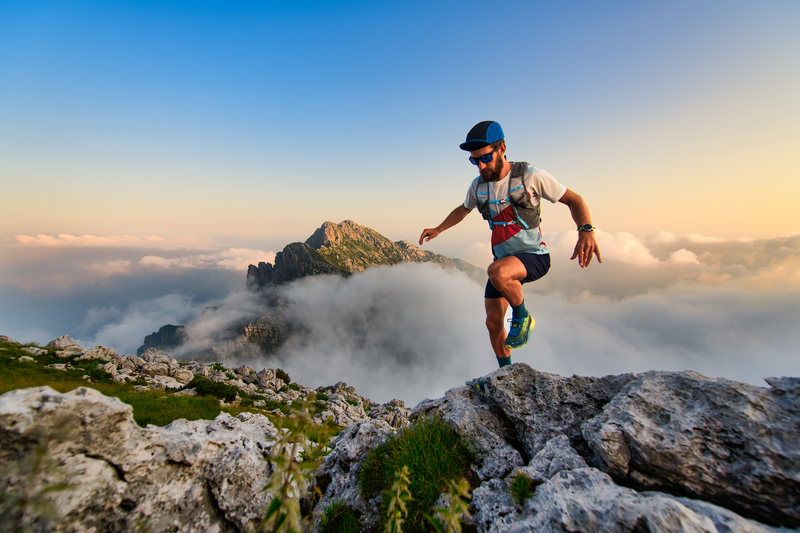
94% of researchers rate our articles as excellent or good
Learn more about the work of our research integrity team to safeguard the quality of each article we publish.
Find out more
ORIGINAL RESEARCH article
Front. Genet.
Sec. Livestock Genomics
Volume 16 - 2025 | doi: 10.3389/fgene.2025.1535730
This article is part of the Research Topic Insights in Livestock Genomics View all 5 articles
The final, formatted version of the article will be published soon.
You have multiple emails registered with Frontiers:
Please enter your email address:
If you already have an account, please login
You don't have a Frontiers account ? You can register here
The genetic background and characteristics of South African smallholder cattle populations remain largely unknown. These cattle exhibit remarkable adaptability to challenging environments with minimal inputs from farmers, making them a valuable genetic resource for sustainable farming. This study aimed to genetically characterize non-descript cattle kept in smallholding systems using singlenucleotide polymorphism (SNP) markers. A total of 188 non-descript smallholder beef cattle were sampled from seven South African provinces; Eastern Cape (n=27), Free State (n=28), Gauteng (n=13), KwaZulu-Natal (n=29), Limpopo (n=34), North West (n=44) and Northern Cape (n=10). In addition, samples were obtained from Afrikaner (n= 42), Bonsmara (BON; n=46), Boran (n=20), Brahman (n=96), Drakensberger (n= 25), Hereford (n=31), Holstein (HOL; n=29), Nguni (n=59) and Shorthorn (n=35) to serve as reference populations. Quality control of the original SNP data removed less informative animals and SNPs, which resulted in a final data set consisting of 185 animals and 119,392 SNPs. Principal coordinate analysis, ancestry, and genomic diversity statistics revealed moderate to high levels of diversity within smallholder cattle and substantial relationship with commercial beef cattle (i.e. Afrikaner, Bonsmara, Brahman, Drakensberger, Hereford, Holstein and Nguni). In NW province, there was tendency towards greater influence of Bonsmara, whereas in KZN the cattle were more closely related to Holstein. The smallholder populations were shown not to be unique, likely due to indiscriminate hybridization with the commercial breeds. Among the provinces, estimates of observed heterozygosity (HO) ranged from 0.328±0.001 to 0.395±0.001, while expected heterozygosity (HE) ranged from 0.326±0.001 to 0.389±0.000.Inbreeding levels were low, with (mean±standard error) per-province inbreeding coefficients ranging from -0.023±0.009 to 0.133±0.0254. The low FROH (<0.05) across all populations indicate a more diverse population, which is less likely to express deleterious recessive traits. Estimates of the population differentiation fixation index (FST) indicated greater genetic distance between animals from KZN and GP provinces (0.083) and less distance between the animals from EC and Free State provinces ( 0.010), suggesting a closer genetic relationship probably as a result of the proximity of the latter provinces and hence trans-boundary use of bulls.These findings suggest indiscriminate crossbreeding in smallholder cattle within and across the provinces of South Africa.
Keywords: Adaptability, Crossbreeding, Genetic characterization, Non-descript, Singlenucleotide polymorphism
Received: 27 Nov 2024; Accepted: 17 Feb 2025.
Copyright: © 2025 Ramoroka, MacNeil, Neser, Lashmar and Makgahlela. This is an open-access article distributed under the terms of the Creative Commons Attribution License (CC BY). The use, distribution or reproduction in other forums is permitted, provided the original author(s) or licensor are credited and that the original publication in this journal is cited, in accordance with accepted academic practice. No use, distribution or reproduction is permitted which does not comply with these terms.
* Correspondence:
M P Ramoroka, Agricultural Research Council of South Africa (ARC-SA), Pretoria, South Africa
Disclaimer: All claims expressed in this article are solely those of the authors and do not necessarily represent those of their affiliated organizations, or those of the publisher, the editors and the reviewers. Any product that may be evaluated in this article or claim that may be made by its manufacturer is not guaranteed or endorsed by the publisher.
Research integrity at Frontiers
Learn more about the work of our research integrity team to safeguard the quality of each article we publish.