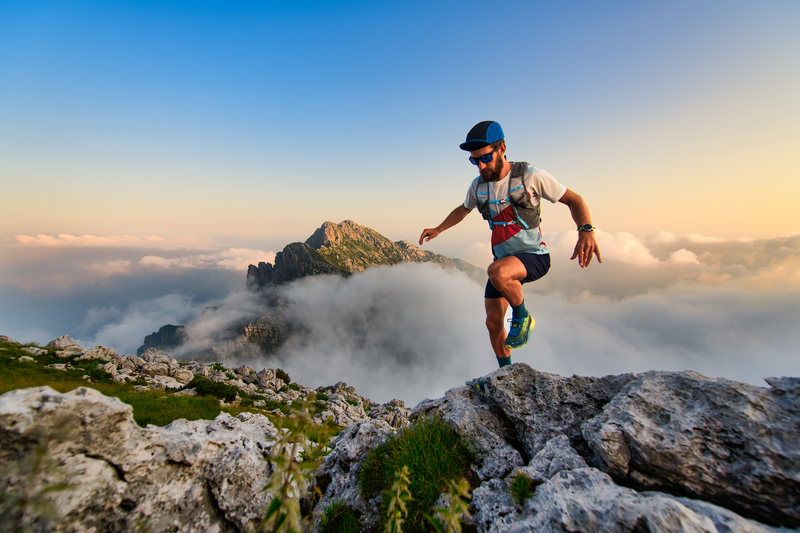
94% of researchers rate our articles as excellent or good
Learn more about the work of our research integrity team to safeguard the quality of each article we publish.
Find out more
ORIGINAL RESEARCH article
Front. Genet.
Sec. Computational Genomics
Volume 16 - 2025 | doi: 10.3389/fgene.2025.1520325
This article is part of the Research Topic Computational Approaches Integrate Multi-Omics Data for Disease Diagnosis and Treatment View all 5 articles
The final, formatted version of the article will be published soon.
You have multiple emails registered with Frontiers:
Please enter your email address:
If you already have an account, please login
You don't have a Frontiers account ? You can register here
Abstract The existing evidence indicates that atherosclerosis (AS) plays a pivotal role in the progression and exacerbation of cardiovascular diseases and their associated complications. Current diagnostic and therapeutic strategies for atherosclerosis are limited in their ability to facilitate early detection and personalized treatment. This study employs a systems biology approach to investigate the role of lactylation-related genes (LRGs) in the pathogenesis of atherosclerosis, while considering the well-established correlation between inflammatory responses and atherosclerosis development. In this study, we utilized datasets obtained from the Gene Expression Omnibus (GEO) as well as data from previous studies on lactylation-related genes (LRGs). Following this, we identified 17 lactylation-related genes associate with atherosclerosis (AS-LRGs) from the GSE100927 dataset. Subsequently, we employed the validation dataset (GSE43292) to assess these 17 AS-LRGs, resulting in the identification of 13 more reliable candidate genes. These genes were further analyzed for functional enrichment through Gene Ontology (GO) annotation, Kyoto Encyclopedia of Genes and Genomes (KEGG) pathway analysis, and gene set enrichment analysis (GSEA). To elucidate the potential utility of AS-LRGs in diagnosing high-risk plaques, we assessed their expression in both early and late stages of atherosclerosis, as well as in high- and low-risk plaques. We then constructed interaction networks to elucidate the potential regulatory relationships among LRGs, miRNAs, transcription factors, and drugs. Finally, we utilized the single-sample Gene Set Enrichment Analysis (ssGSEA) method to investigate immune infiltration in AS and evaluate the levels of immune cell infiltration. In conclusion, this study elucidates the pivotal role of lactylation in atherosclerosis (AS) and establishes a robust foundation for future research into targeted therapies and clinical applications of the identified biomarkers.
Keywords: Atherosclerosis, lactylation, Immune infiltration, risk prediction, biomarkers Arial
Received: 31 Oct 2024; Accepted: 13 Feb 2025.
Copyright: © 2025 Qian and Zhou. This is an open-access article distributed under the terms of the Creative Commons Attribution License (CC BY). The use, distribution or reproduction in other forums is permitted, provided the original author(s) or licensor are credited and that the original publication in this journal is cited, in accordance with accepted academic practice. No use, distribution or reproduction is permitted which does not comply with these terms.
* Correspondence:
Qing Zhou, Department of Cardiothoracic surgery, Nanjing Drum Tower Hospital, Affiliated Hospital of Medical School, Nanjing University, Nanjing, Liaoning Province, China
Disclaimer: All claims expressed in this article are solely those of the authors and do not necessarily represent those of their affiliated organizations, or those of the publisher, the editors and the reviewers. Any product that may be evaluated in this article or claim that may be made by its manufacturer is not guaranteed or endorsed by the publisher.
Research integrity at Frontiers
Learn more about the work of our research integrity team to safeguard the quality of each article we publish.