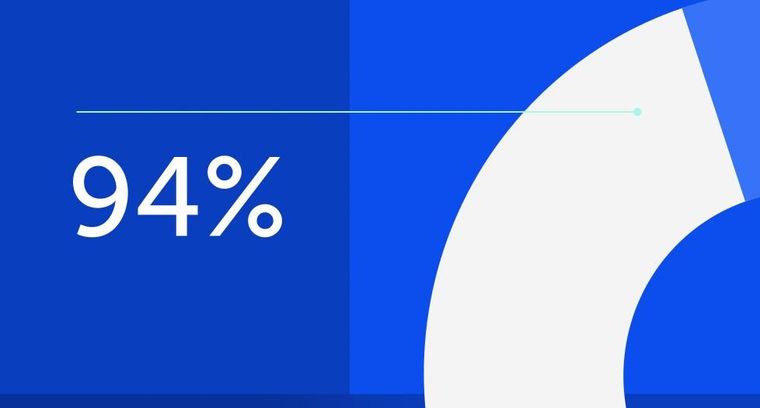
94% of researchers rate our articles as excellent or good
Learn more about the work of our research integrity team to safeguard the quality of each article we publish.
Find out more
ORIGINAL RESEARCH article
Front. Genet.
Sec. Statistical Genetics and Methodology
Volume 16 - 2025 | doi: 10.3389/fgene.2025.1568705
The final, formatted version of the article will be published soon.
Select one of your emails
You have multiple emails registered with Frontiers:
Notify me on publication
Please enter your email address:
If you already have an account, please login
You don't have a Frontiers account ? You can register here
The practical implementation of genomic selection (GS) in plant breeding experiments is challenging due to numerous factors that affect its reliability. To address these challenges, deep learning (DL) models have been adopted as a powerful framework to improve the accuracy of GS. To bolster the empirical evidence supporting DL models, we conducted a comparative study using 14 datasets from real plant breeding programs, comparing the genomic prediction performance of DL models with the best genomic best linear unbiased predictor (GBLUP) model. Our findings indicate that DL models are highly competitive and excel at capturing complex, non-linear patterns, even when working with small datasets like those used in this study. Nevertheless, the successful implementation of DL models requires meticulous parameter tuning to achieve optimal results. This comprehensive comparison highlights the complementary strengths of both DL and GBLUP models. It also underscores the importance of selecting models based on specific traits and prioritized metrics in predictive analyses, as neither DL nor GBLUP universally outperformed the other across all scenarios.
Keywords: Benchmarking, deep learning, GBLUP, genomic selection, plant breeding
Received: 30 Jan 2025; Accepted: 17 Apr 2025.
Copyright: © 2025 Montesinos-López, MONTESINOS-LOPEZ, Ramos-Pulido, Brandon, Guerrero-Arroyo, Crossa and Ortiz. This is an open-access article distributed under the terms of the Creative Commons Attribution License (CC BY). The use, distribution or reproduction in other forums is permitted, provided the original author(s) or licensor are credited and that the original publication in this journal is cited, in accordance with accepted academic practice. No use, distribution or reproduction is permitted which does not comply with these terms.
* Correspondence:
OSVAL A. MONTESINOS-LOPEZ, Facultad de Telemática, Universidad de Colima, Colima, 28040, Colima, Mexico
Rodomiro Ortiz, Swedish University of Agricultural Sciences, Uppsala, Sweden
Disclaimer: All claims expressed in this article are solely those of the authors and do not necessarily represent those of their affiliated organizations, or those of the publisher, the editors and the reviewers. Any product that may be evaluated in this article or claim that may be made by its manufacturer is not guaranteed or endorsed by the publisher.
Supplementary Material
Research integrity at Frontiers
Learn more about the work of our research integrity team to safeguard the quality of each article we publish.