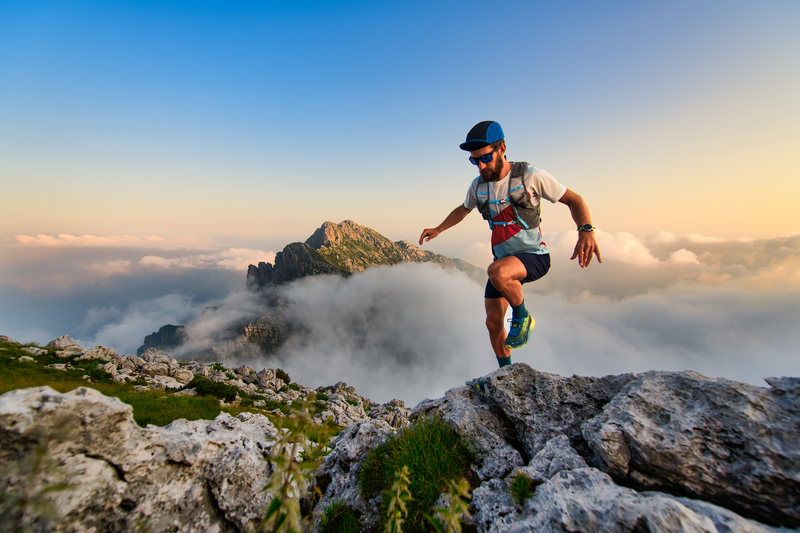
95% of researchers rate our articles as excellent or good
Learn more about the work of our research integrity team to safeguard the quality of each article we publish.
Find out more
ORIGINAL RESEARCH article
Front. Genet.
Sec. Computational Genomics
Volume 16 - 2025 | doi: 10.3389/fgene.2025.1580512
This article is part of the Research Topic Using various physical and genomics markers for smart therapy by expert systems with computer learning: Volume II View all articles
The final, formatted version of the article will be published soon.
You have multiple emails registered with Frontiers:
Please enter your email address:
If you already have an account, please login
You don't have a Frontiers account ? You can register here
In recent years, lots of computational models have been proposed to infer potential lncRNA-disease associations. In this manuscript, we introduced a novel end-to-end learning framework named CNMCLDA, in which, we first adopted two convolutional neural networks to extract hidden features of diseases and lncRNAs separately. And then, by combining these hidden features of diseases and lncRNAs with known lncRNA-disease associations, we designed 5 different loss functions. Next, based on errors obtained by these loss functions, we would perform back propagation to fit parameters in CNMCLDA, and complete those missing values in lncRNA-disease relational matrix according to these fitted parameters. In order to demonstrate the prediction performance of CNMCLDA,, intensive experiments have been carried out and experimental results show that CNMCLDA can achieve better performances than state-of-the-art competitive predictive models in frameworks of five-fold cross validation, ten-fold cross validation and leave-one-disease-out cross validation respectively. Moreover, in case studies of gastric cancer, glioma and breast cancer, there are 19, 17 and 16 out of top 20 candidate lncRNAs inferred by CNMCLDA having been confirmed by recent relevant literatures separately, which demonstrated the outstanding performance of CNMCLDA as well. Hence, it is obvious that CNMCLDA may be an effective tool for prediction of potential lncRNA-disease associations in the future.
Keywords: lncRNA-disease associations, computational model, Prediction model, Convolutional Neural Network, Negative samples
Received: 20 Feb 2025; Accepted: 31 Mar 2025.
Copyright: © 2025 Zhou, Wang, Chen, Le and Xu. This is an open-access article distributed under the terms of the Creative Commons Attribution License (CC BY). The use, distribution or reproduction in other forums is permitted, provided the original author(s) or licensor are credited and that the original publication in this journal is cited, in accordance with accepted academic practice. No use, distribution or reproduction is permitted which does not comply with these terms.
* Correspondence:
Lei Wang, Changsha University, Changsha, China
Sisi Chen, The First Hospital of Hunan University of Chinese Medicine, Changsha, Hunan, China
Jinhai Le, The First Hospital of Hunan University of Chinese Medicine, Changsha, Hunan, China
Disclaimer: All claims expressed in this article are solely those of the authors and do not necessarily represent those of their affiliated organizations, or those of the publisher, the editors and the reviewers. Any product that may be evaluated in this article or claim that may be made by its manufacturer is not guaranteed or endorsed by the publisher.
Research integrity at Frontiers
Learn more about the work of our research integrity team to safeguard the quality of each article we publish.