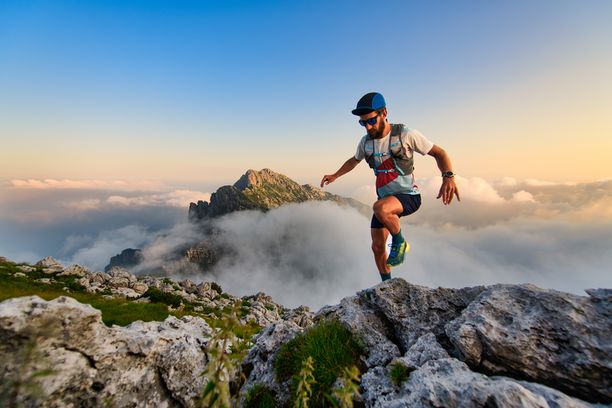
94% of researchers rate our articles as excellent or good
Learn more about the work of our research integrity team to safeguard the quality of each article we publish.
Find out more
ORIGINAL RESEARCH article
Front. Genet.
Sec. Cancer Genetics and Oncogenomics
Volume 16 - 2025 | doi: 10.3389/fgene.2025.1532523
This article is part of the Research TopicComparative Genomics and Functional Genomics Analyses in CancerView all 4 articles
The final, formatted version of the article will be published soon.
Select one of your emails
You have multiple emails registered with Frontiers:
Notify me on publication
Please enter your email address:
If you already have an account, please login
You don't have a Frontiers account ? You can register here
BACKGROUND: The role of immunogenic cell death (ICD) in cervical cancer (CESC) is not well understood. This study sought to investigate the significance of ICD in CESC and to establish an ICDRs prognostic model to improve immunotherapy efficacy for patients with cervical cancer.METHODS: ICD-associated genes were screened at the single-cell and transcriptome levels based on AddModuleScore, single-sample gene set enrichment analysis (ssGSEA) and weighted gene co-expression network (WGCNA) analysis. Immunogenic cell death-related features (ICDRs) were constructed using multiple machine algorithms, and ICDRs were evaluated in training and validation sets to provide quantitative tools for predicting prognosis in clinical practice. Predictive models were used to risk subgroups for response to immunotherapy, as well as drug sensitivity. Finally, the expression of ICD-related genes was verified by RT-qPCR.RESULTS: Through an integrated analysis of single-cell data, transcriptomic profiling, and computational modeling, seven ICD-related genes were identified as highly prognostic for CESC patients. Multivariate analysis demonstrated that low-risk patients had significantly better overall survival compared to high-risk patients, confirming the model as an independent prognostic tool. Assessments of the tumor microenvironment (TME), mutation characteristics, and drug sensitivity within ICDRs risk subgroups indicated a stronger immunotherapy response in the low-risk group.
Keywords: multi-omics, Single-cell RNA, ICDs, immune prognosis, Therapy efficacy, Algorithms
Received: 22 Nov 2024; Accepted: 25 Mar 2025.
Copyright: © 2025 Ning, Xiu, Xu, Si, Zhao and Ren. This is an open-access article distributed under the terms of the Creative Commons Attribution License (CC BY). The use, distribution or reproduction in other forums is permitted, provided the original author(s) or licensor are credited and that the original publication in this journal is cited, in accordance with accepted academic practice. No use, distribution or reproduction is permitted which does not comply with these terms.
* Correspondence: Qingling Ren, Affiliated Hospital of Nanjing University of Chinese Medicine, Nanjing, Liaoning Province, China
Disclaimer: All claims expressed in this article are solely those of the authors and do not necessarily represent those of their affiliated organizations, or those of the publisher, the editors and the reviewers. Any product that may be evaluated in this article or claim that may be made by its manufacturer is not guaranteed or endorsed by the publisher.
Supplementary Material
Research integrity at Frontiers
Learn more about the work of our research integrity team to safeguard the quality of each article we publish.