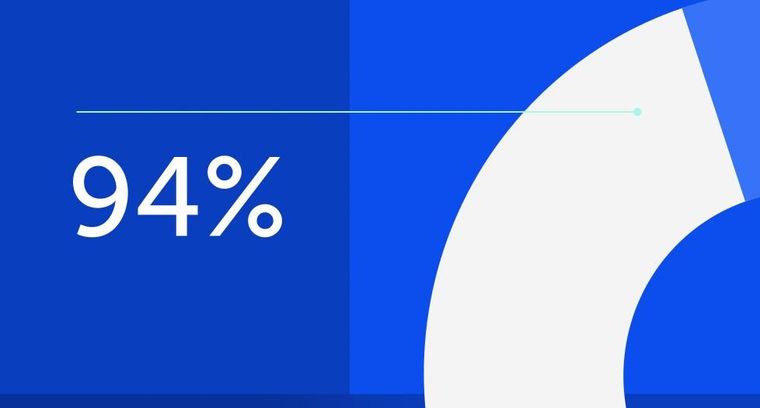
94% of researchers rate our articles as excellent or good
Learn more about the work of our research integrity team to safeguard the quality of each article we publish.
Find out more
ORIGINAL RESEARCH article
Front. Genet.
Sec. Computational Genomics
Volume 16 - 2025 | doi: 10.3389/fgene.2025.1583081
This article is part of the Research TopicLung Adenocarcinoma: From Genomics to Immunotherapy, Volume IIView all 13 articles
The final, formatted version of the article will be published soon.
Select one of your emails
You have multiple emails registered with Frontiers:
Notify me on publication
Please enter your email address:
If you already have an account, please login
You don't have a Frontiers account ? You can register here
Background and Objective: Gene expression analysis plays a critical role in lung cancer research, offering molecular feature-based diagnostic insights that are particularly effective in distinguishing lung cancer subtypes. However, the high dimensionality and inherent imbalance of gene expression data create significant challenges for accurate diagnosis. This study aims to address these challenges by proposing an innovative deep learning-based method for predicting lung cancer subtypes. Methods: We propose a method called Exo-LCClassifier, which integrates feature selection, one-dimensional convolutional neural networks (1D CNN), and an improved Wasserstein Generative Adversarial Network (WGAN). First, differential gene expression analysis was performed using DESeq2 to identify significantly expressed genes from both normal and tumor tissues. Next, the enhanced WGAN was applied to augment the dataset, addressing the issue of sample imbalance and increasing the diversity of effective samples. Finally, a 1D CNN was used to classify the balanced dataset, thereby improving the model's diagnostic accuracy.Results: The proposed method was evaluated using five-fold cross-validation, achieving an average accuracy of 0.9766 ± 0.0070, precision of 0.9762 ± 0.0101, recall of 0.9827 ± 0.0050, and F1-score of 0.9793 ± 0.0068. On an external GEO lung cancer dataset, it also showed strong performance with an accuracy of 0.9588, precision of 0.9558, recall of 0.9678, and F1-score of 0.9616. Conclusion: This study addresses the critical challenge of imbalanced learning in lung cancer gene expression analysis through an innovative computational framework. Our solution integrates three advanced techniques: (1) DESeq2 for differential expression analysis, (2) WGAN for data augmentation, and (3) 1D CNN for feature learning and classification. The source codes are publicly available at: https://github.com/lanlinxxs/Exo-classifier.
Keywords: lung cancer, Gene Expression, WGAN, imbalanced data, DESeq2, 1D CNN
Received: 27 Feb 2025; Accepted: 14 Apr 2025.
Copyright: © 2025 Zhan, Yu, Liu, Qin and Guo. This is an open-access article distributed under the terms of the Creative Commons Attribution License (CC BY). The use, distribution or reproduction in other forums is permitted, provided the original author(s) or licensor are credited and that the original publication in this journal is cited, in accordance with accepted academic practice. No use, distribution or reproduction is permitted which does not comply with these terms.
* Correspondence: Lu Guo, University of Electronic Science and Technology of China, Chengdu, China
Disclaimer: All claims expressed in this article are solely those of the authors and do not necessarily represent those of their affiliated organizations, or those of the publisher, the editors and the reviewers. Any product that may be evaluated in this article or claim that may be made by its manufacturer is not guaranteed or endorsed by the publisher.
Supplementary Material
Research integrity at Frontiers
Learn more about the work of our research integrity team to safeguard the quality of each article we publish.