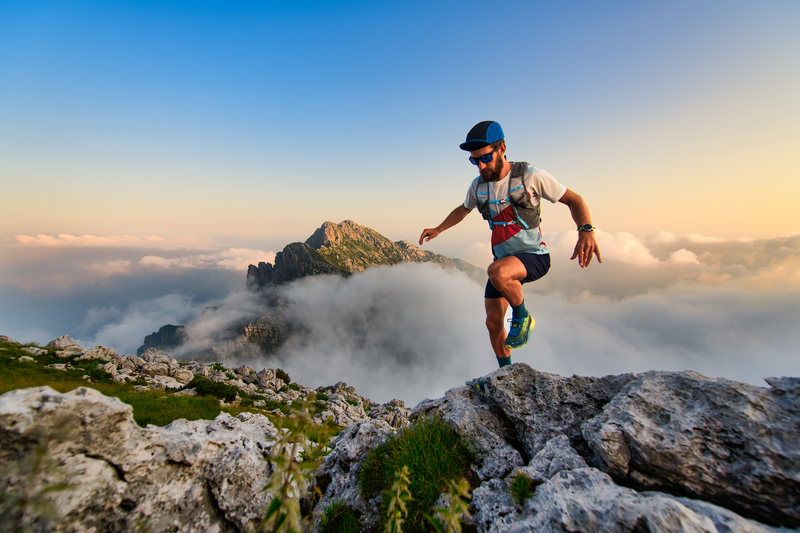
94% of researchers rate our articles as excellent or good
Learn more about the work of our research integrity team to safeguard the quality of each article we publish.
Find out more
ORIGINAL RESEARCH article
Front. Genet.
Sec. Genetics of Common and Rare Diseases
Volume 16 - 2025 | doi: 10.3389/fgene.2025.1542840
The final, formatted version of the article will be published soon.
You have multiple emails registered with Frontiers:
Please enter your email address:
If you already have an account, please login
You don't have a Frontiers account ? You can register here
Background: One of the most prevalent urinary illnesses is kidney stone formation, often known as nephrolithiasis. The precise processes of kidney stone remain poorly known after substantial investigation. In order to successfully prevent and cure stone formation and recurrence, additional research into the pathophysiology of stone formation is of paramount importance. Ferroptosis is linked to a variety of renal diseases and is a critical factor in the death of cells. However, little is known about how ferroptosis-related genes (FRGs) contribute to the development of kidney stones.The Ferroptosis Database and the Gene Expression Omnibus (GEO) database provided us with information on kidney stones and FRGs, respectively (FerrDb).Results: Eight DE-FRGs related to kidney stones were found in total, and they were all closely related to immune response and autophagy management. Following this, among the 8 DE-FRGs, LASSO and SVM-RFE algorithms chose FZD7, STK11, SUV39H1, and LCN2 as marker genes with suitable diagnostic capabilities. These marker genes may be involved in the control of the PPAR signaling pathway, mTOR signaling system, and fatty acid production of kidney stones, according to the functional enrichment analysis that followed. Additionally, 24 drugs that target two marker genes have been found. Despite this, the ceRNA networks have gained that the regulatory relationship between marker genes is rather complex. Additionally, the findings of the CIBERSORT investigation indicated that FZD7 and SUV39H1 may be linked to variations in the immune milieu of people who have kidney stones.We developed a diagnostic tool and provided information on the development of kidney stones. In order to confirm its diagnostic applicability for kidney stones, more studies are needed before it may be used in clinical practice.
Keywords: bioinformatics, Kidney stone, diagnostic biomarkers, ferroptosis, immune microenvironment
Received: 11 Dec 2024; Accepted: 24 Feb 2025.
Copyright: © 2025 He, Song, Wang, Dong, Jiang, Yu and Shan. This is an open-access article distributed under the terms of the Creative Commons Attribution License (CC BY). The use, distribution or reproduction in other forums is permitted, provided the original author(s) or licensor are credited and that the original publication in this journal is cited, in accordance with accepted academic practice. No use, distribution or reproduction is permitted which does not comply with these terms.
* Correspondence:
Xi Yu, Renmin Hospital of Wuhan University, Wuhan, 430060, Hubei Province, China
Disclaimer: All claims expressed in this article are solely those of the authors and do not necessarily represent those of their affiliated organizations, or those of the publisher, the editors and the reviewers. Any product that may be evaluated in this article or claim that may be made by its manufacturer is not guaranteed or endorsed by the publisher.
Research integrity at Frontiers
Learn more about the work of our research integrity team to safeguard the quality of each article we publish.