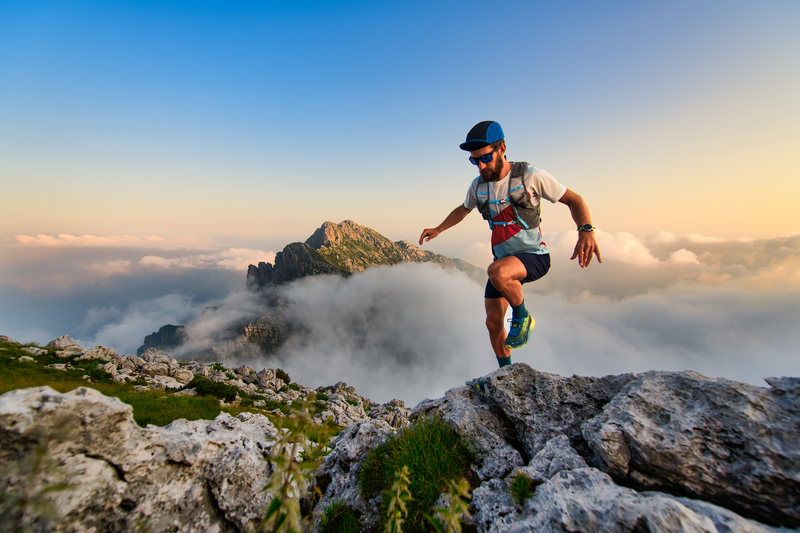
95% of researchers rate our articles as excellent or good
Learn more about the work of our research integrity team to safeguard the quality of each article we publish.
Find out more
ORIGINAL RESEARCH article
Front. Genet. , 11 February 2025
Sec. Computational Genomics
Volume 16 - 2025 | https://doi.org/10.3389/fgene.2025.1511521
Recent studies indicate that microorganisms are crucial for maintaining human health. Dysbiosis, or an imbalance in these microbial communities, is strongly linked to a variety of human diseases. Therefore, understanding the impact of microbes on disease is essential. The DuGEL model leverages the strengths of graph convolutional neural network (GCN) and graph attention network (GAT), ensuring that both local and global relationships within the microbe-disease association network are captured. The integration of the Long Short-Term Memory Network (LSTM) further enhances the model’s ability to understand sequential dependencies in the feature representations. This comprehensive approach allows DuGEL to achieve a high level of accuracy in predicting potential microbe-disease associations, making it a valuable tool for biomedical research and the discovery of new therapeutic targets. By combining advanced graph-based and sequence-based learning techniques, DuGEL addresses the limitations of existing methods and provides a robust framework for the prediction of microbe-disease associations. To evaluate the performance of DuGEL, we conducted comprehensive comparative experiments and case studies based on two databases, HMDAD, and Disbiome to demonstrate that DuGEL can effectively predict potential microbe-disease associations.
Microorganisms play an important and far-reaching role in human life and greatly impact our health (Liang et al., 2018). Recent reports indicate that the human body is host to trillions of microorganisms (Hoffmann et al., 2016) and that the number of microorganisms in the human body far exceeds the number of human cells (Bocci, 1992). These microorganisms constitute the microbiota in the human body (Zhu et al., 2010). The microbiome plays a critical role in human physiology (Heintz-Buschart and Wilmes, 2018), helping the body’s intestinal tract to reduce the growth of pathogenic bacteria and infections and synthesizing some of the vitamins and amino acids needed by the body (Islam et al., 2022). Suppose the microbial community in the human body is out of balance. In that case, it can impair the function of the immune system, increase the risk of infection with pathogens (Pickard et al., 2017), lead to malnutrition or nutritional deficiencies (Burr et al., 2020), and contribute to the development of mental health-related problems such as anxiety and depression (Anisman et al., 2018), as well as metabolic diseases such as obesity and diabetes (Sanz et al., 2015). Of course, the microbiota can help the body regulate and prevent attacks from bacteria outside the body (Barr, 2017); for example, actinomycetes are a class of antibiotic-producing bacteria that produce a wide range of antibiotics such as streptomycin and tetracycline (Grasso et al., 2016). These antibiotics inhibit the growth of other pathogenic microorganisms and help protect the body from infection (Jagannathan et al., 2021). Therefore, predicting potential associations between microorganisms and diseases is vital for unraveling the complex mechanisms of disease occurrence and discovering potential biomarkers (Montaner et al., 2020). By inferring the interactions between microorganisms and diseases, we can better understand the diagnosis and prognosis of diseases and provide new ideas and methods for preventing, diagnosing, and treating diseases (Malla et al., 2019). As technology advances, we no longer rely solely on traditional biological methods to explore the association between microbes and disease (Gilbert et al., 2016). Instead, we are increasingly introducing computational modelling into our research to predict the role of microbes in disease occurrence, development, and treatment through techniques such as big data analytics and deep learning (Marcos-Zambrano et al., 2021), which are more practical and accurate (Najafabadi et al., 2015). Researchers have recently established a series of microbe-disease association databases to conduct an in-depth study of the potential link between microbes and diseases (Jin et al., 2022). These databases combine a large amount of microbial composition data and disease information. For example, the HMDAD database created by Ma et al. became the first to document human microbe-disease associations by manually organizing a large amount of public literature (Ma et al., 2017). This database covers 483 pieces of information about the association between 39 diseases and 292 microorganisms. Second, Janssens et al. created a microbial-disease association database called Disbiome by collecting 10,922 experimental records from 1,191 documents containing 372 diseases and 1,622 microorganisms (Janssens et al., 2018).
Researchers can explore and discover the relationships between microorganisms and different diseases using the above microbe-disease association database as the primary data. Moreover, these recent technological tools can be broadly categorized into four types, namely, network-based methods (Wu et al., 2018), matrix decomposition-based methods (Shen et al., 2021), traditional machine learning-based methods (Afshari et al., 2022), and graph neural network-based methods (Li et al., 2023).
In DuGEL, we use both Graph Convolutional Neural Network (GCN) and Graph Attention Network (GAT), where GCN is specifically designed to process graph data (Jin et al., 2021). GCN can learn feature representations at both node and graph levels, and it achieves the task of learning and predicting the representations of graph data by efficiently exploiting the connectivity relationships between the nodes (Zhou et al., 2023). By adaptively learning the attention weights between each node and its neighbouring nodes, GAT can better capture local structural information in graph data. The GAT introduction enriches the representational capabilities of the graph neural network, allowing it to perform well when dealing with complex graph-structured data (Munikoti et al., 2023). DuGEL can adapt to an extensive range of datasets with solid robustness.
Unlike the above methods, in this paper, we designed a new computational model called DuGEL based on the graph convolutional neural network and the graph attention network to infer possible microbe-disease associations. In DuGEL, we first downloaded known microbe-disease associations to form a heterogeneous microbe-disease network. Then, we input this network into a graph convolutional neural network and a graph attention network separately to obtain the local and global features of nodes in the network. Next, we spliced the outputs of the graph convolutional neural network and the graph attention network and then introduced a Long Short-Term Memory (LSTM) network to process the fused features. Finally, the output of the LSTM would be passed to a fully connected layer to infer potential associations between microbes and diseases. Experiments showed that DuGEL obtained satisfactory predictive performance with a 5-fold cross-validated auc of 0.9698 and 0.9119 for HMDAD and Disbiome datasets, respectively, and may be a potential tool for future microbe-disease association prediction.
HMDAD, constructed by Ma et al. (2017), and Disbiome (Janssens et al., 2018), constructed by Janssens et al., are the main publicly available biomedical databases containing microbe-disease association data. As shown in Table 1, HMDAD database covers 483 known microbe-disease associations, and processing these data, we ended up with 450 known microbial-disease associations. The HMDAD database provides a valuable information resource for studying microbial-disease relationships. In addition, the Disbiome, constructed by Janssens et al., is a publicly available database of microbe-disease associations. As shown in Table 1, Disbiome database collects 5,573 known associations from published academic papers for 240 diseases and 1,098 microorganisms. After de-duplication, we had 4,351 known microbe-disease associations covering 218 diseases and 1,052 microorganisms. Due to its extensive data collection and detailed information records, the Disbiome database has become a vital data support for research in this field.
After acquiring the initial data, we performed data preprocessing steps to ensure the quality of the data and the validity of the model training. First, we removed all duplicate records to ensure that the association of each microbe with a disease was unique. Further, we converted the data into a uniform format to facilitate subsequent processing and model training. For simplicity, for each dataset, let
Based on the assumption that two similar diseases will show similar interaction and non-interaction relationships with the same microorganism, in this section, we adapt the Gaussian interaction profile kernel similarity between a pair of diseases
Where
Based on the assumption that if two diseases are similar to each other, then their cosine curves will be more coincident, we introduce the cosine similarity between a pair of diseases
The result of cosine similarity has good stability and certainty, the calculation speed is fast and the result is more intuitive. Suitable for large-scale information retrieval. Where
Based on the assumption that similar diseases tend to interact with similar genes, in this section, we calculate the disease functional similarity based on the functional associations between disease-related genes as follows: Firstly, we download the gene interactions from the HumanNet database in which, every interaction has an associated log-likelihood score (LLS). And then, for any given diseases
where
where
Thereafter, by combining the above GIP kernel similarity, disease cosine similarity, and functional similarity of disease, we can obtain an integrated similarity matrix of disease as follows:
In the same way, we can calculate the gaussian interaction profile kernel similarity between any two microbes
where
Similarly, the cosine similarity between any two microbes
The calculation process of cosine similarity between two microorganisms is the same as that of disease cosine similarity. Similarly, when the similarity between two microorganisms is exceptionally high, the calculation result tends to be 1. When the similarity between two microorganisms is very low, the calculation result tends to −1.
The functional similarity of the microbe is calculated by using the following method (Zhang et al., 2018): for any given disease
The semantic value of disease
Then, the semantic similarity between any two diseases
Relying on the above formulae, we can further define the similarity between the disease
Hence, for any two given microbes
where
Based on above descriptions, it is easy to see that we can construct a heterogeneous network
As illustrated in above Figure 1, the DuGEL consists of the following five steps:
• Step 1: Construct a heterogeneous microbe-disease network based on newly downloaded known microbe-disease associations and multiple microbe and disease similarity metrics.
• Step 2: Feeding the heterogeneous microbe-disease network forward into a dual channel structure consisting of a Graph Convolutional Neural Network (GCN) and a Graph Attention Network (GAT), where the GCN is utilized to extract spatial features of nodes in the heterogeneous microbe-disease network from local to global, and the GAT is adopted to assign different importance to the neighbors of each node in the heterogeneous microbe-disease network as it is processed.
• Step 3: Splicing the outputs of GCN and GAT by simply fusing the information captured by GCN and GAT and combining structural and node characteristics and the importance between neighboring nodes.
• Step 4: Implementing a Long Short-Term Memory (LSTM) network to process the fused features, then feeding the output of LSTM into a fully connected layer to convert the high-level features captured by LSTM into the target output space.
• Step 5: By feeding the newly obtained feature vectors of the target output space into a Sigmoid function for binary prediction, potential associations between microbes and diseases can be finally computed.
In DuGEL, the input layer is the Microbe-Disease Association Representation Layer, is a component used to convert raw data of known microbe-disease associations into a structured data fame that can be processed by subsequent graph neural networks. Firstly, the newly collected microbe-disease association data need to be pre-processed to ensure consistency and accuracy. The preprocessed data will be used in turn to construct a binary microbe-disease association matrix M, which implies potential relationships between microorganisms and disease (Marsh and Zaura, 2017), and can be defined as follows: Given a microorganism
To enhance the prediction ability of DuGEL, similarity information is also fused in the representation layer (Feng et al., 2023), which contains the microbial similarity matrix
In addition, we will initialize each representative microbe or disease node in the graph with a feature vector. Formally, let
The microbe-disease association representation layer lays the foundation for the entire DuGEL model, and by meticulously structuring the input data, the layer ensures that subsequent graph neural networks are able to effectively capture both local and global patterns in the data. The construction of the graph enables the model to fully utilize all available information, thereby improving the accuracy and robustness of microbe-disease association predictions.
In this study, the dual graph feature extraction module is a core component of the DuGEL designed to extract deep features from the input microbe-disease association feature matrix. The module combines two parallel graph neural network architectures: graph convolutional network (GCN) and graph attention network (GAT), ensuring effective capture of local and global relationships in the graph.
The Graph Convolutional Network (GCN) module extracts the spatial features of the graph by processing the microbe-disease feature matrix
where
GCN effectively smoothies the feature representation over the graph structure and ensures that the representation of each node is influenced by its neighbors (Chen et al., 2021), thus capturing the local structure information. By aggregating a node’s neighbor information, the feature representation of a node is made to reflect its local graph structure. This aggregation operation is performed in each layer, and through the gradual aggregation of multiple layers (Liu et al., 2018), the GCN can capture a wider range of graph information. This is particularly important for microbe-disease association prediction, as some associations may not be directly visible, but indirectly inferred through multi-hop relationships.
The Graph Attention Network (GAT) module introduces an attention mechanism that assigns different importance coefficients to each node’s neighbors. For each node
where
The GAT sublayer enables the model to focus on the most relevant parts of the graph, thus capturing the importance of each neighboring node during the feature aggregation process. The advantage is that it can dynamically adjust the contribution of each neighbor to a node’s feature update, and by learning different attention weights, GAT can allocate different attention between different parts of the graph structure (Chatzianastasis et al., 2023). This is particularly useful when dealing with complex biological networks, where associations between microorganisms and diseases may have different biological significance and importance.
The dual graph feature extraction module can capture local and global information in the graph structure by combining GCN and GAT approaches. GCN emphasizes the aggregated features of a node’s local neighbors. At the same time, GAT dynamically adjusts the weights of the neighboring nodes through the attentional mechanism (Wang et al., 2022), thus providing more flexible and fine-grained control in the feature extraction process. This dual approach ensures that the model understands direct associations between microbes and diseases and identifies potential indirect relationships through graph structural features and attention weights. Combining these two approaches enables the DuGEL model to excel in microbe-disease association prediction tasks, providing more accurate and comprehensive predictions.
The dual graph feature extraction module plays a crucial role in the DuGEL model. Capturing complex graph structure information improves the model’s predictive ability and enhances its robustness and generalization ability.
After processing in the GCN and GAT layers, we obtain two sets of feature representations
In the dual graph feature extraction module, we extract two different feature representations through GCN and GAT. The task of the feature fusion layer is to effectively fuse these different features to obtain a comprehensive feature representation. The fusion operation can be realized in various ways, such as concatenation, weighted summing, or multilayer perceptron (MLP) (Afzal et al., 2023). Here, we adopt the concatenation operation to stitch together feature representations from different sub-networks to form a richer feature vector. Assuming that the feature representation extracted through GCN is
where
After feature fusion, we need to further learn useful information from these high-dimensional features. The sequence learning layer is designed to capture the temporal or sequential dependencies between features to enhance the prediction capability (Yuan et al., 2023). By treating the node features as a sequence, the order in which node features are fed to the LSTM introduces a dependency chain. The model learns how the features of one node are influenced by or related to those of neighboring nodes. As illustrated in Figure 2, we introduce the Long Short-Term Memory Network (LSTM), which is capable of effectively remembering long-term dependencies and is suitable for processing sequence data (Yoo et al., 2023). The LSTM processes each node’s features across multiple time steps. Here, the “time steps” correspond to sequential relationships between node features derived from their embedding in the heterogeneous network. We represent the vector corresponding to the
where
The feature fusion layer effectively integrates feature representations extracted from different perspectives, providing more comprehensive input data (Zhang et al., 2020). The sequence learning layer, on the other hand, further enhances the model’s predictive capability by capturing the temporal dependencies between features. Combining the two ensures the model can fully utilize all available information to achieve higher accuracy and robustness in microbe-disease association prediction tasks.
The feature representation
where
Ultimately, the output layer gives the predicted probability of microbe-disease association (Marsh and Zaura, 2017). By setting an appropriate threshold, it is possible to determine whether there is an association between microbes and diseases.
To measure the difference between the predicted and true values of the model, in the DuGEL model, we use the cross-entropy loss function to evaluate the effectiveness of microbe-disease association prediction (Mao et al., 2023). The cross-entropy loss function is commonly used in classification problems, and in microbe-disease association prediction, we modeled the problem as a binary classification task, i.e., predicting whether there is an association between a certain pair of microorganisms and a disease. The cross-entropy loss function is defined as follows:
where
To prevent model overfitting, we add a regularization term to the loss function. The regularization term improves the generalization ability of the model by adding a penalty to the model complexity in the loss function (Fu et al., 2023), encouraging the model to choose simpler parameter configurations. In the DuGEL model, we use L2 regularization or weight decay. It is defined as follows:
where
Ultimately, the integrated loss function of the DuGEL model consists of a cross-entropy loss and a regularization term of the following form:
where
In this section, we will detail the experimental setup, evaluation metrics, and baseline methodology used to evaluate the performance of the DuGEL model and present the experimental results and analysis. The effectiveness and superiority of the DuGEL model in the microbe-disease association prediction task are verified by comparing it with multiple baseline methods. The corresponding pseudo-code of the DuGEL model is shown in Table 2.
In this study, we extracted disease features, microbial features, and disease-microbe association matrices from the HMDAD and Disbiome databases, and the three feature matrices mentioned above were used to construct heterogeneous maps to reflect the interactions between diseases and microbes for disease characterization and microbial characterization. The number of training rounds was set to 4,000 in the training phase. To optimize the algorithm to adjust the weights, the learning rate was set to 0.01. We set the random deactivation strategy for the adjacency matrix with the dropout ratio set to 0.5, thus preventing overfitting. For the subject model, to randomly discard some network connections during the training process to enhance the model’s generalization ability, we similarly set the random deactivation strategy with the dropout ratio set to 0.5. In addition, we set the similarity weight to 6 to weigh the similarity features of diseases and microorganisms. From the above description, it is easy to see that there are several hyperparameters in DuGEL, such as the dimension k of the embedded features, the number of layers L, the initial learning rate r of the optimizer, the total training epoch α, the node dropout β, and the rule dropout γ. As illustrated in Figures 3, 4, the various results for several combinations of parameters k and L in the 5-fold cv. From the figures, it is easy to see that the optimal combination of k and L is L = 2, k = 128, which indicates that the first and second-layer embedded features contain more information than the third-layer embedded features. After analyzing, this may be due to excessive smoothing of LSTM.
In the training and evaluation phases of the model, we perform multiple cross-validations. In each validation, the dataset is divided into a training set and a test set, which trains the model on the training set and evaluates the model performance on the test set. Specifically, 5-fold cross-validation (k_folds) is used to evaluate the model performance. In each cross-validation, the data are randomly disrupted and divided into five parts, one used as the test set and the rest as the training set. To ensure the stability and reliability of the results, we repeat the execution of the experiment 5 times and report the average performance metrics.
In order to evaluate the method proposed in this paper, we employ a series of evaluation metrics to comprehensively measure the performance of the model, including AUC, accuracy and specificity The formal definitions and calculations of each evaluation metric are given below:
Accuracy is the ratio of the number of correctly categorized samples to the total number of samples, which is defined as follows:
where
AUC (Area Under the ROC Curve) represents the area under the receiver operating characteristic curve (ROC Curve), which is used to measure the classification performance of the model. The ROC Curve plots the True Positive Rate (TPR) and False Positive Rate (FPR) through different thresholds. TPR and FPR are defined as follows:
The value of AUC is between 0 and 1, with larger values indicating better model performance.
Specificity, also known as True Negative Rate (TNR), is formally defined as follows:
Specificity indicates the proportion of all samples that are actually negative that are correctly predicted to be negative.
In order to compare the methods proposed in this paper horizontally, as shown in Table 3, we introduced nine microbe-disease association prediction methods of.
The above methods can be categorised into four groups, with network-based methods constructing complex network structures based on known microbial disease associations. These network-based methods construct complex network structures based on known microbial-disease associations. Then, the potential probability of associations between microorganisms and diseases is inferred by analyzing the structural features of these networks and the lengths and numbers of connecting paths between the nodes (Wang et al., 2011). For example, Chen et al. proposed the KATZHMDA computational model to infer possible microbe-disease associations using the KATZ measure, which takes measurements to capture global information in a network by counting all paths between nodes and then predicts potential associations (Chen et al., 2017). However, the matrix decomposition-based approach focuses on decomposing the known microbe-disease association matrix into two feature matrices and approximating the original association matrix by the product of these two matrices (Ma and Liu, 2022). Information such as similarity and strength of association between microorganisms and diseases can be obtained. Shen et al. proposed a computational model of CMFHMDA based on synergistic matrix decomposition (Shen et al., 2017). In addition, based on the traditional machine learning approach by using known associations as training samples, we can construct a model for predicting the association between unknown microorganisms and diseases (Long et al., 2021). For example, Wang et al. designed the LRLSHMDA model which represents the network structure by constructing a Laplace matrix and predicts the association by regularized least squares (Wang et al., 2017). Finally, the graph neural network-based approach utilizes neural networks to take microbial and disease-related data as inputs and to extract and explore features and patterns from graph-structured data (Bessadok et al., 2022). They can utilize we can utilize the powerful learning ability of neural networks to discover potential associations between microbes and diseases and mine functional patterns and features from complex graph data. For example, Wang et al. designed GCNMA that captures structural information in the network by introducing a graph convolutional neural network and incorporates a multilayer attention mechanism to enhance the ability to model complex relationships between nodes (Wang et al., 2023).
Although the above models can perform reliably in some aspects, they still have limitations. For example, the computation of Katz path correlation must consider all paths between different nodes (Zhang et al., 2017). This may lead to high computational complexity on large datasets, especially when the network size is large (Kumar et al., 2020). In addition, regularized least squares usually introduce a regularization term to avoid overfitting. However, choosing the appropriate regularization parameter is not easy. If it is not chosen correctly, it may lead to underfitting or overfitting problems. When there is noise in the input data, the regularized least squares method may be too sensitive to the noise, resulting in unstable or inaccurate prediction results (Jung and Park, 2017).
In this section, we provide a detailed analysis of the experimental results of our proposed DuGEL model on the HMDAD and Disbiome datasets and compare it with nine other state-of-the-art microbe-disease association prediction methods. First, Tables 4, 5 show the performance of our proposed DuGEL model, and the nine compared methods on the HMDAD and Disbiome datasets, respectively, are mainly compared under the AUC assessment metrics. On the HMDAD dataset, the DuGEL model performs well, with its AUC values of 0.9698 and 0.9606 in 5-fold cross-validation and 2-fold cross-validation, respectively, The higher AUC values indicate the more vital overall predictive ability of the model, which indicates that DuGEL can effectively distinguish between positive and negative samples. For the Disbiome dataset, the DuGEL model still performs well. Under 5-fold cross-validation and 2-fold cross-validation settings, DuGEL reaches 0.9119 and 0.8932.
In comparison with previous methods, the DuGEL model demonstrates excellent performance. we attribute the results to the intrinsic structure of DuGEL. DuGEL successfully achieves efficient prediction of microbial-disease associations by combining a graph convolutional neural network (GCN) and a graph Attention Network (GAT), as well as introducing a Long Short-Term Memory Network (LSTM) to process fused features, enabling efficient prediction of microbe-disease associations. This multilevel feature extraction and sequence modeling approach enables DuGEL to perform well on key metrics (e.g., AUPR and AUC),demonstrating its robustness in microbe-disease association prediction tasks.
In particular, the DuGEL model combines the strengths of GCN and GAT; GCN can efficiently capture both local and global spatial features in the graph and extract complex relationships between microbes and diseases by performing convolutional operations on the features of neighboring nodes. Moreover, through the attention mechanism, GAT can assign different importance weights to its neighbors when processing nodes, thus better capturing the information of critical nodes. After combining the two, the outputs of GCN and GAT are fused through the Dual Graph Enhanced Layer of the DuGEL model, which effectively integrates the structural features and node importance.
Furthermore, introducing the LSTM module further enhances the model’s capabilities. LSTM is good at processing sequence data and can better identify potential associations between microbes and diseases by capturing temporal dependencies (Baranwal et al., 2022). The memory unit of LSTM can preserve information of long-time dependencies (Wu et al., 2021), which is especially important for analyzing potential microbe-disease relationships over long periods.
In addition, considering the role and contribution of every sub-module in the DuGEL model proposed in this study, we function ablation experiments on the HMDAD dataset to inspect the effect of distinctive parts of the model. We carried out three specific ablation experiments:
1. Doing away with the diagram convolution sublayer (denoted as “-GCN Layer”)
2. Removing the sketch attention sublayer (denoted as “-GAT Layer”)
3. Casting off the BiLSTM module (denoted as “-BiLSTM Module”)
Overall, each sub-module of the DuGEL model improves the primary mannequin’s effectiveness by taking pictures of the correlation between microorganisms and diseases. Table 6 suggests the results of the ablation experiments. In the first ablation experiment, after getting rid of the graph convolution sublayer, the mannequin’s AUC drops to 0.9465 and 0.9392 in 5-fold cross-validation and 2-fold pass validation, respectively, which suggests that GCN plays a crucial role in shooting nearby and international spatial points between microbes and diseases. By performing convolutional operations on the elements of neighboring nodes, GCN can extract complex correlation information, and removing this component considerably decreases the model’s predictive power.
In the second ablation experiment, doing away with the GAT sublayer reduces the model’s AUC to 0.9520 and 0.9408 in 5-fold cross-validation and 2-fold pass-validation, respectively. GAT can better capture facts about key nodes via an attention mechanism that assigns unique weights of importance to its neighbors when processing nodes. This mechanism is necessary for improving the model’s prediction accuracy, and the mannequin’s performance in a similar fashion decreases after its removal.
Finally, we explored the function of the BiLSTM module. After eliminating the BiLSTM module, the AUC of the mannequin diminished to 0.9602 and 0.9510 in the 5-fold move validation and 2-fold move validation, respectively. The LSTM module appropriately processes sequence information and can become aware of attainable associations between microbes and diseases by capturing time dependencies. The reminiscence unit of the LSTM can retain lengthy time-dependent information, which is especially essential for examining the viable microbes’ disease. This is mainly necessary for analyzing doable microbe-disease relationships over lengthy periods. The elimination of this module resulted in a considerable reduction in the predictive energy of the model, in addition to demonstrating the necessary function of the BiLSTM module in the DuGEL model.
Overall, every sub-module had a practical impact on the expected performance of the DuGEL model. The GCN and GAT successfully extracted complicated associations between microbes and diseases through shooting spatial features and node importance statistics in the diagram structure, while the BiLSTM module furthermore desirable the predictive capacity of the mannequin via processing sequence features. The effects of the ablation experiments validate the effectiveness and necessity of these sub-modules and reveal the rationality and superiority of the DuGEL model sketch in the microbe-disease affiliation prediction task.3.6.
In this section, we selected three diseases, kidney stones, eczema, and ileal Crohn’s disease, as case studies for HMDAD to validate our model’s performance further. Specifically, we ranked these three relevant microorganisms in the prediction score and selected the top 20. Then, we evaluated the predictive performance of DuGEL by searching the literature. Among the common diseases, kidney stones cause severe back or abdominal pain accompanied by nausea, vomiting, hematuria, and other symptoms (Stevens, 2018). In recent years, kidney stones have been on the rise. It is prevalent in young adult males (Stamatelou and Goldfarb, 2023). A diverse microbial community exists around renal stones; changes in intestinal and urinary microorganisms may cause the occurrence and development of renal stones. Clostridium difficile, Bifidobacterium, and others are more closely associated with kidney stone occurrence (Miller et al., 2022). As shown in Table 7, the relevance of 17 of the top 20 candidate kidney stones-associated microorganisms predicted by DuGEL has been confirmed by previous publications.
In addition, eczema is an inflammatory skin disease. According to many studies, microorganisms are strongly associated with eczema (Zimmermann et al., 2019). People with eczema have a less diverse and less stable skin microbiome than those without. This means the balance of beneficial and harmful bacteria on the skin is disrupted, making it more susceptible to infection and inflammation (Flowers and Grice, 2020). In addition, the presence of Staphylococcus aureus is associated with more severe eczema symptoms (Chapsa et al., 2023), suggesting a direct link between this bacteria and the condition. As shown in Table 8 below, it is evident that existing publications have confirmed 17 of the 20 potential eczema-associated microorganisms predicted by DuGEL.
Ileal Crohn’s disease is an inflammatory bowel disease. It causes swelling of the tissues of the digestive tract, which may lead to abdominal pain, severe diarrhea, fatigue, weight loss, and malnutrition (Fakhoury et al., 2014). The degree of symptoms ranges from mild to severe and usually comes on gradually, but sometimes, it can come on suddenly without warning. The cause of ileal Crohn’s disease is still unknown, but it is often assumed that a virus or bacteria may trigger Crohn’s disease. This paper presents a case study of ileal Crohn’s disease. As shown in Table 9, it is clear that 18 of the top 20 microorganisms associated with ileal Crohn’s disease have been confirmed in the published literature.
Microorganisms play an important role in our lives and exist in countless numbers and diversity. Investigating the potential link between microorganisms and diseases cannot only contribute to the discovery of new therapeutic approaches and preventive strategies but also help advance the field of microbiology and medicine.
In this study, we propose a deep learning model called DuGEL to predict potential microbial disease associations. The DuGEL model combines graph convolutional neural network (GCN), graph attention network (GAT), and long-short-term memory network (LSTM) to efficiently capture and fuse the complex relationships between microbes and diseases. With the dual-channel structure, DuGEL can extract local and global features in the graph structure and enhance the model’s ability to capture critical nodes by assigning different importance weights to neighboring nodes through the attention mechanism. Our comprehensive experiments and case studies consistently show that DuGEL performs very satisfactorily in terms of prediction accuracy.
Although the DuGEL model has been very effective in studying the relationship between microbes and disease, it still has some limitations. Currently, the model relies heavily on the HMDAD and Disbiome datasets. Therefore, future work could focus on expanding the datasets to capture microbe-disease associations more comprehensively and accurately. In addition, the DuGEL model can be applied to drug-target interaction prediction and gene-disease association prediction to validate its broad applicability and effectiveness.
The original contributions presented in the study are included in the article/supplementary material, further inquiries can be directed to the corresponding authors.
JW: Writing–original draft, Writing–review and editing, Conceptualization, Investigation. LX: Data curation, Formal Analysis, Writing–review and editing. LF: Investigation, Software, Writing–review and editing. LW: Funding acquisition, Methodology, Resources, Writing–review and editing. XZ: Funding acquisition, Supervision, Validation, Writing–review and editing.
The author(s) declare that financial support was received for the research, authorship, and/or publication of this article. This work was partly sponsored by the National Natural Science Foundation of China (No. 62272064), the Scientific Research Program of Education Department of Hunan Province (No. 23A0514), the Natural Science Foundation of Hunan Province (No. 2023JJ60185), the Natural Science Foundation of Hunan Province Program (No. 2022JJ50138), the Application-oriented Special Disciplines, Double First-Class University Project of Hunan Province [Xiangjiaotong (2018) 469] and the Hunan Provincial Education Department Scientific Research Project (No. 20B080).
The authors declare that the research was conducted in the absence of any commercial or financial relationships that could be construed as a potential conflict of interest.
The author(s) declare that no Generative AI was used in the creation of this manuscript.
All claims expressed in this article are solely those of the authors and do not necessarily represent those of their affiliated organizations, or those of the publisher, the editors and the reviewers. Any product that may be evaluated in this article, or claim that may be made by its manufacturer, is not guaranteed or endorsed by the publisher.
Abuin-Denis, L., Piloto-Sardiñas, E., Maître, A., Wu-Chuang, A., Mateos-Hernández, L., Obregon, D., et al. (2024). Exploring the impact of Anaplasma phagocytophilum on colonization resistance of Ixodes scapularis microbiota using network node manipulation. Curr. Res. Parasitol. and Vector-Borne Dis. 5, 100177. doi:10.1016/j.crpvbd.2024.100177
Afshari, S. S., Enayatollahi, F., Xu, X., and Liang, X. (2022). Machine learning-based methods in structural reliability analysis: a review. Reliab. Eng. and Syst. Saf. 219, 108223. doi:10.1016/j.ress.2021.108223
Afzal, S., Ziapour, B. M., Shokri, A., Shakibi, H., and Sobhani, B. (2023). Building energy consumption prediction using multilayer perceptron neural network-assisted models; comparison of different optimization algorithms. Energy 282, 128446. doi:10.1016/j.energy.2023.128446
Anisman, H., Hayley, S., and Kusnecov, A. W. (2018). The immune system and mental health. Academic Press. doi:10.1111/cei.13334
Baranwal, M., Clark, R. L., Thompson, J., Sun, Z., Hero, A. O., and Venturelli, O. S. (2022). Recurrent neural networks enable design of multifunctional synthetic human gut microbiome dynamics. Elife 11, e73870. doi:10.7554/eLife.73870
Barr, J. J. (2017). A bacteriophages journey through the human body. Immunol. Rev. 279 (1), 106–122. doi:10.1111/imr.12565
Bessadok, A., Mahjoub, M. A., and Rekik, I. (2022). Graph neural networks in network neuroscience. IEEE Trans. Pattern Analysis Mach. Intell. 45 (5), 5833–5848. doi:10.1109/tpami.2022.3209686
Bocci, V. (1992). The neglected organ: bacterial flora has a crucial immunostimulatory role. Perspect. Biol. Med. 35 (2), 251–260. doi:10.1353/pbm.1992.0004
Burr, A. H., Bhattacharjee, A., and Hand, T. W. (2020). Nutritional modulation of the microbiome and immune response. J. Immunol. 205 (6), 1479–1487. doi:10.4049/jimmunol.2000419
Chapsa, M., Rönsch, H., Löwe, T., Gunzer, F., Beissert, S., and Bauer, A. (2023). The role of bacterial colonisation in severity, symptoms and aetiology of hand eczema: the importance of Staphylococcus aureus and presence of commensal skin flora. Contact Dermat. 89 (4), 270–276. doi:10.1111/cod.14384
Chatzianastasis, M., Lutzeyer, J., Dasoulas, G., and Vazirgiannis, M. (2023). “Graph ordering attention networks,” in Proceedings of the AAAI Conference on Artificial Intelligence, USA, February 2023, 7006–7014. doi:10.1609/aaai.v37i6.25856
Chen, W., Feng, F., Wang, Q., He, X., Song, C., Ling, G., et al. (2021). Catgcn: graph convolutional networks with categorical node features, IEEE Trans. Knowl. Data Eng. 35(4): 3500–3511. doi:10.1016/j.apcatb.2018.01.024
Chen, X., Huang, Y.-A., You, Z.-H., Yan, G.-Y., and Wang, X.-S. (2017). A novel approach based on KATZ measure to predict associations of human microbiota with non-infectious diseases. Bioinformatics 33 (5), 733–739. doi:10.1093/bioinformatics/btw715
Cui, Y., Jia, M., Lin, T.-Y., Song, Y., and Belongie, S. (2019). “Class-balanced loss based on effective number of samples,” in Proceedings of the IEEE/CVF conference on computer vision and pattern recognition, USA, June 15th, 2025, 9268–9277. doi:10.1109/CVPR.2019.00949
Du, H., Yu, R., Bai, L., and Wang, W. (2024). Learning structure perception MLPs on graphs: a layer-wise graph knowledge distillation framework, Int. J. Mach. Learn. Cybern. 15 4357–4372. doi:10.1007/s13042-024-02150-2
Fakhoury, M., Negrulj, R., Mooranian, A., and Al-Salami, H. (2014). Inflammatory bowel disease: clinical aspects and treatments. J. Inflamm. Res. 7, 113–120. doi:10.2147/JIR.S65979
Fan, Y., Chen, M., Zhu, Q., and Wang, W. (2020). Inferring disease-associated microbes based on multi-data integration and network consistency projection. Front. Bioeng. Biotechnol. 8, 831. doi:10.3389/fbioe.2020.00831
Feng, S., Zhao, L., Shi, H., Wang, M., Shen, S., and Wang, W. (2023). One-dimensional VGGNet for high-dimensional data. Appl. Soft Comput. 135, 110035. doi:10.1016/j.asoc.2023.110035
Flowers, L., and Grice, E. A. (2020). The skin microbiota: balancing risk and reward. Cell. host and microbe 28 (2), 190–200. doi:10.1016/j.chom.2020.06.017
Fu, S., Tian, Y., Tang, J., and Liu, X. (2023). Cost-sensitive learning with modified Stein loss function. Neurocomputing 525, 57–75. doi:10.1016/j.neucom.2023.01.052
Gilbert, J. A., Quinn, R. A., Debelius, J., Xu, Z. Z., Morton, J., Garg, N., et al. (2016). Microbiome-wide association studies link dynamic microbial consortia to disease. Nature 535 (7610), 94–103. doi:10.1038/nature18850
Grasso, L. L., Martino, D. C., and Alduina, R. (2016). Production of antibacterial compounds from Actinomycetes. Actinobacteria-basics Biotechnol. Appl. 7, 177–198. doi:10.5772/61525
Heintz-Buschart, A., and Wilmes, P. (2018). Human gut microbiome: function matters. Trends Microbiol. 26 (7), 563–574. doi:10.1016/j.tim.2017.11.002
Hoffmann, A. R., Proctor, L., Surette, M., and Suchodolski, J. (2016). The microbiome: the trillions of microorganisms that maintain health and cause disease in humans and companion animals, Veterinary pathol. 53(1): 10–21. doi:10.1177/0300985815595517
Islam, M. A., Haque, M. A., Rahman, M. A., Hossen, F., Reza, M., Barua, A., et al. (2022). A review on measures to rejuvenate immune system: Natural mode of protection against coronavirus infection. Front. Immunol. 13, 837290. doi:10.3389/fimmu.2022.837290
Jagannathan, S. V., Manemann, E. M., Rowe, S. E., Callender, M. C., and Soto, W. (2021). Marine actinomycetes, new sources of biotechnological products. Mar. Drugs 19 (7), 365. doi:10.3390/md19070365
Janssens, Y., Nielandt, J., Bronselaer, A., Debunne, N., Verbeke, F., Wynendaele, E., et al. (2018). Disbiome database: linking the microbiome to disease. BMC Microbiol. 18, 50–56. doi:10.1186/s12866-018-1197-5
Jin, D., Song, X., Yu, Z., Liu, Z., Zhang, H., Cheng, Z., et al. (2021). “Bite-gcn: a new GCN architecture via bidirectional convolution of topology and features on text-rich networks,” in Proceedings of the 14th ACM International Conference on Web Search and Data Mining, China, 12 October 2021, 157–165. doi:10.1145/3437963.3441774
Jin, H., Hu, G., Sun, C., Duan, Y., Zhang, Z., Liu, Z., et al. (2022). mBodyMap: a curated database for microbes across human body and their associations with health and diseases. Nucleic Acids Res. 50 (D1), D808–D816. doi:10.1093/nar/gkab973
Jung, S. M., and Park, P. (2017). Stabilization of a bias-compensated normalized least-mean-square algorithm for noisy inputs. IEEE Trans. Signal Process. 65 (11), 2949–2961. doi:10.1109/TSP.2017.2675865
Kumar, A., Mishra, S., Singh, S. S., Singh, K., and Biswas, B. (2020). Link prediction in complex networks based on significance of higher-order path index (SHOPI). Phys. A Stat. Mech. its Appl. 545, 123790. doi:10.1016/j.physa.2020.124289
Li, H., Wang, Y., Zhen, Z. Z., Tan, Y., and Wang, L. (2020). Identifying microbe-disease association based on a novel back-propagation neural network model. IEEE/ACM Trans. Comput. Biol. Bioinforma. PP (99), 1. doi:10.1109/TCBB.2020.2986459
Li, X., Sun, L., Ling, M., and Peng, Y. (2023). A survey of graph neural network based recommendation in social networks. Neurocomputing 549, 126441. doi:10.1016/j.neucom.2023.126441
Liang, D., Leung, R.K.-K., Guan, W., and Au, W. W. (2018). Involvement of gut microbiome in human health and disease: brief overview, knowledge gaps and research opportunities. Gut Pathog. 10, 3–9. doi:10.1186/s13099-018-0230-4
Liu, S., Qi, L., Qin, H., Shi, J., and Jia, J. (2018). “Path aggregation network for instance segmentation,” in Proceedings of the IEEE conference on computer vision and pattern recognition, USA, 18-23 June 2018, 8759–8768. doi:10.1109/CVPR.2018.00913
Long, Y., Luo, J., Zhang, Y., and Xia, Y. (2021). Predicting human microbe–disease associations via graph attention networks with inductive matrix completion. Briefings Bioinforma. 22 (3), bbaa146. doi:10.1093/bib/bbaa146
Luo, J., and Long, Y. (2018). NTSHMDA: prediction of human microbe-disease association based on random walk by integrating network topological similarity. IEEE/ACM Trans. Comput. Biol. Bioinforma. 17 (4), 1341–1351. doi:10.1109/TCBB.2018.2883041
Ma, W., Zhang, L., Zeng, P., Huang, C., Li, J., Geng, B., et al. (2017). An analysis of human microbe–disease associations. Briefings Bioinforma. 18 (1), 85–97. doi:10.1093/bib/bbw005
Ma, Y., and Liu, Q. (2022). Generalized matrix factorization based on weighted hypergraph learning for microbe-drug association prediction. Comput. Biol. Med. 145, 105503. doi:10.1016/j.compbiomed.2022.105503
Malla, M. A., Dubey, A., Kumar, A., Yadav, S., Hashem, A., and Abd_Allah, E. F. (2019). Exploring the human microbiome: the potential future role of next-generation sequencing in disease diagnosis and treatment. Front. Immunol. 9, 2868. doi:10.3389/fimmu.2018.02868
Mao, A., Mohri, M., and Zhong, Y. (2023). “Cross-entropy loss functions: theoretical analysis and applications,” in International Conference on Machine Learning, China, 14 Apr 2023 (PMLR), 23803–23828. doi:10.48550/ARXIV.2304.07288
Marcos-Zambrano, L. J., Karaduzovic-Hadziabdic, K., Loncar Turukalo, T., Przymus, P., Trajkovik, V., Aasmets, O., et al. (2021). Applications of machine learning in human microbiome studies: a review on feature selection, biomarker identification, disease prediction and treatment. Front. Microbiol. 12, 634511. doi:10.3389/fmicb.2021.634511
Marsh, P., and Zaura, E. (2017). Dental biofilm: ecological interactions in health and disease. J. Clin. periodontology 44, S12–S22. doi:10.1111/jcpe.12679
Miller, A. W., Penniston, K. L., Fitzpatrick, K., Agudelo, J., Tasian, G., and Lange, D. (2022). Mechanisms of the intestinal and urinary microbiome in kidney stone disease. Nat. Rev. Urol. 19 (12), 695–707. doi:10.1038/s41585-022-00647-5
Montaner, J., Ramiro, L., Simats, A., Tiedt, S., Makris, K., Jickling, G. C., et al. (2020). Multilevel omics for the discovery of biomarkers and therapeutic targets for stroke. Nat. Rev. Neurol. 16 (5), 247–264. doi:10.1038/s41582-020-0350-6
Munikoti, S., Agarwal, D., Das, L., Halappanavar, M., and Natarajan, B. (2023). Challenges and opportunities in deep reinforcement learning with graph neural networks: a comprehensive review of algorithms and applications. IEEE Trans. neural Netw. Learn. Syst. 35, 15051–15071. doi:10.1109/tnnls.2023.3283523
Najafabadi, M. M., Villanustre, F., Khoshgoftaar, T. M., Seliya, N., Wald, R., and Muharemagic, E. (2015). Deep learning applications and challenges in big data analytics. J. big data 2, 1–21. doi:10.1186/s40537-014-0007-7
Pickard, J. M., Zeng, M. Y., Caruso, R., and Núñez, G. (2017). Gut microbiota: role in pathogen colonization, immune responses, and inflammatory disease, Immunol. Rev. 279(1): 70–89. doi:10.1111/imr.12567
Sanz, Y., Olivares, M., Moya-Pérez, Á., and Agostoni, C. (2015). Understanding the role of gut microbiome in metabolic disease risk. Pediatr. Res. 77 (1), 236–244. doi:10.1038/pr.2014.170
Shen, D., Liu, J., Wu, Z., Yang, J., and Xiao, L. (2021). ADMM-HFNet: a matrix decomposition-based deep approach for hyperspectral image fusion. IEEE Trans. Geoscience Remote Sens. 60, 1–17. doi:10.1109/TGRS.2021.3112181
Shen, X., Zhu, H., Jiang, X., Hu, X., and Yang, J. (2018). “A novel approach based on bi-random walk to predict microbe-disease associations,” Intelligent Computing Methodologies: 14th International Conference, ICIC 2018, Wuhan, China, August 15-18, 2018. Springer, 746–752. doi:10.1039/C8EE02656D
Shen, Z., Jiang, Z., and Bao, W. (2017). “CMFHMDA: collaborative matrix factorization for human microbe-disease association prediction,” in Intelligent Computing Theories and Application: 13th International Conference, ICIC 2017, Liverpool, UK, August 7-10, 2017 (Springer), 261–269. doi:10.1007/978-3-319-63312-1_24
Stamatelou, K., and Goldfarb, D. S. (2023). Epidemiology of kidney stones, Healthcare. MDPI, 424. doi:10.3390/healthcare11030424
Stevens, S. (2018). Obstructive kidney disease. Nurs. Clin. 53 (4), 569–578. doi:10.1016/j.cnur.2018.07.007
Wang, F., Huang, Z.-A., Chen, X., Zhu, Z., Wen, Z., Zhao, J., et al. (2017). LRLSHMDA: laplacian regularized least squares for human microbe–disease association prediction. Sci. Rep. 7 (1), 7601. doi:10.1038/s41598-017-08127-2
Wang, J., Mo, H., Wang, F., and Jin, F. (2011). Exploring the network structure and nodal centrality of China’s air transport network: a complex network approach. J. Transp. Geogr. 19 (4), 712–721. doi:10.1016/j.jtrangeo.2010.08.012
Wang, L., Wang, Y., Li, H., Feng, X., Yuan, D., and Yang, J. (2019). A bidirectional label propagation based computational model for potential microbe-disease association prediction. Front. Microbiol. 10, 684. doi:10.3389/fmicb.2019.00684
Wang, L., Yang, X., Kuang, L., Zhang, Z., Zeng, B., and Chen, Z. (2023). Graph convolutional neural network with multi-layer attention mechanism for predicting potential microbe-disease associations. Curr. Bioinforma. 18 (6), 497–508. doi:10.2174/1574893618666230316113621
Wang, M., Wu, L., Li, M., Wu, D., Shi, X., and Ma, C. (2022). Meta-learning based spatial-temporal graph attention network for traffic signal control. Knowledge-based Syst. 250, 109166. doi:10.1016/j.knosys.2022.109166
Wu, K., Wu, J., Feng, L., Yang, B., Liang, R., Yang, S., et al. (2021). An attention-based CNN-LSTM-BiLSTM model for short-term electric load forecasting in integrated energy system. Int. Trans. Electr. Energy Syst. 31 (1), e12637. doi:10.1002/2050-7038.12637
Wu, Z., Li, W., Liu, G., and Tang, Y. (2018). Network-based methods for prediction of drug-target interactions. Front. Pharmacol. 9, 1134. doi:10.3389/fphar.2018.01134
Yoo, G., Kim, H., and Hong, S. (2023). Prediction of cognitive load from electroencephalography signals using long short-term memory network. Bioengineering 10 (3), 361. doi:10.3390/bioengineering10030361
Yu, S., Wang, H., Hua, M., Liang, C., and Sun, Y. (2024). Sparse graph cascade multi-kernel fusion contrastive learning for microbe–disease association prediction. Expert Syst. Appl. 252, 124092. doi:10.1016/j.eswa.2024.124092
Yuan, X., Huang, L., Li, L., Wang, K., Wang, Y., Ye, L., et al. (2023). Multiscale dynamic feature learning for quality prediction based on hierarchical sequential generative network. IEEE Sensors J. 23, 19561–19570. doi:10.1109/jsen.2023.3290163
Zhang, C., Yang, Z., He, X., and Deng, L. (2020). Multimodal intelligence: representation learning, information fusion, and applications. IEEE J. Sel. Top. Signal Process. 14 (3), 478–493. doi:10.1109/JSTSP.2020.2987728
Zhang, W., Yang, W., Lu, X., Huang, F., and Luo, F. (2018). The bi-direction similarity integration method for predicting microbe-disease associations. Ieee Access2018 6, 38052–38061. doi:10.1109/ACCESS.2018.2851751
Zhang, Z., Zhang, J., Fan, C., Tang, Y., and Deng, L. (2017). KATZLGO: large-scale prediction of LncRNA functions by using the KATZ measure based on multiple networks. IEEE/ACM Trans. Comput. Biol. Bioinforma. 16 (2), 407–416. doi:10.1109/tcbb.2017.2704587
Zhou, X., Zhang, T., Cheng, C., and Song, S. (2023). Dynamic multichannel fusion mechanism based on a graph attention network and BERT for aspect-based sentiment classification. Appl. Intell. 53 (6), 6800–6813. doi:10.1007/s10489-022-03851-3
Zhu, B., Wang, X., and Li, L. (2010). Human gut microbiome: the second genome of human body. Protein and Cell. 1 (8), 718–725. doi:10.1007/s13238-010-0093-z
Keywords: long and short-term memory networks, graph attention networks, microbe-disease associations, graph convolutional neural networks, full connectivity
Citation: Wu J, Xiao L, Fan L, Wang L and Zhu X (2025) Dual graph-embedded fusion network for predicting potential microbe-disease associations with sequence learning. Front. Genet. 16:1511521. doi: 10.3389/fgene.2025.1511521
Received: 15 October 2024; Accepted: 15 January 2025;
Published: 11 February 2025.
Edited by:
Marco Mesiti, University of Milan, ItalyReviewed by:
Gianvito Pio, University of Bari Aldo Moro, ItalyCopyright © 2025 Wu, Xiao, Fan, Wang and Zhu. This is an open-access article distributed under the terms of the Creative Commons Attribution License (CC BY). The use, distribution or reproduction in other forums is permitted, provided the original author(s) and the copyright owner(s) are credited and that the original publication in this journal is cited, in accordance with accepted academic practice. No use, distribution or reproduction is permitted which does not comply with these terms.
*Correspondence: Lei Wang, d2FuZ2xlaUB4dHUuZWR1LmNu; Xianyou Zhu, enh5QGh5bnUuZWR1LmNu
Disclaimer: All claims expressed in this article are solely those of the authors and do not necessarily represent those of their affiliated organizations, or those of the publisher, the editors and the reviewers. Any product that may be evaluated in this article or claim that may be made by its manufacturer is not guaranteed or endorsed by the publisher.
Research integrity at Frontiers
Learn more about the work of our research integrity team to safeguard the quality of each article we publish.