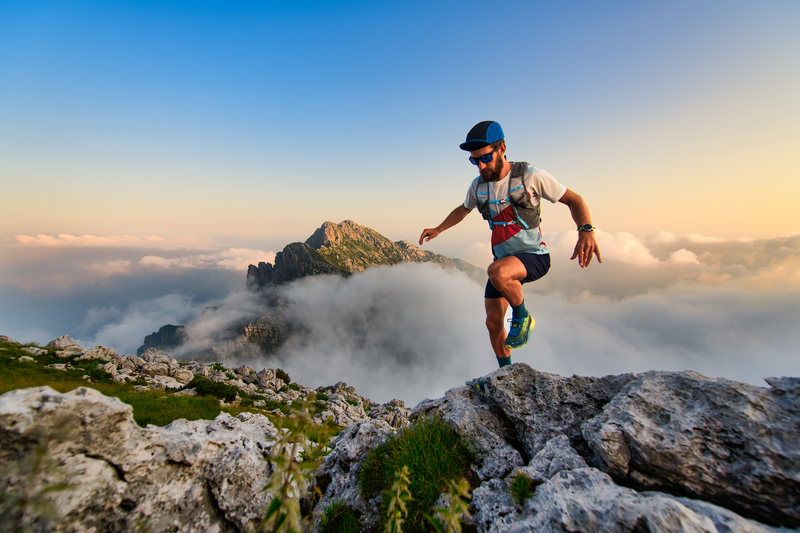
95% of researchers rate our articles as excellent or good
Learn more about the work of our research integrity team to safeguard the quality of each article we publish.
Find out more
TECHNOLOGY AND CODE article
Front. Genet.
Sec. Computational Genomics
Volume 16 - 2025 | doi: 10.3389/fgene.2025.1523015
The final, formatted version of the article will be published soon.
You have multiple emails registered with Frontiers:
Please enter your email address:
If you already have an account, please login
You don't have a Frontiers account ? You can register here
Breast cancer is one of the most common malignancies among women globally, with its incidence rate continuously increasing, posing a serious threat to women's health. Although current treatments, such as drugs targeting estrogen receptor alpha (ERα), have extended patient survival, issues such as drug resistance and severe side effects remain widespread. This study proposes a machine learning-based optimization model for anti-breast cancer candidate drugs, aimed at enhancing biological activity and optimizing ADMET (Absorption, Distribution, Metabolism, Excretion, Toxicity) properties through multi-objective optimization. Initially, grey relational analysis and Spearman correlation analysis were performed on the molecular descriptors of 1,974 compounds, identifying 91 key descriptors. A Random Forest model combined with Shapley Additive Explanations (SHAP) values was then used to further select the top 20 descriptors with the greatest impact on biological activity. The constructed Quantitative Structure-Activity Relationship (QSAR) model, using algorithms such as LightGBM, Random Forest, and XGBoost, achieved an R² value of 0.743 for biological activity prediction, demonstrating strong predictive performance. Additionally, a multi-model fusion strategy and Particle Swarm Optimization (PSO) algorithm were employed to optimize both biological activity and ADMET properties, thereby improving the prediction of Caco-2, CYP3A4, hERG, HOB, and MN properties. For example, the best model for predicting Caco-2 achieved an F1 score of 0.8905, while the model for predicting CYP3A4 reached an F1 score of 0.9733. This multiobjective optimization model provides a novel and efficient tool for drug development, offering significant improvements in both biological activity and pharmacokinetic properties, with practical implications for the optimization of future anti-breast cancer drugs.
Keywords: Breast cancer1, Machine Learning2, Quantitative Structure-Activity Relationship Models(QSAR)3, Particle Swarm Optimization(PSO)4, ADMET Properties5, drug screening6, Biological Activity7
Received: 05 Nov 2024; Accepted: 31 Mar 2025.
Copyright: © 2025 Dong, Chen, Yang and Hao. This is an open-access article distributed under the terms of the Creative Commons Attribution License (CC BY). The use, distribution or reproduction in other forums is permitted, provided the original author(s) or licensor are credited and that the original publication in this journal is cited, in accordance with accepted academic practice. No use, distribution or reproduction is permitted which does not comply with these terms.
* Correspondence:
Zhou Dong, School of Information Engineering, Xi'an Eurasia University, Xi'an, China
Disclaimer: All claims expressed in this article are solely those of the authors and do not necessarily represent those of their affiliated organizations, or those of the publisher, the editors and the reviewers. Any product that may be evaluated in this article or claim that may be made by its manufacturer is not guaranteed or endorsed by the publisher.
Research integrity at Frontiers
Learn more about the work of our research integrity team to safeguard the quality of each article we publish.