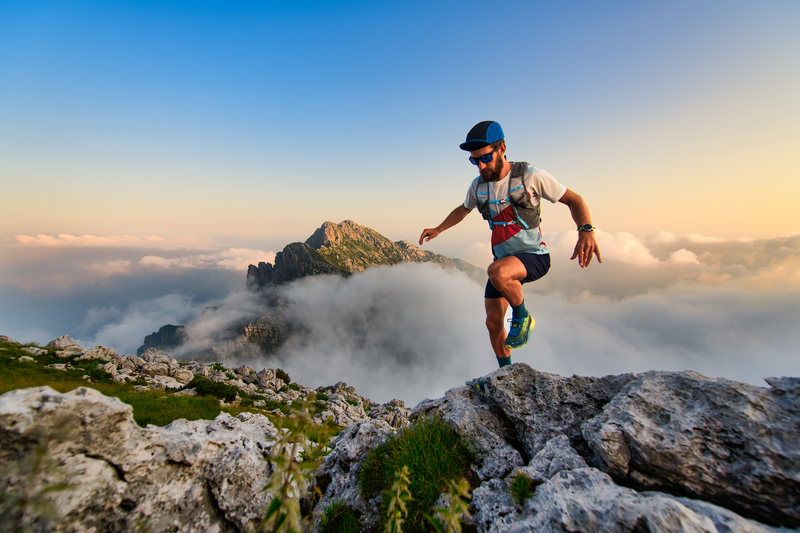
95% of researchers rate our articles as excellent or good
Learn more about the work of our research integrity team to safeguard the quality of each article we publish.
Find out more
ORIGINAL RESEARCH article
Front. Genet.
Sec. Statistical Genetics and Methodology
Volume 16 - 2025 | doi: 10.3389/fgene.2025.1535541
This article is part of the Research Topic Exploring the Molecular Mechanisms of Immune Related Diseases Through Multi-Omics Studies View all articles
The final, formatted version of the article will be published soon.
You have multiple emails registered with Frontiers:
Please enter your email address:
If you already have an account, please login
You don't have a Frontiers account ? You can register here
Objective: Fibromyalgia (FM) is a complex autoimmune disorder characterized by widespread pain and fatigue, with significant diagnostic challenges due to the absence of specific biomarkers. This study aims to identify and validate potential genetic markers for FM to facilitate earlier diagnosis and intervention.Methods: We analyzed gene expression data from the Gene Expression Omnibus (GEO) to identify differentially expressed genes (DEGs) associated with FM. Comprehensive enrichment analyses, including GO, KEGG, and Reactome pathways, were performed to elucidate the biological functions and disease associations of the candidate genes. We employed the eXtreme Gradient Boosting (XGBoost) algorithm to develop a diagnostic model, which was validated using independent datasets.Results: Three genes, Dual Specificity Tyrosine Phosphorylation Regulated Kinase 3 (DYRK3), Regulator Of G Protein Signaling 17 (RGS17), and Rho Guanine Nucleotide Exchange Factor 37 (ARHGEF37), were identified as key biomarkers for FM. These genes are implicated in critical processes such as ion homeostasis, cell signaling, and neurobiological functions, which are perturbed in FM. The diagnostic model demonstrated robust performance, with an Area Under the Curve (AUC) of 0.8338 in the training set and 0.8178 in the validation set, indicating its potential utility in clinical settings.Conclusion: The study successfully identifies three diagnostic biomarkers for FM, supported by both bioinformatics analysis and machine learning models. These findings could significantly improve the diagnostic accuracy for FM, leading to better patient management and treatment outcomes.
Keywords: Fibromyalgia, Diagnostic Markers, DYRK3, RGS17, ArhGEF37
Received: 27 Nov 2024; Accepted: 31 Mar 2025.
Copyright: © 2025 Zhao, Zhao, Li, Song, Cheng, Li, Wu, He, Jiao and Chang. This is an open-access article distributed under the terms of the Creative Commons Attribution License (CC BY). The use, distribution or reproduction in other forums is permitted, provided the original author(s) or licensor are credited and that the original publication in this journal is cited, in accordance with accepted academic practice. No use, distribution or reproduction is permitted which does not comply with these terms.
* Correspondence:
Bingheng He, Tongren Hospital, School of Medicine, Shanghai Jiao Tong University, Shanghai, 200025, China
Juan Jiao, Guang’anmen Hospital, China Academy of Chinese Medical Sciences, Beijing, 100053, Beijing Municipality, China
Cen Chang, Department of Rheumatology, Shanghai Guanghua Hospital, Shanghai University of Traditional Chinese Medicine, Shanghai, China
Disclaimer: All claims expressed in this article are solely those of the authors and do not necessarily represent those of their affiliated organizations, or those of the publisher, the editors and the reviewers. Any product that may be evaluated in this article or claim that may be made by its manufacturer is not guaranteed or endorsed by the publisher.
Research integrity at Frontiers
Learn more about the work of our research integrity team to safeguard the quality of each article we publish.