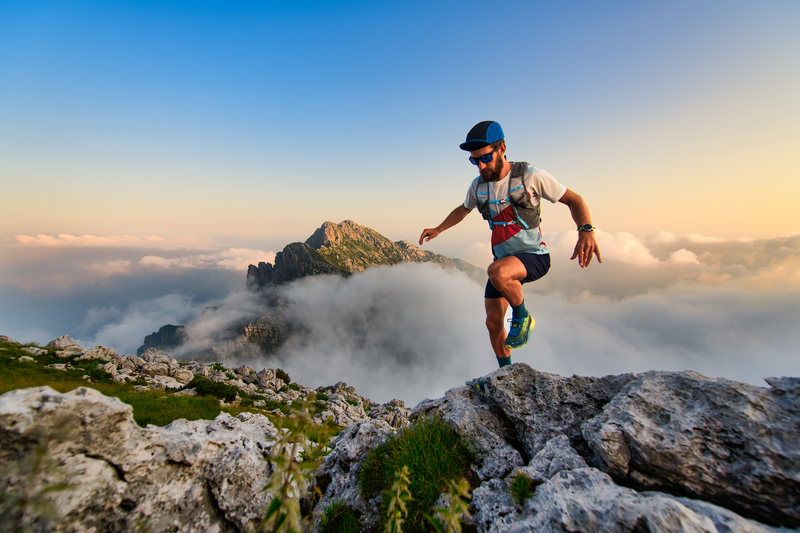
94% of researchers rate our articles as excellent or good
Learn more about the work of our research integrity team to safeguard the quality of each article we publish.
Find out more
ORIGINAL RESEARCH article
Front. Genet.
Sec. Cancer Genetics and Oncogenomics
Volume 16 - 2025 | doi: 10.3389/fgene.2025.1556519
The final, formatted version of the article will be published soon.
You have multiple emails registered with Frontiers:
Please enter your email address:
If you already have an account, please login
You don't have a Frontiers account ? You can register here
The identification of uncommon neutrophilic leukemias presents a challenging task, owing to the analogous morphological characteristics and the dearth of molecular markers.Transcriptomic profile of bone marrow cells in this disease subset has been rarely explored.The OHSU-CNL dataset, encompassing clinical parameters and parallel transcriptomic matrix, was downloaded from the GDC database. Utilizing R software, distinctive coexpressed gene modules and pivotal genes for CNL were identified. Subsequently, a diagnostic model for CNL, denoted as CNL-5, was formulated employing LASSO regression analysis. Diagnostic power of the CNL-5 model was compared with conventional clinical/genetic markers via multi-ROC analysis.Divergence of overall survival between CNL-5 risk groups was delineated by Kaplan-Meier analysis, predictive power (AUC and Harrison's C index) was obtained by time dependent ROC as well.Moreover, cell signaling pathways associating with CNL-5 risk were identified by GESA.Neither clinical indicators nor genetic markers were sufficient to classify neutrophilic leukemias. Through WGCNA, the 'brown' module was discerned to be CNL-specific (p = 8e-16, R² = 0.5). Via LASSO analysis, CNL-5 model, with risk scores based on weighted expression value of five genes (PDCD7/CR2/ZSCAN20/TRIM68/LILRA6), dichotomized patients into "CNL-like" and "Atypical-CNL" groups. Compared to Atypical-CNL group, CNL-like group demonstrated a clinical phenotype more consistent with CNL and had a significantly higher prevalence of CSF3R mutations (p < 0.05). Additionally, AUC of the CNL-5 risk model surpassed that of conventional clinical/genetic markers, which was validated by GSE42731 dataset. Poorer survival was revealed in high-risk group than that of low-risk group defined by CNL-5 model. GSEA identified CNL-5-associated pathways, including the inhibition of oxidative phosphorylation, and the activation of IL6-JAK-STAT3 signaling, etc.A novel diagnostic assessment for CNL was developed according to expression signature, exhibiting superior diagnostic utility compared to conventional indicators.
Keywords: Chronic neutrophilic leukemia, WGCNA, Neutrophil, Expression, LASSO
Received: 08 Jan 2025; Accepted: 20 Feb 2025.
Copyright: © 2025 Guo and Li. This is an open-access article distributed under the terms of the Creative Commons Attribution License (CC BY). The use, distribution or reproduction in other forums is permitted, provided the original author(s) or licensor are credited and that the original publication in this journal is cited, in accordance with accepted academic practice. No use, distribution or reproduction is permitted which does not comply with these terms.
* Correspondence:
Chao Guo, China-Japan Friendship Hospital, Beijing, China
Disclaimer: All claims expressed in this article are solely those of the authors and do not necessarily represent those of their affiliated organizations, or those of the publisher, the editors and the reviewers. Any product that may be evaluated in this article or claim that may be made by its manufacturer is not guaranteed or endorsed by the publisher.
Research integrity at Frontiers
Learn more about the work of our research integrity team to safeguard the quality of each article we publish.