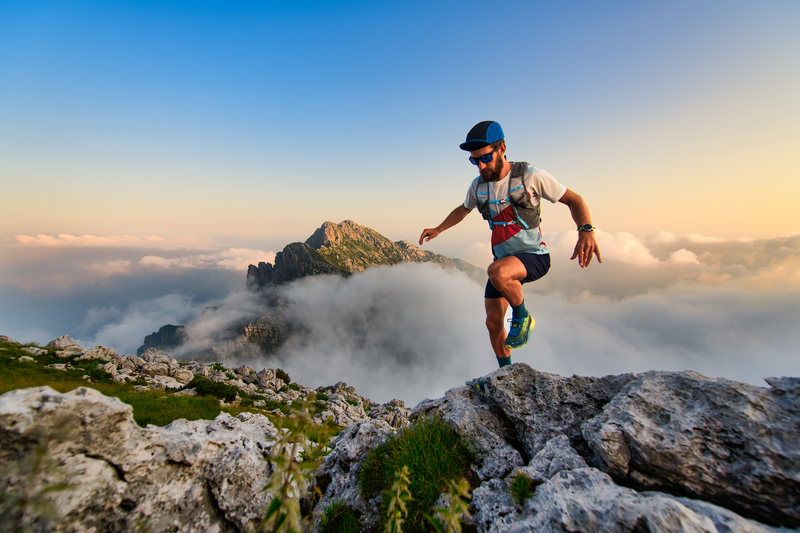
95% of researchers rate our articles as excellent or good
Learn more about the work of our research integrity team to safeguard the quality of each article we publish.
Find out more
ORIGINAL RESEARCH article
Front. Genet. , 24 March 2025
Sec. Cancer Genetics and Oncogenomics
Volume 16 - 2025 | https://doi.org/10.3389/fgene.2025.1530334
This article is part of the Research Topic Investigating Gene-Related Metabolic and Immunological Therapies in Cancer View all 4 articles
Catabolic metabolites of tryptophan (Trp) are considered to be important microenvironmental factors by suppressing anti-tumor immune responses in cancers. Nevertheless, the effect of Trp metabolism (Trp metabolism)-related genes Trp metabolism-related genes on laryngeal squamous cell carcinoma (LSCC) progression is not yet clear. So, in this study, the TCGA-LSCC, GSE27020, and 40 TMRGs were extracted via public databases to explore the effects of TMRGs on laryngeal squamous cell carcinoma. Firstly, Weighted Gene Co-expression Network Analysis (WGCNA) was adopted with LSCC samples in TCGA-LSCC to acquire key module, and differentially expressed genes between LSCC and normal samples from TCGA-LSCC were yielded via differential expression analysis. Next, differentially expressed TMRGs (DE-TMRGs) was obtained in key model and DEGs, and prognostic genes were identifde through multiple algorithms. Five prognostic genes, namely SERPINA1, TMC8, RENBP, SDS and FAM107A were finally identified. A risk model was established based on the expressions of prognostic genes and survival information of LSCC samples while that were divided into high and low risk groups. Obviously, the LSCC immune dysfunction and exclusion score of high-risk patients was dramatically higher than that in low-risk patients, indicating that patients in the high-risk subgroup exhibited reduced responsiveness to immunotherapy. Besides, the drug sensitivity analysis showed that the low -risk subgroup was notably sensitive to Salubrinal, Lenalidomide, Metformin, while high -risk subgroup was more responsive to Docetaxel, AUY922, Embelin. Eventually, two clusters of LSCC samples had notable correlations with LSCC prognosis. The above results indicated that the risk model consisted of TMRGs (SERPINA1, TMC8, RENBP, SDS and FAM107A) was constructed in LSCC, contributing to studies related to the prognosis and treatment of LSCC.
Laryngeal cancer (LCa) is the second most prevalent malignant tumor affecting the head and neck region, with an incidence rate second only to oral carcinoma, accounting for about 1.98% of all malignancies in the whole body, and in 2020 there was about 180,000 new patients with LCa and nearly 100,000 deaths worldwide (Sung et al., 2021; Atef et al., 2019). Squamous cell carcinoma (SCC) accounting for about 90% of LCa, is the predominant LCa subtype (Megwalu and Sikora, 2014; Siegel et al., 2020). The pathogenesis of laryngeal squamous cell carcinoma (LSCC) is still unclear, and related studies have shown that smoking, drinking, environmental factors, gastroesophageal reflux, HPV infection, radiation and other factors are associated with LSCC, which may be the result of a multifactorial interaction (Steuer et al., 2017). With the development of endoscopic and imaging technologies, the diagnosis and treatment of LSCC have been improved significantly. However, due to the lack of typical symptoms in the initial phase, approximately 60% of patients diagnosed with LSCC are already in advanced stages of tumor development. This delay in diagnosis hinders timely treatment and subsequently leads to unfavorable clinical outcomes (Strojan et al., 2013; Calhoun, 1988; Gao et al., 2019). In addition, despite the continuous improvement in surgery, chemotherapy and radiotherapy, the recurrence and metastasis rates of LSCC are still high and impose a serious impact on prognosis (Haddad et al., 2018; Gao et al., 2020; Bray et al., 2018; Lu et al., 2018; Nocini et al., 2020). In recent years, it has been pointed out that a possible way to improve the diagnostic and therapeutic effects of LSCC is the use of effective biomarkers, especially amino acid metabolic-related markers, which play an increasingly important role in the early detection of malignancies and the identification of potential immunotherapeutic targets (Zhang et al., 2018; Liu et al., 2023), but have not yet been well explored in LSCC. Therefore, the identification of effective biomarkers is important for the prediction of prognosis and guidance of personalized therapy in LSCC patients.
Tryptophan (Trp) is a crucial amino acid, constituting approximately 1% of the overall amino acids. It is not biosynthesized within our body and can solely be acquired through dietary intake (Wang et al., 2015). In the body, Trp is metabolized mainly through the Kynurenine pathway (KP) to produce downstream metabolites such as Kynurenine (KYN). Indoleamine 2,3-dioxygenase 1 (IDO1), indoleamine 2,3-dioxygenase 2 (IDO2), and tryptophan 2,3-dioxygenase 2 (TDO2) are the key rate-limiting enzymes for Trp metabolism. Numerous research studies have consistently demonstrated the significant upregulation of IDO1 in diverse tumor types, such as melanoma, cervical cancer, oral squamous cell carcinoma, colorectal cancer, esophageal cancer, and pancreatic cancer (Zhai et al., 2018; Chen et al., 2020; Prendergast et al., 2014). Furthermore, the presence of IDO1 expression in either tumor cells or stromal cells has been linked to an enhanced aggressive phenotype, unfavorable clinical outcomes, and a bleak prognosis (Zhang et al., 2022; Iversen et al., 2015; Rubel et al., 2018; Kozuma et al., 2018; Hornyak et al., 2018). Previous research has indicated that the metabolism of Trp in cancerous cells and/or surrounding cells plays a role in inhibiting the immune system’s ability to fight against tumors (Platten et al., 2021). Trp and its metabolites interact with IDO can modulate not only tumor cells, but also the entire tumor microenvironment, and ultimately promoting immunosuppression and drug resistance (Greene et al., 2019; Li and Zhao, 2021; Maleki et al., 2015). In addition, Trp metabolism, a novel immune checkpoint that can influence the outcome of immunotherapy, is also a potential therapeutic target (Li et al., 2019). From the above mentioned studies, it is known that Trp metabolism plays a pivotal role in initiation and progression of various cancers; however, the role of Trp metabolism-related genes (TMRGs) in LSCC and their prognostic value are not yet clear.
In this study, we extracted TCGA-LSCC, GSE27020, and 40 TMRGs from publicly available databases, and then identified DE-TMRGs by Weighted Gene Co-expression Network Analysis (WGCNA), differential expression analysis, univariate Cox, least absolute shrinkage and selection operator (LASSO), and multivariate Cox regression analysis, and finally combined with relevant clinicopathological information to construct a risk model of Trp metabolism. In addition, we further analyzed the impact of the risk model on the immunotherapy and chemotherapy for LSCC. We also identified the optimal clustering form (molecular subtypes) by consensus clustering analysis of samples from TCGA-LSCC, and explored the relationship between clustering and LSCC prognosis. Therefore, this study aims to construct a risk model by analyzing the TMRGs in LSCC to further predict the prognosis of LSCC patients and guide personalized treatment.
The RNA-seq data, copy number, and somatic mutation data for TCGA-laryngeal squamous cell carcinoma (LSCC) were obtained via University of California Santa Cruz (UCSC) Xena (http://xena.ucsc.edu/), and a total of 111 LSCC samples and 12 control samples (82 LSCC samples with disease-free survival (DFS) information and clinical information) from Larynx were selected as the TCGA-LSCC dataset. The GSE27020 dataset consisting of 109 LSCC samples with DFS information was gained from Gene Expression Omnibus (GEO) (https://www.ncbi.nlm.nih.gov/), and was sequenced by GPL96 paltform. The GSE143224 dataset (GPL5175) consisting of 11 control samples and 14 LSCC samples was also gained from GEO. Then, a total of 40 TMRGs were extracted via Molecular Signatures Database (MSigDB) (https://www.gsea-msigdb.org/gsea/msigdb/index.jsp) (Xue et al., 2022).
In TCGA-LSCC, the wilcox. test was utilized to analyze the differences of TMRGs expression between LSCC and normal samples. After that, the somatic mutation frequency and copy number variant (CNV) frequency of TMRGs in LSCC samples were analyzed by R package maftools (v 2.2.10) (Xu Q. et al., 2021), and the location information of TMRGs in chromosomes was explored via R package RCircos (v 1.2.2) (Zhang et al., 2013). To further understand the correlations among TMRGs, the expressions of TMRGs in LSCC samples were extracted, and Spearman correlation analysis was carried out.
For the purpose of obtaining the key module that related to Trp metabolism (TM), the WGCNA was applied in TCGA-LSCC. First, the TM score of LSCC samples in TCGA-LSCC was scored utilizing the Gene Set Variation Analysis (GSVA) algorithm according to TMRGs, and which was considered as trait for WGCNA. Outliers were removed by clustering the samples to ensure the accuracy of the analysis. A soft threshold was determined to optimize the conformity of gene interactions with a scale-free distribution. Subsequently, the dissimilarity coefficient was introduced based on the adjacency and similarity between genes, leading to the generation of a systematic clustering tree for genes, and modules were screened out according to the dynamic tree cutting criteria. What’s more, the module with the highest correlation with TM score was sifted out as the key module. Finally, differentially expressed genes (DEGs) between LSCC and control samples from TCGA-LSCC were screened out by R package edgeR (v 3.36.0) (Liu S. et al., 2021) by setting |log2FC| > 1 and adj. P < 0.05, and they were overlapped with genes in key module to yield differentially expressed TMRGs (DE-TMRGs).
In order to identify genes that exhibited significant associations with survival outcomes in TCGA-LSCC, a univariate Cox analysis was conducted on DE-TMRGs, focusing on genes with a P value less than 0.05. Then, the LASSO analysis of genes gained in above step was applied by R package glmnet (v 4.0-2) (Zhang et al., 2019) to yield genes corresponding to lambda min, and they were analyzed for multivariate Cox to identify prognostic gene. A risk model was constructed according to the expressions (
To further investigate the relationship between risk score and clinical features of 82 LSCC samples in TCGA-LSCC (age, overall survival (OS), sex, T, N, grade, stage, and DFS), a violin diagram was drawn, and the expression of prognostic genes in various risk subgroups and clinical features was investigated using a heat map. In TCGA-LSCC, risk score and clinical features (age, grade, M, T, N, sex, and stage) were analyzed by univariate Cox analysis, and factor meeting P < 0.05 was defined as the independent prognosis factor. After that, a nomogram was constructed by rms package (v 6.2-0) (Liu TT. et al., 2021) to forecast the likelihood of patient survival. Additionally, to further validate the nomogram, ROC curves, calibration curves, and decision curve analysis (DCA) were run. Ultimately, the risk model was also subjected to hallmark pathway enrichment analysis to further explore the biological functions and pathways (Background gene set: Hallmark: h. all.v7.4. symbols. gmt).
In the high/low-risk subgroups from TCGA-LSCC, we adopted the single-sample gene set enrichment analysis (ssGSEA) algorithm to estimate the levels of immune cell infiltration, and intersubgroup differences of them were compared via wilcox. test. The Spearman correlation between risk score and immune cells was also examined, and variations in conventional immune checkpoints of subgroups were compared. What’s more, the LSCC tumor immune dysfunction and exclusion (TIDE) value was calculated, and difference of it between subgroups was contrasted. To investigate the impact of risk model on chemotherapy for LSCC, an analysis was conducted to assess 138 chemotherapy drugs sensitivity of LSCC in cancer (GDSC) database (https://www.cancerrxgene.org/), and the differences in 50% inhibitory concentration (IC50) of 138 chemotherapy drugs were estimated between subgroups.
For further assessing the role of prognostic gene in clinical classification of LSCC, clustering of LSCC samples from TCGA-LSCC was performed. First, using the ConsensusClusterPlus package (v 1.62.0) (Dong et al., 2021), consensus clustering analysis was carried out on 82 LSCC samples with survival data based on the prognostic gene., and the optimal clustering form was obtained based on the cumulative distribution function (CDF) value. The K-M analysis was adopted to study the association between clusters and LSCC prognosis. Subsequently, the differences of above immune cells, conventional immune checkpoints, TIDE value, as well as IC50 of 138 chemotherapy drugs between clusters were also analyzed.
In GSE143224, Wilcoxon test was performed to analyze the prognostic genes expression in tumor and normal groups (p < 0.05).
Subsequently, to further analyze the prognostic genes expression in clinical samples, we conducted qRT-PCR. A total of 10 pairs of normal and LSCC samples were obtained from the clinic in the Shanxi Provincial Cancer Hospital. All participants were given informed consent. The study had the approval of the Shanxi Provincial Cancer Hospital ethics committee.
Total RNA of 20 samples were extracted by employing the TRIzol reagent (Ambion, United States) as per the manufacturer’s instructions. The SureScript First-strand cDNA Synthesis kit (Servicebio, China) was utilized to perform reverse transcription and generate cDNA. For qRT-PCR analysis, a CFX Connect Thermal Cycler (Bio-Rad, United States) was employed. The 2−ΔΔCT method was utilized to ascertain the relative quantification of mRNAs (Livak and Schmittgen, 2001). The sequence information for all primers was displayed in Table 1.
The R software was utilized for conducting statistical analysis. The Wilcox test was employed to examine the variations among the groups. When P < 0.05, a substantial difference was deemed to exist.
Violin plot revealed that totally 23 TMRGs were notably differentially expressed between LSCC and normal samples (Figure 1A). Besides, IDO1 and OGDHL had high mutation frequencies in LSCC somatic mutations, and the main mutation type was Missense Mutation (Figure 1B). In LSCC samples, EHHADH exhibited the highest frequency of copy number amplification and WARS2 the highest frequency of copy number deletion (Figure 1C). Figure 1D illustrated the location of TMRGs on different chromosomes, where ASMT located on both chromosomes X and Y. Eventually, there was a notable strongest positive correlation between IDO1 and WARS, and AOX1 had a significant strongest negative correlation with ECHS1 (Figure 1E).
Figure 1. Genetic landscape of TMRGs in LSCC. (A) Differential expression of TMRGs between tumor and normal tissue samples. (B) Somatic mutational map of TMRGs in tumor samples. (C) CNV of TMRGs within tumor samples. (D) Infosphere diagram of TMRGs at different chromosomal locations. (where the gene ASMT occurs on both chromosomes X and Y). (E) Heatmap of correlation between TMRGs. * means p < 0.05,** means p < 0.01,*** means p < 0.001,**** means p < 0.0001, - means p > 0.05. TMRGs, tryptophan metabolism-related genes; LSCC, laryngeal squamous cell carcinoma; CNV, copy number variant.
The clustering of TCGA-LSCC samples showed that there was no necessity to exclude any samples (Figure 2A). Based on Figure 2B, a soft threshold was chosen as 9. Meanwhile, the network was approaching scale-free distribution, as seen by the vertical coordinate R^2 roughly converges around 0.85 and the average value of the adjacency function steadily goes towards 0. After that, the 16 modules were finally sifted out according to co-expression matrix (the similar modules had been merged) (Figure 2C), among which the green module was defined as the key module, which exhibited the highest correlation with TM score (Cor = 0.45, and P < 0.05), and which contained 1,232 module genes (Figure 2D). There were 1,483 DEGs between LSCC and normal samples (Figure 2E), and they were intersected with genes in key module to get 98 DE-TMRGs (Figure 2F).
Figure 2. WGCNA analysis of TMRGs in TCGA-LSCC samples. (A) The overall clustering of TCGA-LSCC samples. (B) Scale-free soft threshold distribution and determines the power threshold value to be 9. (C) The Clustering of module eigengenes; Eigengene adjacency heatmap; Module clustering dendrogram. (D) Heat map of module correlation with clinical characteristics, the green module has been selected as a key module, containing 1,232 module genes. (E) Gene expression difference between LSCC and normal samples is displayed by a volcano plot. (F) A total of 98 DE-TMRGs were determined among the differentially expressed genes and module genes. TMRGs, tryptophan metabolism-related genes; LSCC, laryngeal squamous cell carcinoma; CNV, copy number variant.
Based on 98 DE-TMRGs, 14 genes were discovered using univariate Cox regression (HR ≠ 1, P < 0.05) in TCGA-LSCC. The LASSO method further refined this list to 12 genes based on a minimum lambda of 0.02907679. Subsequently, five prognostic genes (SERPINA1, TMC8, RENBP, SDS, and FAM107A) were determined through multivariate Cox regression analysis (HR ≠ 1, P < 0.05) (Figure 3A). Besides, the high-risk subgroup exhibited a substantial lower survival (Figure 3B). The area under the curve (AUC) values for 1- (0.88), 3- (0.82) and 5- (0.76) year all surpassed 0.7, indicating that the risk model performed exceptionally well in predicting LSCC patients. (Figure 3C). Undoubtedly, risk score differed sensibly between Disease Free and Recurred/Progressed groups (Figure3D), and the percentage of Recurred/Progressed patients with high risk was higher than that in Disease Free (Figure 3E). The results were further validated in GSE27020 (Figure 3F).
Figure 3. Determination of genes contributing to risk model. (A) Univariate Cox analysis of DE-TMRGs; Trajectory schemes were drawn for every independent variable associated with lambda; Confidence interval under lambda; Multivariate Cox analysis of DE-TMRGs. (B) K-M curves for different risk groups in TCGA-LSCC samples. (C) ROC curves for different risk groups in TCGA-LSCC samples. (D) Risk score between Disease Free and Recurred/Progressed groups in TCGA-LSCC samples. (E) Disease Free and Recurred/Progressed percentage between different risk groups in TCGA-LSCC samples. (F) ROC curves, K-M curves, Risk score and percentage between different risk groups were validated in GSE27020 dataset. TMRGs, tryptophan metabolism-related genes; LSCC, laryngeal squamous cell carcinoma; ROC, Receiver operating characteristic; K-M, Kaplan-Meier.
Violin plot showed notable difference in risk score both between OS and DFS subgroups (Figure 4A), and heat map illustrated the prognostic gene expressions in different risk subgroups and different clinical features (Figure 4B). The independent prognosis factor risk score, was finally obtained through univariate Cox (Figure 4C). Subsequently, the nomogram indicated that the risk model had a good capacity to predict patients with LSCC, and the results were further supported by the calibration, ROC, and decision curves (Figure 4D). Moreover, the risk model was engaged in xenobiotic metabolism, fatty acid metabolism, bile acid metabolism, heme metabolism, IL6 JAK stat3 signaling and other hallmark pathways (Figure 4E).
Figure 4. The risk score was defined as an independent prognosis factor, and the risk model was involved in metabolism related pathways: (A) Various clinicopathological groups derived from TCGA-LSCC cohort are compared pairwise in the parameter of risk score. (B) Heat map of risk model genes expressions in different risk groups and different clinical characteristics. (C) Univariate Cox analysis of independent prognostic factors. (D) Nomogram model; The nomogram showed the most powerful capacity for survival prediction; ROC curves of the nomogram; DCA curves of the nomogram. (E) The different Hallmark pathways in high-risk and low-risk groups displayed as a heatmap. LSCC, laryngeal squamous cell carcinoma; DCA, Decision curve analysis; ROC, Receiver operating characteristic.
In box plot, it could be seen that the infiltration levels of CD56 bright natural killer (NK) cell, Type 2 helper T cell, and Activated CD8 T cell differed notably between two risk subgroups (Figure 5A). Besides, the risk score showed a noteworthy positive correlation with Type 2 helper T cell, and had a notable adverse correlation with Activated B cell (Figure 5B). A total of nine immune checkpoints exhibited sensible expression disparities among high/low-risk subgroups, including BTNL2, CD276, CD44, HHLA2, and TNFRSF18, etc. (Figure 5C). Obviously, the TIDE score was significantly lower in the low-risk subgroup compared to the high-risk group, indicating a lower likelihood of immune evasion and better response to immunotherapy among low-risk LSCC patients (Figure 5D). Eventually, the study found that patients with low-risk were notably sensitive to Salubrinal, Lenalidomide, Metformin and others, while those with high-risk were noticeably sensitive to Docetaxel, AUY922, Embelin, etc. (Figure 5E).
Figure 5. Immune microenvironment analysis of the TCGA-LSCC cohort: (A) Box plot of infiltration abundance of 28 immune cells in high and low-risk groups. (B) Correlation analysis between 28 immune cell and risk score; Correlation scatter plot between risk score and Type 2 helper T cell; Correlation scatter plot between risk score and activated B cell; (C) Differentially expressed immune checkpoint genes in high and low-risk groups. (D) The TIDE score in high and low-risk groups. (E) The estimated IC50 values for top five drugs of significant differences between high and low-risk groups. * means p < 0.05,** means p < 0.01,*** means p < 0.001,**** means p < 0.0001. LSCC, laryngeal squamous cell carcinoma; IC50, half maximal inhibitory concentration; TIDE, tumor immune dysfunction and exclusion.
Through consensus clustering analysis, the LSCC samples were classified into two clusters (Figure 6A), and cluster1 had a poorer survival state than that of cluster 2 (Figure 6B). The heat map illustrated the prognostic gene expressions in different clusters and clinical characteristics (Figure 6C). Moreover, the infiltration levels of 13 immune cells like Macrophage and Regulatory T cell, and expression levels of 15 immune checkpoints including BTNL2, TNFSF9 and others were sensibly different between cluster1 and cluster2 (Figure 6D). Meanwhile, the TIDE score of cluster 1 was notably higher than that of the cluster 2, suggesting a higher probability of LSCC immune evasion of cluster 1 patients (Figure 6E). Apparently, the patients in cluster 2 were sensibly sensitive to Rapamycin, Salubrinal, Tipifarnib and so on, while patients in cluster 1 were more responsive to AZ628, Pazopanib, AZD7762, etc. (Supplementary Figure S1, Figure 6F). Sankey diagram demonstrated the relationship among molecular clusters, high/low-risk subgroups, and DFS status (Figure 6G).
Figure 6. Consensus clustering analysis of the TCGA-LSCC cohort. (A) Cumulative distribution function curves for samples that are from TCGA-LSCC cohort; Curves for the delta area under the cumulative distribution function curves for samples that are from TCGA-LSCC cohort; The two sample clusters (k = 2) was displayed as a heat map. (B) The prognosis of two TCGA-LSCC clusters is displayed as a K-M curve. (C) The risk model genes expressions in different clusters and clinical characteristics as a heat map. (D) Differentially infiltrated immune cells between different clusters; Differentially expressed immune checkpoints between different clusters. (E) The TIDE score between different clusters. (F) The estimated IC50 values for top five drugs of significant differences between different clusters. (G) The relationship among molecular clusters, high/low-risk groups, and DFS status are displayed as sankey diagram. LSCC, laryngeal squamous cell carcinoma; IC50, half maximal inhibitory concentration, TIDE, tumor immune dysfunction and exclusion; K-M, Kaplan-Meier.
To investigate the expression of prognostic gene (SERPINA1, TMC8, RENBP, SDS, and FAM107A), firstly, the three prognostic genes (RENBP, SDS, and SERPINA1) were remarkably upregulated in the tumor group compared with the normal in GSE143224 (P < 0.05), in contrast, FAM107A exhibited significantly higher expression levels in normal samples (P < 0.05). The expression level of TMC8 was higher in the tumor group samples than in the normal group, but the difference was not significant (Figure 7A). Meanwhile,we examined their expression in LSCC tissue by qRT-PCR and compared it with normal tissue samples, we obtained similar conclusions. Specifically, our data showed a significant decrease in the expression of FAM107A and a increase in the expression of SERPINA1, TMC8, RENBP, in LSCC patients compared to normal (Figure 7B).
Figure 7. (A): The expression of prognostic gene in GSE143224. (B): Expression validation of prognostic gene in our study.
LSCC is the most common pathological type of LCa and also one of the most common malignant tumors of the head and neck (Johnson et al., 2020; Cohen et al., 2019). The incidence of LSCC has been on the rise in recent years, both in China and other countries, and its poor prognosis and high mortality rate have become a major risk factor for human health (Echanique et al., 2021; Costa et al., 2021; Deng et al., 2020). Despite the continuous improvement in the diagnosis and treatment of LSCC, including surgery, radiotherapy, chemotherapy, neoadjuvant therapy, immunotherapy, or strategies combining different treatment modalities,the overall survival rate has not been significantly improved (Qin et al., 2021; Bryan et al., 2016; Karam and Raben, 2019; Guidi et al., 2018). Due to the insidious onset of LSCC, which has already progressed to mid and late stages in most patients at the time of diagnosis, and the high recurrence rate, the 3, 5-year DFS of LSCC has not been significantly improved either (Volkov, 1972; Obid et al., 2019). Therefore, searching for new targets, early prediction, early diagnosis, early intervention, and providing new diagnostic and therapeutic approaches for LSCC may be important means to improve the prognosis of LSCC patients (Sung et al., 2021).
Trp metabolism produces a variety of active substances with physiological effects, which are important for maintaining cell activation and proliferation (Neavin et al., 2018; Conejos et al., 2021). Trp and its metabolites have diverse biological effects in the regulation of multiple diseases (Conejos et al., 2021; Ding et al., 2020; Wu et al., 2020). In particular, Trp and its metabolites play an important role in tumor formation and evolution (Kwiatkowska et al., 2021; Tanaka et al., 2021). Perez L et al. (Perez-Castro et al., 2023) showed that Trp metabolites activate the aromatic receptor (AHR), increase malignant degree, and inhibit anti-tumor immune responses. In a study of oral squamous cell carcinoma (OSCC), Tryptophanyl-tRNA synthetase (TrpRS) expression was upregulated in tumor tissues, and the expression level of TrpRS correlated with the clinicopathological features of OSCC patients (Lee et al., 2015). Another study regarding head and neck squamous carcinoma (HNSC) showed that IDO and TDO2 are indicators of immunosuppression and poor survival in HNSC patients, and that IDO1 in HNSC is negatively correlated with programmed cell death protein ligand 1 (PDL-1) (Riess et al., 2020). Additionally, studies revealed that TMRGs also play significant roles in LSCC and OSCC. For instance, drugs that inhibit the overexpression of Akt1 (RAC-α serine/threonine-protein kinase) and Akt2 (RAC-β serine/threonine-protein kinase) without reducing MAOB expression could serve as lead compounds for the treatment of oral squamous cell carcinoma (OSCC) (Sharif Siam et al., 2021). Furthermore, ACAT1 inhibitors have been shown to enhance the therapeutic efficacy of CSCs-DC vaccines in a mouse model of postoperative recurrence of head and neck squamous cell carcinoma (HNSCC), suggesting that ACAT1 may play an important role in cancer immunotherapy (Chen et al., 2017). Additionally, both low and high expression levels of ALDH2 have been associated with tumor progression, depending on the type of cancer, indicating that ALDH2 is a potential therapeutic target for cancer treatment (Zhang and Fu, 2021).
In this study, we analyzed the RNA-seq data, copy number, somatic mutation data and clinicopathological features of the TCGA-LSCC dataset to explore the mechanisms related to Trp metabolism in LSCC, and finally identified five genes (SERPINA1, TMC8, RENBP, SDS, and FAM107A) to establish a risk model. Tryptophan metabolism, particularly through the kynurenine pathway, plays a crucial role in shaping the tumor immune microenvironment (Qin et al., 2021). Key enzymes such as IDO1 and TDO2 catalyze the conversion of tryptophan to kynurenine, producing immunosuppressive metabolites that inhibit anti-tumor immune responses (Prendergast et al., 2014). In LSCC, the upregulation of these enzymes may lead to increased immune evasion and tumor progression. Additionally, TMRGs may affect the infiltration of immune cells such as Tregs and MDSCs, which contribute to an immunosuppressive microenvironment (Perez-Castro et al., 2023). The potential pathway interactions of TMRGs in LSCC are illustrated in Figure 8. In addition, two molecular clusters related to Trp metabolism were also identified.
The SERPINA1 encodes the alpha-1 antitrypsin (AAT) protein, which is involved in the regulation of enzyme activity and protein metabolism, as well as cell growth, differentiation, motility and signaling (Ando et al., 2021; Lim et al., 2020; Cipriani et al., 2018). Previous research findings have indicated that there is a significant upregulation of SERPINA1 in various types of tumor tissues, which has been strongly linked to the advancement and prognosis of tumors. Jiang and Hu (2020) found that SERPINA1 expression was linked to the development of gastric cancer and enhanced the invasion and migration of the disease’s cells. As a result, it may offer a viable target for the disease’s prevention. Kwon et al. (2015) found that SERPINA1 expression was linked to poor prognosis of colorectal cancer, as well as tumor stage, and lymph node metastasis, and that overexpression of SERPINA1 increased the capacity of cancer cells to invade and migrate, whereas silencing of SERPINA1 expression decreased this capacity. In hepatocellular carcinoma, Dongre and Weinberg (2019) reached a similar conclusion that patients with high expression of SERPINA1 in hepatocellular carcinoma tissues may have a higher risk of recurrence and metastasis. The expression level of SERPINA1 in oral tumor tissues was notably higher than normal tissues, which was associated with the progression, metastasis and prognosis of the tumor (Kawahara et al., 2016). In our study, we used qRT-PCR to detect the expression level of SERPINA1 in tumor and adjacent tissues of LSCC patients, and the results showed that the expression level of SERPINA1 in LSCC was significantly higher, which was in line with the results of other scholars’ studies.
The mammalian transmembrane channel-like (TMC) gene family consists of eight genes, ranging from TMC 1 to 8, and TMC8 is also known as EVER2. It is widely acknowledged that individuals diagnosed with HNSC frequently exhibit HPV infection, and studies in recent years have suggested that common variants of TMC8 are noteworthily related to HPV infection and HNSC patients prognosis (Lin et al., 2021). Liang et al. (2015) also have reported a correlation between genetic variation in TMC8 and the occurrence of high-risk HPV infection as well as its role in the development of HNSC, and it is also a fundamental regulatory factor for the persistence and carcinogenicity of HPV infection. In addition, TMC8 has the potential to impact tumor behavior by modulating immune pathways; The presence of TMC8 in tumor tissues showed a significant and positive correlation with the infiltration of various immune cells, including B cells, CD4+ T cells, macrophages, CD8+ T cells, and dendritic cells, and TMC8 may increase the infiltration of cytotoxic T cells and B lymphocytes by influencing the functionality of CD4+ T cells (Liang et al., 2015). According to a study by Lin et al. (2021) TMC8 expression was shown to be considerably higher in HPV-positive HNSC patients. This finding indicated a positive link between Th2 infiltration and B cell enrichment, indicating that TMC8 may operate as a barrier to HPV through Th2 cells. We propose that TMC8 could potentially contribute to the development of resistance against HPV infection, consequently reducing the vulnerability of LSCC patients.
RENBP encodes renin-binding protein, an important regulator in the renin-angiotensin-aldosterone system, which is responsible for participating in blood pressure regulation and maintenance of sodium homeostasis. RENBP has been proved to be expressed in mouse lungs and may promote local lesion development through an inflammatory response (Huffnagle et al., 2017), so we hypothesized that micro-metabolic changes induced by inflammation in LSCC may affect RENBP gene expression and consequently affects tumorigenesis.
The family with sequence similarity 107 member A (FAM107A) gene, also known as DRR1 or TU3A, a specific set of codons encodes multiple amino acids. Previously published studies have shown that FAM107A has reduced expression in various tumor tissues, including prostate cancer, hepatocellular carcinoma, lymphoma and non-small cell lung cancer, which exhibits the properties of an oncogene (Vanaja et al., 2006; Udali et al., 2015; Lawrie et al., 2018; Pastuszak-Lewandoska et al., 2015); while FAM107A was highly expressed in the aggressive component of gliomas and was associated with increased aggressiveness and disease progression in neuroblastoma, meningioma and malignant glioma (Mu et al., 2017; Asano et al., 2010; Fevre-Montange et al., 2009; Nakajima and Koizumi, 2014). The study of Kiwerska et al. (2017) found that FAM107A functioned as an oncogene in LSCC, and it led to the decrease of its expression in LSCC through DNA methylation and combined deletion. In the present study, the expression level of FAM107A in tumor tissues was significantly lower than that in the adjacent normal tissues, which was in line with the findings of other scholars.
Compared with other genes, SDS has not been deeply studied in LSCC, and the specific mechanism of SDS as a pro-carcinogenic gene in LSCC still needs to be further explored in the future.
We further confirmed that risk score alone was an independent prognostic factor by univariate Cox analysis of clinical characteristics and risk score, and in turn created a nomogram for prediction of risk and survival probability in LSCC. Hallmark pathway enrichment analysis reveals the risk model was also engaged in bile acid metabolism, fatty acid metabolism, xenobiotic metabolism, heme metabolism, IL6 JAK stat3 signaling and other hallmark pathways.
Immunotherapy is currently a more promising means of tumor treatment, especially immune checkpoint-based immunotherapy, which has made significant breakthroughs in the treatment of various tumors and reshaped the landscape of tumor treatment (Marabelle et al., 2020; Kruger et al., 2019; Ribas and Wolchok, 2018). In this study, we found that a total of nine immune checkpoints were sensitively expressed in high/low-risk subgroups. The high risk subgroup had much higher levels of immune checkpoint gene expression, such as Neuropilin-1 (NRP1). NRP1 is a neuronal and endothelial cell receptor essential for normal embryonic development and angiogenesis, whereas it suppresses anti-tumor immune response in the formation and development of malignant tumors, which correlates with poor clinical outcome (Delgoffe et al., 2013; Overacre-Delgoffe et al., 2017). On the one hand, NRP1 promotes tumor cell growth through multiple pathways, such as neoangiogenesis, metastasis; on the other hand, NRP1 facilitates immune escape by regulating intra-tumor Treg cells and CD8+ T and orchestrating multiple inhibitory processes in the tumor microenvironment (TME) (Overacre-Delgoffe et al., 2017; Liu et al., 2020). As a member of the B7/CD28 family, the molecular structure of human endogenous retrovirus-H long terminal repeat-associated protein 2 (HHLA2) shares some homology and similarity with other B7 family members, such as PD-L1, and exhibits both co-stimulatory and co-inhibitory effects on T cells (Janakiram et al., 2017; Xiao and Freeman, 2015) TMIGD2 is one of the specific receptors for HHLA2, often also known as CD28H (Zhu et al., 2013). It has been discovered that several solid tumors have high expressions of HHLA2, including breast cancer (Liu et al., 2017), osteosarcoma (Koirala et al., 2016), colon cancer (Zhu and Dong, 2018), clear cell carcinoma of the kidney (Chen et al., 2019), and bladder cancer (Lin et al., 2019), and so on. Based on this, HHLA2/TMIGD2 is expected to be one of the new pathways and targets for tumor immunotherapy, especially providing new ideas for PD-L1-negative tumor immunotherapy. Some published research indicated that HHLA2 may have different effects on prognosis in different types of tumors. On the one hand, overexpression of HHLA2 in pancreatic, ampullary and ovarian carcinoma is closely associated with a favorable prognosis (Boor et al., 2020; Xu G. et al., 2021). On the other hand, co-expression of HHLA2 and PD-L1 in renal clear cell carcinoma shows a poor prognosis (Zhou et al., 2020). In our study, HHLA2 was highly expressed in patients with low-risk, suggesting that LSCC patients with low-risk may benefit from immunotherapy based on this target. CD40LG (also known as CD154) is the ligand gene for CD40, belonging to the tumor necrosis factor (TNF) gene superfamily, predominantly expressed on activated T cells and platelets (Kuhn et al., 2019). It is also involved in tumor pathogenesis and anti-tumor immunity (Hassan et al., 2015). CD40−CD40LG interaction is an important signal for B-cell proliferation, activation biomarker expression and immunoglobulin production, which directly inhibits the growth of CD40-positive tumor cells and may indirectly inhibit tumor growth by downregulating the immune response (Laman et al., 2017; Jansen et al., 2016). Yuan et al. (2021) found that CD40LG is a valuable prognostic molecular marker in breast cancer, and its low expression is associated with poor outcomes. In our study, we found that CD40LG was lowly expressed in the high risk subgroup, which may be associated with a poorer prognosis in these patients. For the TIDE score, it was higher in patients with high-risk than low-risk, suggesting that high-risk patients may have a higher likelihood of immune escape in response to immune checkpoint therapy, which may have a negative impact on the effect of immunotherapy in these patients.
To explore the role of risk model in LSCC chemotherapy and find chemotherapy-sensitive drugs in high/low-risk subgroup, we performed a drug sensitivity analysis and found that patients in the low-risk subgroup were sensitive to Salubrinal, while those in the high-risk subgroup were sensitive to these drugs including Docetaxel, AUY922, Embelin, and CHIR.99021. Salubrinal is a selective inhibitor of eIF2α that acts by inducing phosphorylation and inhibiting dephosphorylation of eIF2α. Salubrinal was found to act differently when combined with different types of chemotherapeutic agents, it can enhance the inhibitory effect of drugs such as doxorubicin on tumors (Jeon et al., 2016); whereas in combination with platinum-based drugs, Salubrinal attenuates the antineoplastic efficacy of platinum-based chemotherapeutic agents (Chen et al., 2011; Feng et al., 2013). Therefore, when selecting chemotherapeutic drugs for patients in the high-risk subgroup, attention should be paid to the rational selection of drugs to maximize the antitumor activity of different types of drugs.
Based on consensus clustering analysis, we identified two different clusters, and the cluster 1 had worse DFS compared to the cluster 2. The proportions of immune cells such as MDSC, Macrophage, and Regulatory T cells were also higher in cluster 1 than in cluster 2 subtype, which is important for the formation of an immunosuppressive tumor microenvironment and the inhibition of migration and infiltration of immune cells into tumor tissues, and this may be one of the reasons for the worse DFS of the cluster 1, which is in line with the studies of other scholars (Sica and Massarotti, 2017; Wang et al., 2021).
Trp metabolism is closely associated with the formation of the tumor immune microenvironment and impacts anti-tumor immune responses through multiple pathways. Increased activity of rate-limiting enzymes in Trp metabolism leads to Trp depletion in the tumor microenvironment, which in turn induces cell-cycle arrest of peripheral T cells and promotes the generation of Tregs (Wolf et al., 2015; Fallarino et al., 2006). Moreover, Kyn, a metabolite of Trp metabolism, can activate AHR on CD4+ T cells and induce differentiation of CD4+ T cells to regulatory T cells (Mezrich et al., 2010). Within the tumor microenvironment, Tregs inhibit the function of effector T cells by secreting inhibitory cytokines or interacting with antigen-presenting cells, thereby modulating anti-tumor immune responses. This aligns with the immune cell infiltration pattern we observed in cluster 1, suggesting that Trp metabolism plays a pivotal role in shaping an immunosuppressive microenvironment. We further examined the expression of immune checkpoint genes across various clusters. Intriguingly, a higher degree of suppression was observed in cluster 2 compared to cluster 1, suggesting that a favorable clinical outcome for cluster 2 may be achieved through immune checkpoint blockade rather than immune cell infiltration, and this is similar to the study by Luo P et al. on Trp metabolism in gastric cancer (Luo et al., 2023). Meanwhile, the TIDE score of cluster 1 was significantly higher than that of cluster 2, indicating that patients in cluster 1 are more likely to be refractory to immunotherapy, leading to a poorer prognosis. In the analysis of chemotherapeutic drug sensitivity between the two molecular subtypes, we found that patients in cluster 1 were more sensitive to drugs such as AZ628, AZD7762, CEP.701, KIN001.135, and Pazopanib, while patients in cluster 2 were more sensitive to rapamycin, Salubrinal, and Tipifarnib.
Our study suggests that high-risk LSCC patients may have a poorer response to immunotherapy, as indicated by higher TIDE scores and lower immune cell infiltration. While our findings are based on bioinformatics analysis and require experimental validation, they provide a potential framework for identifying patients who may benefit less from standard immunotherapy approaches. In clinical settings, this could help in stratifying patients for alternative or combination therapies. For instance, for high-risk patients, personalized immunotherapy strategies could be developed. In addition to conventional immunotherapy, combination treatments (such as chemotherapy, targeted therapy, or other immunotherapeutic approaches) could be considered to improve treatment outcomes. Future research should focus on validating these findings through prospective clinical trials and functional studies to explore the therapeutic potential of targeting specific TMRGs or immune checkpoints in LSCC.
Nevertheless, it is important to acknowledge certain limitations in our investigation. Firstly, the data utilized in our study were retrospectively collected from the TCGA and GEO databases. Therefore, the potential bias in case selection might affect the results. Secondly, these data were analyzed only by bioinformatics, and future investigations should include relevant in vivo and in vitro validation experiments to confirm the efficacy of risk modeling. In addition, the molecular mechanisms associated with Trp metabolism in LSCC remain to be further validated. In addition, the molecular mechanisms associated with Trp metabolism in LSCC remain to be further validated. Moreover, the sample size of our qRT-PCR validation was relatively small, which limits the robustness of our findings. Future studies should validate the identified TMRGs in larger patient cohorts. Furthermore, functional assays, such as gene knockdown or overexpression studies, are needed to establish the mechanistic roles of these genes in LSCC progression. Additionally, immunohistochemistry or Western blot analysis should be incorporated to verify protein-level expression and localization.
In this study, five TMRGs (SERPINA1, TMC8, RENBP, SDS and FAM107A) were identified to further establish a risk model, which had specifically good predictive performance, and can provide an effective method for evaluating the prognosis LSCC patients. The nomogram based on the five TMRGs can provide a concise and digital predictions to patients through simple calculations. Our study also reveals the clinical significance of Trp metabolism and its underlying association with LSCC, which may provide a new approach for personalized comprehensive treatment of LSCC patients.
The datasets presented in this study can be found in online repositories. The names of the repository/repositories and accession number(s) can be found in the article/Supplementary Material.
The studies involving humans were approved by This study has received approval from the Medical Ethics Committee of Shanxi Cancer Hospital (Ethics number:KY2023033). The studies were conducted in accordance with the local legislation and institutional requirements. The human samples used in this study were primarily isolated as part of a previous study for which ethical approval was obtained. Informed consent for participation was provided by the participants or the participants' legal guardians/next of kin in accordance with the national legislation and institutional requirements.
FL: Conceptualization, Data curation, Writing–original draft, Writing–review and editing, Software, Visualization. YQ: Conceptualization, Methodology, Writing–original draft, Writing–review and editing, Supervision. WL: Formal Analysis, Methodology, Writing–review and editing, Investigation. Xianhui XR: Resources, Supervision, Writing–original draft, Visualization. LL: Conceptualization, Software, Writing–review and editing, Project administration. BF: Resources, Software, Validation, Writing–review and editing. JY: Conceptualization, Methodology, Resources, Writing–review and editing.
The author(s) declare that no financial support was received for the research and/or publication of this article.
We acknowledge the Shanxi Cancer Hospital for their contributions and help in sample collection.
The authors declare that the research was conducted in the absence of any commercial or financial relationships that could be construed as a potential conflict of interest.
The author(s) declare that no Generative AI was used in the creation of this manuscript.
All claims expressed in this article are solely those of the authors and do not necessarily represent those of their affiliated organizations, or those of the publisher, the editors and the reviewers. Any product that may be evaluated in this article, or claim that may be made by its manufacturer, is not guaranteed or endorsed by the publisher.
PCOS, Polycystic ovary syndrome; DEGs, Differentially expressed genes; HA, Hyperandrogenism; SVM-RFE, Support vector machine recursive feature elimination; DCA, Decision curve analysis; FC, Fold change; IR, Insulin resistance; LASSO, Least absolute shrinkage and selection operator; MSigDBq, Molecular signatures database; qRT-PCR, Quantitative real-time polymerase chain reaction; PCA, Principal component analysis; GEO, Gene expression omnibus; GO, Gene ontology; KEGG, Kyoto encyclopedia of genes and genomes; AUC, Area under curve; BP, Biological process; MF, Molecular function; CC, Cellular component; ROC, Receiver operating characteristic; ssGSEA, Single-sample gene set enrichment analysis; TFs, Transcription factors; CTD, Comparative toxicogenomics database; CRG, Cell adhesion-related genes.
Ando, Y., Kuroda, A., Kusama, K., Matsutani, T., Matsuda, A., and Tamura, K. (2021). Impact of serine protease inhibitor alpha1-antitrypsin on expression of endoplasmic reticulum stress-induced proinflammatory factors in adipocytes. Biochem. Biophy. Rep. 26, 100967. doi:10.1016/j.bbrep.2021.100967
Asano, Y., Kishida, S., Mu, P., Sakamoto, K., Murohara, T., and Kadomatsu, K. (2010). DRR1 is expressed in the developing nervous system and downregulated during neuroblastoma carcinogenesis. Biochem. Biophys. Res. Commun. 394, 829–835. doi:10.1016/j.bbrc.2010.03.085
Atef, A., El-Rashidy, M. A., Elzayat, S., and Kabel, A. M. (2019). The prognostic value of sex hormone receptors expression in laryngeal carcinoma. Tissue Cell 57, 84–89. doi:10.1016/j.tice.2019.02.007
Boor, P. P. C., Sideras, K., Biermann, K., Hosein Aziz, M., Levink, I. J. M., Mancham, S., et al. (2020). HHLA2 is expressed in pancreatic and ampullary cancers and increased expression is associated with better post-surgical prognosis. Br. J. Cancer 122, 1211–1218. doi:10.1038/s41416-020-0755-4
Bray, F., Ferlay, J., Soerjomataram, I., Siegel, R. L., Torre, L. A., and Jemal, A. (2018). Global cancer statistics 2018: GLOBOCAN estimates of incidence and mortality worldwide for 36 cancers in 185 countries. CA Cancer J. Clin. 68, 394–424. doi:10.3322/caac.21492
Bryan, R. B., Gough, M. J., Seung, S. K., Jutric, Z., Weinberg, A. D., Fox, B. A., et al. (2016). Cytoreductive surgery for head and neck squamous cell carcinoma in the new age of immunotherapy. Oral Oncol. 61, 166–176. doi:10.1016/j.oraloncology.2016.08.020
Chen, B., Alvarado, D. M., Iticovici, M., Kau, N. S., Park, H., Parikh, P. J., et al. (2020). Interferon-induced IDO1 mediates radiation resistance and is a therapeutic target in colorectal cancer. Cancer Immunol. Res. 8, 451–464. doi:10.1158/2326-6066.CIR-19-0282
Chen, D., Chen, W., Xu, Y., Zhu, M., Xiao, Y., Shen, Y., et al. (2019). Upregulated immune checkpoint HHLA2 in clear cell renal cell carcinoma: a novel prognostic biomarker and potential therapeutic target. J. Med. Genet. 56, 43–49. doi:10.1136/jmedgenet-2018-105454
Chen, R., Dai, R. Y., Duan, C. Y., Liu, Y. P., Chen, S. K., Yan, D. M., et al. (2011). Unfolded protein response suppresses cisplatin-induced apoptosis via autophagy regulation in human hepatocellular carcinoma cells. Folia Biol. (Praha) 57, 87–95. doi:10.14712/fb2011057030087
Chen, X., Song, Q., Xia, L., and Xu, X. (2017). Synergy of dendritic cell vaccines and avasimibe in treatment of head and neck cancer in mice. Med. Sci. Monit. 23, 4471–4476. doi:10.12659/msm.905814
Cipriani, C., Pascarella, S., Errante, F., Menicacci, B., Magnelli, L., Mocali, A., et al. (2018). Serpin A1 and the modulation of type I collagen turnover: effect of the C-terminal peptide 409-418 (SA1-III) in human dermal fibroblasts. Cell Biol. Int. 42, 1340–1348. doi:10.1002/cbin.11018
Cohen, E. E. W., Bell, R. B., Bifulco, C. B., Burtness, B., Gillison, M. L., Harrington, K. J., et al. (2019). The Society for Immunotherapy of Cancer consensus statement on immunotherapy for the treatment of squamous cell carcinoma of the head and neck (HNSCC). J. Immunother. Cancer 7, 184. doi:10.1186/s40425-019-0662-5
Conejos, J. R. V., Ghassemi Nejad, J., Kim, J. E., Moon, J. O., Lee, J. S., and Lee, H. G. (2021). Supplementing with L-tryptophan increases medium protein and alters expression of genes and proteins involved in milk protein synthesis and energy metabolism in bovine mammary cells. Int. J. Mol. Sci. 22, 2751. doi:10.3390/ijms22052751
Costa, S. N. L., Fernandes, F., Souza, D. L. B., Bezerra, H. S., Santos, E. G. O., and Barbosa, I. R. (2021). Incidence and mortality by larynx cancer in central and south America. Rev. Gaucha Enferm. 42, e20190469. doi:10.1590/1983-1447.2021.20190469
Delgoffe, G. M., Woo, S. R., Turnis, M. E., Gravano, D. M., Guy, C., Overacre, A. E., et al. (2013). Stability and function of regulatory T cells is maintained by a neuropilin-1-semaphorin-4a axis. Nature 501, 252–256. doi:10.1038/nature12428
Deng, Y., Wang, M., Zhou, L., Zheng, Y., Li, N., Tian, T., et al. (2020). Global burden of larynx cancer, 1990-2017: estimates from the global burden of disease 2017 study. Aging (Albany NY) 12, 2545–2583. doi:10.18632/aging.102762
Ding, X., Bin, P., Wu, W., Chang, Y., and Zhu, G. (2020). Tryptophan metabolism, regulatory T cells, and inflammatory bowel disease: a mini review. Mediat. Inflamm. 2020, 9706140. doi:10.1155/2020/9706140
Dong, C., Dang, D., Zhao, X., Wang, Y., Wang, Z., and Zhang, C. (2021). Integrative characterization of the role of IL27 in melanoma using bioinformatics analysis. Front. Immunol. 12, 713001. doi:10.3389/fimmu.2021.713001
Dongre, A., and Weinberg, R. A. (2019). New insights into the mechanisms of epithelial-mesenchymal transition and implications for cancer. Nat. Rev. Mol. Cell Biol. 20, 69–84. doi:10.1038/s41580-018-0080-4
Echanique, K. A., Evans, L. K., Han, A. Y., Chhetri, D. K., and St John, M. A. (2021). Cancer of the larynx and hypopharynx. Hematol. Oncol. Clin. North Am. 35, 933–947. doi:10.1016/j.hoc.2021.05.005
Fallarino, F., Grohmann, U., You, S., McGrath, B. C., Cavener, D. R., Vacca, C., et al. (2006). The combined effects of tryptophan starvation and tryptophan catabolites down-regulate T cell receptor zeta-chain and induce a regulatory phenotype in naive T cells. J. Immunol. 176, 6752–6761. doi:10.4049/jimmunol.176.11.6752
Feng, C. H., Chen, R., Chen, S. K., Li, J., Duan, C. Y., Liu, Y. P., et al. (2013). Phosphorylation of eukaryotic initiation factor 2-alpha inhibits cisplatin-mediated apoptosis of hepatocellular carcinoma cells. Zhonghua Gan Zang Bing Za Zhi 21, 290–294. doi:10.3760/cma.j.issn.1007-3418.2013.04.012
Fevre-Montange, M., Champier, J., Durand, A., Wierinckx, A., Honnorat, J., Guyotat, J., et al. (2009). Microarray gene expression profiling in meningiomas: differential expression according to grade or histopathological subtype. Int. J. Oncol. 35, 1395–1407. doi:10.3892/ijo_00000457
Gao, W., Zhang, C., Li, W., Li, H., Sang, J., Zhao, Q., et al. (2019). Promoter methylation-regulated miR-145-5p inhibits laryngeal squamous cell carcinoma progression by targeting FSCN1. Mol. Ther. 27, 365–379. doi:10.1016/j.ymthe.2018.09.018
Gao, W., Zhang, Y., Luo, H., Niu, M., Zheng, X., Hu, W., et al. (2020). Targeting SKA3 suppresses the proliferation and chemoresistance of laryngeal squamous cell carcinoma via impairing PLK1-AKT axis-mediated glycolysis. Cell Death Dis. 11, 919. doi:10.1038/s41419-020-03104-6
Greene, L. I., Bruno, T. C., Christenson, J. L., D'Alessandro, A., Culp-Hill, R., Torkko, K., et al. (2019). A role for tryptophan-2,3-dioxygenase in CD8 T-cell suppression and evidence of tryptophan catabolism in breast cancer patient plasma. Mol. Cancer Res. 17, 131–139. doi:10.1158/1541-7786.MCR-18-0362
Guidi, A., Codeca, C., and Ferrari, D. (2018). Chemotherapy and immunotherapy for recurrent and metastatic head and neck cancer: a systematic review. Med. Oncol. 35, 37. doi:10.1007/s12032-018-1096-5
Haddad, R. I., Posner, M., Hitt, R., Cohen, E. E. W., Schulten, J., Lefebvre, J. L., et al. (2018). Induction chemotherapy in locally advanced squamous cell carcinoma of the head and neck: role, controversy, and future directions. Ann. Oncol. 29, 1130–1140. doi:10.1093/annonc/mdy102
Hassan, G. S., Stagg, J., and Mourad, W. (2015). Role of CD154 in cancer pathogenesis and immunotherapy. Cancer Treat. Rev. 41, 431–440. doi:10.1016/j.ctrv.2015.03.007
Hornyak, L., Dobos, N., Koncz, G., Karanyi, Z., Pall, D., Szabo, Z., et al. (2018). The role of indoleamine-2,3-dioxygenase in cancer development, diagnostics, and therapy. Front. Immunol. 9, 151. doi:10.3389/fimmu.2018.00151
Huffnagle, G. B., Dickson, R. P., and Lukacs, N. W. (2017). The respiratory tract microbiome and lung inflammation: a two-way street. Mucosal Immunol. 10, 299–306. doi:10.1038/mi.2016.108
Iversen, T. Z., Andersen, M. H., and Svane, I. M. (2015). The targeting of indoleamine 2,3 dioxygenase -mediated immune escape in cancer. Basic Clin. Pharmacol. Toxicol. 116, 19–24. doi:10.1111/bcpt.12320
Janakiram, M., Shah, U. A., Liu, W., Zhao, A., Schoenberg, M. P., and Zang, X. (2017). The third group of the B7-CD28 immune checkpoint family: HHLA2, TMIGD2, B7x, and B7-H3. Immunol. Rev. 276, 26–39. doi:10.1111/imr.12521
Jansen, M. F., Hollander, M. R., van Royen, N., Horrevoets, A. J., and Lutgens, E. (2016). CD40 in coronary artery disease: a matter of macrophages? Basic Res. Cardiol. 111, 38. doi:10.1007/s00395-016-0554-5
Jeon, Y. J., Kim, J. H., Shin, J. I., Jeong, M., Cho, J., and Lee, K. (2016). Salubrinal-Mediated upregulation of eIF2α phosphorylation increases doxorubicin sensitivity in MCF-7/ADR cells. Mol. Cells 39, 129–135. doi:10.14348/molcells.2016.2243
Jiang, L., and Hu, L. G. (2020). Serpin peptidase inhibitor clade A member 1-overexpression in gastric cancer promotes tumor progression in vitro and is associated with poor prognosis. Oncol. Lett. 20, 278. doi:10.3892/ol.2020.12141
Johnson, D. E., Burtness, B., Leemans, C. R., Lui, V. W. Y., Bauman, J. E., and Grandis, J. R. (2020). Head and neck squamous cell carcinoma. Nat. Rev. Dis. Prim. 6, 92. doi:10.1038/s41572-020-00224-3
Karam, S. D., and Raben, D. (2019). Radioimmunotherapy for the treatment of head and neck cancer. Lancet Oncol. 20, e404–e416. doi:10.1016/S1470-2045(19)30306-7
Kawahara, R., Bollinger, J. G., Rivera, C., Ribeiro, A. C., Brandao, T. B., Paes Leme, A. F., et al. (2016). A targeted proteomic strategy for the measurement of oral cancer candidate biomarkers in human saliva. Proteomics 16, 159–173. doi:10.1002/pmic.201500224
Kiwerska, K., Szaumkessel, M., Paczkowska, J., Bodnar, M., Byzia, E., Kowal, E., et al. (2017). Combined deletion and DNA methylation result in silencing of FAM107A gene in laryngeal tumors. Sci. Rep. 7, 5386. doi:10.1038/s41598-017-05857-1
Koirala, P., Roth, M. E., Gill, J., Chinai, J. M., Ewart, M. R., Piperdi, S., et al. (2016). HHLA2, a member of the B7 family, is expressed in human osteosarcoma and is associated with metastases and worse survival. Sci. Rep. 6, 31154. doi:10.1038/srep31154
Kozuma, Y., Takada, K., Toyokawa, G., Kohashi, K., Shimokawa, M., Hirai, F., et al. (2018). Indoleamine 2,3-dioxygenase 1 and programmed cell death-ligand 1 co-expression correlates with aggressive features in lung adenocarcinoma. Eur. J. Cancer 101, 20–29. doi:10.1016/j.ejca.2018.06.020
Kruger, S., Ilmer, M., Kobold, S., Cadilha, B. L., Endres, S., Ormanns, S., et al. (2019). Advances in cancer immunotherapy 2019 - latest trends. J. Exp. Clin. Cancer Res. 38, 268. doi:10.1186/s13046-019-1266-0
Kuhn, N. F., Purdon, T. J., van Leeuwen, D. G., Lopez, A. V., Curran, K. J., Daniyan, A. F., et al. (2019). CD40 ligand-modified chimeric antigen receptor T cells enhance antitumor function by eliciting an endogenous antitumor response. Cancer Cell 35, 473–488.e6. doi:10.1016/j.ccell.2019.02.006
Kwiatkowska, I., Hermanowicz, J. M., Przybyszewska-Podstawka, A., and Pawlak, D. (2021). Not only immune escape-the confusing role of the TRP metabolic pathway in carcinogenesis. Cancers (Basel) 13, 2667. doi:10.3390/cancers13112667
Kwon, C. H., Park, H. J., Choi, J. H., Lee, J. R., Kim, H. K., Jo, H. J., et al. (2015). Snail and serpinA1 promote tumor progression and predict prognosis in colorectal cancer. Oncotarget 6, 20312–20326. doi:10.18632/oncotarget.3964
Laman, J. D., Claassen, E., and Noelle, R. J. (2017). Functions of CD40 and its ligand, gp39 (CD40L). Crit. Rev. Immunol. 37, 371–420. doi:10.1615/CritRevImmunol.v37.i2-6.100
Lawrie, A., Han, S., Sud, A., Hosking, F., Cezard, T., Turner, D., et al. (2018). Combined linkage and association analysis of classical Hodgkin lymphoma. Oncotarget 9, 20377–20385. doi:10.18632/oncotarget.24872
Lee, C. W., Chang, K. P., Chen, Y. Y., Liang, Y., Hsueh, C., Yu, J. S., et al. (2015). Overexpressed tryptophanyl-tRNA synthetase, an angiostatic protein, enhances oral cancer cell invasiveness. Oncotarget 6, 21979–21992. doi:10.18632/oncotarget.4273
Li, C., and Zhao, H. (2021). Tryptophan and its metabolites in lung cancer: basic functions and clinical significance. Front. Oncol. 11, 707277. doi:10.3389/fonc.2021.707277
Li, H., Bullock, K., Gurjao, C., Braun, D., Shukla, S. A., Bosse, D., et al. (2019). Metabolomic adaptations and correlates of survival to immune checkpoint blockade. Nat. Commun. 10, 4346. doi:10.1038/s41467-019-12361-9
Liang, C., Kelsey, K. T., McClean, M. D., Christensen, B. C., Marsit, C. J., Karagas, M. R., et al. (2015). A coding variant in TMC8 (EVER2) is associated with high risk HPV infection and head and neck cancer risk. PLoS One 10, e0123716. doi:10.1371/journal.pone.0123716
Lim, J. H., Park, S. M., Yook, J. M., Ahn, J. S., Choi, S. Y., Oh, S. H., et al. (2020). Alpha-1 antitrypsin inhibits formaldehyde-induced apoptosis of human peritoneal mesothelial cells. Perit. Dial. Int. 40, 124–131. doi:10.1177/0896860819887288
Lin, B., Wang, S., Yao, Y., Shen, Y., and Yang, H. (2021). Comprehensive co-expression analysis reveals TMC8 as a prognostic immune-associated gene in head and neck squamous cancer. Oncol. Lett. 22, 498. doi:10.3892/ol.2021.12759
Lin, G., Ye, H., Wang, J., Chen, S., Chen, X., and Zhang, C. (2019). Immune checkpoint human endogenous retrovirus-H long terminal repeat-associating protein 2 is upregulated and independently predicts unfavorable prognosis in bladder urothelial carcinoma. Nephron 141, 256–264. doi:10.1159/000495887
Liu, C., Somasundaram, A., Manne, S., Gocher, A. M., Szymczak-Workman, A. L., Vignali, K. M., et al. (2020). Neuropilin-1 is a T cell memory checkpoint limiting long-term antitumor immunity. Nat. Immunol. 21, 1010–1021. doi:10.1038/s41590-020-0733-2
Liu, J., Zhang, M., Sun, Q., Qin, X., Gao, T., Xu, Y., et al. (2023). Construction of a novel MPT-driven necrosis-related lncRNAs signature for prognosis prediction in laryngeal squamous cell carcinoma. Environ. Sci. Pollut. Res. Int. 30, 77210–77225. doi:10.1007/s11356-023-26996-1
Liu, S., Chen, S., Yuan, W., Wang, H., Chen, K., Li, D., et al. (2017). PD-1/PD-L1 interaction up-regulates MDR1/P-gp expression in breast cancer cells via PI3K/AKT and MAPK/ERK pathways. Oncotarget 8, 99901–99912. doi:10.18632/oncotarget.21914
Liu, S., Wang, Z., Zhu, R., Wang, F., Cheng, Y., and Liu, Y. (2021a). Three differential expression analysis mfor RNA sequencing: limma, EdgeR, DESeq2. J. Vis. Exp. 10, e003811.
Liu, T. T., Li, R., Huo, C., Li, J. P., Yao, J., Ji, X. L., et al. (2021b). Identification of CDK2-related immune forecast model and ceRNA in lung adenocarcinoma, a pan-cancer analysis. Front. Cell Dev. Biol. 9, 682002. doi:10.3389/fcell.2021.682002
Livak, K. J., and Schmittgen, T. D. (2001). Analysis of relative gene expression data using real-time quantitative PCR and the 2(-Delta Delta C(T)) Method. Methods 25, 402–408. doi:10.1006/meth.2001.1262
Lu, C., Shi, X., Wang, A. Y., Tao, Y., Wang, Z., Huang, C., et al. (2018). RNA-Seq profiling of circular RNAs in human laryngeal squamous cell carcinomas. Mol. Cancer 17, 86. doi:10.1186/s12943-018-0833-x
Luo, P., Chen, G., Shi, Z., Yang, J., Wang, X., Pan, J., et al. (2023). Comprehensive multi-omics analysis of tryptophan metabolism-related gene expression signature to predict prognosis in gastric cancer. Front. Pharmacol. 14, 1267186. doi:10.3389/fphar.2023.1267186
Maleki, V. S., Chen, D., Di Cresce, C., Ferguson, P. J., Figueredo, R., Pampillo, M., et al. (2015). Ido downregulation induces sensitivity to pemetrexed, gemcitabine, FK866, and methoxyamine in human cancer cells. PLoS One 10, e0143435. doi:10.1371/journal.pone.0143435
Marabelle, A., Le, D. T., Ascierto, P. A., Di Giacomo, A. M., De Jesus-Acosta, A., Delord, J. P., et al. (2020). Efficacy of pembrolizumab in patients with noncolorectal high microsatellite instability/mismatch repair-deficient cancer: results from the phase II KEYNOTE-158 study. J. Clin. Oncol. 38, 1–10. doi:10.1200/JCO.19.02105
Megwalu, U. C., and Sikora, A. G. (2014). Survival outcomes in advanced laryngeal cancer. JAMA Otolaryngol. Head. Neck Surg. 140, 855–860. doi:10.1001/jamaoto.2014.1671
Mezrich, J. D., Fechner, J. H., Zhang, X., Johnson, B. P., Burlingham, W. J., and Bradfield, C. A. (2010). An interaction between kynurenine and the aryl hydrocarbon receptor can generate regulatory T cells. J. Immunol. 185, 3190–3198. doi:10.4049/jimmunol.0903670
Mu, P., Akashi, T., Lu, F., Kishida, S., and Kadomatsu, K. (2017). A novel nuclear complex of DRR1, F-actin and COMMD1 involved in NF-κB degradation and cell growth suppression in neuroblastoma. Oncogene 36, 5745–5756. doi:10.1038/onc.2017.181
Nakajima, H., and Koizumi, K. (2014). Family with sequence similarity 107: a family of stress responsive small proteins with diverse functions in cancer and the nervous system. Biomed. Rep. 2, 321–235.doi:10.3892/br.2014.243
Neavin, D. R., Liu, D., Ray, B., and Weinshilboum, R. M. (2018). The role of the aryl hydrocarbon receptor (AHR) in immune and inflammatory diseases. Int. J. Mol. Sci. 19, 3851. doi:10.3390/ijms19123851
Nocini, R., Molteni, G., Mattiuzzi, C., and Lippi, G. (2020). Updates on larynx cancer epidemiology. Chin. J. Cancer Res. 32, 18–25. doi:10.21147/j.issn.1000-9604.2020.01.03
Obid, R., Redlich, M., and Tomeh, C. (2019). The treatment of laryngeal cancer. Oral Maxillofac. Surg. Clin. North Am. 31, 1–11. doi:10.1016/j.coms.2018.09.001
Overacre-Delgoffe, A. E., Chikina, M., Dadey, R. E., Yano, H., Brunazzi, E. A., Shayan, G., et al. (2017). Interferon-gamma drives T(reg) fragility to promote anti-tumor immunity. Cell 169, 1130–1141.e11. doi:10.1016/j.cell.2017.05.005
Pastuszak-Lewandoska, D., Czarnecka, K. H., Migdalska-Sek, M., Nawrot, E., Domanska, D., Kiszalkiewicz, J., et al. (2015). Decreased FAM107A expression in patients with non-small cell lung cancer. Adv. Exp. Med. Biol. 852, 39–48. doi:10.1007/5584_2014_109
Perez-Castro, L., Garcia, R., Venkateswaran, N., Barnes, S., and Conacci-Sorrell, M. (2023). Tryptophan and its metabolites in normal physiology and cancer etiology. FEBS J. 290, 7–27. doi:10.1111/febs.16245
Platten, M., Friedrich, M., Wainwright, D. A., Panitz, V., and Opitz, C. A. (2021). Tryptophan metabolism in brain tumors - IDO and beyond. Curr. Opin. Immunol. 70, 57–66. doi:10.1016/j.coi.2021.03.005
Prendergast, G. C., Smith, C., Thomas, S., Mandik-Nayak, L., Laury-Kleintop, L., Metz, R., et al. (2014). Indoleamine 2,3-dioxygenase pathways of pathogenic inflammation and immune escape in cancer. Cancer Immunol. Immunother. 63, 721–735. doi:10.1007/s00262-014-1549-4
Qin, Y., Zheng, X., Gao, W., Wang, B., and Wu, Y. (2021). Tumor microenvironment and immune-related therapies of head and neck squamous cell carcinoma. Mol. Therapy-oncolytics 20, 342–351. doi:10.1016/j.omto.2021.01.011
Ribas, A., and Wolchok, J. D. (2018). Cancer immunotherapy using checkpoint blockade. Science 359, 1350–1355. doi:10.1126/science.aar4060
Riess, C., Schneider, B., Kehnscherper, H., Gesche, J., Irmscher, N., Shokraie, F., et al. (2020). Activation of the kynurenine pathway in human malignancies can be suppressed by the cyclin-dependent kinase inhibitor dinaciclib. Front. Immunol. 11, 55. doi:10.3389/fimmu.2020.00055
Rubel, F., Kern, J. S., Technau-Hafsi, K., Uhrich, S., Thoma, K., Hacker, G., et al. (2018). Indoleamine 2,3-dioxygenase expression in primary cutaneous melanoma correlates with breslow thickness and is of significant prognostic value for progression-free survival. J. Invest Dermatol 138, 679–687. doi:10.1016/j.jid.2017.09.036
Sharif Siam, M. K., Sarker, A., and Sayeem, M. M. S. (2021). In silico drug design and molecular docking studies targeting Akt1 (RAC-alpha serine/threonine-protein kinase) and Akt2 (RAC-beta serine/threonine-protein kinase) proteins and investigation of CYP (cytochrome P450) inhibitors against MAOB (monoamine oxidase B) for OSCC (oral squamous cell carcinoma) treatment. J. Biomol. Struct. Dyn. 39 (17), 6467–6479. doi:10.1080/07391102.2020.1802335
Sica, A., and Massarotti, M. (2017). Myeloid suppressor cells in cancer and autoimmunity. J. Autoimmun. 85, 117–125. doi:10.1016/j.jaut.2017.07.010
Siegel, R. L., Miller, K. D., and Jemal, A. (2020). Cancer statistics, 2020. CA Cancer J. Clin. 70, 7–30. doi:10.3322/caac.21590
Steuer, C. E., El-Deiry, M., Parks, J. R., Higgins, K. A., and Saba, N. F. (2017). An update on larynx cancer. CA Cancer J. Clin. 67, 31–50. doi:10.3322/caac.21386
Strojan, P., Haigentz, M., Bradford, C. R., Wolf, G. T., Hartl, D. M., Langendijk, J. A., et al. (2013). Chemoradiotherapy vs. total laryngectomy for primary treatment of advanced laryngeal squamous cell carcinoma. Oral Oncol. 49, 283–286. doi:10.1016/j.oraloncology.2012.11.002
Sung, H., Ferlay, J., Siegel, R. L., Laversanne, M., Soerjomataram, I., Jemal, A., et al. (2021). Global cancer statistics 2020: GLOBOCAN estimates of incidence and mortality worldwide for 36 cancers in 185 countries. CA Cancer J. Clin. 71, 209–249. doi:10.3322/caac.21660
Tanaka, M., Toth, F., Polyak, H., Szabo, A., Mandi, Y., and Vecsei, L. (2021). Immune influencers in action: metabolites and enzymes of the tryptophan-kynurenine metabolic pathway. Biomedicines 9, 734. doi:10.3390/biomedicines9070734
Udali, S., Guarini, P., Ruzzenente, A., Ferrarini, A., Guglielmi, A., Lotto, V., et al. (2015). DNA methylation and gene expression profiles show novel regulatory pathways in hepatocellular carcinoma. Clin. Epigenetics 7, 43. doi:10.1186/s13148-015-0077-1
Vanaja, D. K., Ballman, K. V., Morlan, B. W., Cheville, J. C., Neumann, R. M., Lieber, M. M., et al. (2006). PDLIM4 repression by hypermethylation as a potential biomarker for prostate cancer. Clin. Cancer Res. 12, 1128–1136. doi:10.1158/1078-0432.CCR-05-2072
Volkov, M. V. (1972). Experience in the organization of boarding schools for teaching and treatment of children with diseases of the support-motor apparatus. Vestn. Akad. Med. Nauk. SSSR 27, 48–52.
Wang, Q., Liu, D., Song, P., and Zou, M. H. (2015). Tryptophan-kynurenine pathway is dysregulated in inflammation, and immune activation. Front. Biosci. (Landmark Ed.) 20, 1116–1143. doi:10.2741/4363
Wang, Y., Tiruthani, K., Li, S., Hu, M., Zhong, G., Tang, Y., et al. (2021). mRNA delivery of a bispecific single-domain antibody to polarize tumor-associated macrophages and synergize immunotherapy against liver malignancies. Adv. Mater 33, e2007603. doi:10.1002/adma.202007603
Wolf, D., Sopper, S., Pircher, A., Gastl, G., and Wolf, A. M. (2015). Treg(s) in cancer: friends or foe? J. Cell Physiol. 230, 2598–2605. doi:10.1002/jcp.25016
Wu, M. H., Lin, C. N., Chiu, D. T., and Chen, S. T. (2020). Kynurenine/tryptophan ratio predicts angiotensin receptor blocker responsiveness in patients with diabetic kidney disease. Diagn. (Basel) 10, 207. doi:10.3390/diagnostics10040207
Xiao, Y., and Freeman, G. J. (2015). A new B7:CD28 family checkpoint target for cancer immunotherapy: HHLA2. Clin. Cancer Res. 21, 2201–2203. doi:10.1158/1078-0432.CCR-14-2658
Xu, G., Shi, Y., Ling, X., Wang, D., Liu, Y., Lu, H., et al. (2021b). HHLA2 predicts better survival and exhibits inhibited proliferation in epithelial ovarian cancer. Cancer Cell Int. 21, 252. doi:10.1186/s12935-021-01930-y
Xu, Q., Chen, S., Hu, Y., and Huang, W. (2021a). Landscape of immune microenvironment under immune cell infiltration pattern in breast cancer. Front. Immunol. 12, 711433. doi:10.3389/fimmu.2021.711433
Xue, C., Gu, X., Zhao, Y., Jia, J., Zheng, Q., Su, Y., et al. (2022). Prediction of hepatocellular carcinoma prognosis and immunotherapeutic effects based on tryptophan metabolism-related genes. Cancer Cell Int. 22, 308. doi:10.1186/s12935-022-02730-8
Yuan, M., Pei, J., Li, R., Tian, L., He, X., and Li, Y. (2021). CD40LG as a prognostic molecular marker regulates tumor microenvironment through immune process in breast cancer. Int. J. Gen. Med. 14, 8833–8846. doi:10.2147/IJGM.S336813
Zhai, L., Ladomersky, E., Lenzen, A., Nguyen, B., Patel, R., Lauing, K. L., et al. (2018). IDO1 in cancer: a gemini of immune checkpoints. Cell Mol. Immunol. 15, 447–457. doi:10.1038/cmi.2017.143
Zhang, H., and Fu, L. (2021). The role of ALDH2 in tumorigenesis and tumor progression: targeting ALDH2 as a potential cancer treatment. Acta Pharm. Sin. B 11 (6), 1400–1411. doi:10.1016/j.apsb.2021.02.008
Zhang, H., Li, J., and Zhou, Q. (2022). Prognostic role of indoleamine 2,3-dioxygenase 1 expression in solid tumors: a systematic review and meta-analysis. Front. Oncol. 12, 954495. doi:10.3389/fonc.2022.954495
Zhang, H., Meltzer, P., and Davis, S. (2013). RCircos: an R package for circos 2D track plots. BMC Bioinforma. 14, 244. doi:10.1186/1471-2105-14-244
Zhang, M., Zhu, K., Pu, H., Wang, Z., Zhao, H., Zhang, J., et al. (2019). An immune-related signature predicts survival in patients with lung adenocarcinoma. Front. Oncol. 9, 1314. doi:10.3389/fonc.2019.01314
Zhang, X., Hou, H., Chen, H., Liu, Y., Wang, A., and Hu, Q. (2018). Serum metabolomics of laryngeal cancer based on liquid chromatography coupled with quadrupole time-of-flight mass spectrometry. Biomed. Chromatogr. 32, e4181. doi:10.1002/bmc.4181
Zheng, Y., Wen, Y., Cao, H., Gu, Y., Yan, L., Wang, Y., et al. (2021). Global characterization of immune infiltration in clear cell renal cell carcinoma. Onco Targets Ther. 14, 2085–2100. doi:10.2147/OTT.S282763
Zhou, Q. H., Li, K. W., Chen, X., He, H. X., Peng, S. M., Peng, S. R., et al. (2020). HHLA2 and PD-L1 co-expression predicts poor prognosis in patients with clear cell renal cell carcinoma. J. Immunother. Cancer 8, e000157. doi:10.1136/jitc-2019-000157
Zhu, Y., Yao, S., Iliopoulou, B. P., Han, X., Augustine, M. M., Xu, H., et al. (2013). B7-H5 costimulates human T cells via CD28H. Nat. Commun. 4, 2043. doi:10.1038/ncomms3043
Keywords: tryptophan metabolism, laryngeal squamous cell carcinoma, bioinformatic, risk model, immune
Citation: Liu F, Qin Y, Luo W, Ruan X, Lu L, Feng B and Yu J (2025) Construction of a risk model associated with tryptophan metabolism and identification of related molecular subtypes in laryngeal squamous cell carcinoma. Front. Genet. 16:1530334. doi: 10.3389/fgene.2025.1530334
Received: 18 November 2024; Accepted: 07 March 2025;
Published: 24 March 2025.
Edited by:
Dakui Luo, Fudan University, ChinaReviewed by:
Xutong Xue, Boston Children’s Hospital, Harvard Medical School, United StatesCopyright © 2025 Liu, Qin, Luo, Ruan, Lu, Feng and Yu. This is an open-access article distributed under the terms of the Creative Commons Attribution License (CC BY). The use, distribution or reproduction in other forums is permitted, provided the original author(s) and the copyright owner(s) are credited and that the original publication in this journal is cited, in accordance with accepted academic practice. No use, distribution or reproduction is permitted which does not comply with these terms.
*Correspondence: Jianfei Yu, eXVqaWFuZmVpQHN4bXUuZWR1LmNu
†These authors have contributed equally to this work and share first authorship
Disclaimer: All claims expressed in this article are solely those of the authors and do not necessarily represent those of their affiliated organizations, or those of the publisher, the editors and the reviewers. Any product that may be evaluated in this article or claim that may be made by its manufacturer is not guaranteed or endorsed by the publisher.
Research integrity at Frontiers
Learn more about the work of our research integrity team to safeguard the quality of each article we publish.