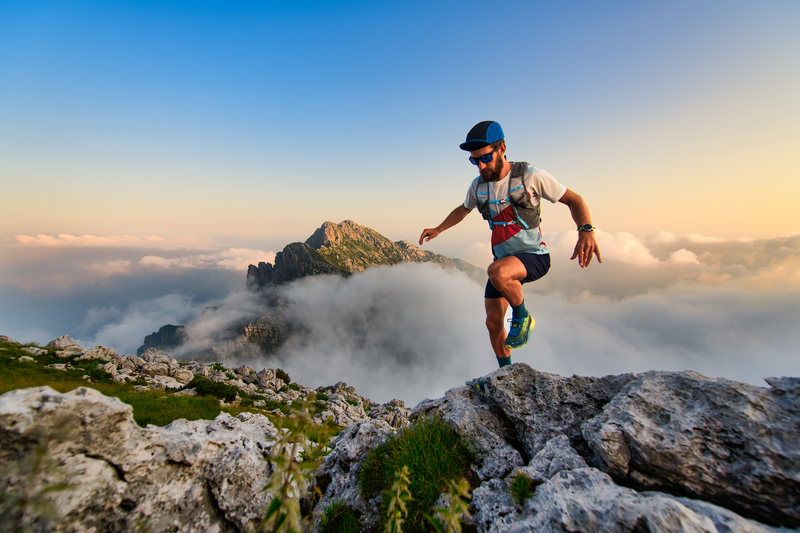
95% of researchers rate our articles as excellent or good
Learn more about the work of our research integrity team to safeguard the quality of each article we publish.
Find out more
ORIGINAL RESEARCH article
Front. Genet.
Sec. Computational Genomics
Volume 16 - 2025 | doi: 10.3389/fgene.2025.1568270
The final, formatted version of the article will be published soon.
You have multiple emails registered with Frontiers:
Please enter your email address:
If you already have an account, please login
You don't have a Frontiers account ? You can register here
The functionality of long noncoding RNAs (lncRNAs) is complex, which facilitates their interaction with a wide range of biological macromolecules, including microRNAs (miRNAs), proteins, genes, and cyclic RNAs, to regulate various physiological processes. These macromolecules are interconnected within a sophisticated regulatory network that is implicated in numerous diseases. The aim of the association prediction methodology, which leverages network analysis and deep learning, is to extract topological structural features from the network and to elucidate intricate associations within the regulatory relationship network, therefore enhancing the reliability of predictions. In this research, we introduce a graph convolutional network (GCN)-based approach to predict the disease relationships of lncRNAs, utilizing macromolecule-disease regulatory networks. Recognizing the collaborative role of miRNAs and lncRNAs in gene expression regulation, we developed a lncRNA-miRNA disease network. Additionally, to mitigate the challenges posed by isolated diseases and lncRNAs, we incorporate disease semantics and the similarity network of lncRNAs into a heterogeneous network framework. We present MVIGCN, an association prediction method for lncRNA-disease relationships that integrates multiview data sources and employs deep learning techniques with attention mechanisms.
Keywords: Graph convolutional network, lncRNA-miRNA, multiview data, deep learning, Similarity network
Received: 29 Jan 2025; Accepted: 04 Apr 2025.
Copyright: © 2025 Zhang, Zeng, Xiang, Zhao, Hu, Li, Zhu and Wang. This is an open-access article distributed under the terms of the Creative Commons Attribution License (CC BY). The use, distribution or reproduction in other forums is permitted, provided the original author(s) or licensor are credited and that the original publication in this journal is cited, in accordance with accepted academic practice. No use, distribution or reproduction is permitted which does not comply with these terms.
* Correspondence:
Lei Wang, Changsha University, Changsha, China
Disclaimer: All claims expressed in this article are solely those of the authors and do not necessarily represent those of their affiliated organizations, or those of the publisher, the editors and the reviewers. Any product that may be evaluated in this article or claim that may be made by its manufacturer is not guaranteed or endorsed by the publisher.
Research integrity at Frontiers
Learn more about the work of our research integrity team to safeguard the quality of each article we publish.