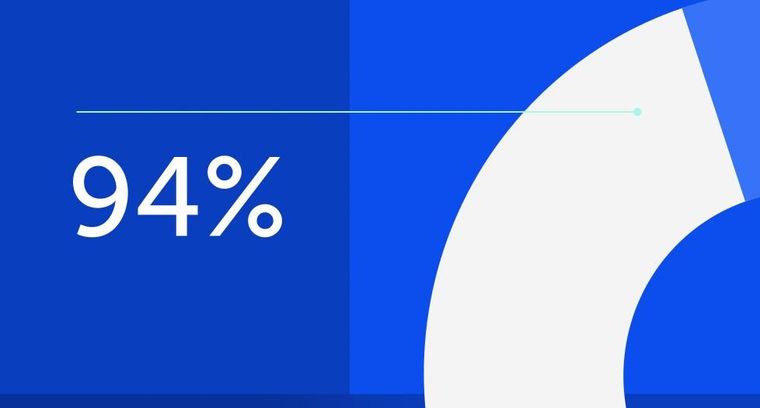
94% of researchers rate our articles as excellent or good
Learn more about the work of our research integrity team to safeguard the quality of each article we publish.
Find out more
ORIGINAL RESEARCH article
Front. Genet., 02 April 2025
Sec. Behavioral and Psychiatric Genetics
Volume 16 - 2025 | https://doi.org/10.3389/fgene.2025.1522729
This article is part of the Research TopicInsights in Behavioral and Psychiatric GeneticsView all articles
Introduction: The conventional approach to estimating heritability in twin studies implicitly assumes either the absence of measurement error or that any measurement error is incorporated into the nonshared environment component. However, this assumption can be problematic when it does not hold or when measurement error cannot be reasonably classified as part of the nonshared environment.
Methods: In this study, we demonstrate the need for improvement in the conventional structural equation modeling (SEM) used for estimating heritability when applied to trait data with measurement errors. The critical issue revolves around an assumption concerning measurement errors in twin studies. In cases where traits are measured using samples, data is aggregated during preprocessing, with only a centrality measure (e.g., mean) being used for modeling. Additionally, measurement errors resulting from sampling are assumed to be part of the nonshared environment and are thus overlooked in heritability estimation. Consequently, the presence of intra-individual variability remains concealed. Moreover, recommended sample sizes are typically based on the assumption of no measurement errors.
Results: We argue that measurement errors in the form of intra-individual variability are an intrinsic limitation of finite sampling and should not be considered as part of the nonshared environment. Previous studies have shown that the intra-individual variability of psychometric effects is significantly larger than the inter-individual counterpart. Here, to demonstrate the appropriateness and advantages of our hierarchical linear modeling approach in heritability estimation, we utilize simulations as well as a real dataset from the ABCD (Adolescent Brain Cognitive Development) study. Moreover, we showcase the following analytical insights for data containing non-negligible measurement errors: i) The conventional SEM may underestimate heritability. ii) A hierarchical model provides a more accurate assessment of heritability. iii) Large samples, exceeding 100 observations or thousands of twins, may be necessary to reduce imprecision.
Discussion: Our study highlights the impact of measurement error on heritability estimation and introduces a hierarchical model as a more accurate alternative. These findings have significant implications for understanding individual differences and improving the design and analysis of twin studies.
As an indication of potential predictability, heritability is an important concept in assessing individual differences. As the proportion of trait variability ascribed to genetics, heritability offers a unique perspective for quantifying the role of genetics in complex traits (Downes and Turkheimer, 2022; Robette et al., 2022). Twins provide a hypothetically well-controlled scenario where genetics, environment, and their interaction can be statistically separated and apportioned.
Conventional twin studies are typically conceptualized with three hierarchies of data structure: individual, family, and zygosity. The individual measures are nested within families, which are further categorized as either monozygotic (MZ) or dizygotic (DZ) twins. A model can be formulated for a quantitative trait of interest that is measured at the individual level. In the popular ACE formulation (Maes, 2005; Downes and Matthews, 2020; Hunter, 2021), the trait data
Each pair of parentheses indicates a nesting structure among the subscripts. The intercept
The variances associated with the three latent components are crucial parameters in twin studies. One may make the following assumptions for two twins
The relatedness
Table 1. A reference table of major variables and parameters used with heritability modeling. Quantities which originate in hierarchical linear modeling (HLM) and structural equation modeling (SEM) are noted explicitly.
A crucial feature of the Model 2 is the inclusion of the homogeneity assumption. This assumption is necessary when estimating variances within the model framework in (Model 2). Six variances, namely
The assumption effectively reduces the number of variance parameters by half. However, this also leads to having homogeneity of total variance between MZ and DZ:
The heritability in a twin study can be defined under the homogeneity assumption (Equation 3). The classical methodology is to adopt structural equation modeling (SEM) (e.g., Rijsdijk and Sham, 2002; Holst et al., 2016; Neale et al., 2016; Bates et al., 2019) to estimate the three variances. Then, the three proportions of total variability attributed to additive genetic effects, common environment, and nonshared environment are expressed respectively as,
Effect decomposition under the SEM (Model 2) and the associated estimation of heritability can be visually represented as a path diagram (Figure 1), which is analogous to a directed acyclic diagram in causal inference. The values of
Figure 1. Path diagram (reticular action model) for the ACE formulation
The three variability proportions of
The expression (Equation 5) leads to Falconer’s formula (Falconer and MacKay, 1996):
We address the challenges of heritability estimation from the perspectives of accuracy and precision, which are typically the objectives of studies to maximize. Here, we define the accuracy of an estimate as the absence of systematic biases. Inaccuracy, for example, implies an upward or downward shift in a point estimate of effect centrality (e.g., mean, mode, median), an uncertainty interval, or a posterior distribution. On the other hand, we define imprecision as the extent of uncertainty in an estimate, which can be quantified as the standard error or an uncertainty interval.
An implicit assumption is present within the conventional SEM regarding measurement errors, the random or stochastic fluctuations in a measurement from one instance to another. Specifically, the SEM formulation assumes one of two possibilities: 1) the absence of measurement errors in the phenotypic data
Measurement errors are not a significant concern for many measures, such as physical traits that can typically be assessed with high precision. We focus here on heritability estimation in situations where measurement errors are not negligible. For example, in a Stroop task where a large number of trials (e.g., 100) are presented in the experiment for each congruent and incongruent condition. To utilize the SEM formulation directly, the data are typically aggregated, and a centrality measure is used as input.
Data aggregation is a common practice in heritability estimation. Examples can be found in the fields of psychometrics (e.g., Smith et al., 2023; Rea-Sandin et al., 2023; Hung et al., 2023; Gustavson et al., 2023; Vellani et al., 2022; Viktorsson et al., 2022; Yeom et al., 2022; Wang et al., 2020; Routledge et al., 2018; Stins et al., 2005; Schachar et al., 2010; Fan et al., 2001) and neuroimaging (Kastrati et al., 2022; van Drunen et al., 2021; Chen et al., 2019; Harper et al., 2019; van der Meulen et al., 2018; Anokhin et al., 2017; Blokland et al., 2011; Matthews et al., 2007; Polk et al., 2007). However, data aggregation can be a double-edged sword, as valuable information can be lost. The impact of ignoring intra-individual variability has been explored in different contexts. For instance, the issue can be traced back to Spearman (1904) who pointed out the underestimation problem in estimating the correlation between two variables when measurement errors occur. It has also been recently shown that, without proper accountability, test-retest reliability can be substantially underestimated (Rouder and Haaf, 2019; Haines et al., 2020; Chen et al., 2021). Even in heritability estimation, underestimation has been revealed in item response theory due to the adoption of the aggregation process in the form of sum–scores (van den Berg et al., 2007; Schwabe et al., 2019). Recently, the bias problem due to measurement errors has been investigated regarding the reliability of polygenic score and that of a phenotypic trait framed under SEM (Pingault et al., 2022).
Here, we employ a hierarchical linear modeling (HLM) approach to account for intra-individual variability. We propose that measurement errors should not be considered part of the nonshared environment component, but rather partitioned appropriately within the model hierarchy. In addition, we employ the HLM approach to reexamine the conventional SEM framework, with the latter being a special case of the former. Specifically, we demonstrate that the common practice of data aggregation fails to adequately address the impact of intra-individual variability. With simulations and real datasets, we address the following questions:
1) Does disregarding intra-individual variability result in biased estimation? If so, to what extent?
2) To what degree does intra-individual variability contribute to precision in heritability estimation?
3) Are typical twin study sample sizes sufficient to achieve a proper precision of heritability estimation?
It is important to note that SEM has been extended to accommodate complex hierarchical data structures (e.g., Mehta and Neale, 2005). Although the SEM framework could potentially be applied to our work, we have opted for HLM due to our preference and familiarity. For clarity, our model comparisons are based on the conventional SEM approach for heritability estimation, not on SEM’s extended capabilities.
A hierarchical model partitions data variability by mapping the stratified structure to effects at various levels. The modeling approach is well-established in behavior genetics. For example, van den Oord (2001) proposed using HLM to characterize latent genetic and environmental components of variance in extended families. Guo and Wang (2002) derived heritability estimation through direct variance decomposition. McArdle and Prescott (2005) demonstrated the equivalence between conventional SEM and the variance decomposition approach. Hunter (2021) extended this approach to multiple phenotypes, exploring the feasibility of handling nested data and repeated measures. However, the literature lacks a discussion on how to incorporate intra-individual variability.
We construct the following model by decomposing the data
Similar to Model 5, the correlation between any two twins,
The HLM Formulation 7 has been proposed and explored in previous studies in contexts where intra-individual variability is absent (e.g., van den Oord, 2001; Guo and Wang, 2002; McArdle and Prescott, 2005). Here, we aim to extend the framework to accommodate cases where intra-individual variability is present. We highlight three advantages regarding the HLM framework. First, the conventional SEM Formulation 2 assumes the homogeneity of variances (Equation 3), which leads to total variance homogeneity between the two zygosities,
The equivalence between the two modeling approaches can be established when we equate the total variance of
The HLM (Formulation 7) does not assume variance homogeneity. Instead, a less stringent assumption–proportionality homogeneity across zygosities–is made when using Falconer’s Formula 6: the variance proportions accounted for by genetic and shared environmental effects –
Second, the HLM formulation has the flexibility to accommodate different distribution types. As shown in the Model 7, the individual- and family-level variances in the two Gaussian distributions
We utilized a publicly available dataset of body mass index (BMI) from the R package mets (Holst et al., 2016) to validate the HLM approach. Despite having only one BMI measurement per individual, the presence of intra-individual variability can be considered negligible. In summary, the BMI data comprised
Heritability estimation for the BMI dataset was performed using SEM with the following specifications. Alongside the three latent components
For the HLM approach, we adopted the model (Formulation 7) with log-normal and Gaussian density for the individual- and family-level distributions, respectively based on the tendency of the BMI data skewed to the right (Figure 2A). The following covariates were included: zygosity and nonlinear age effect for each sex using smooth splines with thin plate bases. The model was implemented under the Bayesian framework using the R package brms (Bürkner, 2017). The resulting
Figure 2. (A) Data distribution. BMI exhibits a right-skewed distribution, with slightly greater dispersion among DZ twins compared to MZ twins. (B) Heritability estimation of BMI data. The proportions of BMI variability attributed to each of the three components are depicted. The distributions for
Within the hierarchical framework, we will employ simulations to systematically investigate the influence of intra-individual variability, trial and participant sample sizes on precision, and determine the necessary sample sizes to achieve a satisfactory level of precision. The insights obtained from these simulations will be further validated by applying the HLM framework to a behavioral dataset.
Measurement errors have traditionally been regarded as part of the nonshared environment component in the heritability model, either implicitly or explicitly (e.g., Maes, 2005; Germine et al., 2015). In other words, it has been considered appropriate to include any measurement errors in the trait measurement
We contend that an ideal modeling approach should appropriately allocate measurement errors rather than grouping them together with the nonshared environment. Suppose that the observation
Thus, the sample mean
Measurement errors can be appropriately accounted for as a separate component from the nonshared environment. Suppose we consider the individual-level trait effect
Figure 3. Path diagram (reticular action model) for the augmented SEM (Formulation 11) and the HLM (Formulation 12). Subscript indices for family
The same distribution assumptions in Model 2 apply to the three latent effects of A, C, and E here. Solving this augmented SEM (Formulation 11) directly is challenging. However, in Section 3.4, we will present an approximate approach to heritability estimation.
Measurement errors can also be incorporated into the HLM framework. In particular, we augment the HLM Formulation 7 to
When the cross-trial sampling variance
Framing the presence of measurement errors as a distinction between observed and latent effects helps in understanding the associated impact. As illustrated in the path diagram (Figure 3), one can estimate heritability directly based on the correlations
We begin by extending the HLM (Equation 7) to a case where data are collected at the observation level with repeated measures through trials under a single task condition. The data
The corresponding path diagram is shown in Figure 4. The Model 13 can be extended to include various distributions. For instance, the trial-level effects can be characterized through Bernoulli, gamma, and Poisson distributions, which allow for modeling different types of data (count, binary, skewed).
Figure 4. Path diagram (reticular action model) for the HLM Formulation 13. Subscript indices for family
Heritability can be estimated using the HLM (Formulation 13) for trial-level data as follows. Similar to the situation with HLM for the conventional SEM in (Formulation 7), we compute the correlations between two twins within a family using the inter-individual and inter-family variances,
Now we examine the common practice of data aggregation in light of the HLM (Formulation 13). To capture the crucial role of intra-individual variability
The variability ratio
When the trial-level data
In comparing the model (Formulation 15) with aggregated data to its counterpart (Formulation 7) for data without measurement errors, we note that ignoring intra-individual variability leads to its combination with the inter-individual variance
where the introduced bias into
The parameter
One direct way to view the distinction between SEM and HLM is to compare their respective path diagrams (Figures 3, 4). HLM preserves the hierarchical structure and cross-trial variability, ensuring this information propagates across other hierarchical levels (Model 13). In contrast, SEM obscures this variability through data aggregation, compromising the hierarchical integrity. This loss of data structure fidelity in SEM leads to biased underestimation of heritability, as captured through the parameter
The biases induced in the SEM formulation can be theoretically corrected by introducing an adjustment term in the denominator of (Formula 16) to counteract the contaminating effect of
Similarly, decontamination can be achieved for the Formulas 4, 8. However, these adjustments rely on the availability of the intra-individual variance
Similarly, as
These approximate adjustments (Formulas 18, 19) offer a solution to the augmented SEM Formulation 11 and its hierarchical counterpart (Model 12). We will further explore and validate their effectiveness as approximate adjustments later with an experimental dataset.
An intriguing aspect of biased estimation for heritability is its analogy to the phenomenon of correlation attenuation in the presence of measurement errors. Spearman (1904) recognized the problem of bias caused by measurement errors and proposed an adjustment method to disattenuate the correlation between two variables. In Formula 16, the term
We now extend the HLM (Formulation 13) to accommodate two task conditions. In fields such as psychometrics and neuroimaging, the focus often lies in comparing and analyzing the contrast between two conditions. We expand the previous HLM (Formulation 13) to one with hierarchical levels using five indices: family
The differences from Section 2.2 are twofold: (a) the presence of two intercepts,
Heritability estimation is straightforward for each condition under the HLM (Formulation 20). First, we calculate the correlation between two twins within a family for condition
Then, we estimate heritability for each condition by plugging
Two approaches are available for estimating heritability and the variability ratio for the contrast between two conditions. One approach involves reparameterizing the model (Formulation 20) through an indicator variable using effect coding for the two conditions,
Alternatively, within the Bayesian framework, one can directly obtain the distribution of the contrast by formulating it based on each condition’s posterior draws from the HLM (Formulation 20).
Biased estimation due to data aggregation and its adjustment in the previous subsection also apply to the case with two conditions. For each condition and their contrast, the variability ratio
We have shown that, by closely reflecting the actual data structure, an HLM framework appropriately accounts for different sources of data variability. However, the precision of heritability estimation remains unclear, as there is no analytical quantification available. Simulations were conducted to assess the precision of heritability estimation from the following aspects regarding the impact of intra-individual variability that the analytical approach cannot easily reveal: 1) the precision of heritability estimation, and 2) the requirement of family and trial sample sizes in twin studies.
Detailed information about the simulations and results can be found in Supplementary Appendix A. As expected, HLM showed no bias in heritability estimation, whereas biases under the SEM framework become more pronounced as the variability ratio
We apply the HLM approach to an experimental dataset to address two primary questions. First, do the insights gained from numerical simulations in the previous section align with the findings when real data is analyzed? Second, what is the range of the relative magnitude of intra-individual variability, as indicated by the ratio
We utilize a behavioral dataset obtained from an experiment conducted as part of the ABCD study. The experiment investigated selective attention during adolescence using an emotional Stroop task (Smolker et al., 2022), with reaction time (RT) as a phenotypic trait. The data was collected during the 1-year follow-up visit and is publicly accessible through the 2020 ABCD Annual Curated Data Release 4.0 (https://nda.nih.gov/study.html?id=1299). The analysis scripts can be found online at: https://github.com/afni/apaper_heritability.
A subset of the original dataset, specifically containing twins, was utilized for the analysis. Refer to Table 2 for detailed information on participant counts and demographic data. The subset consisted of 1,102 twins (including some triplets) from 555 families and was selected from a larger dataset of 11,876 participants (Iacono et al., 2018). Among the included twins, there were 461 MZ and 641 DZ participants.
We focus on the RT data for estimating heritability. The RT was measured for two levels of congruency in a Stroop task: congruent and incongruent. Each participant was instructed to respond to a total of 48 trials, consisting of 24 congruent and 24 incongruent trials. The response window for each trial was set to 2,000 ms. Among 1,102 twins, a total of 49,524 trials were included in the analysis after excluding incorrect responses. This resulted in an overall correct response rate of approximately 93.6%. The distribution of RTs is heavily right-skewed (Figure 5), with a mode of 962 ms and a 95% highest density interval ranging from 634 to 1,827 ms.
Figure 5. Reaction time (RT) distributions. The shaded area under each density represents the 95% highest density interval, while the vertical dashed line indicates the mode. The RT distribution is 1) right-skewed, 2) slightly right-shifted under the incongruent condition compared to the congruent condition, and 3) slightly more dispersed for DZ twins than MZ twins.
The RT data was analyzed using two different approaches: HLM and SEM. For HLM, the trial-level data was fitted using the Formulation 20. Site, sex, race, age, and zygosity were included as covariates. To account for the right-skewness (Figure 5), a log-normal distribution was used for the trial-level effects. The Bayesian framework was employed to implement the HLM approach, utilizing the brms package in R (Bürkner, 2017). Heritabilty was estimated for each of the three RT effects: the congruent condition, incongruent condition, and the Stroop effect (the contrast between incongruent and congruent conditions). The SEM was applied with aggregated data. Specifically, RT was aggregated across trials for each condition at the individual level. Similar to the HLM approach, covariates including site, sex, race, age, and zygosity were included. The SEM formulation was implemented using the R packages of mets and umx. The computational time for SEM with aggregated data was negligible. In contrast, HLM-based estimation, using Markov chain Monte Carlo simulations, required 3.5 hours with four chains and 12 threads per chain. These computations were performed on an Intel Server S2600WFT equipped with 96 CPUs running at 2,933 MHz.
The estimation results are presented in Figure 6. The performance of HLM relative to SEM, as summarized below, aligns with our simulations in Section 3.6. Overall, both SEM and HLM exhibited a significant amount of uncertainty in estimating heritability for both conditions. SEM displayed noticeable underestimation of
Figure 6. Estimated heritability for RT data. (A) The three columns represent the two conditions (congruent and incongruent), as well as their contrast, with the corresponding variability ratio
Below are a few detailed elaborations:
1) Impact of relative intra-individual variability on estimation precision. First, under each of the individual congruent and incongruent conditions, the observed intra-individual variability was not large
2) Impact of relative intra-individual variability on estimation bias. Under both conditions, the intra-individual variability is moderate
3) Impact of relative intra-individual variability on sample sizes. The larger
4) Bias adjustment for SEM estimates. The biases under the SEM framework, due to data aggregation, adjusted using Formula 18, were reduced. The adjusted estimates for
We also explored the HLM approach for the ABCD-Stroop data using a conventional linear mixed-effects modeling framework instead of a Bayesian approach. The lmer function from the lme4 package in R (Bates et al., 2015) was utilized to fit the models (Formulations 13, 20) with RT log-transformed. Although the point estimates (not shown here) for
Heritability estimation based on data with a non-negligible intra-individual variability necessitates a model that accurately represents the underlying hierarchical structure. When assessing a phenotypic trait with repeated measures, we propose a hierarchical model that encompasses all relevant levels, allowing for the incorporation, propagation, and separation of intra-individual measurement error from parameter estimation at higher levels. Through numerical simulations and a real behavioral dataset, we have demonstrated a few advantages of HLM. These advantages include: 1) avoidance of estimation bias, 2) the ability to account for the significant influence of intra-individual variability on heritability estimation, and 3) enhanced interpretability and explanatory power of results, such as identifying the challenges associated with reducing estimation uncertainty due to sample size limitations.
Data reduction through aggregation is a commonly used in statistical applications, particularly in studying individual differences. Even though intraindividual variability has long been recognized (Fiske and Rice, 1955), many classical frameworks have been applied in contexts where measurement errors are minimal or nonexistent. For example, intraclass correlation (Fisher, 1954) for test-retest reliability in individual differences is typically assessed without considering intra-individual variability. A similar situation can be observed in heritability estimation: to date, common modeling approaches do not explicitly account for the level of measurement units (e.g., individual trials), and instead they simplify the data through preliminary aggregation steps such as averaging.
Can data aggregation be justified by attributing intraindividual variability to the nonshared environment (the
In heritability estimation, path diagrams are commonly used to depict causal relationships among variables. Within this framework, a latent trait or condition is conceptualized as a higher-level theoretical construct that causally influences each individual measurement or trial (see Figure 4). In other words, individual measurements are specific realizations determined by the underlying latent construct. Crucially, heritability is defined at the level of this latent construct, not at the level of single measurements.
A hierarchical modeling framework more accurately maps the causal structure outlined in the path diagram. In contrast, conventional SEM, which treats intraindividual variability as residual errors, does not fully align with the causal structure and can lead to underestimated heritability, as demonstrated in Section 3.3. This underscores the necessity of explicitly modeling hierarchical data structures to account for both between- and within-individual variability.
Our empirical evidence here from both a real dataset and simulations demonstrates that adopting an HLM framework—which respects the full hierarchical structure of the data—yields more accurate heritability estimates than the conventional SEM for psychometric traits. When intraindividual variability is minimal—as is often the case with many physical traits—the conventional SEM can be seen as a special asymptotic case of a more general HLM, and aggregation is justified because the impact of such variability is negligible
To recapitulate, the HLM approach acknowledges that heritability is defined at the latent trait level and avoids biases associated with oversimplified data aggregation. While data aggregation may be acceptable for traits with minimal intraindividual variability, a hierarchical modeling approach that directly incorporates the causal structure of the data is essential for accurately estimating heritability when variability is pronounced.
To improve the accuracy of heritability estimation, we recommend adopting HLM in the presence of intra-individual variability. It is important to note that the HLM framework is not mutually exclusive with SEM, on which SEM and other methods such as common pathway model are based. As the path diagrams in Figures 1, 4 illustrate, both frameworks are conceptually consistent, as discussed in Section 2.2. Nevertheless, we emphasize that the broader framework of HLM combined with the Bayesian approach offers several advantages:
1) It supports a wider range of numerically implemented distributions (e.g., Student’s
2) It integrates uncertainty assessment into a single process.
3) It robustly handles variance-covariance structures.
While the last point is important for theoretical and interpretational reasons, it also has useful practical benefits. In the commonly-used R software packages, there are severe challenges faced when using methods implemented in the nlme and lme4 packages, which can struggle with numerical singularities when correlations (or variances) approach boundary values such as −1, 1, or 0, as encountered in this Stroop dataset. The proposed framework avoids these difficulties.
There are two aspects of accuracy compromise that need to be considered in heritability estimation. The first aspect pertains to estimation biases. As demonstrated in this study, failure to fully incorporate the data structure can lead to biased estimates of heritability. The second aspect concerns the uncertainty in heritability estimation. In addition to providing a point estimate for the effect of interest, it is equally important to quantify its uncertainty, characterized through measures such as standard error, an uncertainty interval (e.g., 95%), or even a full distribution (as depicted in Figure 6). However, uncertainty is often not well emphasized in common practice. In some cases, only the central tendency (e.g., mean) of heritability estimation is reported. However, to truly comprehend the generalizability of results, understanding uncertainty is crucial. One of the benefits of the HLM framework is its ability to directly generate posterior distributions that illustrate estimate precision.
In the presence of intra-individual variability, one might be tempted to adopt the bias adjustment approach using the conventional SEM. Our findings demonstrate that the biases resulting from data aggregation can be mitigated to some extent if variability can be determined separately (e.g., through repeated measures), as indicated by Formula 18 or Formula 19. However, in practical applications, these adjustments are suboptimal due to distributional deviations, as demonstrated in our example using the Stroop dataset. Furthermore, an effective adjustment for biases in uncertainty assessment is currently lacking. Hence, a comprehensive HLM framework remains the preferred choice.
Sample sizes remain a challenge in twin studies. The dataset we used for demonstration, exemplifying a cognitive inhibition study, suggests that reasonable levels of uncertainty can be achieved with sample sizes of less than 1,000 families and less than 100 trials per individual condition (congruent and incongruent). The estimated heritability of approximately 40% (first two columns, Figure 6) aligns with the general range observed in typical phenotypic traits in the literature (Polderman et al., 2015). However, the contrast between conditions is often the focal point of interest. Even with HLM estimation, the uncertainty of heritability for this contrast remains unresolved (third column, Figure 6), creating imprecision regarding its magnitude. In other words, despite attempts by the Consortium (Iacono et al., 2018; Smolker et al., 2022) to address the sample size issue, the dataset from the ABCD Study (consisting of 461 MZ and 641 DZ twin pairs, with less than 48 trials per condition) does not provide sufficient certainty for estimating the heritability of the Stroop effect. Achieving a reasonable level of precision may require impractical sample sizes (e.g., hundreds of trials and thousands of individuals).
The relative magnitude of intra-individual variability, as quantified by the ratio
To date, there has been an increasing number of twin studies utilizing task-based functional magnetic resonance imaging (fMRI). In these studies, it has been a common practice to aggregate data across trials during fMRI data analysis, resulting in the neglect of intra-individual variability, which is neither accounted for nor reported. For instance, Polk et al. (2007) reported a small heritability estimate of blood oxygenation level-dependent (BOLD) response
Is intra-individual variability a concern for heritability estimation in neuroimaging? The aforementioned task-based fMRI experiments have primarily relied on a large number of trials to obtain reliable estimates of condition-level effects. This is similar to the Stroop dataset we investigated here, with the distinction that the focus is on BOLD response rather than reaction time. However, the family sample size has often been relatively small, leading to larger uncertainty ranges in the estimates. This issue is particularly pronounced because the relative intra-individual variability across the brain, as reported in the literature, tends to be substantial, with
The HLM approach comes with additional costs. Firstly, introducing an extra level in the data hierarchy significantly increases the complexity of the model structure. Secondly, and perhaps the greater challenge, this increased complexity brings along numerical burdens. Traditional tools like linear mixed-effects estimation are not well-suited for solving hierarchical models of heritability. Instead, resorting to a Bayesian approach may be necessary to handle the numerical challenges (e.g., singularity).
There is always room for improvement in modeling. For example, the full details of the underlying mechanism and framework of cognitive inhibition involved in the reaction time of the Stroop effect are not fully known to researchers. Therefore, no model can fully replicate their structure. However, HLM attempts to model as much as is known and observed in a study. Model fitting can be improved by incorporating auxiliary information, such as accommodating abnormalities like skewness, outliers, and truncation through more inclusive and adaptive distributions (e.g., log-normal, ex-Gaussian). Additionally, one could reconsider the chosen partitioning into three components of
Further integrating HLM with the conventional SEM framework presents a promising avenue for future research. SEM, with its long-established history, offers distinct advantages, including intuitive interpretation, specialized applications, and computational efficiency. While beyond the scope of this study, leveraging the strengths of both SEM and HLM (e.g., Mehta and Neale, 2005) holds significant potential. A unified approach could enable greater flexibility in modeling distributions, account for intraindividual variability, and reduce estimation biases. In addition, this study focuses on within-individual categorical variables, such as task conditions. Future research could extend this framework to within-individual quantitative variables, particularly in the context of longitudinal data (e.g., Eaves et al., 1986).
The interpretation of heritability is subtle and sometimes controversial. Our focus here is solely on the technical aspects of heritability estimation. Nevertheless, we emphasize caution in its interpretation. As a statistical metric, heritability captures variation and/or correlation rather than causation. Therefore, one must not confuse the extent of phenotype variability with the contribution of genetic factors. The concept of heritability effectively pertains to the population level and cannot be realistically applied to a particular individual. On the other hand, the information provided by heritability lies in its potential predictability. It can probabilistically predict, but not causally determine, the extent of phenotypic variability. A high heritability for a phenotypic trait may warrant further investigation into the underlying complex genetic mechanisms or the etiology of genetic risk factors, such as biomarkers. This perspective highlights the need to complement heritability research of variance partitioning with mechanism elucidation (Downes and Turkheimer, 2022).
We propose an HLM approach to improve heritability estimation in twin studies when the phenotypic trait is measured with multiple samples. The methodology aims to separate measurement errors from the variations of interest and addresses issues such as information loss due to data reduction, distribution violations, and uncertainty characterization in current modeling approaches. We demonstrated that the conventional SEM is likely to underestimate heritability when intra-individual variability is moderate to high (which is common in many real-world scenarios). We supported this finding with analytical derivations, simulations and an experimental dataset from the ABCD study, validating the performance of the HLM approach. Our simulation results suggest that traits with small effect sizes may require much larger sample sizes than currently practiced.
The data presented in the study are deposited in the repository https://nda.nih.gov/study.html?id=1299, accession number 1299.
The studies involving humans were approved by Institutional Review Board of the University of California, San Diego (UCSD). The studies were conducted in accordance with the local legislation and institutional requirements. Written informed consent for participation in this study was provided by the participants’ legal guardians/next of kin.
GC: Conceptualization, Data curation, Formal Analysis, Investigation, Methodology, Project administration, Resources, Software, Supervision, Validation, Visualization, Writing–original draft, Writing–review and editing. DM: Data curation, Writing–review and editing. PT: Funding acquisition, Supervision, Writing–review and editing.
The author(s) declare that financial support was received for the research, authorship, and/or publication of this article. GC and PT were supported by the NIMH Intramural Research Program (ZICMH002888) of the NIH/HHS, United States. DM was also supported by the NIMH Intramural Research Program (ZICMH002960) of the NIH/HHS, United States. Data used in the preparation of this article were obtained from the Adolescent Brain Cognitive Development (ABCD) Study (https://abcdstudy.org), held in the NIMH Data Archive (NDA).
The authors declare that the research was conducted in the absence of any commercial or financial relationships that could be construed as a potential conflict of interest.
The author(s) declare that no Generative AI was used in the creation of this manuscript.
All claims expressed in this article are solely those of the authors and do not necessarily represent those of their affiliated organizations, or those of the publisher, the editors and the reviewers. Any product that may be evaluated in this article, or claim that may be made by its manufacturer, is not guaranteed or endorsed by the publisher.
The Supplementary Material for this article can be found online at: https://www.frontiersin.org/articles/10.3389/fgene.2025.1522729/full#supplementary-material
1The term “uncertainty interval” is used here to include confidence intervals in frequentist usage and credible intervals in Bayesian methods.
Anokhin, A. P., Golosheykin, S., Grant, J. D., and Heath, A. C. (2017). Heritability of brain activity related to response inhibition: a longitudinal genetic study in adolescent twins. Int. J. Psychophysiol. 115, 112–124. doi:10.1016/j.ijpsycho.2017.03.002
Arbet, J., McGue, M., and Basu, S. (2020). A robust and unified framework for estimating heritability in twin studies using generalized estimating equations. Statistics Med. 39, 3897–3913. doi:10.1002/sim.8564
Baker, D. H., Vilidaite, G., Lygo, F. A., Smith, A. K., Flack, T. R., Gouws, A. D., et al. (2021). Power contours: optimising sample size and precision in experimental psychology and human neuroscience. Psychol. Methods 26, 295–314. doi:10.1037/met0000337
Bates, D., Mächler, M., Bolker, B., and Walker, S. (2015). Fitting linear mixed-effects models using lme4. J. Stat. Softw. 67, 1–48. doi:10.18637/jss.v067.i01
Bates, T. C., Maes, H., and Neale, M. C. (2019). Umx: twin and path-based structural equation modeling in R. Twin Res. Hum. Genet. 22, 27–41. doi:10.1017/thg.2019.2
Blokland, G. A. M., McMahon, K. L., Thompson, P. M., Martin, N. G., de Zubicaray, G. I., and Wright, M. J. (2011). Heritability of working memory brain activation. J. Neurosci. 31, 10882–10890. doi:10.1523/JNEUROSCI.5334-10.2011
Bürkner, P. C. (2017). Brms: an R package for bayesian multilevel models using stan. J. Stat. Softw. 80, 1–28. doi:10.18637/jss.v080.i01
Chen, G., Pine, D. S., Brotman, M. A., Smith, A. R., Cox, R. W., and Haller, S. P. (2021). Trial and error: a hierarchical modeling approach to test-retest reliability. NeuroImage 245, 118647. doi:10.1016/j.neuroimage.2021.118647
Chen, G., Pine, D. S., Brotman, M. A., Smith, A. R., Cox, R. W., Taylor, P. A., et al. (2022). Hyperbolic trade-off: the importance of balancing trial and subject sample sizes in neuroimaging. NeuroImage 247, 118786. doi:10.1016/j.neuroimage.2021.118786
Chen, X., Formisano, E., Blokland, G. A. M., Strike, L. T., McMahon, K. L., de Zubicaray, G. I., et al. (2019). Accelerated estimation and permutation inference for ACE modeling. Hum. Brain Mapp. 40, 3488–3507. doi:10.1002/hbm.24611
Chung, Y., Rabe-Hesketh, S., Dorie, V., Gelman, A., and Liu, J. (2013). A nondegenerate penalized likelihood estimator for variance parameters in multilevel models. Psychometrika 78, 685–709. doi:10.1007/s11336-013-9328-2
Downes, S. M., and Matthews, L. (2020). Heritability in The stanford encyclopedia of philosophy. Editor E. N. Zalta spring 2020 edn (Metaphysics Research Lab, Stanford University).
Downes, S. M., and Turkheimer, E. (2022). An early history of the heritability coefficient applied to humans (1918–1960). Biol. Theory 17, 126–137. doi:10.1007/s13752-021-00392-9
Eaves, L. J., Long, J., and Heath, A. C. (1986). A theory of developmental change in quantitative phenotypes applied to cognitive development. Behav. Genet. 16, 143–162. doi:10.1007/BF01065484
Falconer, D. S. D. S., and MacKay, T. F. C. (1996). Introduction to quantitative genetics. Harlow: Prentice Hall.
Fan, J., Wu, Y., Fossella, J. A., and Posner, M. I. (2001). Assessing the heritability of attentional networks. BMC Neurosci. 2, 14. doi:10.1186/1471-2202-2-14
Fisher, R. A. (1954). “Statistical methods for research workers,” in Biological monographs and manuals. 12th ed., rev ed. Edinburgh: Oliver & Boyd.
Fiske, D. W., and Rice, L. (1955). Intra-individual response variability. Psychol. Bull. 52, 217–250. doi:10.1037/h0045276
Germine, L., Russell, R., Bronstad, P. M., Blokland, G. A., Smoller, J. W., Kwok, H., et al. (2015). Individual aesthetic preferences for faces are shaped mostly by environments, not genes. Curr. Biol. CB 25, 2684–2689. doi:10.1016/j.cub.2015.08.048
Guo, G., and Wang, J. (2002). The mixed or multilevel model for behavior genetic analysis. Behav. Genet. 32, 37–49. doi:10.1023/a:1014455812027
Gustavson, D. E., Nayak, S., Coleman, P. L., Iversen, J. R., Lense, M. D., Gordon, R. L., et al. (2023). Heritability of childhood music engagement and associations with language and executive function: insights from the adolescent brain cognitive development (abcd) study. Behav. Genet. 53, 189–207. doi:10.1007/s10519-023-10135-0
Haines, N., Kvam, P. D., Irving, L. H., Smith, C., Beauchaine, T. P., Pitt, M. A., et al. (2020). Learning from the reliability paradox: how theoretically informed generative models can advance the social. Behav. Brain Sci. doi:10.31234/osf.io/xr7y3
Harper, J., Malone, S. M., and Iacono, W. G. (2019). Target-related parietal P3 and medial frontal theta index the genetic risk for problematic substance use. Psychophysiology 56, e13383. doi:10.1111/psyp.13383
Holst, K. K., Scheike, T. H., and Hjelmborg, J. B. (2016). The liability threshold model for censored twin data. Comput. Statistics and Data Analysis 93, 324–335. doi:10.1016/j.csda.2015.01.014
Hung, I. T., Ganiban, J. M., and Saudino, K. J. (2023). Using the flanker task to examine genetic and environmental contributions in inhibitory control across the preschool period. Behav. Genet. 53, 132–142. doi:10.1007/s10519-022-10129-4
Hunter, M. D. (2021). Multilevel modeling in classical twin and modern molecular behavior genetics. Behav. Genet. 51, 301–318. doi:10.1007/s10519-021-10045-z
Iacono, W. G., Heath, A. C., Hewitt, J. K., Neale, M. C., Banich, M. T., Luciana, M. M., et al. (2018). The utility of twins in developmental cognitive neuroscience research: how twins strengthen the ABCD research design. Dev. Cogn. Neurosci. 32, 30–42. doi:10.1016/j.dcn.2017.09.001
Kastrati, G., Rosén, J., Thompson, W. H., Chen, X., Larsson, H., Nichols, T. E., et al. (2022). Genetic influence on nociceptive processing in the human brain—a twin study. Cereb. Cortex 32, 266–274. doi:10.1093/cercor/bhab206
Maes, H. H. (2005). “Ace model,” in Encyclopedia of statistics in behavioral science. Editors B. S. Everitt, and D. C. Howell (John Wiley and Sons, Ltd), 603–605.
Martin, N. G., Eaves, L. J., Kearsey, M. J., and Davies, P. (1978). The power of the classical twin study. Heredity 40, 97–116. doi:10.1038/hdy.1978.10
Matthews, S. C., Simmons, A. N., Strigo, I., Jang, K., Stein, M. B., and Paulus, M. P. (2007). Heritability of anterior cingulate response to conflict: an fMRI study in female twins. NeuroImage 38, 223–227. doi:10.1016/j.neuroimage.2007.07.015
McArdle, J. J., and Prescott, C. A. (2005). Mixed-effects variance components models for biometric family analyses. Behav. Genet. 35, 631–652. doi:10.1007/s10519-005-2868-1
Mehta, P. D., and Neale, M. C. (2005). People are variables too: multilevel structural equations modeling. Psychol. Methods 10, 259–284. doi:10.1037/1082-989X.10.3.259
Neale, M. C., Hunter, M. D., Pritikin, J. N., Zahery, M., Brick, T. R., Kirkpatrick, R. M., et al. (2016). OpenMx 2.0: extended structural equation and statistical modeling. Psychometrika 81, 535–549. doi:10.1007/s11336-014-9435-8
Pingault, J. B., Allegrini, A. G., Odigie, T., Frach, L., Baldwin, J. R., Rijsdijk, F., et al. (2022). Research Review: how to interpret associations between polygenic scores, environmental risks, and phenotypes. J. Child Psychol. Psychiatry 63, 1125–1139. doi:10.1111/jcpp.13607
Polderman, T. J. C., Benyamin, B., de Leeuw, C. A., Sullivan, P. F., van Bochoven, A., Visscher, P. M., et al. (2015). Meta-analysis of the heritability of human traits based on fifty years of twin studies. Nat. Genet. 47, 702–709. doi:10.1038/ng.3285
Polk, T. A., Park, J., Smith, M. R., and Park, D. C. (2007). Nature versus nurture in ventral visual cortex: a functional magnetic resonance imaging study of twins. J. Neurosci. 27, 13921–13925. doi:10.1523/JNEUROSCI.4001-07.2007
Rea-Sandin, G., Clifford, S., Doane, L. D., Davis, M. C., Grimm, K. J., Russell, M. T., et al. (2023). Genetic and environmental links between executive functioning and effortful control in middle childhood. J. Exp. Psychol. General 152, 780–793. doi:10.1037/xge0001298
Rijsdijk, F. V., and Sham, P. C. (2002). Analytic approaches to twin data using structural equation models. Briefings Bioinforma. 3, 119–133. doi:10.1093/bib/3.2.119
Robette, N., Génin, E., and Clerget-Darpoux, F. (2022). Heritability: what’s the point? What is it not for? A human genetics perspective. Genetica 150, 199–208. doi:10.1007/s10709-022-00149-7
Rouder, J.N., Kumar, A., and Haaf, J. M. (2023). Why many studies of individual differences with inhibition tasks may not localize correlations. Psychonomic Bulletin Review 30, 2049–2066. doi:10.3758/s13423-023-02293-3
Rouder, J. N., and Haaf, J. M. (2019). A psychometrics of individual differences in experimental tasks. Psychonomic Bull. and Rev. 26, 452–467. doi:10.3758/s13423-018-1558-y
Routledge, K. M., Williams, L. M., Harris, A. W. F., Schofield, P. R., Clark, C. R., and Gatt, J. M. (2018). Genetic correlations between wellbeing, depression and anxiety symptoms and behavioral responses to the emotional faces task in healthy twins. Psychiatry Res. 264, 385–393. doi:10.1016/j.psychres.2018.03.042
Schachar, R. J., Forget-Dubois, N., Dionne, G., Boivin, M., and Robaey, P. (2010). Heritability of response inhibition in children. J. Int. Neuropsychological Soc. 17, 238–247. doi:10.1017/S1355617710001463
Schwabe, I., Gu, Z., Tijmstra, J., Hatemi, P., and Pohl, S. (2019). Psychometric modelling of longitudinal genetically informative twin data. Front. Genet. 10, 837. doi:10.3389/fgene.2019.00837
Sham, P. C., Purcell, S. M., Cherny, S. S., Neale, M. C., and Neale, B. M. (2020). Statistical power and the classical twin design. Twin Res. Hum. Genet. 23, 87–89. doi:10.1017/thg.2020.46
Smith, D. M., Loughnan, R., Friedman, N. P., Parekh, P., Frei, O., Thompson, W. K., et al. (2023). Heritability estimation of cognitive phenotypes in the ABCD Study® using mixed models. Behav. Genet. 53, 169–188. doi:10.1007/s10519-023-10141-2
Smolker, H. R., Wang, K., Luciana, M., Bjork, J. M., Gonzalez, R., Barch, D. M., et al. (2022). The Emotional Word-Emotional Face Stroop task in the ABCD study: psychometric validation and associations with measures of cognition and psychopathology. Dev. Cogn. Neurosci. 53, 101054. doi:10.1016/j.dcn.2021.101054
Spearman, C. (1904). The proof and measurement of association between two things. Am. J. Psychol. 15, 72–101. doi:10.2307/1412159
Stins, J. F., de Sonneville, L. M. J., Groot, A. S., Polderman, T. C., van Baal, C. G. C. M., and Boomsma, D. I. (2005). Heritability of selective attention and working memory in preschoolers. Behav. Genet. 35, 407–416. doi:10.1007/s10519-004-3875-3
van den Berg, S. M., Glas, C. A. W., and Boomsma, D. I. (2007). Variance decomposition using an IRT measurement model. Behav. Genet. 37, 604–616. doi:10.1007/s10519-007-9156-1
van den Oord, E. J. (2001). Estimating effects of latent and measured genotypes in multilevel models. Stat. Methods Med. Res. 10, 393–407. doi:10.1177/096228020101000603
van der Meulen, M., Steinbeis, N., Achterberg, M., van Ijzendoorn, M. H., and Crone, E. A. (2018). Heritability of neural reactions to social exclusion and prosocial compensation in middle childhood. Dev. Cogn. Neurosci. 34, 42–52. doi:10.1016/j.dcn.2018.05.010
van Drunen, L., Dobbelaar, S., van der Cruijsen, R., van der Meulen, M., Achterberg, M., Wierenga, L. M., et al. (2021). The nature of the self: neural analyses and heritability estimates of self-evaluations in middle childhood. Hum. Brain Mapp. 42, 5609–5625. doi:10.1002/hbm.25641
Vellani, V., Garrett, N., Gaule, A., Patil, K. R., and Sharot, T. (2022). Quantifying the heritability of belief formation. Sci. Rep. 12, 11833. doi:10.1038/s41598-022-15492-0
Viktorsson, C., Lindskog, M., Li, D., Tammimies, K., Taylor, M. J., Ronald, A., et al. (2022). Infants’ sense of approximate numerosity: heritability and link to other concurrent traits. Dev. Sci. n/a 26, e13347. doi:10.1111/desc.13347
Wang, L., Wang, Y., Xu, Q., Liu, D., Ji, H., Yu, Y., et al. (2020). Heritability of reflexive social attention triggered by eye gaze and walking direction: common and unique genetic underpinnings. Psychol. Med. 50, 475–483. doi:10.1017/S003329171900031X
Keywords: heritability, twin studies, ACE model, Falconer’s method, intra-individual variability, hierarchical modeling, data generating mechanism, Bayesian statistics
Citation: Chen G, Moraczewski D and Taylor PA (2025) Improving accuracy and precision of heritability estimation in twin studies through hierarchical modeling: reassessing the measurement error assumption. Front. Genet. 16:1522729. doi: 10.3389/fgene.2025.1522729
Received: 04 November 2024; Accepted: 06 March 2025;
Published: 02 April 2025.
Edited by:
Roseann E. Peterson, Suny Downstate Health Sciences University, United StatesReviewed by:
Felix Christian Tropf, ENSAE ParisTech Ecole Nationale de la Statistique et de l’Administration Economique, FranceCopyright © 2025 Chen, Moraczewski and Taylor. This is an open-access article distributed under the terms of the Creative Commons Attribution License (CC BY). The use, distribution or reproduction in other forums is permitted, provided the original author(s) and the copyright owner(s) are credited and that the original publication in this journal is cited, in accordance with accepted academic practice. No use, distribution or reproduction is permitted which does not comply with these terms.
*Correspondence: Gang Chen, Z2FuZ2NoZW5AbWFpbC5uaWguZ292
Disclaimer: All claims expressed in this article are solely those of the authors and do not necessarily represent those of their affiliated organizations, or those of the publisher, the editors and the reviewers. Any product that may be evaluated in this article or claim that may be made by its manufacturer is not guaranteed or endorsed by the publisher.
Research integrity at Frontiers
Learn more about the work of our research integrity team to safeguard the quality of each article we publish.