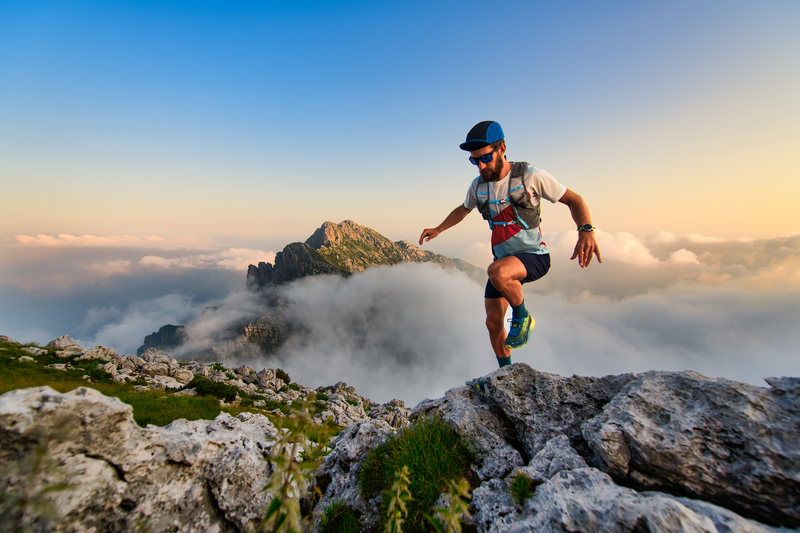
95% of researchers rate our articles as excellent or good
Learn more about the work of our research integrity team to safeguard the quality of each article we publish.
Find out more
ORIGINAL RESEARCH article
Front. Glob. Womens Health , 26 February 2021
Sec. Maternal Health
Volume 2 - 2021 | https://doi.org/10.3389/fgwh.2021.599731
This article is part of the Research Topic Maternal Health in Conflict Settings View all 8 articles
Introduction: African countries facing conflict have higher levels of maternal mortality. Understanding the gaps in the utilization of high-quality maternal health care is essential to improving maternal survival in these states. Few studies have estimated the impact of conflict on the quality of health care. In this study, we estimated the impact of conflict on the quality of health care in Kenya, a country with multiple overlapping conflicts and significant disparities in maternal survival.
Materials and Methods: We drew on data on the observed quality of 553 antenatal care (ANC) visits between January and April 2010. Process quality was measured as the percentage of elements of client–provider interactions performed in these visits. For structural quality, we measured the percentage of required components of equipment and infrastructure and the management and supervision in the facility on the day of the visit. We spatially linked the analytical sample to conflict events from January to April 2010. We modeled the quality of ANC as a function of exposure to conflict using spatial difference-in-difference models.
Results: ANC visits that occurred in facilities within 10,000 m of any conflict event in a high-conflict month received 18–21 percentage points fewer components of process quality on average and had a mean management and supervision score that was 12.8–13.5 percentage points higher. There was no significant difference in the mean equipment and infrastructure score at the 5% level. The positive impact of conflict exposure on the quality of management and supervision was driven by rural facilities. The quality of management and supervision and equipment and infrastructure did not modify the impact of conflict on process quality.
Discussion: Our study demonstrates the importance of designing maternal health policy based on the context-specific evidence on the mechanisms through which conflict affects health care. In Kenya, deterioration of equipment and infrastructure does not appear to be the main mechanism through which conflict has affected ANC quality. Further research should focus on better understanding the determinants of the gaps in process quality in conflict-affected settings, including provider motivation, competence, and incentives.
In September 2000, the United Nations Millennium Summit concluded with the adoption of the Millennium Development Goals, including a commitment to reduce the global maternal mortality ratio (MMR) by three-quarters between 1990 and 2015. Between 2000 and 2017, the global MMR fell by 38%, from 342 to 211 deaths per 100,000 live births. This progress was significantly short of the target (1). Declines in maternal mortality were also uneven between the two regions with the highest burden of disease. While the MMR in South Asia declined by 58.7% between 2000 and 2017, Sub-Saharan Africa (SSA) saw a more modest decline of 38.8% (1). About 66% of global maternal deaths still occur in SSA. Relatively higher average levels of maternal mortality have persisted in African countries facing conflict and post-conflict (1). In a 2007 study, the median-adjusted MMR was 45% higher in countries with recent conflict (2). Countries with internal conflict have also experienced greater subnational disparities in maternal outcomes (1). Therefore, understanding the determinants of maternal mortality in conflict-affected countries in SSA is essential to reducing disparities in maternal survival both across and within countries.
Good quality maternal health care is essential to maternal survival but might be particularly challenging to provide and deliver in conflict-affected contexts (1). In most cases, maternal deaths are preventable (3). An estimated 40% of pregnant women will experience obstetric disorders that are not immediately fatal given medical intervention (3, 4). However, in countries with high mortality rates, maternal interventions are often not accessible or are of suboptimal quality, leading to lost opportunities to improve outcomes. Global coverage of antenatal care (ANC) and skilled birth attendance (SBA) is above 75% on average. However, coverage in several conflict-affected states is below this average. ANC coverage falls to 9.4% in Somalia, and SBA coverage in Afghanistan is 17.8% (5). Quality-adjusted coverage is even lower in high-mortality countries. In a cross-sectional study of eight countries, the quality-adjusted coverage of ANC was 28.3%, far below the proportion of women who received any ANC at 95.7% (6). The quality of maternal health care has also been shown to predict utilization in African countries, illustrating the interconnectedness of the challenges of underutilization and poor quality (7). Health system investments that have increased maternal health care use without improving the quality of care have contributed minimally to reducing mortality. Illustratively, India's Janani Suraskha Yojana, a program aimed at removing financial barriers to giving birth in health facilities, led to a 5.6 percentage points increase in SBA in districts with higher program coverage (8). Nonetheless, there was no significant reduction in mortality in districts with higher program coverage, attributed in part to the lack of access to high-quality obstetric care (9, 10).
A clear conceptualization of quality is essential to examining its implications for health service delivery in conflict-affected contexts. Maternal health care is of high quality to the degree that these health services increase the likelihood of the desired health outcomes and are consistent with current professional knowledge (11). In a seminal publication, Donabedian described three measurable components of the quality of care (12). Structural quality refers to health care inputs, including the adequacy of facilities and equipment, the qualifications of medical staff, and management procedures. Process quality refers to the extent to which client–provider interactions are consistent with current professional knowledge, including the use of evidence-based guidelines, minimization of errors, and avoidance of delays (13). Structural and process quality interacts, as improvements in client–provider interactions correlate with the adequacy of health care inputs, including the availability of providers, and facility management. However, process quality also depends on provider competence, intrinsic motivation, and external incentives (14, 15). Outcome quality reflects the combined impact of structural and process quality on health outcomes and is the ultimate validator of high quality. There may be a time lag between the delivery of services with adequate structural and process quality and improvements in health outcomes. Outcome measures attributable to improvements in quality may also be more difficult to measure than structural and process measures, particularly in data-poor environments. Furthermore, despite adequate structural and process quality, health outcomes may not improve due to other factors beyond the control of the health facility and provider, including access to nutrition, water, and sanitation. While there are other conceptualizations of the quality of health care, we draw on Donabedian's framework in reviewing the empirical literature (13, 16).
Empirical studies of the potential impact of conflict on maternal mortality have examined its implications for health care utilization and structural and process quality. To this end, there is a significant body of research that links maternal health care use to conflict, drawing on qualitative and quantitative approaches. In a study of the Syrian conflict, DeJong et al. (17) demonstrate that ANC use fell from 87.7 to 62%, whereas SBA coverage decreased from 96.2 to 72%, following conflict exposure. Akseer et al. (18) published a time-series ecological study in 2020 that showed that inequalities in maternal health care use were higher in conflict than in non-conflict countries, with lower coverage rates among the poorest, least educated, and rural-dwelling households. Chukwuma and Ekhator-Mobayode also show that exposure to the Boko Haram insurgency reduced the probability of receiving any ANC and SBA, drawing on spatial difference-in-difference (DID) models (19). Price and Bohara document a negative correlation between ANC coverage and violent events in Nepal during the Maoist insurgency after controlling for other determinants of health care access. Reductions in ANC, SBA, and other maternal health care have also been demonstrated in Lebanon, Eastern Burma, and Uganda (20–22). Hence, overall, the empirical evidence indicates that exposure to conflict predicts falls in maternal health care utilization.
Several descriptive studies have highlighted the important implications of conflict for the supply and adequacy of facilities, equipment, and health workers, which have direct negative implications for the structural quality of health care. Studies of conflict in Nicaragua, Burundi, Northern Uganda, and Syria have described the deterioration of health system resources, including through the destruction of facility infrastructure, disruption of supply chains for medical supplies, and direct attacks or emigration of health workers (23–25). Chi et al. (23) also describe disruptions in the training of health workers, preferential emigration of skilled health workers, and overall reductions in the knowledge levels of health professionals. Fewer studies estimate the impact of conflict on structural or process quality quantitatively (26). Notably, a rigorous 2019 study by Akseer et al. (26) finds that equipment functionality reduced following moderate or severe intensity conflict, proxied by conflict-associated fatalities, between 2004 and 2010. Between 2011 and 2016, they do not find any impact of conflict intensity on equipment functionality. They also find no significant impact of conflict on drug availability, infrastructure adequacy and functionality, and service delivery interactions, including history, physical examinations, and client counseling. Akseer et al. (26) also document an increase in vaccine availability and health care professional knowledge in conflict-affected provinces. Hence, empirical estimations of the impact of conflict on the quality of care do not unambiguously align with findings described in the qualitative literature. There is a need for further evaluations of the implications of conflict for structural and process quality, across contexts, and an exploration of potential mechanisms for these effects empirically.
In this study, we focus on Kenya, given the availability of data on the quality of maternal health care through the Service Provision Assessment (SPA) that can be temporally and spatially linked to conflict events in the Armed Conflict Location and Events Data Project (ACLED) (27, 28). Kenya is the seventh most violent country in the ACLED, with over 3,500 politically violent events recorded between 1997 and 2013 (29). The multiple overlapping conflicts are attributable to a range of factors, including the politicization of ethnicity, inequities in land allocation, exploitation of local politics by Al-Shabaab, and the proliferation of small arms (30). Since 2010, there has been an increase in the intensity and frequency of violence, including terror attacks, conflicts between communities, and extra-judicial executions (31). Anecdotal accounts have linked these conflicts to subsequent disruptions in health and educational services (31). There are also significant disparities in maternal health outcomes in Kenya. Between 1990 and 2016, the MMR in Kenya fell from 315.7 to 257.6 deaths per 100,000 live births. However, maternal mortality increased in 25 of the 47 counties. Maternal mortality increased most substantially in Kirinyaga, by 4.9%, which is a county with a relatively high level of conflict incidence in Central Kenya (32). The coverage of ANC and SBA has remained low and inequitable in Kenya, with higher disparities in coverage for ANC (33, 34). However, studies examining the determinants of subnational disparities in maternal health care use in Kenya have not considered an independent role for conflict (35–37). There are also no published studies estimating the impact of conflict on the quality of health care in Kenya. Hence, in this study, we estimate the impact of conflict exposure on the quality of ANC in Kenya.
The empirical analysis draws on data on the observed process and structural quality of ANC in the 2010 Kenya SPA, the locations of temporally and spatially matched conflict events in the ACLED, sociodemographic predictors of ANC use and quality in the SPA, and data on urbanicity of health facility locations from the Africover dataset.
The study sample was constructed from the 2010 Kenya SPA, which is a survey that collected nationally representative information from 695 health facilities, including hospitals, health centers, maternity and nursing homes, clinics, and stand-alone voluntary counseling and testing facilities offering maternal and child health care (27). The final sample covered ~11% of facilities in the country. Data collection commenced on 21st January 2010 and ended on 18th May 2010, which coincides with a year of increased conflict intensity and frequency in Kenya (31). In each facility, health service providers were sampled, if they were present on the day of the survey and provided one of the services being assessed. In facilities with fewer than eight health care providers, all the providers that were present were interviewed. In facilities with more than eight providers, not more than eight providers were interviewed. The survey includes responses from 3,051 providers.
Where many clients were present and eligible for observation, consultations were observed for up to 5 clients per provider and up to 15 clients per facility. To achieve the target number of observations, the total number of expected clients was divided by five to derive the nth interval for selecting the next client to be observed (27). A total of 1,409 ANC visits were observed. The Kenya SPA includes data on facility structural quality, including staffing levels, training and supervision of staff, availability of service delivery protocols, basic infrastructure (electricity, water, and client amenities), and systems for general facility management. Observations of ANC consultations assessed the process quality of care, including information shared between the client and provider, physical examinations, and treatment provided. Our analytical sample was restricted to the first ANC visit in a woman's pregnancy at the index facility, given that most recommended clinical content, for adequate process quality, occurs during this visit. This is consistent with recent studies that have examined the quality of ANC (38). Given that there was only one eligible ANC visit in May 2010, we focus the analysis on ANC visits that occurred between January and April 2010. Our analysis is robust to inclusion or exclusion of this datapoint. The survey also includes data on the geo-coordinates of the health facility, the date of the survey, as well as the sociodemographic characteristics of each provider and client.
We spatially linked the analytical sample with data from the ACLED, which documents the dates, actors, types of violence, locations, geo-coordinates, and fatalities of all reported political violence and protest events across Africa, South Asia, Southeast Asia, the Middle East, Central Asia and the Caucasus, and Southeastern and Eastern Europe and the Balkans. Finally, we derived information on the urbanicity of facility locations, by matching the geo-coordinates of each facility with urban areas as defined in the Africover dataset compiled by the Food and Agricultural Organization of the United Nations (39).
We estimated DID models of the impact of conflict on the quality of women's first ANC visits in Kenya, by comparing structural and process quality measures, during high- and low-conflict months, in health facilities at different levels of exposure to conflict.
We identified a total of 40 conflict events in Kenya within the ACLED that occurred between January and April 2010, overlapping with the timing of the analytical sample of ANC observations from the SPA. The ACLED events consist of violent events, demonstration events, and non-violent actions. Violent events in the ACLED are further classified as follows: battles, explosions/remote violence, and violence against civilians. Demonstration events are also classified into protests and riots, and non-violent actions in the ACLED are events related to strategic developments. The month of January is notable for recording 45% of all conflict events, 62% of all fatalities linked to conflict, 42% of violent conflict events, and 51% of fatalities linked to violent conflict (Table 1). Hence, regardless of the measure of conflict intensity considered, the month of January has a higher conflict intensity than other months. In our main models, we consider January to be a high-conflict month and compared January to the other months, which are considered low-conflict months. Our main models examine the impact of exposure to any conflict. We also examined the sensitivity of our findings to the type of conflict event, by limiting to violent and non-violent events, respectively.
We determined facility exposure to conflict based on the distance from any conflict event within the ACLED sample to the facility location. We calculated the straight-line distance between each sampled facility to the location of all conflict attacks between January and April 2010. We classified a facility as exposed to a conflict event if any event was located within the catchment area of the facility. There is no consensus on the definition of the radius in which conflict may affect health care. Furthermore, the distances used to define catchment areas in health service research, within which physical access to care can be affected, in SSA, vary significantly (40). Hence, in our main models, we define the facility catchment area as a radius of 10,000 m. We examined the sensitivity of our findings to the definition of the catchment area radius, by varying this distance from 3,000 to 50,000 m.
We modeled the process and structural quality of ANC as a function of exposure to conflict and other determinants of high-quality maternal health care coverage, using DID models, specified as follows:
Below, we describe the main empirical model in the order in which variables appear. All model variables are listed in Table 2.
Qilt is the score on an index of quality for a first ANC visit i at facility l in month t. We constructed three separate quality indexes—one process quality index and two structural quality indexes, of equipment and infrastructure and management and supervision, respectively.
We constructed an index of process quality drawing on 20 binary indicators of essential elements of the client–provider interaction during the first ANC visit, including history-taking, physical examination, laboratory investigations, treatment, and counseling, identified in the WHO recommended guidelines at the time (41). We calculated the percentage of items fulfilled in each visit, to develop a continuous index ranging from 0 to 100, whereby a higher score corresponded to greater adherence to the recommended clinical practices. A description of the components of the process quality index can be found in Table 3.
We created indexes of structural quality of the ANC clinic and the facility using variables within the SPA dataset and consistent with recent studies of ANC quality (38). We then assigned to each ANC visit the structural quality score for each index on the day of that visit. The equipment and infrastructure index included 27 binary indicators of functional equipment, safety and sanitation items in the ANC clinic, and overall facility infrastructure. A separate index of management and supervision included 12 binary indicators of actions undertaken by district supervisors during facility visits and internal facility management processes, which have implications for the supervision of ANC services. We calculated the percentage of items that were fulfilled, to develop continuous indexes ranging from 0 to 100. A description of the components of the indexes can be found in Tables 4, 5.
The variable, HCMt, is a binary variable indicating if the ANC visit occurred in a high-conflict month and is equal to 1 if the month was January, and 0 otherwise. The variable, EFl, is a binary variable indicating if the facility in which the ANC visit occurred was exposed to any conflict event, equal to 1 if the facility was within 10,000 m of a conflict event, and 0 otherwise. The DID estimator can be conceptualized as the average difference between HCMt = 1 and HCMt = 0 in areas not within 10,000 m (EFl = 0) subtracted from the average difference between HCM = 1 and HCM = 0 in areas within 10,000 m of a conflict even (EFl = 1).
In our DID model, the parameter, β3, measures the impact of conflict on the quality of health care. We assume that in the absence of high intensity conflict, there would be no significant difference in the trends in the quality of ANC in conflict-exposed and -unexposed facilities. This is the corollary of the parallel trends' assumption given the absence of a distinct pre- and post-conflict period. We provide evidence in support of this assumption.
Lastly, the variable, Xilt, is a vector of controls that are client-, provider-, and facility-level determinants of high-quality maternal health care use that were collected in the SPA. We included a categorical variable for educational attainment of the ANC client, coded as 1 if the individual had at least secondary education, and 0 otherwise; a categorical variable for provider type, coded as 1 if the provider was a medical doctor, registered nurse, or registered midwife, and 0 otherwise; a categorical variable for facility type, coded as 1 if the facility was a national-, provincial-, or district-level hospital (which correspond to secondary and tertiary health facilities), and 0 otherwise; and a binary variable for urbanicity of facility location, coded as 1 if the facility was located in an urban area, and 0 otherwise. Our main model included client sampling weights and restricted to the 91% of observations for which no covariates were missing.
Of the 1,409 ANC visits observed, 553 were the first ANC visits in the client's pregnancy in the index facility and thus constitute the analytical sample. Of the 553 first ANC visits, 363 (66%) were made by women with primary-level education or less; 246 (44%) were attended by a doctor, registered nurse, or registered midwife; and 158 (29%) occurred in national, provincial, or district hospitals, whereas 103 (19%) occurred in facilities located in a rural area. A total of 87 visits (16%) occurred in January 2010. The number of clients that occurred within a facility exposed to any conflict varied with the catchment area radius, ranging from 32 visits (5.7%) at 3,000 m to 326 visits (59%) at 50,000 m. For the catchment area radius of 10,000 m, 79 visits (14.3%) occurred in health facilities exposed to conflict. These descriptive statistics are summarized in Tables 6, 7.
We examined the variation in process and structural quality scores within the analytical sample (Table 8). The process quality score was 70% on average. The most commonly received components of process quality were examination of blood pressure (94%), measurement of client weight (94%), measurement of fundal height (91%), and recording of maternal age (90%). The five least commonly received components were a vaginal examination (9%), counseling on breastfeeding (32%), nutrition counseling (53%), and discussion of danger signs in pregnancy (56%). The mean process quality score did not vary significantly by facility exposure to conflict, month, or characteristics of the client, provider, or facility (Table 9). There were also no significant differences in the percentage of ANC visits that received any of the 20 process quality components at the 5% level.
The index of equipment and infrastructure was 70% on average. The majority of the facilities had all rooms equipped with boxes for sharps (98%), at least one functional client toilet (98%), an appropriate waiting room (98%), clean floor and surfaces (96%), and an ambulance or capacity to communicate with other facilities (95%). The least commonly observed components of the equipment and infrastructure index were adequate waste disposal (19%), an examination light in the ANC clinic (36%), appropriate storage of specific equipment, such as speculum and forceps (39%), central electricity or a functional generator with fuel (41%), and receiving the last medical supply within the past 4 weeks (45%).
Facilities located within 10,000 m of a conflict event were 14 percentage points more likely to have a fetal stethoscope, 7 percentage points more likely to have a functional scale, 16 percentage points less likely to adequately dispose of waste, and 32 percentage points more likely to have received a medication supply within the past 4 weeks, compared with facilities outside the catchment area radius. There were no other significant differences between unexposed and exposed facilities on components of the equipment and infrastructure score. The mean score for equipment and infrastructure was higher in facilities used by women with secondary education or above; in national, provincial, and district hospitals; and in urban areas. However, it did not significantly vary by provider cadre and month at the 5% level (Table 9).
The index of management and supervision was 71%. The most commonly observed components of management and supervision quality were receiving a supervisory visit within the last 6 months (97%), possessing a Health Management Information System (HMIS) unit (95%), discussing problems with a supervisor (93%), a supervisor checking the registers (91%), and a management meeting holding once every 6 months (84%). The least commonly observed components were a record of personnel receiving HMIS training in the past year (24%), having an external supervisory visit in the past 6 months (44%), a supervisor holding an official staff meeting (56%), a supervisor observing service provision (57%), and a staff community meeting having held within the past 6 months (65%).
On average, less facilities located within 10,000 m of a conflict event had personnel in more than one unit that had received training in HMIS in the past 12 months (by 16 percentage points), but more of these facilities had a HMIS unit (by 6 percentage points), a management meeting in the past 6 months (by 18 percentage points), and a staff community meeting within the past 6 months (by 31 percentage points). Overall, the mean management and supervision score in facilities within 10,000 m of a conflict event was 10 percentage points below the mean among facilities outside the catchment area radius. The mean management and supervision score was higher in national, provincial, and district hospitals, as well as in rural areas, and did not significantly vary by women's education, provider cadre, and month at the 5% level (Table 9).
In our main DID models, we show that ANC visits that occurred in facilities within 10,000 m of any conflict event in a high-conflict month received 18.0–21.1 percentage points fewer components of process quality on average. This effect was statistically significant at the 5% level, with or without adjustment for confounders. There was no significant difference in the mean equipment and infrastructure score, at the 5% level. However, facilities within 10,000 m of any conflict event in a high-conflict month had a mean management and supervision score that was 12.8–13.5 percentage points higher on average. This effect was also statistically significant at the 5% level, with adjustment for confounders. We summarize these results in Table 10.
We examined the sensitivity of our findings to the exposure definition. First, we varied the catchment area radius from 3,000 to 50,000 m and re-estimated the DID models (Figure 1). We find that the point estimates of the impact of conflict exposure on process quality remained negatively signed and varied between −0.211 and −0.133 for catchment area radii below 10,000 m. However, the 95% confidence intervals overlapped with the null value between 20,000 and 35,000 m. Similar to the main models, there was no significant difference between exposed and unexposed facilities in the equipment and infrastructure score. At lower catchment area radii, where exposed facilities were closer to conflict, the point estimates of the impact were negatively signed. We find that the point estimates of the impact of conflict exposure on management and supervision were positive and statistically significant at the 5% level between 3,000 and 10,000 m and varied between 0.128 and 0.182 for catchment area radii below 10,000 m. As the catchment area radius increased, including facilities further away from the location of the conflict event, the point estimates become null and then negatively signed. The 95% confidence intervals overlap with the null value between 15,000 and 50,000 m. These findings are summarized in Table 11.
We then re-estimated the DID models, restricting to violent and non-violent conflicts, respectively. The ACLED defines a violent event as one that involves the exchange of armed force, or the use of armed force at close distance, between armed groups capable of inflicting harm upon the opposing side, including battles, explosions, and violence against civilians (42). Our findings do not qualitatively change following restriction to violent events or non-violent events only. The process quality score for visits in facilities within 10,000 m of a violent conflict event is 18.2 percentage points lower, whereas location within 10,000 m of a non-violent conflict event results in a score that is 22.1 percentage points lower on average, compared with facilities outside this radius. Both estimates are statistically significant at the 5% level. There is no statistically significant impact of conflict on the equipment and infrastructure score, irrespective of conflict type. The management and supervision score of facilities within 10,000 m of a violent conflict event is 20.4 percentage points above, whereas location within 10,000 m of a non-violent conflict event results in a score that is 9.3 percentage points higher on average, compared with facilities outside this radius. Both estimates are statistically significant at the 5% level. These results are summarized in Table 12.
We examined heterogeneity in the impact of conflict exposure on ANC quality by client, provider, and facility characteristics (Table 13). The DID estimates remained statistically significant at the 5% level for provider type, equipment and infrastructure index, management and supervision index, and for area of residence. We found that among women with secondary or more education, exposure to conflict had a more negative impact on process quality, with a score that was 23.1 percentage points lower on average relative to women with primary education or less. We also find that the positive impact of conflict exposure on the quality of management and supervision is driven by rural facilities. All other interaction terms are not statistically significant at the 5% level. We also examined effect modification via adequacy of facility equipment, infrastructure, management, and supervision on the impact of conflict exposure on the process quality of ANC. However, we did not find significant impacts at the 5% level (Table 14).
Our DID models estimate the impact of conflict on ANC quality on the assumption that in the absence of conflict, there would be no significant difference in trends in the quality of ANC in conflict-exposed and -unexposed facilities. We provide evidence in support of this assumption by re-estimating the DID models, excluding the observations in the high-conflict month of January, and adjusting for confounders (Table 15). Hence, we compare ANC visits in facilities within 10,000 m of any conflict, and otherwise, in the months of February, March, and April. We assign the status of high-conflict month to February. We do not find significant differences in the process or structural quality of ANC care in facilities within 10,000 m of any conflict event, and otherwise, when we restrict to low-conflict months. This suggests that the effects we find in our main models are unlikely to reflect chance.
In this study, we estimate the impact of conflict exposure on ANC quality in Kenya, a country that has recorded subnational disparities in maternal health outcomes and an increase in multiple overlapping conflict events. We draw on observed indicators of the quality of patient–provider interactions, infrastructure and equipment, and management and supervision in 695 health facilities. Our study is one of the few that quantifies the impact of conflict on maternal health care quality in an African country. We find that when an initial ANC visit occurs in a facility within 10,000 m of any conflict event, in a high-conflict month, the client receives fewer recommended components of care in evidence-based clinical guidelines, but the facilities on average have higher management and supervision scores. We do not find any significant impact of conflict exposure on the mean equipment and infrastructure score for the ANC clinics and facilities. Our findings did not qualitatively vary in a statistically significant manner, at the 5 or 10% level, on limiting our analysis to violent or non-violent conflict events, varying the facility catchment area radius, or adjusting for confounders.
The determinants of gaps in access to high-quality ANC and other maternal health services are multifaceted, including poverty, lower educational levels, and physical distance from health facilities (35–37). In this study, we have demonstrated that conflict is also an important determinant of the quality of ANC that women receive in this context. Our study highlights the importance of designing and targeting maternal health policy based on the context-specific evidence on the mechanisms through which conflict affects health care. There is significant descriptive and quasi-experimental evidence from other contexts of the negative impact of conflict on facility infrastructure and equipment, including in the neighboring countries of Burundi and Uganda (23–25). However, we find that in Kenya, deterioration of equipment and infrastructure does not appear to be the main mechanism through which conflict has hitherto affected ANC quality. In Afghanistan, Akseer et al. (26) find negative impacts of conflict on equipment and infrastructure between 2004 and 2010 and no effect between 2011 and 2016. Our models demonstrate clear reductions in the process quality of ANC, which includes history-taking, physical examination, laboratory tests, and counseling, among facilities exposed to conflict in high-conflict months. This contrasts sharply with the study by Akseer et al. (26) who report no significant impacts of conflict on history, physical examination, and client counseling.
We did not find significant difference in the impact of violent and non-violent conflicts on the process quality of care. This aligns with the absence of a significant negative impact of conflict on equipment and infrastructure in the surveyed facilities and the absence of effect modification of the impact of conflict on process quality by the equipment and infrastructure index. Facilities in rural areas score 9.5 percentage points higher, on average, on the management and supervision score. We also find that when facilities are exposed to conflict, there is increased management and supervision support, particularly in rural areas. Since 1994, the Kenyan Government has systematically devolved health care decision-making. In 2010, a new constitution created a decentralized government, increasing district-level autonomy and financing for health care (43). Rural facilities appear to have benefitted from increased supervision support. It has been argued that management and supervision may play a role in increasing the resilience of service delivery to shocks, including conflict (44). However, in this study, management and supervision does not modify the negative impact of conflict on that quality of client–provider interactions in ANC. This finding aligns with studies that show that supervision has not meaningfully improved the quality of obstetric or sick childcare in African countries (45).
There are clear policy implications of our findings. Even in the absence of the destruction of equipment and infrastructure, following conflict, the quality of maternal health care may be negatively affected, as in Kenya. Hence, facilities with conflict events within their catchment area in Kenya should be targeted for support to improve client–provider interactions. While the impact of conflict does not vary with variation in management supervision in our sample, several critical components of supportive supervision were missing. For example, over 50% of facilities do not report an external supervisor visit in the past 6 months, and in 43% of cases, a supervisor does not observe actual service provision. These represent missed opportunities to detect and address gaps in service delivery interactions. Hence, it may be useful to explore if further improvements in supportive supervision increase the resilience of maternal health care delivery to conflict shocks. Future research may explore a potential role for other factors, including changes in provider motivation, in modulating the impact of conflict on process quality in Kenya (15).
Our study has limitations. We provide evidence in support of the assumption that there are no significant differences among exposed and unexposed facilities in the absence of conflict. However, in practice, this assumption cannot be definitively proven to hold. We demonstrate that our findings are robust to adjustment for confounding and variation in the definition of conflict and conflict exposure. The controls adjusted for in our models were constrained by data collected through the surveys. Hence, we were unable to adjust for some time-varying confounders of maternal health care utilization and quality, such as socioeconomic status, maternal age, parity, and decision-making autonomy. As we adjusted for client education, we consider this a reasonable proxy for some of the information that is unavailable, including socioeconomic status and decision-making autonomy. Given data limitations, we are also unable to explore the role of provider competence, intrinsic motivation, and external incentives in modifying the impact of conflict on process quality. Future studies would benefit from the use of primary data similar to the study by Akseer et al. Our study uses the latest SPA survey, conducted in 2010, highlighting the urgent need for investments in data on the quality of care. To explore the generalizability of our findings over time, future studies can explore if the impact of conflict on ANC quality has remained stable over time. Finally, our study was also underpowered to examine the impact of specific types of conflict events, such as protests or battles, on the quality of health care.
Despite these limitations, this study contributes to the emerging literature on the impact of conflict on the quality of maternal health care. Kenya is not classified by the World Bank Group as a fragile and conflict-affected state, due to a relatively strong institutional environment and the absence of a peace-keeping mission in recent years. Our findings buttress the fact that countries that do not meet this criterion may also experience negative impacts of conflict on health service delivery. Hence, the World Bank has acknowledged that the definition of a fragile and conflict-affected state may not take full account of contextual challenges, including fragilities in middle-income countries and the spatial dynamics of conflict (46). Given our findings, further research and policy that aims to improve the quality of care in facilities exposed to conflict in Kenya should focus on better understanding the determinants of the gaps in process quality, including provider motivation, competence, and incentives.
Publicly available datasets were analyzed in this study. This data can be found at: https://dhsprogram.com/data/available-datasets.cfm; https://acleddata.com/curated-data-files/; http://www.fao.org/geonetwork/srv/en/main.home (accessed February 16, 2021).
This study involved a secondary analysis of anonymous data, collected as part of research protocols with ethical approvals obtained through the Demographic and Health Survey Program and the ACLED Project.
AC conceptualized the study. KW undertook the spatial and statistical analysis. AC, KW, and UE-M contributed to a review of the study methodology, interpretation of the results, drafting of the manuscript, and revisions of the intellectual content of the manuscript. All authors read and approved the final manuscript.
The motivations, findings, interpretations, and conclusions expressed in this work do not necessarily reflect the views of the World Bank, its Board of Executive Directors, or the governments they represent. The World Bank does not guarantee the accuracy of the data included in this work.
The authors declare that the research was conducted in the absence of any commercial or financial relationships that could be construed as a potential conflict of interest.
1. WHO, UNICEF, UNFPA and The World Bank. Trends in Maternal Mortality: 2000 to 2017 WHO. Geneva: WHO (2019).
2. O'hare BAM, and David PS. First do no harm: the impact of recent armed conflict on maternal and child health in Sub-Saharan Africa. J R Soc Med. (2007)100:564–70. doi: 10.1258/jrsm.100.12.564
3. Institute of Medicine (US) Committee on Improving Birth Outcomes;, Bale JR, Stoll BJ, and Lucas AO. Improving Birth Outcomes: Meeting the Challenge in the Developing World. Washington, DC: National Academies Press (US); Reducing Maternal Mortality and Morbidity. (2003). Available online at: https://www.ncbi.nlm.nih.gov/books/NBK222105/
4. Weil O, and Fernandez H. Is safe motherhood an orphan initiative? Lancet. (1999) 354:940–3. doi: 10.1016/S0140-6736(99)02369-7
5. Yaya S, and Ghose B. Global inequality in maternal health care service utilization: implications for sustainable development goals. Health Equity. (2019) 3:145–54. doi: 10.1089/heq.2018.0082
6. Leslie HH, Malata A, Ndiaye Y, and Kruk N. Effective coverage of primary care services in eight high-mortality countries. BMJ Global Health. (2017) 2:e000424. doi: 10.1136/bmjgh-2017-000424
7. Chukwuma A, Wosu AC, Mbachu C, and Weze K. Quality of antenatal care predicts retention in skilled birth attendance: a multilevel analysis of 28 African countries. BMC Pregnancy Childbirth. (2017) 17:152. doi: 10.1186/s12884-017-1337-1
8. Powell-Jackson T, Mazumdar S, and Mills A. Financial incentives in health: new evidence from India's Janani Suraksha Yojana. J Health Econ. (2015) 43:154–69. doi: 10.1016/j.jhealeco.2015.07.001
9. Chaturvedi S, Randive B, Diwan V, and De Costa A. Quality of obstetric referral services in India's JSY cash transfer programme for institutional births: a study from Madhya Pradesh province. PLoS ONE. (2014) 9:e96773. doi: 10.1371/journal.pone.0096773
10. Nagpal J, Sachdeva A, Sengupta Dhar R, Bhargava VL, and Bhartia A. Widespread non-adherence to evidence-based maternity care guidelines: a population-based cluster randomized household survey. BJOG. (2015) 122:238–47. doi: 10.1111/1471-0528.13054
11. Institute of Medicine. Crossing the Quality Chasm: A New Health System for the 21st century. Washington, DC:National Academy Press (2001). Available online at: http://www.nationalacademies.org/hmd/Reports/2001/Crossing-the-Quality-Chasm-A-New-Health-System-for-the-21st-Century.aspx
12. Donabedian A. Evaluating the quality of medical care. Milbank Quart. (2005) 83:691–729. doi: 10.1111/j.1468-0009.2005.00397.x
13. Delivering Quality Health Services: A Global Imperative for Universal Health Coverage. Geneva: World Health Organization; Organization for Economic Co-operation and Development; The World Bank (2018).
14. Kak N, Burkhalter B, and Cooper M. Measuring the Competence of Healthcare Providers. Bethesda, MD: U.S. Agency for International Development (USAID) by the Quality Assurance (QA) Project. Operations Research Issue Paper (2001).
15. Franco LM, Bennett S, and Kanfer R. Health sector reform and public sector health worker motivation: a conceptual framework. Soc Sci Med. (2002) 54:1255–66. doi: 10.1016/S0277-9536(01)00094-6
16. Wiig S, Aase K, von Plessen C, Burnett S, Nunes F, Weggelaar AM, et al. Talking about quality: exploring how ‘quality' is conceptualized in European hospitals and healthcare systems. BMC Health Serv Res. (2014) 14:478. doi: 10.1186/1472-6963-14-478
17. DeJong J, Ghattas H, Bashour H, Mourtada R, Akiki C, and Reese_Masterson A. Reproductive, maternal, neonatal and child health in conflict: a case study on Syria using countdown indicators. BMJ Global Health. (2017) 2:e000302. doi: 10.1136/bmjgh-2017-000302
18. Akseer N, Wright J, Tasic H, Everett K, Scudder E, Amsalu R, et al. Women, children and adolescents in conflict countries: an assessment of inequalities in intervention coverage and survival BMJ Global Health. (2020) 5:e002214. doi: 10.1136/bmjgh-2019-002214
19. Chukwuma A, and Ekhator-Mobayode UE. Armed conflict and maternal health care utilization: evidence from the Boko Haram insurgency in Nigeria. Soc Sci Med. (2019) 226:104–12. doi: 10.1016/j.socscimed.2019.02.055
20. Mullany LC, Lee CI, Yone L, Paw P, Oo EKS, Maung C, et al. Access to essential maternal health interventions and human rights violations among vulnerable communities in Eastern Burma. PLoS Med. (2008) 5:e242. doi: 10.1371/journal.pmed.0050242
21. Namasivayam Amrita González, Pedro Arcos, Delgado, Rafael Castro, Chi, and Primus Che. The effect of armed conflict on the utilization of maternal health services in Uganda: a population-based study. PLoS Curr Disast. (2017) 9:1689–98. doi: 10.1371/currents.dis.557b987d6519d8c7c96f2006ed3c271a
22. Kabakian-Khasholian Tamar Shayboub Rawan El-Kak Faysal. Seeking maternal care at times of conflict: the case of Lebanon. Health Care Women Int. (2013) 34:352–62. doi: 10.1080/07399332.2012.736570
23. Chi PC, Bulage P, Urdal H, and Sundby J. Perceptions of the effects of armed conflict on maternal and reproductive health services and outcomes in Burundi and Northern Uganda: a qualitative study. BMC Int Health Hum Rights. (2015) 15:7. doi: 10.1186/s12914-015-0045-z
24. McKay S. The effects of armed conflict on girls and women. Peace Conflict. (1998) 4:381–92. doi: 10.1207/s15327949pac0404_6
25. Fouad FM, Sparrow A, Tarakji A, Alameddine M, El-Jardali F, Coutts AP, et al. Health workers and the weaponisation of health care in Syria: a preliminary inquiry for the Lancet-American University of Beirut commission on Syria. Lancet. (2017) 390:2516–26. doi: 10.1016/S0140-6736(17)30741-9
26. Akseer N, Rizvi A, Bhatti Z, Das JK, Everett K, Arur A, et al. Association of exposure to civil conflict with maternal resilience and maternal and child health and health system performance in afghanistan. JAMA Netw Open. (2019) 2:e1914819. doi: 10.1001/jamanetworkopen.2019.14819
27. National Coordinating Agency for Population and Development (NCAPD) [Kenya], Ministry of Medical Services (MOMS) [Kenya], Ministry of Public Health and Sanitation (MOPHS) [Kenya], Kenya National Bureau of Statistics (KNBS) [Kenya], ICF Macro. Kenya Service Provision Assessment Survey 2010. Nairobi: National Coordinating Agency for Population and Development, Ministry of Medical Services, Ministry of Public Health and Sanitation, Kenya National Bureau of Statistics, and ICF Macro (2011).
28. Raleigh C, Andrew L, Håvard H, and Joakim K. Introducing ACLED-armed conflict location and event data. J Peace Res. (2010) 47:651–60. doi: 10.1177/0022343310378914
29. Dowd C, and Raleigh C. ACLED Country Report. Kenya: ACLED (2013). Available online at: http://www.acleddata.com/wpcontent/uploads/2014/10/ACLED-Country-Report_Kenya_December-2013_updated.pdf
31. KNCHR. Are We Under Siege? The State of Security in Kenya: An Occasional Report (2010 – 2014) Nairobi: KNCHR (2014). Available online at: http://www.ke.undp.org/content/dam/kenya/docs/Democratic%20Governance/State%20of%20Security%20in%20Kenya%20-Occassional%20Report.pdf
32. Achoki T, Miller-Petrie M, Glenn SD, Kalra N, Lesego A, Gathecha GK, et al. Health disparities across the counties of Kenya and implications for policy makers, 1990–2016: a systematic analysis for the Global Burden of Disease Study 2016. Lancet Global Health. (2019) 7:e81–95. doi: 10.1016/S2214-109X(18)30472-8
33. Nguhiu PK, Barasa EW, and Chuma J. Determining the effective coverage of maternal and child health services in Kenya, using demographic and health survey data sets: tracking progress towards universal health coverage. Trop Med Int Health. (2017) 22:442–53. doi: 10.1111/tmi.12841
34. Ruktanonchai C, Nilsen K, Alegana VA, Bosco C, Ayiko R, Seven Kajeguka AC, et al. Temporal trends in spatial inequalities of maternal and newborn health services among four east African countries, 1999–2015. BMC Public Health. (2018) 18:1339. doi: 10.1186/s12889-018-6241-8
35. Wairoto KG, Joseph NK, Macharia PM, and Okiro EA. Determinants of subnational disparities in antenatal care utilisation: a spatial analysis of demographic and health survey data in Kenya. BMC Health Serv Res. (2020) 20:665. doi: 10.1186/s12913-020-05531-9
36. Fotso JC, Ezeh A, and Oronje R. Provision and use of maternal health services among urban poor women in Kenya: what do we know and what can we do? J Urban Health. (2008) 85:428–42. doi: 10.1007/s11524-008-9263-1
37. Ziraba AK, Madise N, Mills S, Kyobutungi C, and Ezeh A. Maternal mortality in the informal settlements of Nairobi city: what do we know? Reprod Health. (2009) 6:6. doi: 10.1186/1742-4755-6-6
38. Kruk ME, Chukwuma A, Mbaruku G, and Leslie HH. Variation in quality of primary-care services in Kenya, Malawi, Namibia, Rwanda, Senegal, Uganda and the United Republic of Tanzania. Bull World Health Organ. (2017) 95:408–18. doi: 10.2471/BLT.16.175869
39. Food Agriculture Organization (FAO). Africover Multipurpose Land Cover Databases for Kenya. Rome: FAO (2000). Available online at: https://datasets.wri.org/dataset/urban-areas-in-kenya
40. Guenther T, Sadruddin S, Chimuna T, Sichamba B, Yeboah-Antwi K, Diakite B, et al. Beyond distance: an approach to measure effective access to case management for sick children in Africa. Am J Trop Med Hyg. (2012) 87(Suppl. 5):77–84. doi: 10.4269/ajtmh.2012.11-0747
41. Opportunities for Africa's Newborns: Practical Data Policy and Programmatic Support for Newborn Care in Africa. Geneva: World Health Organization; for the Partnership for Maternal Newborn and Child Health (2006).
42. Raleigh C, Linke A, Hegre H, and Karlsen J. Introducing ACLED-armed conflict location and event data. J Peace Res. (2010) 47:651–60. Retrieved from http://www.acleddata.com/data/realtime-data
43. Netherlands Enterprise Agency. Kenyan Healthcare Sector: Opportunities for the Dutch & Health Sector. (2016). Available online at: https://www.rvo.nl/sites/default/files/2016/10/2016_Kenyan_Healthcare_Sector_Report_Compleet.pdf
44. Gilson L, Barasa E, Nxumalo N, Cleary S, Goudge J, Molyneux S, et al. Everyday resilience in district health systems: emerging insights from the front lines in Kenya and South Africa. BMJ Global Health. (2017) 2:e000224. doi: 10.1136/bmjgh-2016-000224
45. Leslie HH, Gage A, Nsona H, Hirschhorn LR, and Kruk ME. Training and supervision did not meaningfully improve quality of care for pregnant women or sick children in sub-Saharan Africa. Health Affairs. (2016) 35:1716–24. doi: 10.1377/hlthaff.2016.0261
Keywords: antenatal care, conflict, violence, quality of care, maternal health, Kenya
Citation: Chukwuma A, Wong KLM and Ekhator-Mobayode UE (2021) Disrupted Service Delivery? The Impact of Conflict on Antenatal Care Quality in Kenya. Front. Glob. Womens Health 2:599731. doi: 10.3389/fgwh.2021.599731
Received: 28 August 2020; Accepted: 28 January 2021;
Published: 26 February 2021.
Edited by:
Tabassum Firoz, Yale New Haven Health System, United StatesReviewed by:
Bilal Ahmed Usmani, NED University of Engineering and Technology, PakistanCopyright © 2021 Chukwuma, Wong and Ekhator-Mobayode. This is an open-access article distributed under the terms of the Creative Commons Attribution License (CC BY). The use, distribution or reproduction in other forums is permitted, provided the original author(s) and the copyright owner(s) are credited and that the original publication in this journal is cited, in accordance with accepted academic practice. No use, distribution or reproduction is permitted which does not comply with these terms.
*Correspondence: Adanna Chukwuma, YWNodWt3dW1hQHdvcmxkYmFuay5vcmc=
Disclaimer: All claims expressed in this article are solely those of the authors and do not necessarily represent those of their affiliated organizations, or those of the publisher, the editors and the reviewers. Any product that may be evaluated in this article or claim that may be made by its manufacturer is not guaranteed or endorsed by the publisher.
Research integrity at Frontiers
Learn more about the work of our research integrity team to safeguard the quality of each article we publish.