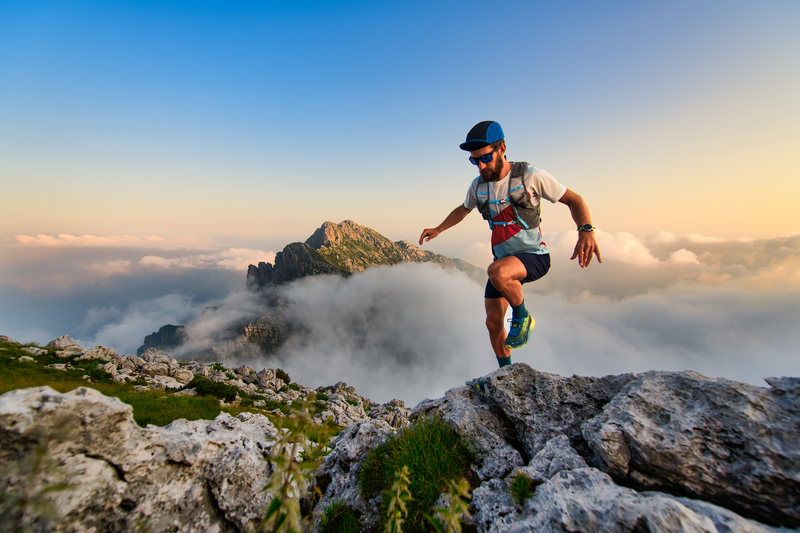
94% of researchers rate our articles as excellent or good
Learn more about the work of our research integrity team to safeguard the quality of each article we publish.
Find out more
ORIGINAL RESEARCH article
Front. Glob. Womens Health
Sec. Maternal Health
Volume 6 - 2025 | doi: 10.3389/fgwh.2025.1368575
This article is part of the Research Topic Use of Artificial Intelligence to Improve Maternal and Neonatal Health in Low-Resource Settings View all 4 articles
The final, formatted version of the article will be published soon.
You have multiple emails registered with Frontiers:
Please enter your email address:
If you already have an account, please login
You don't have a Frontiers account ? You can register here
A machine-learning-based paradigm, combining unsupervised and supervised components, is proposed for the problem of real-time monitoring and decision support during labour, addressing the limitations of current state-of-the-art approaches, such as the partograph or purely supervised models.The proposed approach is illustrated with World Health Organisation's Better Outcomes in Labour Difficulty (BOLD) prospective cohort study data, including 9995 women admitted for labour in 2014-2015 in thirteen major regional health care facilities across Nigeria and Uganda. Unsupervised dimensionality reduction is used to map complex labour data to a visually intuitive space. In this space, an ongoing labour trajectory can be compared to those of a historical cohort of women with similar characteristics and known outcomes -this information can be used to estimate personalised "healthy" trajectory references (and alert the healthcare provider to significant deviations), as well as draw attention to high incidences of different interventions/adverse outcomes among similar labours. To evaluate the proposed approach, the predictive value of simple risk scores quantifying deviation from normal progress and incidence of complications among similar labours is assessed in a caesarean section prediction context and compared to that of the partograph and state-of-the-art supervised machine-learning models.Results. Considering all women, our predictors yielded sensitivity and specificity of ~0.70. It was observed that this predictive performance could increase or decrease when looking at different subgroups.Discussion. With a simple implementation, our approach outperforms the partograph and matches the performance of state-of-the-art supervised models, while offering superior flexibility and interpretability as a real-time monitoring and decision-support solution.
Keywords: machine learning, unsupervised learning, Maternal health, labour, Monitoring, trajectory analysis, Decision Support Language style: British English
Received: 10 Jan 2024; Accepted: 17 Feb 2025.
Copyright: © 2025 Nogueira, Sanchez-Martinez, Piella, De Craene, Yagüe, Martí Castellote, Bonet, Oladapo and Bijnens. This is an open-access article distributed under the terms of the Creative Commons Attribution License (CC BY). The use, distribution or reproduction in other forums is permitted, provided the original author(s) or licensor are credited and that the original publication in this journal is cited, in accordance with accepted academic practice. No use, distribution or reproduction is permitted which does not comply with these terms.
* Correspondence:
Sergio Sanchez-Martinez, BCN MedTech (UPF), Barcelona, Catalonia, Spain
Disclaimer: All claims expressed in this article are solely those of the authors and do not necessarily represent those of their affiliated organizations, or those of the publisher, the editors and the reviewers. Any product that may be evaluated in this article or claim that may be made by its manufacturer is not guaranteed or endorsed by the publisher.
Research integrity at Frontiers
Learn more about the work of our research integrity team to safeguard the quality of each article we publish.