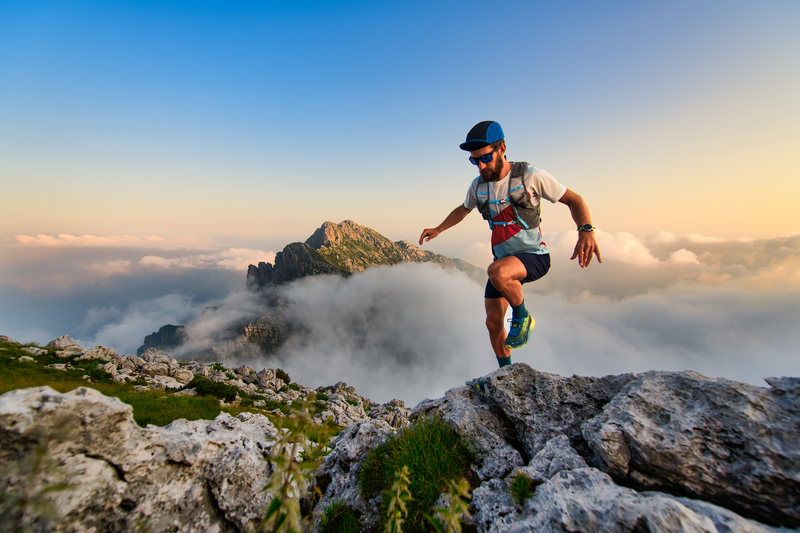
94% of researchers rate our articles as excellent or good
Learn more about the work of our research integrity team to safeguard the quality of each article we publish.
Find out more
ORIGINAL RESEARCH article
Front. Glob. Womens Health , 21 December 2023
Sec. Aging in Women
Volume 4 - 2023 | https://doi.org/10.3389/fgwh.2023.1320640
This article is part of the Research Topic Women’s Brain Health and Aging through the Lifespan View all 11 articles
Introduction: The menopause transition is associated with several cardiometabolic risk factors. Poor cardiometabolic health is further linked to microvascular brain lesions, which can be detected as white matter hyperintensities (WMHs) using T2-FLAIR magnetic resonance imaging (MRI) scans. Females show higher risk for WMHs post-menopause, but it remains unclear whether changes in cardiometabolic risk factors underlie menopause-related increase in brain pathology.
Methods: In this study, we assessed whether cross-sectional measures of cardiometabolic health, including body mass index (BMI) and waist-to-hip ratio (WHR), blood lipids, blood pressure, and long-term blood glucose (HbA1c), as well as longitudinal changes in BMI and WHR, differed according to menopausal status at baseline in 9,882 UK Biobank females (age range 40–70 years, n premenopausal = 3,529, n postmenopausal = 6,353). Furthermore, we examined whether these cardiometabolic factors were associated with WMH outcomes at the follow-up assessment, on average 8.78 years after baseline.
Results: Postmenopausal females showed higher levels of baseline blood lipids (HDL = 0.14, p < 0.001, LDL = 0.20, p < 0.001, triglycerides = 0.12, p < 0.001) and HbA1c ( = 0.24, p < 0.001) compared to premenopausal women, beyond the effects of age. Over time, BMI increased more in the premenopausal compared to the postmenopausal group ( = −0.08, p < 0.001), while WHR increased to a similar extent in both groups ( = −0.03, p = 0.102). The change in WHR was however driven by increased waist circumference only in the premenopausal group. While the group level changes in BMI and WHR were in general small, these findings point to distinct anthropometric changes in pre- and postmenopausal females over time. Higher baseline measures of BMI, WHR, triglycerides, blood pressure, and HbA1c, as well as longitudinal increases in BMI and WHR, were associated with larger WMH volumes ( range = 0.03–0.13, p ≤ 0.002). HDL showed a significant inverse relationship with WMH volume ( = −0.27, p < 0.001).
Discussion: Our findings emphasise the importance of monitoring cardiometabolic risk factors in females from midlife through the menopause transition and into the postmenopausal phase, to ensure improved cerebrovascular outcomes in later years.
The menopause is a natural biological process that characterises the change from reproductive to post-reproductive life among females. The phase leading up to the cessation of menstrual cycles, known as perimenopause, involves irregular menstrual cycles, hormonal fluctuations, and a gradual decline in ovarian function. Decreasing endogenous oestradiol levels during the menopause transition have been associated with increased risk for poor cardiometabolic health, including abdominal adiposity, dyslipidaemia, diabetes, and hypertension (1–7). Poor cardiometabolic health is a key risk factor for white matter (WM) lesions or areas of dysmyelination in the brain (8, 9), which can be quantified using WM hyperintensities (WMH) from magnetic resonance imaging (MRI) scans (10, 11). Although WMHs are common with advancing age (9, 12), larger WMH volumes have also been associated with increased risk of dementia (13–16), of which females have higher prevalence (17, 18). Pertinently, a number of studies report greater WMH load in postmenopausal females compared to age-matched males or premenopausal females (19–25). It is however unclear whether changes in cardiometabolic risk across menopausal years and beyond are linked to WMH outcomes.
Both cross-sectional and longitudinal studies indicate that the menopause transition poses a risk for accumulation of abdominal adipose tissue (6, 26–28) and an unfavourable lipid profile (4, 5, 29, 30), beyond the risk related to advancing age. However, higher levels of abdominal adiposity, blood lipids, blood pressure (BP), and blood glucose are generally linked to greater WMH volumes (8, 31–41), and it is challenging to disentangle menopause-specific risks from those linked to increasing age (6). In addition, recent studies show age- and sex-specific associations between cardiometabolic risk factors and brain measures (24, 42, 43), indicating dynamic body-brain relationships across the lifespan. To our knowledge, no population-based studies have yet assessed the relationships between both cross-sectional and longitudinal measures of cardiometabolic risk and WMH outcomes in pre- and postmenopausal females.
In the present study, we aimed to investigate associations between markers of cardiometabolic health, menopause status, and WMH volumes in 9,882 female UK Biobank participants. First, we examined whether females who were pre- and postmenopausal, as categorised based on self-reports at the baseline assessment, differed on baseline measures of body anthropometrics (body mass index (BMI) and waist-to-hip ratio (WHR)), blood lipids (high density lipoprotein (HDL), low density lipoprotein (LDL), and triglycerides), BP (systolic and diastolic), and long-term glucose levels (glycated haemoglobin; HbA1c). Next, we assessed changes in BMI and WHR between baseline and the imaging timepoint (timepoint 2; mean assessment interval 8.78 years) by menopause status. Lastly, we examined the relationships of the baseline markers and the longitudinal BMI and WHR changes with WMH volume measured at timepoint 2.
The initial sample was drawn from the UK Biobank cohort (www.ukbiobank.ac.uk), and included 21,930 female participants with data entries across self-reported demographic factors (education, ethnic background, and assessment location), blood lipids (HDL, LDL, and triglycerides), BP (systolic and diastolic), and HbA1c measurements at baseline, WMH volume, hysterectomy (removal of the uterus), and bilateral oophorectomy (removal of both ovaries) at timepoint 2, and body anthropometrics (BMI and WHR), age, and menopausal status at both timepoints. An overview of the variables, including their UK Biobank data-fields, is available in Supplementary Table S1. Participants with missing values (“Not a Number (NaN),” “prefer not to answer,” “do not know”), were excluded (missing datapoints 520 for demographic factors, 2,974 for WMH volume, 8,448 for cardiometabolic risk factors, 4,562 for menopausal status, and 101 for hysterectomy/bilateral oophorectomy, with 10% of all participants having missing values for more than 2 variables). 1,015 participants who had undergone a hysterectomy and/or bilateral oophorectomy were excluded in order to focus the study on variation in natural menopause, as surgical menopause may involve independent risks for cardiometabolic diseases (4, 44), as well as brain ageing and dementia (45–47). 17 participants were excluded due to implausible menopause status data or age at menopause outliers (see Section 2.2 for details). 794 participants with known brain disorders were excluded based on ICD10 diagnoses including Alzheimer’s disease and dementia, mild cognitive disorder, neurodegenerative diseases, stroke, mental and behavioural disorders (36, 48). 9,882 participants were included in the final dataset. Sample demographics are provided in Table 1.
All females were classified into two groups based on their self-assessment to the question “Have you had your menopause (periods stopped)?”. Participants answering “no” at baseline were classed as premenopausal, and those answering “yes” at baseline were classed as postmenopausal. Premenopausal females who were older than 63 at baseline and postmenopausal females who were younger than 39 at baseline were excluded (n = 4), based on outlier estimations for the variable ‘age at menopause’ conducted on all UK Biobank females in our previous work (36). 13 participants were removed due to implausible menopause status data (e.g., responses indicating postmenopausal status at baseline and premenopausal status at timepoint 2). The final sample consisted of 3,529 premenopausal females and 6,353 postmenopausal females. Supplementary Figure S1 shows the baseline age distributions in the two groups. Due to the minimal age overlap between the groups in this sample, we were unable to use propensity matching [see e.g., (49)] to analyse sub-samples matched on age.
The primary measures of body anthropometrics included BMI (kg/m2) and WHR (waist circumference/hip circumference), which were obtained at both timepoints. The other cardiometabolic markers (collected only at baseline) consisted of BP (systolic and diastolic), HbA1c, triglycerides, and cholesterol (LDL and HDL; the latter of which is considered protective (50)). The BP measurements were taken using the Omron Digital BP monitor with the default automated option. All other markers (i.e., HDL, LDL, triglycerides, HbA1c) were obtained through blood assays. Cholesterol (HDL and LDL in mmol/L) were measured by enzyme immunoinhibition analysis on a Beckman Coulter AU5800, and triglycerides (mmol/L) were measured by GPO-POD analysis on the same device. The HbA1c assay was conducted using a Bio-Rad Variant II Turbo analyser, which utilises a High Performance Liquid Chromatography (HPLC) method to obtain a measurement (mmol/mol). Detailed descriptions of all assessment procedures can be found in the UK Biobank protocol (51). The means, standard deviations, and ranges for each marker are provided in Table 1. Detailed descriptions of the markers can be found in Supplementary Table S2, the distribution plots are provided in Supplementary Figure S2, and the correlations between markers are depicted in Supplementary Figure S3. As some variables did not show a normal distribution, the statistical analyses provided in Section 2.5 were re-run after log-transforming the variables. The results are provided in Supplementary section 6.4.
Information about the UK Biobank data acquisition protocols is available in Alfaro-Almagro et al. (52) and Miller et al. (53). Total volume of WMH was derived for each participant based on T2 fluid-attenuated inversion recovery (FLAIR) images and T1-weighted data (https://biobank.ndph.ox.ac.uk/showcase/field.cgi?id=25781) using the Brain Intensity Abnormality Classification Algorithm (BIANCA) (10), which is part of the FMRIB Software Library FSL (54). BIANCA is a fully automated tool for segmentation of WMH based on the k-nearest neighbour algorithm, and is documented as a reliable method for WMH segmentation in large cross-sectional cohort studies (10). The WMH volume measures were log-transformed to normalise and stabilise the variance (55, 56).
The statistical analyses were conducted using Python 3.8.17. All variables were standardised. Multiple comparisons using false discovery rate (FDR) correction (57, 58) were conducted across all p-values of the four main analyses, and separately across all p-values of the twelve supplementary analyses.
To test for group differences in cardiometabolic markers at baseline, a weighted least squares approach was used by assigning weights based on the number of participants in each group (59) to account for differences in menopause status group size. Menopause status group was used as the independent variable. Due to indications of multicollinearity (see Supplementary section 4 for correlations and variance inflation factors), the analyses were run separately for each of the cardiometabolic markers (dependent variables), whilst adjusting for age at baseline:
where represents the cardiometabolic marker (body anthropometrics, blood lipids, BP, or HbA1c; all measured at baseline), indicates the intercept, indicates the categorical group assignment based on menopause status, and is age measured at baseline.
To assess age-adjusted changes in BMI and WHR over time, and whether changes depended on menopause status group, we ran two separate linear mixed effects models with (BMI and WHR, respectively) as the dependent variable, timepoint and menopause status as categorical predictors, and timepoint * menopause status group as an interaction term.
In this equation, indicates the timepoint of measurement (baseline, timepoint 2), indicates the categorical group assignment based on menopause status, indicates the interaction term between time and menopause status, represents the age term, is the random intercept term for each participant , which allows for modeling individual-level variability in the baseline BMI and WHR that is not explained by the fixed effects, and is the residual error term.
To test whether baseline cardiometabolic markers were related to WMH outcomes (measured only at timepoint 2), we ran a series of linear regressions to test for main effects of each marker on WMH volume, respectively:
where represents the cardiometabolic marker (body anthropometrics, blood lipids, BP, or HbA1c; all measured at baseline), is age measured at timepoint 2, and is the time between baseline and timepoint 2 assessments in years. The models were run separately for each cardiometabolic marker (see section 2.6.1 for models run with all other markers as covariates).
To test whether changes in BMI and WHR were associated with WMH volume at timepoint 2, we first regressed the effect of baseline values on the timepoint 2 values to capture changes in BMI and WHR independent of starting point, and used the residuals as independent variables in a linear regression model.
Here, represents timepoint 2 BMI or WHR residualised for baseline values, is age measured at timepoint 2, and is the time between baseline and timepoint 2 assessments in years.
To account for potential confounding factors that could influence brain structure, hormone levels, or cardiometabolic health, models 3 and 4 were rerun with the following covariates, in addition to age: lifestyle factors including alcohol use (60–62), and smoking status (63, 64), socioeconomic factors including education level (65–67) and ethnic background (68), and female-specific factors including hormone replacement therapy use (user vs never user) (69, 70), oral contraceptive use (user vs never user) (71), and number of previous childbirths (48, 72). In addition, we included assessment location to adjust for potential effects on the measurements (73, 74). Missing values (“Not a Number (NaN),” “prefer not to answer,” “do not know”), were imputed for 71 participants using the SimpleImputer function from the scikit-learn Python library (75) (70 participants had one missing value, and one participant had three missing values). Additionally, model 3 was re-run while including all other markers as covariates in addition to age, to probe independent contributions of each baseline cardiometabolic marker. We also tested if the effects of BMI and WHR change on WMH volume (model 4) persisted when adjusting for baseline cardiometabolic markers, as well as change in systolic and diastolic BP in a subsample of participants who had BP data available at both baseline and timepoint 2 (n = 8,101).
To evaluate the independence of the group status effect from age-related influences, we report supplementary results from models 1 and 2 both with and without age as a covariate. In addition, the models were repeated including both age and age2 as covariates, to account for possible non-linear relationships between age and the dependent variables.
To assess if results were consistent when utilising stricter exclusion criteria, we repeated the analyses using a subsample excluding participants whose values for the cardiometabolic markers exceeded established healthy thresholds, as defined by the World Health Organisation (WHO) and the National Cholesterol Education Program Expert Panel (76–80). This included values for BMI above 30, WHR above 0.85, HDL below 1.03 mmol/L, LDL above 4.13 mmol/L, triglycerides above 2.26 mmol/L, Hba1c levels above 48 mmol/mol, in addition to hypertension, determined manually using systolic and diastolic BP measurements with a threshold of 140/90, and a confirmed medical diagnosis of diabetes (see also Supplementary Table S2). 6,019 participants were excluded (3,575 for body anthropometrics, 3,584 for blood lipids, 3,502 for hypertension, and 269 for diabetes and HbA1c, with 48.9% of excluded participants having values above the thresholds for two or more variables). The final subsample consisted of 3,863 participants (n premenopausal = 1,974, n postmenopausal = 1,889).
Given the mean age at baseline (46.58 ± 3.77) and the mean assessment interval (8.93 ± 1.63) in the premenopausal group, we separated females in this group who were transitioning to menopause between timepoints from those who remained premenopausal. We classed participants as “transitioning” if their self-reported menopause status changed between baseline and timepoint 2 (“Have you had your menopause (periods stopped)?”). Since we did not have data on whether participants were perimenopausal or not, we use the term “transitioning” to describe participants changing from a “premenopausal” status to a postmenopausal one between timepoints. To assess whether this fine-grained group assignment could influence the results, we repeated the analyses testing for group differences of longitudinal changes in body anthropometrics (model 2) and associations with WMHs (model 4) using three groups: premenopausal (n = 735), transitioning (n = 2,794), and postmenopausal (n = 6,353).
As shown in Table 2, postmenopausal females had significantly higher values for HDL, LDL, triglycerides, and HbA1c compared to premenopausal females, when adjusting for age. BMI, WHR, systolic BP, and diastolic BP did not show significant group differences.
Table 2. Results from the weighted regression models measuring group differences in baseline cardiometabolic factors by menopause status ( premenopausal 3,529, postmenopausal 6,353), with age included as a covariate in the models.
Figure 1 shows mean BMI and WHR plotted at both timepoints for each menopause status group. We found significant main effects of time for BMI and WHR, and significant interactions with menopause status group for BMI (Table 3). On average, age-adjusted BMI increased over time in the premenopausal group, while the postmenopausal group showed a decrease; however with small changes at group level. WHR increased on average in both groups over time. To interpret the WHR results in more detail, we ran exploratory analyses utilising waist circumference (WC) and hip circumference (HC) measures in each of the groups (Supplementary section 5). The results showed that premenopausal females had a steeper increase of WC compared to the postmenopausal group, while postmenopausal females had a steeper decrease of HC compared to the premenopausal group.
Figure 1. Body mass index (BMI) and waist-to-hip ratio (WHR) at baseline and timepoint 2, plotted separately for the premenopausal and postmenopausal groups. The shaded bands indicate 95% confidence intervals. Note that these plots illustrate the raw mean values and are not adjusted for age.
Table 3. Results from the mixed linear models for BMI (body mass index) and WHR (waist-to-hip ratio).
All baseline cardiometabolic markers except LDL were significantly associated with WMH volume at timepoint 2. Figure 2 displays the associations between baseline cardiometabolic markers and WMH volume. Full results are provided in Table 4.
Figure 2. Associations between baseline cardiometabolic markers and white matter hyperintensity (WMH) volume at timepoint 2, with age and assessment interval included in the models. The figure shows the standardised coefficients and their standard errors. Positive values indicate relationships between higher marker levels and greater WMH volume. BMI, body mass index; WHR, waist-to-hip ratio; HDL, high density lipoprotein; LDL, low density lipoprotein; BP, blood pressure; HbA1c, glycated haemoglobin.
Table 4. Results from the linear regression models testing associations between baseline cardiometabolic markers and WMH (white matter hyperintensity) volume at timepoint 2, with age and assessment interval included in the models.
As shown in Table 5, a greater increase of BMI and WHR between timepoints was significantly related to higher WMH volume at timepoint 2. Figure 3 displays the associations between BMI and WHR changes and WMH volume.
Table 5. Results from the linear regression models testing associations between BMI (body mass index) or WHR (waist-to-hip ratio) changes and WMH (white matter hyperintensity) volume at timepoint 2, with age and assessment interval included in the models.
Figure 3. Associations between age-adjusted body mass index (BMI) and waist-to-hip ratio (WHR) changes (from baseline to timepoint 2) and white matter hyperintensity (WMH) volume. BMI and WHR changes were measured using timepoint 2 values residualised for baseline values (change independent of starting point, see section 2.5.4). The shaded bands indicate 95% confidence intervals.
When including the additional covariates specified in (2.7), the results were highly consistent with our main results, as seen in Supplementary section 6.1 and 6.2 (Supplementary Tables S5 and S6, and S8–S13). When adjusting for all other cardiometabolic markers in each model, blood lipids and HbA1c no longer showed statistically significant associations with WMH volume (Supplementary Table S7). Similarly, when excluding participants whose values for the cardiometabolic markers exeeded established healthy thresholds, the associations of blood lipids and HbA1c with WMH volume were no longer statistically significant (Supplementary Table S16).
Supplementary section 6.5 shows the longitudinal changes of BMI and WHR in females who remained premenopausal at both timepoints, transitioned between timepoints, and were postmenopausal across timepoints. Premenopausal and transitioning females did not differ significantly in their BMI and WHR slopes over time (Supplementary Figure S6 and Table S19).
In summary, this population-based study of 9,882 UK Biobank females showed that poorer cardiometabolic health, as indicated by higher baseline levels of blood lipids, BP, and HbA1c, as well as baseline levels and longitudinal increases of BMI and WHR, was associated with larger WMH volume measured up to a decade later. The findings highlight the importance of maintaining cardiometabolic health in females across midlife, during the menopause, and into postmenopausal years, to optimise future cerebrovascular health outcomes.
Postmenopausal females exhibited significantly higher levels of baseline HDL, LDL, triglycerides, and HbA1c compared to premenopausal females, after adjusting for the effects of age. These results align with prior studies reporting higher levels of blood lipids and blood glucose in post- compared to premenopausal females (3–5, 7, 81–83), and indicate that hormonal changes related to menopause may exacerbate cardiometabolic risks beyond those of advancing age.
However, the postmenopausal group also demonstrated higher levels of HDL, which is typically considered protective (50, 84, 85), and the groups did not differ in baseline BP and body anthropometrics when adjusting for the effects of age. While these results are in contrast to some studies showing group differences in BP (86) and body anthropometrics (4, 87), a population-based study of 908 females found no difference in BP between pre- and postmenopausal females (88), and another study of 3,064 females found no association between change in menopausal status and changes in body anthropometrics (89). Recent reviews and meta-analyses highlight conflicting findings on the links between menopause-related processes and BP (7, 90), body anthropometrics (6), and HDL levels (5, 91), noting that observed differences in some cardiometabolic risk factors may be largely driven by increasing age rather than menopause-specific processes (6, 30), and that discrepancies in methodology and design may also contribute to conflicting findings.
A key challenge in menopause research is to disentangle the effects of chronological vs endocrine ageing given their concurrent progression in females. Although methods such as age-based propensity matching can be useful [see e.g., (49)], they rely on sufficient overlap in age distributions between groups, which was minimal in our sample (Supplementary Figure S1). Given the complex interplay between endocrine and cardiometabolic processes (92, 93), longitudinal menopause research (e.g., (94)) is crucial to clarify how these processes interact in females over time. Moreover, future studies could aim for classifications of menopausal status based on hormonal or symptom profiles (95, 96), which may provide more accurate results than self-assessment (97).
The premenopausal group demonstrated an average increase in both BMI and WHR between baseline and the imaging timepoint. In this group, the observed change in WHR was driven by an increase in waist circumference (Supplementary Figure S4). Since 79.2% of the females in the premenopausal group underwent menopause between timepoints (see section 2.6.4), this finding aligns with previous literature highlighting a redistribution of adipose tissue towards the waist during the menopause transition (2–4, 6). For example, a longitudinal study in 1,246 females from the Study of Women’s Health Across the Nation (SWAN) showed that the trajectory of fat mass doubled across menopausal years before decreasing two years post-menopause (98). In our study, however, changes in body anthropometrics did not differ between transitioning and premenopausal females (Supplementary section 6.5). Given the lower age limit of our sample (40 years), this could indicate overlapping changes in adipose tissue distribution between transitioning and pre- or perimenopausal females in this cohort.
The years prior to menopause are characterised by changing levels of reproductive hormones such as oestradiol (99–102), with perimenopause often highlighted as a cardiometabolic risk phase (3, 7, 92, 103). Although oestradiol assessments could potentially have clarified the changes observed in premenopausal and transitioning females, these measures were only available for a smaller subset of our sample from the baseline assessment. As previous studies highlight fluctuations and high variability of such reproductive hormones across early and late perimenopause (99, 104, 105), relying on a single baseline measure would prevent definitive conclusions. Given that the years surrounding menopause are characterised by distinct endocrine, menstrual, and ovarian markers that could influence cardiometabolic risk and brain health, future research should aim to include detailed data on hormone levels, menstrual cycle length and regularity, and occurrence of symptoms, in line with criteria established at the Stages of Reproductive Aging Workshop (STRAW) (106).
Importantly, our results might also reflect influences of factors beyond menopause, such as genetic predisposition for cardiometabolic disease (107–109) or lifestyle behaviours in early adulthood and midlife (110, 111). For future studies, it will be crucial to distinguish oestradiol-related effects from other contributing factors to enhance our understanding of cardiometabolic health trajectories in females across menopausal years.
In the postmenopausal group, we found an average decline of BMI, in addition to an increase in WHR that was driven by decreasing hip circumference (Supplementary Figure S4). Lower BMI and sarcopenia (muscle loss) are commonly observed in ageing (112–115), but have also been linked to lower oestradiol levels during menopause (30, 116, 117). Although the group-level changes in body anthropometrics were in general small, these findings could be indicative of processes such as sarcopenia among the postmenopausal participants. Overall, our longitudinal results point to time-sensitive impacts on body anthropometrics in females, and highlight the complexity of the relationships between menopause, ageing, and changes in cardiometabolic factors.
Our results showed associations between markers of poor cardiometabolic health at baseline and greater WMH volume measured almost a decade later. This is in line with previous literature linking midlife cardiometabolic health to WMHs, highlighting the long-term implications of cardiometabolic risk factors on brain health (8, 31–41, 56, 118).
Higher systolic and diastolic BP showed prominent associations with larger WMH volume, and the associations persisted when adjusting for other cardiometabolic markers as well as excluding participants whose marker levels exceeded healthy thresholds. This finding corresponds to studies reporting stronger relationships between BP and WMHs compared to body anthropometrics, blood lipids, or HbA1c (119–121), particularly in females (9), and highlights the importance of monitoring and controlling midlife BP levels to protect against cerebrovascular decline (56, 122).
In addition to BP, greater baseline BMI and WHR, as well as increasing levels between timepoints, showed robust associations with larger WMH volume. Previous studies in females have shown associations between increasing BMI and cortical thinning (123, 124), as well as reductions total grey matter volume (125) and hippocampal volume (126). To our knowledge, the current study is the first to support a link between longitudinal increases in body anthropometric measures and WMH volume in mid- to older-aged females. While a global measure of total WMH volume was used in the current study, future research could aim to investigate periventricular and deep WMHs (127–129) to elucidate their distinct associations with changes in cardiometabolic risk factors [see e.g., (34, 130)], as well as menopause-related processes and vasomotor symptoms (20, 131).
Furthermore, our study measured changes in BMI and WHR independent of starting point, and prospective studies might benefit from characterising differences in trajectories based on initial level (126, 132). While this approach could help to identify individuals at high risk for deteriorating cardiometabolic and brain health, it is important to note that research indicates influences of genetics (133) and early life factors (134) on individual variation in WMHs, as well as age-sensitive variations in body-brain relationships across the lifespan (42, 43). Hence, multifactorial studies with longitudinal designs are needed to map the factors linked to cardiometabolic and microvascular risk across adulthood, the menopause transition, and into older age.
Finally, the demographic context of our study should be taken into account. The UK Biobank cohort is highly homogeneous in terms of “WEIRD” criteria (from Western, educated, industrialised, rich, and democratic societies (135)), and additionally characterized by a “healthy volunteer effect” (136). Consequently, our results offer valuable insights but may not necessarily generalize to other samples. Yet, our study contributes to a critical area in public health, given the rising prevalence of chronic, non-communicable diseases such as cardiometabolic disease and neurodegenerative conditions (137, 138). Despite known sex differences in the aetiology, prevalence, and outcomes of these diseases (139–142), there remains a severe lack of research on female-specific factors and risks (143–145). While our study highlights distinct cardiometabolic patterns in pre- and postmenopausal females and relationships of these with cerebrovascular outcomes, it also underscores the critical need for detailed, longitudinal studies encompassing both midlife and older age in females. Future research should assess the interplay between cardiometabolic health, female endocrine processes, and brain outcomes to lay the groundwork for effective health interventions.
This population-based study demonstrates that markers of cardiometabolic health in middle- and older aged females are linked to future cerebrovascular health outcomes. The results highlight the importance of maintaining cardiometabolic health in females across menopausal years, and emphasises the critical need for longitudinal studies addressing cardiometabolic risk and brain health in females throughout adulthood, menopausal years, and into older age.
Publicly available datasets were analyzed in this study. This data can be found here: https://www.ukbiobank.ac.uk/.
The studies involving humans were approved by National Health Service National Research Ethics Service (ref 11/NW/0382). The studies were conducted in accordance with the local legislation and institutional requirements. The participants provided their written informed consent to participate in this study.
LSS: Writing – original draft, Writing – review & editing, Conceptualization, Data curation, Formal analysis, Investigation, Methodology, Software, Validation, Visualization; SS: Writing – review & editing, Writing – original draft; AA: Writing – review & editing, Methodology; CB: Writing – review & editing, Methodology; AC: Writing – review & editing; IV: Writing – review & editing; DB: Writing – review & editing; TPG: Writing – review & editing; AT: Writing – review & editing; SS: Writing – review & editing; KPE: Methodology, Project administration, Writing – review & editing; OAA: Methodology, Project administration, Resources, Writing – review & editing; BD: Resources, Supervision, Writing – review & editing; LTW: Methodology, Project administration, Resources, Supervision, Writing – review & editing; AMGL: Conceptualization, Data curation, Formal analysis, Funding acquisition, Investigation, Methodology, Project administration, Resources, Software, Supervision, Validation, Visualization, Writing – original draft, Writing – review & editing.
The author(s) declare financial support was received for the research, authorship, and/or publication of this article.
The authors received funding from the Swiss National Science Foundation (PZ00P3_193658; TMPFP3_217174; NCCR Synapsy, project grants Nr 32003B_135679, 32003B_159780, 324730_192755, and CRSK-3_190185), the Leenaards Foundation, the Natural Sciences and Engineering Research Council of Canada, the Research Council of Norway (323961, 273345, 249795, 298646, 300768, 223273, 283799, 283798), the South-Eastern Norway Regional Health Authority (2018076, 2019101, 2017112, 2022080, 2022103, 2023037), the European Research Council under the European Union's Horizon 2020 research and innovation programme (802998, 732592, 847776), the HDH Wills 1965 Charitable Trust (1117747), the Alzheimer's Society (Grant Ref 441), and the Academy of Medical Sciences/the Wellcome Trust/the Government Department of Business, Energy and Industrial Strategy/the British Heart Foundation/Diabetes UK Springboard Award (SBF006\1078). The Wellcome Centre for Integrative Neuroimaging is supported by core funding from the Wellcome Trust (203139/Z/16/Z).
This research has been conducted using the UK Biobank data under Application 27412. UKB has received ethics approval from the National Health Service National Research Ethics Service (ref 11/NW/0382). The work was performed on the Service for Sensitive Data (TSD) platform, owned by the University of Oslo, operated and developed by the TSD service group at the University of Oslo IT-Department (USIT). Computations were also performed using resources provided by UNINETT Sigma2—the National Infrastructure for High Performance Computing and Data Storage in Norway.
The authors declare that the research was conducted in the absence of any commercial or financial relationships that could be construed as a potential conflict of interest.
The reviewer FB declared a shared affiliation with the author BD to the handling editor at the time of review.
The author(s) declared that they were an editorial board member of Frontiers, at the time of submission. This had no impact on the peer review process and the final decision.
All claims expressed in this article are solely those of the authors and do not necessarily represent those of their affiliated organizations, or those of the publisher, the editors and the reviewers. Any product that may be evaluated in this article, or claim that may be made by its manufacturer, is not guaranteed or endorsed by the publisher.
The Supplementary Material for this article can be found online at: https://www.frontiersin.org/articles/10.3389/fgwh.2023.1320640/full#supplementary-material. Scripts for running the main and supplementary analyses are available at https://osf.io/4ub83/
1. Carr MC. The emergence of the metabolic syndrome with menopause. J Clin Endocrinol Metab. (2003) 88:2404–11. doi: 10.1210/jc.2003-030242
2. Leeners B, Geary N, Tobler PN, Asarian L. Ovarian hormones, obesity. Hum Reprod Update. (2017) 23:300–21. doi: 10.1093/humupd/dmw045
3. Marlatt KL, Pitynski-Miller DR, Gavin KM, Moreau KL, Melanson EL, Santoro N, et al. Body composition, cardiometabolic health across the menopause transition. Obesity. (2022) 30:14–27. doi: 10.1002/oby.23289
4. Pu D, Tan R, Yu Q, Wu J. Metabolic syndrome in menopause and associated factors: a meta-analysis. Climacteric. (2017) 20:583–91. doi: 10.1080/13697137.2017.1386649
5. Ambikairajah A, Walsh E, Cherbuin N. Lipid profile differences during menopause: a review with meta-analysis. Menopause. (2019) 26:1327–33. doi: 10.1097/GME.0000000000001403
6. Ambikairajah A, Walsh E, Tabatabaei-Jafari H, Cherbuin N. Fat mass changes during menopause: a metaanalysis. Am J Obstet Gynecol. (2019) 221:393–409. doi: 10.1016/j.ajog.2019.04.023
7. El Khoudary SR, Greendale G, Crawford SL, Avis NE, Brooks MM, Thurston RC, et al. The menopause transition and women’s health at midlife: a progress report from the study of women’s health across the nation (swan). Menopause. (2019) 26:1213. doi: 10.1097/GME.0000000000001424
8. García-García I, Michaud A, Dagher MÁ, Jurado A, Morys F. Mechanisms linking obesity and its metabolic comorbidities with cerebral grey and white matter changes. Rev Endocr Metab Disord. (2022) 23:833–43. doi: 10.1007/s11154-021-09706-5
9. Scharf EL, Graff-Radford J, Przybelski SA, Lesnick TG, Mielke MM, Knopman DS, et al., Cardiometabolic health, longitudinal progression of white matter hyperintensity: the mayo clinic study of aging. Stroke. (2019) 50:3037–44. doi: 10.1161/STROKEAHA.119.025822
10. Griffanti L, Zamboni G, Khan A, Li L, Bonifacio G, Sundaresan V, et al., BIANCA (brain intensity abnormality classification algorithm): a new tool for automated segmentation of white matter hyperintensities. Neuroimage. (2016) 141:191–205. doi: 10.1016/j.neuroimage.2016.07.018
11. Wardlaw JM, Valdés Hernández MC, Muñoz-Maniega S. What are white matter hyperintensities made of? Relevance to vascular cognitive impairment. J Am Heart Assoc. (2015) 4:e001140. doi: 10.1161/JAHA.114.001140
12. Garnier-Crussard A, Bougacha S, Wirth M, André C, Delarue M, Landeau B, et al., White matter hyperintensities across the adult lifespan: relation to age, A load, cognition. Alzheimer’s Res Ther. (2020) 12:1–11. doi: 10.1186/s13195-020-00669-4
13. Debette S, Markus H. The clinical importance of white matter hyperintensities on brain magnetic resonance imaging: systematic review and meta-analysis. BMJ. (2010) 341:c3666. doi: 10.1136/bmj.c3666
14. Jansen MG, Griffanti L, Mackay CE, Anatürk M, Melazzini L, de Lange A-MG, et al., Association of cerebral small vessel disease burden with brain structure, cognitive, vascular risk trajectories in mid-to-late life. J Cereb Blood Flow Metab. (2022) 42:600–12. doi: 10.1177/0271678X211048411
15. Lee S, Viqar F, Zimmerman ME, Narkhede A, Tosto G, Benzinger TL, et al., White matter hyperintensities are a core feature of Alzheimer’s disease: evidence from the dominantly inherited Alzheimer network. Ann Neurol. (2016) 79:929–39. doi: 10.1002/ana.24647
16. Prins ND, Scheltens P. White matter hyperintensities, cognitive impairment and dementia: an update. Nat Rev Neurol. (2015) 11:157–65. doi: 10.1038/nrneurol.2015.10
17. Ferretti MT, Santuccione Chadha A. The missing X factor in alzheimer disease. Nat Rev Neurol. (2021) 17:727–8. doi: 10.1038/s41582-021-00573-x
18. Buckley RF, Gong J, Woodward M. A call to action to address sex differences in alzheimer disease clinical trials. JAMA Neurol. (2023) 80(8):769–70. doi: 10.1001/jamaneurol.2023.1059
19. Alqarni A, Jiang J, Crawford JD, Koch F, Brodaty H, Sachdev P, et al. Sex differences in risk factors for white matter hyperintensities in non-demented older individuals. Neurobiol Aging. (2021) 98:197–204. doi: 10.1016/j.neurobiolaging.2020.11.001
20. Alqarni A, Wen W, Lam BC, Crawford JD, Sachdev PS, Jiang J. Hormonal factors moderate the associations between vascular risk factors, white matter hyperintensities. Brain Imaging Behav. (2023) 17:172–84. doi: 10.1007/s11682-022-00751-5
21. Fatemi F, Kantarci K, Graff-Radford J, Preboske GM, Weigand SD, Przybelski SA, et al., Sex differences in cerebrovascular pathologies on flair in cognitively unimpaired elderly. Neurology. (2018) 90:e466–73. doi: 10.1212/WNL.0000000000004913
22. Lohner V, Pehlivan G, Sanroma G, Miloschewski A, Schirmer MD, Stöcker T, et al. Relation between sex, menopause, and white matter hyperintensities: the Rhineland Study. Neurology. (2022) 99:e935–43. doi: 10.1212/WNL.0000000000200782
23. Than S, Moran C, Beare R, Vincent AJ, Collyer TA, Wang W, et al., Interactions between age, sex, menopause, and brain structure at midlife: a UK Biobank study. J Clin Endocrinol Metab. (2021) 106:410–20. doi: 10.1210/clinem/dgaa847
24. Than S, Moran C, Collyer TA, Beare RJ, Lane EM, Vincent AJ, et al., Associations of sex, age, and cardiometabolic risk profiles with brain structure and cognition: a UK Biobank latent class analysis. Neurology. (2022) 99:e1853–65. doi: 10.1212/WNL.0000000000201028
25. Sachdev PS, Parslow R, Wen W, Anstey K, Easteal S. Sex differences in the causes and consequences of white matter hyperintensities. Neurobiol Aging. (2009) 30:946–56. doi: 10.1016/j.neurobiolaging.2007.08.023
26. Abdulnour J, Doucet E, Brochu M, Lavoie J-M, Strychar I, Rabasa-Lhoret R, et al. The effect of the menopausal transition on body composition and cardiometabolic risk factors: a Montreal-Ottawa new emerging team group study. Menopause. (2012) 19:760–7. doi: 10.1097/gme.0b013e318240f6f3
27. Ho S, Wu S, Chan S, Sham A. Menopausal transition and changes of body composition: a prospective study in Chinese perimenopausal women. Int J Obes. (2010) 34:1265–74. doi: 10.1038/ijo.2010.33
28. Lovejoy J, Champagne C, De Jonge L, Xie H, Smith S. Increased visceral fat and decreased energy expenditure during the menopausal transition. Int J Obes. (2008) 32:949–58. doi: 10.1038/ijo.2008.25
29. Janssen I, Powell LH, Crawford S, Lasley B, Sutton-Tyrrell K. Menopause and the metabolic syndrome: the study of women’s health across the nation. Arch Intern Med. (2008) 168:1568–75. doi: 10.1001/archinte.168.14.1568
30. Matthews KA, Crawford SL, Chae CU, Everson-Rose SA, Sowers MF, Sternfeld B, et al. Are changes in cardiovascular disease risk factors in midlife women due to chronological aging or to the menopausal transition? J Am Coll Cardiol. (2009) 54:2366–73. doi: 10.1016/j.jacc.2009.10.009
31. Arnoldussen IA, Gustafson DR, Leijsen EM, de Leeuw F-E, Kiliaan AJ. Adiposity is related to cerebrovascular and brain volumetry outcomes in the run DMC study. Neurology. (2019) 93:e864–78. doi: 10.1212/WNL.0000000000008002
32. Han Y-P, Tang X, Han M, Yang J, Cardoso MA, Zhou J, et al. Relationship between obesity and structural brain abnormality: accumulated evidence from observational studies. Ageing Res Rev. (2021) 71:101445. doi: 10.1016/j.arr.2021.101445
33. Kim KW, Seo H, Kwak M-S, Kim D. Visceral obesity is associated with white matter hyperintensity and lacunar infarct. Int J Obes (Lond). (2017) 41:683–8. doi: 10.1038/ijo.2017.13
34. Lampe L, Zhang R, Beyer F, Huhn S, Kharabian Masouleh S, Preusser S, et al. Visceral obesity relates to deep white matter hyperintensities via inflammation. Ann Neurol. (2019) 85:194–203. doi: 10.1002/ana.25396
35. Pasha EP, Birdsill A, Parker P, Elmenshawy A, Tanaka H, Haley AP. Visceral adiposity predicts subclinical white matter hyperintensities in middle-aged adults. Obes Res Clin Pract. (2017) 11:177–87. doi: 10.1016/j.orcp.2016.04.003
36. Schindler LS, Subramaniapillai S, Barth C, van der Meer D, Pedersen ML, Kaufmann T, et al., Associations between abdominal adipose tissue, reproductive span, and brain characteristics in post-menopausal women. NeuroImage Clin. (2022) 36:103239. doi: 10.1016/j.nicl.2022.103239
37. Widya RL, Kroft LJ, Altmann-Schneider I, van der Bijl N, de Roos A, Lamb HJ, et al., Visceral adipose tissue is associated with microstructural brain tissue damage. Obesity. (2015) 23:1092–6. doi: 10.1002/oby.21048
38. Cox SR, Lyall DM, Ritchie SJ, Bastin ME, Harris MA, Buchanan CR, et al., Associations between vascular risk factors and brain MRI indices in UK Biobank. Eur Heart J. (2019) 40:2290–300. doi: 10.1093/eurheartj/ehz100
39. Morys F, Dadar M, Dagher A. Association between midlife obesity and its metabolic consequences, cerebrovascular disease, and cognitive decline. J Clin Endocrinol Metab. (2021) 106:e4260–74. doi: 10.1210/clinem/dgab135
40. Tamura Y, Araki A. Diabetes mellitus and white matter hyperintensity. Geriatr Gerontol Int. (2015) 15:34–42. doi: 10.1111/ggi.12666
41. Jorgensen DR, Shaaban CE, Wiley CA, Gianaros PJ, Mettenburg J, Rosano C. A population neuroscience approach to the study of cerebral small vessel disease in midlife and late life: an invited review. Am J Physiol Heart Circ Physiol. (2018) 314:H1117–36. doi: 10.1152/ajpheart.00535.2017
42. Kivimäki M, Luukkonen R, Batty GD, Ferrie JE, Pentti J, Nyberg ST, et al., Body mass index and risk of dementia: analysis of individual-level data from 1.3 million individuals. Alzheimer Dement. (2018) 14:601–9. doi: 10.1016/j.jalz.2017.09.016
43. Subramaniapillai S, Suri S, Barth C, Maximov II, Voldsbekk I, van der Meer D, et al., Sex-and age-specific associations between cardiometabolic risk and white matter brain age in the UK Biobank cohort. Hum Brain Mapp. (2022) 43:3759–74. doi: 10.1002/hbm.25882
44. Karia PS, Joshu CE, Visvanathan K. Association of oophorectomy and fat and lean body mass: evidence from a population-based sample of US women. Cancer Epidemiol Biomarkers Prev. (2021) 30:1424–32. doi: 10.1158/1055-9965.EPI-20-1849
45. Brown A, Gravelsins L, Almey A, Gervais NJ, Duchesne A, Rieck J, et al., Early structural brain markers for Alzheimer’s disease in women: brain-behaviour relationships after ovarian removal. Alzheimer Dement. (2023) 19:e063700. doi: 10.1007/s12035-023-03424-6
46. Gravelsins L, Brown A, Almey A, Gervais NJ, Duchesne A, Rieck J, et al., Hypogyrification in midlife women with surgical menopause, a population at risk for late life Alzheimer’s. Alzheimer Dement. (2023) 19:e068247. doi: 10.1002/alz.068247
47. Zeydan B, Tosakulwong N, Schwarz CG, Senjem ML, Gunter JL, Reid RI, et al., Association of bilateral salpingo-oophorectomy before menopause onset with medial temporal lobe neurodegeneration. JAMA Neurol. (2019) 76:95–100. doi: 10.1001/jamaneurol.2018.3057
48. Voldsbekk I, Barth C, Maximov II, Kaufmann T, Beck D, Richard G, et al. A history of previous childbirths is linked to women’s white matter brain age in midlife and older age. Hum Brain Mapp. (2021) 42:4372–86. doi: 10.1002/hbm.25553
49. Ambikairajah A, Tabatabaei-Jafari H, Hornberger M, Cherbuin N. Age, menstruation history, and the brain. Menopause. (2021) 28:167–74. doi: 10.1097/GME.0000000000001688
50. Nicholls SJ, Nelson AJ. HDL and cardiovascular disease. Pathology. (2019) 51:142–7. doi: 10.1016/j.pathol.2018.10.017
51. Elliott P, Peakman TC. The UK Biobank sample handling and storage protocol for the collection, processing and archiving of human blood and urine. Int J Epidemiol. (2008) 37:234–44. doi: 10.1093/ije/dym276
52. Alfaro-Almagro F, Jenkinson M, Bangerter NK, Andersson JL, Griffanti L, Douaud G, et al., Image processing and quality control for the first 10,000 brain imaging datasets from UK Biobank. Neuroimage. (2018) 166:400–24. doi: 10.1016/j.neuroimage.2017.10.034
53. Miller KL, Alfaro-Almagro F, Bangerter NK, Thomas DL, Yacoub E, Xu J, et al., Multimodal population brain imaging in the UK Biobank prospective epidemiological study. Nat Neurosci. (2016) 19:1523–36. doi: 10.1038/nn.4393
54. Jenkinson M, Beckmann CF, Behrens TEJ, Woolrich MW, Smith SM. FSL. NeuroImage. (2012) 62:782–90. doi: 10.1016/j.neuroimage.2011.09.015
55. Veldsman M, Kindalova P, Husain M, Kosmidis I, Nichols TE. Spatial distribution and cognitive impact of cerebrovascular risk-related white matter hyperintensities. NeuroImage Clin. (2020) 28:102405. doi: 10.1016/j.nicl.2020.102405
56. Wartolowska KA, Webb AJS. Midlife blood pressure is associated with the severity of white matter hyperintensities: analysis of the UK Biobank cohort study. Eur Heart J. (2021) 42:750–7. doi: 10.1093/eurheartj/ehaa756
57. Benjamini Y, Hochberg Y. Controlling the false discovery rate: a practical and powerful approach to multiple testing. J R Stat Soc B (Methodol). (1995) 57:289–300. doi: 10.1111/j.2517-6161.1995.tb02031.x
58. Korthauer K, Kimes PK, Duvallet C, Reyes A, Subramanian A, Teng M, et al. A practical guide to methods controlling false discoveries in computational biology. Genome Biol. (2019) 20:1–21. doi: 10.1186/s13059-019-1716-1
59. Cho BR, Park C. Robust design modeling and optimization with unbalanced data. Comput Ind Eng. (2005) 48:173–80. doi: 10.1016/j.cie.2005.01.004
60. Lankester J, Zanetti D, Ingelsson E, Assimes TL. Alcohol use and cardiometabolic risk in the UK Biobank: a mendelian randomization study. PLoS ONE. (2021) 16:e0255801. doi: 10.1371/journal.pone.0255801
61. Topiwala A, Ebmeier KP, Maullin-Sapey T, Nichols TE. Alcohol consumption and MRI markers of brain structure and function: cohort study of 25,378 UK Biobank participants. NeuroImage Clin. (2022) 35:103066. doi: 10.1016/j.nicl.2022.103066
62. Topiwala A, Wang C, Ebmeier KP, Burgess S, Bell S, Levey DF, et al., Associations between moderate alcohol consumption, brain iron, and cognition in UK Biobank participants: Observational and mendelian randomization analyses. PLoS Med. (2022) 19:e1004039. doi: 10.1371/journal.pmed.1004039
63. Erhardt L. Cigarette smoking: an undertreated risk factor for cardiovascular disease. Atherosclerosis. (2009) 205:23–32. doi: 10.1016/j.atherosclerosis.2009.01.007
64. Power MC, Deal JA, Sharrett AR, Jack CR, Knopman D, Mosley TH, et al. Smoking and white matter hyperintensity progression: the ARIC-MRI study. Neurology. (2015) 84:841–8. doi: 10.1212/WNL.0000000000001283
65. Meng X, D’arcy C. Education and dementia in the context of the cognitive reserve hypothesis: a systematic review with meta-analyses and qualitative analyses. PLoS ONE. (2012) 7:e38268. doi: 10.1371/journal.pone.0038268
66. Walhovd KB, Fjell AM, Wang Y, Amlien IK, Mowinckel AM, Lindenberger U, et al., Education and income show heterogeneous relationships to lifespan brain and cognitive differences across European and US cohorts. Cereb Cortex. (2022) 32:839–54. doi: 10.1093/cercor/bhab248
67. Fotenos AF, Mintun MA, Snyder AZ, Morris JC, Buckner RL. Brain volume decline in aging: evidence for a relation between socioeconomic status, preclinical alzheimer disease, and reserve. Arch Neurol. (2008) 65:113–20. doi: 10.1001/archneurol.2007.27
68. Goff LM. Ethnicity and type 2 diabetes in the UK. Diabet Med. (2019) 36:927–38. doi: 10.1111/dme.13895
69. Hogervorst E, Williams J, Budge M, Riedel W, Jolles J. The nature of the effect of female gonadal hormone replacement therapy on cognitive function in post-menopausal women: a meta-analysis. Neuroscience. (2000) 101:485–512. doi: 10.1016/S0306-4522(00)00410-3
70. Maki PM, Dennerstein L, Clark M, Guthrie J, LaMontagne P, Fornelli D, et al. Perimenopausal use of hormone therapy is associated with enhanced memory and hippocampal function later in life. Brain Res. (2011) 1379:232–43. doi: 10.1016/j.brainres.2010.11.030
71. De Bondt T, Jacquemyn Y, Van Hecke W, Sijbers J, Sunaert S, Parizel P. Regional gray matter volume differences and sex-hormone correlations as a function of menstrual cycle phase and hormonal contraceptives use. Brain Res. (2013) 1530:22–31. doi: 10.1016/j.brainres.2013.07.034
72. de Lange A-MG, Kaufmann T, van der Meer D, Maglanoc LA, Alnæs D, Moberget T, et al. Population-based neuroimaging reveals traces of childbirth in the maternal brain. Proc Natl Acad Sci. (2019) 116:22341–6. doi: 10.1073/pnas.1910666116
73. Bayer JM, Thompson PM, Ching CR, Liu M, Chen A, Panzenhagen AC, et al. Site effects how-to and when: an overview of retrospective techniques to accommodate site effects in multi-site neuroimaging analyses. Front Neurol. (2022) 13:923988. doi: 10.3389/fneur.2022.923988
74. Lee H, Nakamura K, Narayanan S, Brown RA, Arnold DL; Alzheimer’s Disease Neuroimaging Initiative. Estimating and accounting for the effect of MRI scanner changes on longitudinal whole-brain volume change measurements. Neuroimage. (2019) 184:555–65. doi: 10.1016/j.neuroimage.2018.09.062
75. Pedregosa F, Varoquaux G, Gramfort A, Michel V, Thirion B, Grisel O, et al. Scikit-learn: machine learning in Python. J Mach Learn Res. (2011) 12:2825–30.
76. Expert Panel on Detection, Evaluation, and Treatment of High Blood Cholesterol in Adults. Executive summary of the third report of the national cholesterol education program (NCEP) expert panel on detection, evaluation, and treatment of high blood cholesterol in adults (adult treatment panel III). JAMA. (2001) 285:2486–97. doi: 10.1001/jama.285.19.2486
77. World Health Organization. Use of glycated haemoglobin (HbA1c) in diagnosis of diabetes mellitus: abbreviated report of a WHO consultation. Technical Report World Health Organization (2011).
78. World Health Organization. Waist circumference and waist-hip ratio: report of a WHO expert consultation, 2008 Dec 8–11, Geneva. (2011).
79. World Health Organization. Guideline for the pharmacological treatment of hypertension in adults. Geneva, Switzerland: World Health Organization (2021).
81. Dehghan A, Vasan SK, Fielding BA, Karpe F. A prospective study of the relationships between change in body composition and cardiovascular risk factors across the menopause. Menopause. (2021) 28:400. doi: 10.1097/GME.0000000000001721
82. De Kat A, Dam V, Onland-Moret N, Eijkemans M, Broekmans F, Van Der Schouw Y. Unraveling the associations of age and menopause with cardiovascular risk factors in a large population-based study. BMC Med. (2017) 15:1–11. doi: 10.1186/s12916-016-0762-8
83. Song DK, Hong YS, Sung Y-A, Lee H. The effect of menopause on cardiovascular risk factors according to body mass index in middle-aged Korean women. PLoS ONE. (2023) 18:e0283393. doi: 10.1371/journal.pone.0283393
84. Assmann G, Gotto AM Jr. HDL cholesterol and protective factors in atherosclerosis. Circulation. (2004) 109:III8. doi: 10.1161/01.CIR.0000131512.50667.46
85. Francis GA. The complexity of HDL. Biochim Biophys Acta. (2010) 1801:1286–93. doi: 10.1016/j.bbalip.2010.08.009
86. Samargandy S, Matthews KA, Brooks MM, Barinas-Mitchell E, Magnani JW, Thurston RC, et al. Trajectories of blood pressure in midlife women: does menopause matter? Circ Res. (2022) 130:312–22. doi: 10.1161/CIRCRESAHA.121.319424
87. Gould LM, Gordon AN, Cabre HE, Hoyle AT, Ryan ED, Hackney AC, et al. Metabolic effects of menopause: a cross-sectional characterization of body composition and exercise metabolism. Menopause. (2022) 29:377–89. doi: 10.1097/GME.0000000000001932
88. Cifkova R, Pitha J, Lejskova M, Lanska V, Zecova S. Blood pressure around the menopause: a population study. J Hypertens. (2008) 26:1976–82. doi: 10.1097/HJH.0b013e32830b895c
89. Sternfeld B, Wang H, Quesenberry CP Jr, Abrams B, Everson-Rose SA, Greendale GA, et al. Physical activity and changes in weight and waist circumference in midlife women: findings from the study of women’s health across the nation. Am J Epidemiol. (2004) 160:912–22. doi: 10.1093/aje/kwh299
90. Tikhonoff V, Casiglia E, Gasparotti F, Spinella P. The uncertain effect of menopause on blood pressure. J Hum Hypertens. (2019) 33:421–8. doi: 10.1038/s41371-019-0194-y
91. El Khoudary SR. HDL and the menopause. Curr Opin Lipidol. (2017) 28:328–36. doi: 10.1097/MOL.0000000000000432
92. Nappi RE, Chedraui P, Lambrinoudaki I, Simoncini T. Menopause: a cardiometabolic transition. Lancet Diabetes Endocrinol. (2022) 10(6):442–56. doi: 10.1016/S2213-8587(22)00076-6
93. Roa-Díaz ZM, Raguindin PF, Bano A, Laine JE, Muka T, Glisic M. Menopause and cardiometabolic diseases: what we (don’t) know and why it matters. Maturitas. (2021) 152:48–56. doi: 10.1016/j.maturitas.2021.06.013
94. Mosconi L, Rahman A, Diaz I, Wu X, Scheyer O, Hristov HW, et al. Increased Alzheimer’s risk during the menopause transition: a 3-year longitudinal brain imaging study. PLoS ONE. (2018) 13:e0207885. doi: 10.1371/journal.pone.0207885
95. Bastian LA, Smith CM, Nanda K. Is this woman perimenopausal? JAMA. (2003) 289:895–902. doi: 10.1001/jama.289.7.895
96. Soules MR, Sherman S, Parrott E, Rebar R, Santoro N, Utian W, et al. Executive summary: stages of reproductive aging workshop (STRAW). Climacteric. (2001) 4:267–72. doi: 10.1080/cmt.4.4.267.272
97. Schoeler T, Pingault J-B, Kutalik Z. Self-report inaccuracy in the UK Biobank: impact on inference and interplay with selective participation. medRxiv (2023). p. 2023.10.
98. Greendale GA, Sternfeld B, Huang M, Han W, Karvonen-Gutierrez C, Ruppert K, et al. Changes in body composition and weight during the menopause transition. JCI Insight. (2019) 4. doi: 10.1172/jci.insight.124865
99. Brinton RD, Yao J, Yin F, Mack WJ, Cadenas E. Perimenopause as a neurological transition state. Nat Rev Endocrinol. (2015) 11:393–405. doi: 10.1038/nrendo.2015.82
100. Burger H, Woods NF, Dennerstein L, Alexander JL, Kotz K, Richardson G. Nomenclature and endocrinology of menopause and perimenopause. Expert Rev Neurother. (2007) 7:S35–S43. doi: 10.1586/14737175.7.11s.S35
101. Butler L, Santoro N. The reproductive endocrinology of the menopausal transition. Steroids. (2011) 76:627–35. doi: 10.1016/j.steroids.2011.02.026
102. Hall JE. Endocrinology of the menopause. Endocrinol Metab Clin. (2015) 44:485–96. doi: 10.1016/j.ecl.2015.05.010
103. Jull J, Stacey D, Beach S, Dumas A, Strychar I, Ufholz L-A, et al., Lifestyle interventions targeting body weight changes during the menopause transition: a systematic review. J Obes. (2014) 2014. doi: 10.1155/2014/824310
104. Randolph JF Jr, Zheng H, Sowers MR, Crandall C, Crawford S, Gold EB, et al. Change in follicle-stimulating hormone and estradiol across the menopausal transition: effect of age at the final menstrual period. J Clin Endocrinol Metab. (2011) 96:746–54. doi: 10.1210/jc.2010-1746
105. Tepper PG, Randolph JF Jr, McConnell DS, Crawford SL, El Khoudary SR, Joffe H, et al. Trajectory clustering of estradiol and follicle-stimulating hormone during the menopausal transition among women in the Study of Women’s Health Across the Nation (SWAN). J Clin Endocrinol Metab. (2012) 97:2872–80. doi: 10.1210/jc.2012-1422
106. Harlow SD, Gass M, Hall JE, Lobo R, Maki P, Rebar RW, et al. Executive summary of the stages of reproductive aging workshop + 10: addressing the unfinished agenda of staging reproductive aging. Climacteric. (2012) 15:105–14. doi: 10.3109/13697137.2011.650656
107. Jurgens SJ, Choi SH, Morrill VN, Chaffin M, Pirruccello JP, Halford JL, et al., Analysis of rare genetic variation underlying cardiometabolic diseases and traits among 200,000 individuals in the UK Biobank. Nat Genet. (2022) 54:240–50. doi: 10.1038/s41588-021-01011-w
108. Knowles JW, Ashley EA. Cardiovascular disease: the rise of the genetic risk score. PLoS Med. (2018) 15:e1002546. doi: 10.1371/journal.pmed.1002546
109. Nilsson PM, Tuomilehto J, Rydén L. The metabolic syndrome—what is it and how should it be managed? Eur J Prev Cardiol. (2019) 26:33–46. doi: 10.1177/2047487319886404
110. Cassidy S, Chau JY, Catt M, Bauman A, Trenell MI. * Cross-sectional study of diet, physical activity, television viewing and sleep duration in 233,110 adults from the UK Biobank; the behavioural phenotype of cardiovascular disease and type 2 diabetes. BMJ Open. (2016) 6:e010038. doi: 10.1136/bmjopen-2015-010038
111. Seidu BS, Osman H, Seidu S. Lifestyle or pharmacotherapy in cardio-metabolic disease prevention. Ther Adv Cardiovasc Dis. (2023) 17:17539447231177175. doi: 10.1177/17539447231177175
112. Cruz-Jentoft AJ, Sayer AA. Sarcopenia. Lancet. (2019) 393:2636–46. doi: 10.1016/S0140-6736(19)31138-9
113. Linge J, Heymsfield SB, Dahlqvist Leinhard O. On the definition of sarcopenia in the presence of aging and obesity—initial results from UK Biobank. J Gerontol A. (2020) 75:1309–16. doi: 10.1093/gerona/glz229
114. Marzetti E, Leeuwenburgh C. Skeletal muscle apoptosis, sarcopenia and frailty at old age. Exp Gerontol. (2006) 41:1234–8. doi: 10.1016/j.exger.2006.08.011
115. Wall BT, Dirks ML, Van Loon LJ. Skeletal muscle atrophy during short-term disuse: implications for age-related sarcopenia. Ageing Res Rev. (2013) 12:898–906. doi: 10.1016/j.arr.2013.07.003
116. Geraci A, Calvani R, Ferri E, Marzetti E, Arosio B, Cesari M. Sarcopenia and menopause: the role of estradiol. Front Endocrinol. (2021) 12:682012. doi: 10.3389/fendo.2021.682012
117. Messier V, Rabasa-Lhoret R, Barbat-Artigas S, Elisha B, Karelis AD, Aubertin-Leheudre M. Menopause and sarcopenia: a potential role for sex hormones. Maturitas. (2011) 68:331–6. doi: 10.1016/j.maturitas.2011.01.014
118. Barnes JN, Harvey RE, Zuk SM, Lundt ES, Lesnick TG, Gunter JL, et al., Aortic hemodynamics and white matter hyperintensities in normotensive postmenopausal women. J Neurol. (2017) 264:938–45. doi: 10.1007/s00415-017-8476-1
119. Debette S, Seshadri S, Beiser A, Au R, Himali J, Palumbo C, et al. Midlife vascular risk factor exposure accelerates structural brain aging and cognitive decline. Neurology. (2011) 77:461–8. doi: 10.1212/WNL.0b013e318227b227
120. Koohi F, Harshfield EL, Markus HS. Contribution of conventional cardiovascular risk factors to brain white matter hyperintensities. J Am Heart Assoc. (2023) 12:e030676. doi: 10.1161/JAHA.123.030676
121. Taylor-Bateman V, Gill D, Georgakis MK, Malik R, Munroe P, Traylor M, et al., Cardiovascular risk factors and MRI markers of cerebral small vessel disease: a mendelian randomization study. Neurology. (2022) 98:e343–51. doi: 10.1212/WNL.0000000000013120
122. van Dijk EJ, Breteler MM, Schmidt R, Berger K, Nilsson L-G, Oudkerk M, et al., The association between blood pressure, hypertension, and cerebral white matter lesions: cardiovascular determinants of dementia study. Hypertension. (2004) 44:625–30. doi: 10.1161/01.HYP.0000145857.98904.20
123. Bobb JF, Schwartz BS, Davatzikos C, Caffo B. Cross-sectional and longitudinal association of body mass index and brain volume. Hum Brain Mapp. (2014) 35:75–88. doi: 10.1002/hbm.22159
124. Shaw M, Sachdev PS, Abhayaratna W, Anstey K, Cherbuin N. Body mass index is associated with cortical thinning with different patterns in mid-and late-life. Int J Obes. (2018) 42:455–61. doi: 10.1038/ijo.2017.254
125. Soreca I, Rosano C, Jennings JR, Sheu LK, Kuller LH, Matthews KA, et al. Gain in adiposity across 15 years is associated with reduced gray matter volume in healthy women. Psychosom Med. (2009) 71:485. doi: 10.1097/PSY.0b013e3181a5429d
126. Ambikairajah A, Tabatabaei-Jafari H, Walsh E, Hornberger M, Cherbuin N. Longitudinal changes in fat mass and the hippocampus. Obesity. (2020) 28:1263–9. doi: 10.1002/oby.22819
127. Alber J, Alladi S, Bae H-J, Barton DA, Beckett LA, Bell JM, et al., White matter hyperintensities in vascular contributions to cognitive impairment and dementia (VCID): knowledge gaps and opportunities. Alzheimer Dement. (2019) 5:107–17. doi: 10.1016/j.trci.2019.02.001
128. Armstrong NJ, Mather KA, Sargurupremraj M, Knol MJ, Malik R, Satizabal CL, et al., Common genetic variation indicates separate causes for periventricular and deep white matter hyperintensities. Stroke. (2020) 51:2111–21. doi: 10.1161/STROKEAHA.119.027544
129. Griffanti L, Jenkinson M, Suri S, Zsoldos E, Mahmood A, Filippini N, et al., Classification and characterization of periventricular and deep white matter hyperintensities on MRI: a study in older adults. Neuroimage. (2018) 170:174–81. doi: 10.1016/j.neuroimage.2017.03.024
130. Keller JA, Kant IM, Slooter AJ, van Montfort SJ, van Buchem MA, van Osch MJ, et al. Different cardiovascular risk factors are related to distinct white matter hyperintensity MRI phenotypes in older adults. NeuroImage Clin. (2022) 35:103131. doi: 10.1016/j.nicl.2022.103131
131. Thurston RC, Wu M, Chang Y-F, Aizenstein HJ, Derby CA, Barinas-Mitchell EA, et al. Menopausal vasomotor symptoms and white matter hyperintensities in midlife women. Neurology. (2023) 100:e133–41. doi: 10.1212/WNL.0000000000201401
132. Walhovd KB, Lövden M, Fjell AM. Timing of lifespan influences on brain and cognition. Trends Cogn Sci (2023) 27(10):901–15. doi: 10.1016/j.tics.2023.07.001
133. Sachdev PS, Thalamuthu A, Mather KA, Ames D, Wright MJ, Wen W. White matter hyperintensities are under strong genetic influence. Stroke. (2016) 47:1422–8. doi: 10.1161/STROKEAHA.116.012532
134. Backhouse EV, McHutchison CA, Cvoro V, Shenkin SD, Wardlaw JM. Early life risk factors for cerebrovascular disease: a systematic review and meta-analysis. Neurology. (2017) 88:976–84. doi: 10.1212/WNL.0000000000003687
135. Henrich J, Heine SJ, Norenzayan A. Most people are not weird. Nature. (2010) 466:29. doi: 10.1038/466029a
136. Fry A, Littlejohns TJ, Sudlow C, Doherty N, Adamska L, Sprosen T, et al. Comparison of sociodemographic and health-related characteristics of UK Biobank participants with those of the general population. Am J Epidemiol. (2017) 186:1026–34. doi: 10.1093/aje/kwx246
137. Mensah GA, Roth GA, Fuster V. The global burden of cardiovascular diseases and risk factors 2020 and beyond (2019).
138. Nichols E, Szoeke CE, Vollset SE, Abbasi N, Abd-Allah F, Abdela J, et al., Global, regional, and national burden of Alzheimer’s disease and other dementias, 1990–2016: a systematic analysis for the global burden of disease study 2016. Lancet Neurol. (2019) 18:88–106. doi: 10.1016/S1474-4422(18)30403-4
139. Mauvais-Jarvis F, Merz NB, Barnes PJ, Brinton RD, Carrero J-J, DeMeo DL, et al., Sex and gender: modifiers of health, disease, and medicine. Lancet. (2020) 396:565–82. doi: 10.1016/S0140-6736(20)31561-0
140. Gerdts E, Regitz-Zagrosek V. Sex differences in cardiometabolic disorders. Nat Med. (2019) 25:1657–66. doi: 10.1038/s41591-019-0643-8
141. Hogervorst E, Temple S, O’Donnell E. Sex differences in dementia. Sex differences in brain function and dysfunction. Germany: Current Topics in Behavioral Neurosciences (2023). p. 309–31.
142. Ferretti MT, Iulita MF, Cavedo E, Chiesa PA, Schumacher Dimech A, Santuccione Chadha A, et al., Sex differences in alzheimer disease—the gateway to precision medicine. Nat Rev Neurol. (2018) 14:457–69. doi: 10.1038/s41582-018-0032-9
143. de Lange A-MG, Jacobs EG, Galea LA. The scientific body of knowledge: whose body does it serve? A spotlight on women’s brain health. Front Neuroendocrinol. (2021) 60:100898. doi: 10.1016/j.yfrne.2020.100898
144. Jacobs EG. Only 0.5% of neuroscience studies look at women’s health. Here’s how to change that. Nature. (2023) 623:667. doi: 10.1038/d41586-023-03614-1
Keywords: menopause, female health, cardiometabolic health, body anthropometrics, white matter hyperintensities, brain health, UK Biobank
Citation: Schindler LS, Subramaniapillai S, Ambikairajah A, Barth C, Crestol A, Voldsbekk I, Beck D, Gurholt TP, Topiwala A, Suri S, Ebmeier KP, Andreassen OA, Draganski B, Westlye LT and de Lange A-MG (2023) Cardiometabolic health across menopausal years is linked to white matter hyperintensities up to a decade later. Front. Glob. Womens Health 4:1320640. doi: 10.3389/fgwh.2023.1320640
Received: 12 October 2023; Accepted: 6 December 2023;
Published: 21 December 2023.
Edited by:
Aleksandra Pikula, University of Toronto, CanadaReviewed by:
Elizabeth Thomas, Monash Alfred Psychiatry Research Centre, Australia© 2023 Schindler, Subramaniapillai, Ambikairajah, Barth, Crestol, Voldsbekk, Beck, Gurholt, Topiwala, Suri, Ebmeier, Andreassen, Draganski, Westlye and de Lange. This is an open-access article distributed under the terms of the Creative Commons Attribution License (CC BY). The use, distribution or reproduction in other forums is permitted, provided the original author(s) and the copyright owner(s) are credited and that the original publication in this journal is cited, in accordance with accepted academic practice. No use, distribution or reproduction is permitted which does not comply with these terms.
*Correspondence: Louise S. Schindler bG91aXNlLnNjaGluZGxlckBjaHV2LmNo Ann-Marie G. de Lange YW5uLW1hcmllLmRlLWxhbmdlQGNodXYuY2g=
Disclaimer: All claims expressed in this article are solely those of the authors and do not necessarily represent those of their affiliated organizations, or those of the publisher, the editors and the reviewers. Any product that may be evaluated in this article or claim that may be made by its manufacturer is not guaranteed or endorsed by the publisher.
Research integrity at Frontiers
Learn more about the work of our research integrity team to safeguard the quality of each article we publish.