as having a mediating role, positioned between characteristics and AI use. The thematic analysis yielded three themes: individual characteristics, AI characteristics and contextual characteristics, which influence trust in AI in relation to implementation in healthcare.
Conclusions: Findings showed that the conceptualization of trust in AI differed between the studies, as well as which determinants they accounted for as influencing trust. Few studies looked beyond individual characteristics and AI characteristics. Future empirical research addressing trust in AI in relation to implementation in healthcare should have a more holistic view of the concept to be able to manage the many challenges, uncertainties, and perceived risks.
Artificial intelligence (AI) can be understood as “a computerized system that is equipped with the capacity to perform tasks or reasoning processes that we usually associated with the intelligence level of a human being” (1). These systems have the potential to transform healthcare at many levels and solve many of its current challenges (2–4), e.g., by reducing costs and workloads, improving efficiency and quality, as well as by making earlier and more accurate diagnoses (2, 5). The expectations on AI are high and the European Union (2) and the European Commission are making significant investments in AI (6).
Despite the rapid development of AI and its potential benefits when implemented in healthcare, the process of translation into practice has been slow (7). AI systems tend to be complex, unpredictable, lack evidence, and difficult to grasp, hence the many uncertainties and risks related to its use, e.g., patient harm, bias, and lack of privacy (2). Trust in AI and its trustworthiness have therefore been regarded as important aspects to address (6, 8, 9). Based on literature from other scientific fields, trust is fundamental for a functioning health system (10) where patients are in vulnerable situations since it is known to increase the tolerance of uncertainty, as well as to reduce the perceived complexity (11). Trust is understood as a way of dealing with uncertainty (12), and according to Luhmann (13), trust is an attitude which leaves room for risk-taking behavior. To be trustworthy is a characteristic of someone who is competent to perform an action and has the moral attitude toward those who depend on the performance (14, 15). Being trustworthy helps in gaining trust but does not imply trust per se (16, 17).
Most research in AI in healthcare has so far been primarily focused on AI's performance (18), fairness, trustworthiness (8, 19–22), legal and ethical issues (21–27), and transparency and explainability (19–22, 24, 27).
Aspects such as AI's influence and interaction with the context in which it is implemented are also important to consider for successful implementation of AI (28). There appears to be a general lack of empirical research investigating implementation processes in relation to AI in healthcare (7, 28, 29). Health professionals are trusted and authorized to give advice and treatment based on their profession and expertise (30–33), and an implementation of AI into practice is believed to disrupt healthcare by questioning these health professionals' existing authority, as well as influencing organizational structures, roles, and practices (1, 7, 29). The many challenges, uncertainties, and perceived risks reflect the importance of trust in AI in relation to implementation in healthcare.
In order to successfully implement AI into routine applications in healthcare and change clinical practice, an understanding of trust in AI in relation to the change processes is needed. No previous studies exploring the concept trust in AI in relation to implementation in healthcare have to our knowledge been performed, which implies there could be a lack of conceptual clarity. Without a clear understanding of trust in AI, it could be difficult to identify implementation strategies, which means that AI will not advance despite the significant investments and possibilities. The aim of this paper was thus to explore the scientific literature regarding how trust in AI is conceptualized in relation to implementation in healthcare and what influences trust in AI in relation to implementation in healthcare.
We chose a scoping review methodology to explore all relevant literature addressing trust in AI in relation to implementation in healthcare, since this methodology is useful for identifying knowledge gaps, scoping a body of literature, or clarifying concepts (34). We used the methodological framework developed by Arksey and O'Malley (35) and followed the five stages: (1) identifying the research question, (2) identifying relevant articles, (3) selecting articles, (4) charting the data, and (5) collating, summarizing, and reporting the results. The review followed the recommendations in the Preferred Reporting Items for Systematic Reviews and Meta-Analysis for Scoping Reviews (PRISMA-ScR) checklist (34), and since it was based on publicly available studies there was no ethical consideration related to the handling of personal and sensitive information. A review protocol based on Arksey and O'Malley's (35) framework was developed, and the final version of the protocol can be found in Data Sheet 1.
To address the aim, we formulated two research questions:
1. How is trust in AI conceptualized in relation to implementation in healthcare?
2. What influences trust in AI in relation to implementation in healthcare?
A thorough search for published literature was developed and carried out together with an experienced librarian. Search terms included a combination of terms related to implementation, AI, and healthcare. We used standardized subject headings describing the terms and subcategories provided by the databases. Truncation of words allowed for alternative endings and were used for implementation, improvement, innovation, and intervention. The term trust had to be specific since the aim was to explore how trust was conceptualized in AI in relation to implementation in healthcare. The electronic database search was recorded in a table (Data Sheet 2). An initial search was carried out in CINAHL and PubMed to identify keywords and subject headings, which were then included in the search strategy for the selected databases. Five electronic databases (PubMed, CINAHL, PsychINFO, Web of Science and Scopus) were systematically searched to identify relevant scientific literature. In addition, reference lists of the identified research articles were reviewed manually.
The eligibility criteria ensured that the content of the included studies was relevant to the research question (36). The focus was on trust in AI in relation to implementation in healthcare, and there was no restriction placed on the type of methodology used in the paper (e.g., qualitative, quantitative, mixed methods or theoretical). To be included, articles had to: (a) address “trust” in AI in (b) relation to implementation in healthcare. Although there are closely related terms for trust, we found it important to be specific since the aim was to conceptualize “trust” in AI in relation to its implementation in healthcare. Articles were excluded if they were non-English, not available in full text, not peer reviewed or published before 2012 (Table 1). The decision to exclude articles published before 2012 was made to allow a focus on more recent development of AI, due to its fast-changing nature. AI was uncommon in healthcare settings prior to 2012 (3).
We defined implementation as “An intentional effort designed to change or adapt or uptake interventions into routines”, which was based on a definition used by two earlier reviews with a focus on implementation of AI into healthcare practice (7, 28). We also made a distinction between trust and trustworthiness, and we excluded studies that were only mentioning trust without giving it further attention or dealing with it in relation to implementation in healthcare.
The eligible articles were uploaded into Endnote X9 software where duplicates were removed, and thereafter imported into Rayyan. The initial screening of titles and abstracts was conducted in collaboration between two reviewers (authors 1 and 2), who communicated and met regularly to discuss any disagreements or uncertainties regarding which articles to include or exclude based on selected criteria. If agreement could not be reached, the other authors were consulted through discussions. The full article was read if focus of an article was unclear based on title and abstract. In the next step, the same two reviewers (authors 1 and 2) independently conducted the full-text review on the remaining articles, and disagreements and uncertainties were again resolved through discussion with the other authors.
First, we developed a standard data charting form, following the guidelines by Arksey and O'Malley (35), based on characteristics of the articles: (1) country; (2) publication year; (3) methodological design; (4) healthcare setting; (5) aim of the study; (6) application area; (7) intended user; (8) definition of trust (Table 2). Two reviewers (authors 1 and 2) extracted the data from the articles and thereafter confirmed with the other authors. The aim was to explore all relevant literature rather than provide a quantitative or qualitative synthesis. The methodological quality or risk of bias of the included studies were therefore not reviewed, which is consistent with guidance on the conduct of scoping reviews (35, 37).
We then used a thematic analysis with an inductive approach to analyze data associated with the research questions, how trust in AI is conceptualized in relation to implementation in healthcare and what influences trust in AI in relation to implementation in healthcare. We followed the guide of Braun and Clarke (50) with six phases: (1) data familiarization; (2) initial code generation; (3) generating themes; (4) theme review; (5) theme defining and naming; (6) and report production. The first step involved reading and rereading the articles, as well as making notes. Two reviewers (authors 1 and 2) reflected individually and generated independently lists of codes from words and phrases, which were coded regarding trust in AI in relation to implementation in healthcare. The reviewers then compared their codes and interpretations, and the relationships between the codes were discussed, which were referred to as subthemes. The conceptualization of trust was either clearly defined or defined by its determinants. The subthemes were then analyzed, and three overarching themes were generated. All authors discussed continuously the data analysis to enhance its quality and validity. No qualitative data analysis software was used.
A total of 815 articles were retrieved from the five databases. Three articles were identified through manual searches of reference lists. The number of articles for review was reduced to 454 after duplicates were removed. 426 of the 454 (93.8%) were excluded in the title and abstract screening, for reasons highlighted in Figure 1. The term trust was often only mentioned, but not further addressed (n = 170). 235 articles investigated trust but not in AI in relation to implementation, thirteen articles were not in the healthcare setting, six articles were published before 2012 and two articles had no abstract. This resulted in a high number of excluded articles. Only 28 articles remained for full text review. Twelve of these articles were excluded because they only mentioned trust and did not further address or elaborate on the concept in the full text, and eight articles were excluded because they did not address trust in relation to AI implementation in healthcare. A total of eight articles met all criteria and were included in the study.
The included studies were published between 2018 and 2022. Most articles were from the United States (n = 3), two from China, and the remainder from the United Kingdom (n = 1), India (n = 1), Canada (n = 1) and Netherlands (n = 1). Many of the studies (n = 6) were conducted in hospital settings (neurological practice, radiology, dermatology, and robotic surgery), except for two studies which involved healthcare management at home and healthcare in general. AI was often used for diagnosis (n = 4). Other application areas were brain modelling (1), image recognition (1), smart healthcare services (1), treatment (1), surgery (1), communication (1). One study was too general to have a specific purpose. Four studies were based on quantitative studies, three were opinion papers, and one mixed method. The studies examined the perceptions of different intended users: clinicians (n = 4), general population (n = 2), and patients (n = 1). The characteristics of the included studies are shown in Table 2.
Six out of the eight studies provided a definition of trust (Table 2). Most empirical studies had an individual perspective where trust was directed toward the technology's capability (n = 4), e.g., describing trust as human's propensity or willingness to submit to the vulnerability of the technology's capability (39, 43, 46) or the perception of AI as being dependable, reliable, and trustworthy in supporting healthcare activities (45). Two studies had a contextual perspective and focused on trust as relational between people in the context of the AI application rather than having trust in the technology itself. Datta Burton et al. (38) argued that it is necessary to develop the human side of these tools, which represents a triangle of trust relationships: between patients and clinicians, and between clinicians and researchers. Esmaeilzadeh et al. (41) focused on care encounters and understood trust as the degree to which an individual believes that the clinical encounter is trustworthy and referred to Reddy et al. (42) who understood trust as “Trust is in the clinicians and the clinical tools they use”. Two studies only defined trust indirectly by describing trust determinants (48, 49).
The inductive coding yielded three themes regarding what influences trust in AI implementation in healthcare, which could be understood as interconnected: individual characteristics, AI characteristics, and contextual characteristics. These themes were based on 10 subthemes and 34 codes (Table 3).
Table 3. Influences of trust in relation to implementation of AI in healthcare based on inductive thematic analysis.
The individual characteristics capture those qualities that make the individuals different from each other, such as age, sex/gender, personality. These characteristics influence individuals’ trust in AI in relation to an implementation in healthcare. Demographic characteristics such as gender, age and education were found to relate to trust by moderating the relationship between antecedents and behavioral intention (n = 4). For example, being male, higher educated, employed or student, and with Western background were predictors of trust in AI among the general population (49). Disposition to trust technology (a person's general tendency to be willing to depend on technology) varied among clinicians based on their living experiences (43) and cultural background (43, 46). Knowledge and technological skills were found to influence trust in AI (n = 5), which emphasized the need for education and training (49). Four studies understood trust as influenced by earlier usage experience or technological skills (38, 43, 45, 46), e.g., radiologists were used to highly complex machines in their routine clinical practice, and ease of use may therefore not be a concern in the adoption-related decision making (46). Personal traits such as cognition and having a positive attitude were associated with higher levels of trust (n = 3), e.g., disposition to trust technology was related to trust in AI use (43, 46), and understood as influenced by the individual's cognition and personality (46). Health conditions and healthcare consumption were also something that influenced trust (n = 2), e.g., individuals with chronic conditions may not trust AI clinical applications if no physician interaction were included in healthcare delivery (41) and individuals who utilized less healthcare were associated with a higher level of trust in AI (49).
Trust in relation to the characteristics of AI was frequently mentioned in the literature, where aspects such as its performance, capacity, and trustworthiness were focused on. AI's ability to individualization was shown to enhance trust, which was understood as care tailored to the patients' unique needs (n = 2). Personalization was based on patients' health information, which required sharing sensitive personal data and caused concerns such as risks of privacy breaches (41, 45). AI's anthropomorphic characteristics enhanced trust in AI in relation to an implementation since it generated a sense of social presence. It was referred to as the perceived level of humanlike characteristics such as human appearance, self-consciousness, and emotion (45). AI characteristics such as “black box”, self-learning, non-transparent and autonomous characteristics brought uncertainty and threatened trust in the implementation of AI (n = 5), since inputs and operations were not visible to the user. Technical objectivity, which included characteristics such as data-driven, accuracy, lack of moral values, and lack of empathy, was also related to trust (n = 5), since they in some cases could produce results that were more accurate and reliable than those of even the most skilled diagnostician (38).
The theme contextual characteristics concerned the influence on trust in AI in relation to implementation in healthcare regarding the context in which individuals and AI are embedded. The contextual characteristics in relation to implementation of AI in healthcare consisted of the following subthemes: healthcare culture, interpersonal relationships, and governance. Healthcare culture included medical area, professional expertise, and opinion of important others (n = 5). For example, a “skilled clinician” was considered someone who had embodied tacit knowledge through years of experience in a community of experts (38). Opinion of important others, such as clinicians, colleagues, and seniors, shaped individuals’ initial trust (43, 46). Trust in AI in relation to implementation in healthcare depended also on the medical area, e.g., the perceived risks of using AI in radiology and dermatology compared to robotic surgery (49). Interpersonal relationship, collaboration, personal interactions, and mutual understanding were found to influence trust (n = 4), especially between different stakeholders (38, 48). Thus, reduced communication in relation to AI implementation was believed to result in less trust among patients (41, 49). Yakar et al. (49) investigated trust in AI in the areas of radiology, surgery and dermatology, and the results showed that those who found personal interactions important had less trust in all three areas. Governance, including policies, standards, and guidelines had to be defined to enhance trust in AI (n = 4). The lack of clear guidelines in medical context was believed to lead to more uncertainties and less trust (41). Roski et al. (48) highlighted the importance of different stakeholder-consented framework and goals to enhance trust, which was also a condition for self-governance. Datta Burton et al. (38) suggested policies that encourage greater clinician engagement in the evaluation of a computational model that would lead to more responsible adoption.
This study was conducted to explore the scientific literature regarding how trust in AI is conceptualized in relation to implementation in healthcare and what influences trust in AI in relation to implementation in healthcare. Only eight studies were found to meet the strict inclusion criteria. The results showed that the conceptualization of trust in AI differed between the studies, as well as what they accounted for as influencing trust. We identified three themes that influenced trust in AI in relation to implementation in healthcare: individual characteristics, AI characteristics and contextual characteristics. Most research focused on the individual characteristics or AI characteristics, and the focus was rarely on the context or implementation processes.
AI in healthcare is a relatively new endeavor but the use of AI has become more common in healthcare setting during the past decade (3). Studies on the implementation of AI in healthcare are therefore fairly new research areas. This could explain the low number of included studies, which all were recently published and mostly from high income countries. Another explanation for the low number could be that trust is rarely mentioned in implementation science frameworks, theories, or models (51). The findings showed that the intended users were often clinicians (38, 39, 43, 46), which also aligns with implementation science where the focus is on clinicians rather than patients. Most of the empirical studies were cross-sectional where questionnaires were used to measure trust as the individual's attitudes and perceptions of AI's capability (41, 43, 45, 49) rather than considering other influencing variables. These studies discussed AI at a general level where the individuals had no or very little experience with practical AI tools, instead of addressing trust where the tools have been implemented and used over longer periods. One should thus be careful in using these perspectives in the development of implementation strategies to avoid building strategies on opinions, perceptions, and potential misconceptions rather than on actual experiences. Moreover, these fairly superficial perspectives on trust in AI in relation to implementation give little insight since they do not consider the context and the underlying values.
The conceptualization of trust in AI in relation to implementation in healthcare differed between the included studies. Some studies focused on individual characteristics and AI characteristics (39, 43, 45, 46, 49), and other studies concentrated on the relations between people (38, 41). Trust in AI in relation to implementation in healthcare did not always have a specific definition. Instead, it was understood indirectly as influenced by different characteristics or determinants, and as having a mediating role, positioned between perceptions of AI characteristics and AI use. These different approaches to trust in AI reveal its complexity and the need of having a holistic understanding of the concept spanning different levels and dimensions.
The three themes that was found to influence trust in AI in relation to implementation in healthcare can be compared to implementation science, which emphasizes the determinants that influence the implementation by understanding the context in which they are used (52, 53). In line with Leeman et al. (54). The determinants to facilitate implementation need to be known for appropriate strategies to be chosen. The themes are well-aligned with the Consolidated Framework for Implementation Research (CFIR), which is one of the most widely used determinant frameworks in implementation science (Figure 2). Trust could be placed in the assessment category in CFIR, situated between determinants and outcomes, where also the concepts of acceptability, appropriateness, feasibility, implementation readiness and implementation climate are placed (55).
Figure 2. The determinants associated with trust in AI in relation to implementation in healthcare mapped onto CFIR domains and Constructs (55).
The theme individual characteristics such as an individual's circumstances was shown to influence trust in AI (38, 41, 43, 45, 46, 49). The result showed that individuals in vulnerable positions (less educated people, unemployed, people with non-Western immigration background, older people, and patients with chronic conditions) had low degree of trust in AI (49). Hence, the relationship between trust and the individuals' perception of control or empowerment. This may be consistent with Luhmann (11) who argued that people are willing to trust if they possess inner security. Moreover, perceptions of AI characteristics such as being a non-transparent “black box” with autonomous and self-learning capacity were related to lack of trust in AI since these characteristics obstruct the understanding of its decisions. Knowledge and technological skills were other aspects that were shown to enhance trust in AI, which may also be understood as related to control or empowerment.
This study showed that trust in AI in relation to implementation in healthcare may be related to knowledge within a context. People's perception of AI as meaningful, useful, or valuable contributed to trust (38, 39, 41, 43, 45, 46). The results showed that trust in AI was not only influenced by its “technical” objectivity, efficiency, and accuracy. For example, person-centered care does not only consider medical competence as technical skills but also relational moral competency, empathy, compassion, and trust (41), which could explain why AI's anthropomorphic characteristics and personalization enhanced trust in AI (45). Healthcare culture is based on knowledge within a context and could be why the individuals' trust in AI was often shaped by important others (43, 46, 49), as well as why interpersonal relationships, collaboration and common understanding were found to influence trust (38, 41, 48, 49). It also explains the importance of governance and the need of common guidelines (38, 39, 41, 48).
Knowledge within a context and its influence on trust in AI in relation to implementation in healthcare could be compared to Normalization Process Theory (NPT), another widely used theoretical approach in implementation science. The theory understands implementation as a possible challenge toward individuals' existing ways of working or thinking about care (56). NPT suggests that people need to make sense of AI together to understand their specific roles and responsibilities in relation to AI use in healthcare, and the importance of new agreements and values that give meanings to their actions (57). This could be explained by our ability to contextualize information through narratives (58), which is also in line with Luhmann (11) who viewed trust as possible only in a familiar world.
Only considering AI's technical aspects when implementing AI in healthcare is not enough. AI tools should not be understood apart from the context and the people using them. Existing values and understanding of care can become barriers to trust in AI in relation to implementation in healthcare if there is a lack of coherence. There is thus a need to understand the context in relation to implementation (59) to be able to align AI to existing values (38, 57). Differences in values must be considered for trust to be present when implementing AI in healthcare. The use of AI could thus add value to clinical reasoning rather than competing with it according to Datta Burton et al. (38).
The study has some strengths that are worth highlighting. The search was designed together with a librarian and the selection of relevant studies were conducted independently by two reviewers with consensus. We used a comprehensive search strategy and adhered to a structure for scoping reviews outlined by Arksey and O'Malley (35).
The study also has shortcomings that must be considered when interpreting the findings. Trust in AI in relation to implementation in healthcare relates to a young research field, and we found it therefore necessary to include any type of methodology in this study. This means the conceptualization of trust in AI was based on both results and reflections. The study was limited to the published literature in English, and we did not search wider grey literature where we may have identified additional relevant literature. Only a small number of articles met the strict inclusion criteria since many of the articles were excluded because they only mentioned trust or did not address trust in AI in relation to implementation in healthcare. Most of the included studies were conducted in high-income countries and the results may therefore not be relevant to other countries.
This scoping review showed that there were different approaches to trust, which demonstrates that trust can be understood at different levels and dimensions. Only considering one aspect could mean that inappropriate strategies are used to support implementation. For example, there were few empirical studies that addressed trust beyond individual characteristics and AI characteristics. Future empirical studies thus need to have a holistic view on trust. The results also showed that in order to establish trust in AI in relation to implementation in healthcare, it is important to align AI to existing values and to take account of social interactions and negotiants of values in relation to care. This scoping review also found that trust in AI was often influenced by the opinion of important others (43, 46). Future studies could therefore investigate how these important others facilitate trust in AI in relation to implementation in healthcare. Three of the included studies mentioned that trust grows with time and maturity (39, 43, 46). However, none of these studies investigated this change empirically. There is therefore also a need for a better understanding of how trust in AI changes during implementation in healthcare.
Findings from the scoping review revealed that there is a variation in the scientific literature how trust in AI in relation to its implementation in healthcare has been conceptualized. Trust is often conceptualized by its determinants and having a mediating role, positioned between characteristics and AI use. There were also differences in what was believed to influence trust in AI. We found three themes that influenced trust in AI in relation to implementation in healthcare: individual characteristics, AI characteristics and contextual characteristics. Today, most research focuses only on one or two perspectives, for example the individual characteristics or the AI characteristics. Future studies addressing trust in AI in relation to implementation in healthcare should have a more holistic view on trust to be able to manage the many challenges and develop appropriate strategies to support the implementation of AI in healthcare.
The original contributions presented in the study are included in the article/Supplementary Material, further inquiries can be directed to the corresponding author/s.
ES conceptualized the study with input from author PN, PS and JN. All authors contributed to the study design. ES retrieved the records from the databases. Authors ES and ES participated in the screening process and the extraction of the data. Data analysis was performed by authors ES and ES, and then discussed with all authors. The manuscript was drafted by ES with input from the other authors. All authors thereafter drafted and revised the manuscript and approved the final version.
Knowledge Foundation (grant 20200208 01H) and the Swedish Research Council (grant 2022054 06). The funders were not involved in any aspect of study design, collection, analysis, interpretation of data, or in the writing or publication design.
The authors declare that the research was conducted in the absence of any commercial or financial relationships that could be construed as a potential conflict of interest.
The author PN declared that they were an editorial board member of Frontiers, at the time of submission. This had no impact on the peer review process and the final decision.
All claims expressed in this article are solely those of the authors and do not necessarily represent those of their affiliated organizations, or those of the publisher, the editors and the reviewers. Any product that may be evaluated in this article, or claim that may be made by its manufacturer, is not guaranteed or endorsed by the publisher.
The Supplementary Material for this article can be found online at https://www.frontiersin.org/articles/10.3389/frhs.2023.1211150/full#supplementary-material
1. Petersson L, Larsson I, Nygren JM, Nilsen P, Neher M, Reed JE, et al. Challenges to implementing artificial intelligence in healthcare: a qualitative interview study with healthcare leaders in Sweden. BMC Health Serv Res. (2022) 22:850. doi: 10.1186/s12913-022-08215-8
2. EPRS. Artificial intelligence in healthcare: Applications, risks, and ethical and societal impacts (2022). https://www.europarl.europa.eu/RegData/etudes/STUD/2022/729512/EPRS_STU(2022)729512_EN.pdf (Accessed November 22, 2022).
3. Topol EJ. High-performance medicine: the convergence of human and artificial intelligence. Nat Med. (2019) 25:44–56. doi: 10.1038/s41591-018-0300-7
4. Bajwa J, Munir U, Nori A, Williams B. Artificial intelligence in healthcare: transforming the practice of medicine. Future Healthcare J. (2021) 8(2):e188–94. doi: 10.7861/fhj.2021-0095
5. Mehta N, Pandit A, Shukla S. Transforming healthcare with big data analytics and artificial intelligence: a systematic mapping study. J Biomed Inform. (2019) 100:103311. doi: 10.1016/j.jbi.2019.103311
6. European Commission. A European approach to artificial intelligence (2022). https://digital-strategy.ec.europa.eu/en/policies/european-approach-artificial-intelligence (Accessed November 9, 2022).
7. Sharma M, Savage C, Nair M, Larsson I, Svedberg P, Nygren JM. Artificial intelligence application in health care practice: scoping review. J Med Internet Res. (2022) 24:e40238. doi: 10.2196/40238
8. HLEG. Ethics guidelines for trustworthy AI (2019). https://digital-strategy.ec.europa.eu/en/library/ethics-guidelines-trustworthy-ai (Accessed March 2, 2023).
9. Gille F, Jobin A, Ienca M. What we talk about when we talk about trust: theory of trust in healthcare. Intell-Based Med. (2020) 1-2:100001. doi: 10.1016/j.ibmed.2020.100001
10. Gille F, Smith S, Mays N. Why public trust in health care systems matters and deserves greater research attention. J Health Serv Res Policy. (2015) 20(1):62–4. doi: 10.1177/1355819614543161
12. Asan O, Emrah Bayrak A, Choudhury A. Artificial intelligence and human trust in healthcare: focus on clinicians. J Med Internet Res. (2020) 22:e15154. doi: 10.2196/15154
13. Luhmann N. Familiarity, confidence, trust: problems and alternatives. In: Gambetta D, editors. Trust: Making and breaking cooperative relations. Oxford: University of Oxford (2000). p. 94–107.
14. Dlugatch R, Georgieva A, Kerasidou A. Trustworthy artificial intelligence and ethical design: public perceptions of trustworthiness of an AI-based decision-support tool in the context of intrapartum care. BMC Med Ethics. (2023) 24:42. doi: 10.1186/s12910-023-00917-w
16. Ryan M. In AI we trust: ethics, artificial intelligence, and reliability. Sci Eng Ethics. (2020) 26(4):2749–67. doi: 10.1007/s11948-020-00228-y
17. O’Neill O. Linking trust to trustworthiness. Int J Philos Stud. (2018) 26(2):293–300. doi: 10.1080/09672559.2018.1454637
18. Fernandes M, Vieira SM, Leite F, Palos C, Finkelstein S, Sousa JMC. Clinical decision support systems for triage in the emergency department using intelligent systems: a review. Artif Intell Med. (2020) 102:101762. doi: 10.1016/j.artmed.2019.101762
19. Zhang J, Zhang Z-M. Ethics and governance of trustworthy medical artificial intelligence. BMC Med Inform Decis Mak. (2023) 23:7. doi: 10.1186/s12911-023-02103-9
20. Obermeyer Z, Powers B, Vogeli C, Mullainathan S. Dissecting racial bias in an algorithm used to manage the health of populations. Science. (2019) 366:447–53. doi: 10.1126/science.aax2342
21. Siala H, Wang Y. SHIFTing artificial intelligence to be responsible in healthcare: a systematic review. Soc Sci Med. (2022) 296:114782. doi: 10.1016/j.socscimed.2022.114782
22. Trocin C, Mikalef P, Papamitsiou Z, Conboy K. Responsible AI for digitial health: a synthesis and a research agenda. Info Syst Front. (2021). doi: 10.1007/s10796-021-10146-4
23. Gooding P, Kariotis T. Ethics and law in research on algorithmic and data-driven technology in mental health care: scoping review. JMIR Ment Health. (2021) 8:e24668. doi: 10.2196/24668
24. Čartolovni A, Tomičić A, Lazić Mosler E. Ethical, legal, and social consideration of AI-based medical-support tools: a scoping review. Int J Med Inf. (2022) 161:104738. doi: 10.1016/j.ijmedinf.2022.104738
25. Beil M, Proft I, van Heerden D, Sviri S, van Heerden PV. Ethical consideration about artificial intelligence for prognosis in intensive care. Intensive Care Med Exp. (2020) 7:70. doi: 10.1186/s40635-019-0286-6
26. Murphy K, Di Ruggiero E, Upshur R, Willison DJ, Malhotra N, Cai JC, et al. Artificial intelligence for good health: a scoping review of the ethics literature. BMC Med Ethics. (2021) 22:14. doi: 10.1186/s12910-021-00577-8
27. Coeckelberg M. Ethics of artificial intelligence: some ethical issues and regulatory challenges. Technol Regul. (2019) 1:31–4. doi: 10.26116/techreg.2019.003
28. Gama F, Tyskbo D, Nygren J, Barlow J, Reed J, Svedberg P. Implementation frameworks for artificial intelligence translation into health care practice: scoping review. J Med Internet Res. (2022) 24:e32215. doi: 10.2196/32215
29. Svedberg P, Reed J, Nilsen P, Barlow J, Macrae C, Nygren J. Toward successful implementation of artificial intelligence in health care practice: protocol for a research program. JMIR Res Protoc. (2022) 11:e34920. doi: 10.2196/34920
31. Asan O, Yu Z, Crotty BH. How clinician-patient communication affects trust in health information sources: temporal trends from a national cross-sectional survey. PLoS ONE. (2021) 16:e0247583. doi: 10.1371/journal.pone.0247583
32. Kerasidou A. Artificial intelligence and the ongoing need for empathy, compassion and trust in healthcare. Bull World Health Organ. (2020) 98:245–50. doi: 10.2471/BLT.19.237198
33. Marková I. The dialogical mind. Common sense and ethics. Cambridge: Cambridge University Press (2016). 260.
34. Tricco AC, Lillie E, Zarin W, O'Brien KK, Colquhoun H, Levac D, et al. PRISMA Extension for scoping reviews (PRISMA-ScR): checklist and explanation. Ann Intern Med. (2018) 169:467–73. doi: 10.7326/M18-0850
35. Arksey H, O’Malley L. Scoping studies: towards a methodological framework. Int J Soc Res Methodol. (2005) 8:19–32. doi: 10.1080/1364557032000119616
36. Booth A, Sutton A, Clowes M, Martyn-St James M. Systematic approach to a successful literature review. London: Sage Publications (2021). 424.
37. Peters MDJ, Marnie C, Colquhoun H, Garritty CM, Hempel S, Horsley T, et al. Scoping reviews: reinforcing and advancing the methodology and application. Syst Rev. (2021) 10(263):1–6. doi: 10.1186/s13643-021-01821-3
38. Datta Burton S, Mahfoud T, Aicardi C, Rose N. Clinical translation of computational brain models: understanding the salience of trust in clinician-researcher relationships. Interdiscip Sci Rev. (2021) 46:1–2. doi: 10.1080/03080188.2020.1840223
39. Choi HH, Chang SD, Kohli MD. Implementation and design of artificial intelligence in abdominal imaging. Abdom Radiol. (2020) 45:4084–9. doi: 10.1007/s00261-020-02471-0
40. Sheridan TB. Individual differences in attributes of trust in automation: measurement and application to system design. Front Psychol. (2019) 10:1117. doi: 10.3389/fpsyg.2019.01117
41. Esmaeilzadeh P, Mirzaei T, Dharanikota S. Patients’ perception toward human—artificial intelligence interaction in health care: experimental study. JMIR. (2021) 23:e25856. doi: 10.2196/25856
42. Reddy S, Allan S, Coghlan S, Cooper PA. A governance model for the application of AI in health care. J Am Med Inform Assoc. (2020) 27:491–7. doi: 10.1093/jamia/ocz192
43. Fan W, Liu J, Zhu W, Pardalos PM. Investigating the impacting factors for the healthcare professionals to adopt artificial intelligence-based medical diagnosis support system (AIMDSS). Ann Oper Res. (2018) 294:567–92. doi: 10.1007/s10479-018-2818-y
44. McKnight DH. Trust in information technology. In: Davis GB, editors. The blackwell encyclopedia of management. Vol. 7 management information systems. Malden, MA: Blackwell (2005). p. 329–31.
45. Liu K, Tao D. The roles of trust, personalization, loss of privacy, and anthropomorphism in public acceptance of smart healthcare services. Comput Human Behav. (2022) 127:107026. doi: 10.1016/j.chb.2021.107026
46. Prakash AW, Das S. Medical practitioner’s adoption of intelligent clinical diagnostic decision support systems: a mixed-methods study. Info Manage. (2021) 58:103524. doi: 10.1016/j.im.2021.103524
47. Mayer RC, Davis JH, Schoorman FD. An integrative model of organizational trust. Acad Manage Rev. (1995) 20:709–34. doi: 10.2307/258792
48. Roski J, Maier EJ, Vigilante K, Kane EA, Matheny ME. Enhancing trust in AI through industry self-governance. J Am Med Inform Assoc. (2021) 28:1582–90. doi: 10.1093/jamia/ocab065
49. Yakar D, Ongena YP, Kwee TC, Haan M. Do people favor artificial intelligence over physicians? A survey among the general population and their view on artificial intelligence in medicine. Value Health. (2021) 25:374–81. doi: 10.1016/j.jval.2021.09.004
50. Braun V, Clarke V. Thematic analysis. In: Cooper H, editors. APA Handbook of research methods in psychology: research designs. Washington, DC: American Psychological Association (2022). p. 57–91.
51. Nilsen P. Overview of theories, models and frameworks in implementation science. In: Nilsen P, Birken SA, editors. Handbook on implementation science. Cheltenham: Edward Elgar Publishing Limited (2020). p. 8–31. https://www.elgaronline.com/display/edcoll/9781788975988/9781788975988.00008.xml
52. Damschroder LJ. Clarity out of chaos: use of theory in implementation research. Psychiatry Res. (2020) 283:112461. doi: 10.1016/j.psychres.2019.06.036
53. May CR, Mair F, Finch T, MacFarlane A, Dowrick C, Treweek S, et al. Development of a theory of implementation and integration: normalization process theory. Implement Sci. (2009) 4:29. doi: 10.1186/1748-5908-4-29
54. Leeman J, Birken SA, Powell BJ, Rohweder C, Shea CM. Beyond “implementation strategies”: classifying the full range of strategies used in implementation science and practice. Implement Sci. (2017) 12:125. doi: 10.1186/s13012-017-0657-x
55. Damschroder LJ, Reardon CM, Opra Widerquist MA, Lowery J. Conceptualizing outcomes for use with the consolidated framework for implementation research (CFIR): the CFIR outcomes addendum. Implement Sci. (2022) 17:7. doi: 10.1186/s13012-021-01181-5
56. May C, Cummings A, Girling M, Bracher M, Mair FS, May CM, et al. Using normalization process theory in feasibility studies and process evaluations of complex healthcare interventions: a systematic review. Implement Sci. (2018) 13:18. doi: 10.1186/s13012-018-0758-1
57. May CR, Albers B, Bracher M, Finch TL, Gilbert A, Girling M, et al. Translational framework for implementation evaluation and research: a normalization process theory coding manual for qualitative research and instrument development. Implement Sci. (2022) 17:19. doi: 10.1186/s13012-022-01191-x
58. Coeckelberg M. Narrative responsibility and artificial intelligence: how AI challenges human responsibility and sense-making. AI Soc. (2021):1–4. doi: 10.1007/s00146-021-01375-x
Keywords: trust, artificial intelligence, implementation, healthcare, scoping review
Citation: Steerling E, Siira E, Nilsen P, Svedberg P and Nygren J (2023) Implementing AI in healthcare—the relevance of trust: a scoping review. Front. Health Serv. 3:1211150. doi: 10.3389/frhs.2023.1211150
Received: 24 April 2023; Accepted: 11 August 2023;
Published: 24 August 2023.
Edited by:
Marjan Askari, Erasmus University Rotterdam, NetherlandsReviewed by:
David Sommerfeld, University of California, San Diego, United States© 2023 Steerling, Siira, Nilsen, Svedberg and Nygren. This is an open-access article distributed under the terms of the Creative Commons Attribution License (CC BY). The use, distribution or reproduction in other forums is permitted, provided the original author(s) and the copyright owner(s) are credited and that the original publication in this journal is cited, in accordance with accepted academic practice. No use, distribution or reproduction is permitted which does not comply with these terms.
*Correspondence: Emilie Steerling ZW1pbGllLnN0ZWVybGluZ0BoaC5zZQ==
Disclaimer: All claims expressed in this article are solely those of the authors and do not necessarily represent those of their affiliated organizations, or those of the publisher, the editors and the reviewers. Any product that may be evaluated in this article or claim that may be made by its manufacturer is not guaranteed or endorsed by the publisher.
Research integrity at Frontiers
Learn more about the work of our research integrity team to safeguard the quality of each article we publish.