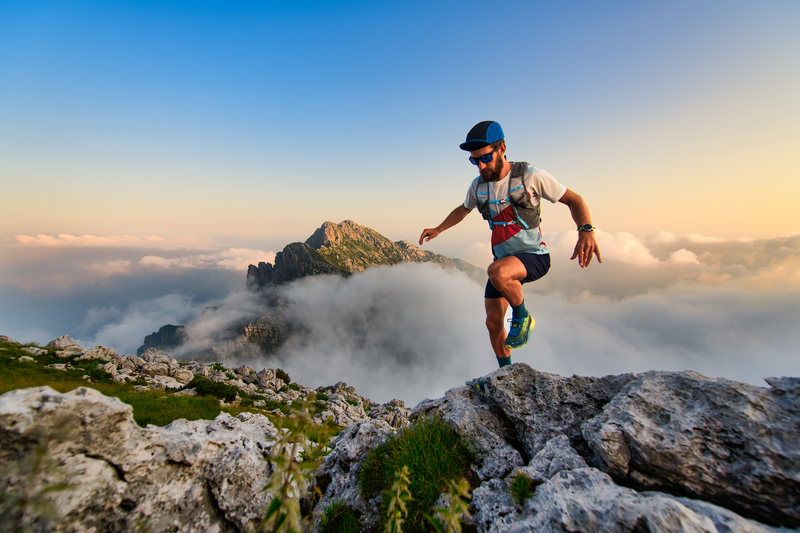
94% of researchers rate our articles as excellent or good
Learn more about the work of our research integrity team to safeguard the quality of each article we publish.
Find out more
ORIGINAL RESEARCH article
Front. Genet.
Sec. Computational Genomics
Volume 16 - 2025 | doi: 10.3389/fgene.2025.1528810
The final, formatted version of the article will be published soon.
You have multiple emails registered with Frontiers:
Please enter your email address:
If you already have an account, please login
You don't have a Frontiers account ? You can register here
Microarray gene expression data have emerged as powerful tools in cancer classification and diagnosis. However, the high dimensionality of these datasets presents significant challenges for feature selection, leading to the development of various computational methods. In this paper, we utilized the Eagle Prey Optimization (EPO), a novel genetically inspired approach for microarray gene selection in cancer classification. EPO draws inspiration from the remarkable hunting strategies of eagles, which exhibit unparalleled precision and efficiency in capturing prey. Similarly, our algorithm aims to identify a small subset of informative genes that can discriminate between cancer subtypes with high accuracy and minimal redundancy. To achieve this, EPO employs a combination of genetic mutation operator with EPO fitness function, to evolve a population of potential gene subsets over multiple generations. The key innovation of EPO lies in its incorporation of a fitness function specifically designed for cancer classification tasks. This function considers not only the discriminative power of selected genes but also their diversity and redundancy, ensuring the creation of compact and informative gene subsets.Moreover, EPO incorporates a mechanism for adaptive mutation rates, allowing the algorithm to explore the search space efficiently. To validate the effectiveness of EPO, extensive experiments were conducted on several publicly available microarray datasets representing different cancer types. Comparative analysis with state-of-the-art gene selection algorithms demonstrates that EPO consistently outperforms these methods in terms of classification accuracy, dimensionality reduction, and robustness to noise.
Keywords: Feature optimization, Microarray gene selection, Cancer classification, Meta-heuristic optimization, Feature Selection
Received: 19 Nov 2024; Accepted: 20 Feb 2025.
Copyright: © 2025 Dhamercherla, Reddy Edla and Dara. This is an open-access article distributed under the terms of the Creative Commons Attribution License (CC BY). The use, distribution or reproduction in other forums is permitted, provided the original author(s) or licensor are credited and that the original publication in this journal is cited, in accordance with accepted academic practice. No use, distribution or reproduction is permitted which does not comply with these terms.
* Correspondence:
Suresh Dara, VIT-AP University, Amaravati, India
Disclaimer: All claims expressed in this article are solely those of the authors and do not necessarily represent those of their affiliated organizations, or those of the publisher, the editors and the reviewers. Any product that may be evaluated in this article or claim that may be made by its manufacturer is not guaranteed or endorsed by the publisher.
Research integrity at Frontiers
Learn more about the work of our research integrity team to safeguard the quality of each article we publish.