- 1Huzhou Central Hospital, The Fifth School of Clinical Medicine of Zhejiang Chinese Medical University, Huzhou, China
- 2Huzhou Central Hospital, The Affiliated Central Hospital of Huzhou University, Huzhou, China
Background: Atrial fibrillation (AF) is a prevalent cardiac arrhythmia associated with substantial morbidity and mortality. Oxidative stress (OS) has been implicated in the pathogenesis of AF, suggesting that targeting OS-related genes could offer novel therapeutic opportunities. This study aimed to identify causal OS-related genes contributing to AF through a comprehensive multi-omics Summary-based Mendelian Randomization (SMR) approach.
Methods: This study integrated data from genome-wide association studies (GWAS) with methylation quantitative trait loci (mQTL), expression QTL (eQTL), and protein QTL (pQTL) to explore the relationships between oxidative stress-related (OS-related) genes and AF risk. Genes associated with oxidative stress and AF were obtained from the Nielsen et al. study (discovery) and the FinnGen study (replication). The SMR analysis and HEIDI test were utilized to assess causal associations, followed by Bayesian co-localization analysis (PPH4 > 0.5) to confirm shared causal variants. Multi-omics data were employed to analyze the associations within mQTL-eQTL pathways. A two-sample MR analysis was conducted for sensitivity verification. The significance of findings was determined using a false discovery rate (FDR) < 0.05 and p_HEIDI > 0.01.
Results: At the DNA methylation level, 19 CpG sites near 7 unique genes were found to have causal effects on AF and strong co-localization evidence support (PPH4 > 0.70). At the gene expression level, six oxidative stress-related genes from eQTLGen and three from GTEx (v8), including TNFSF10, CDKN1A, ALOX15, TTN, PTK2, ALB, KCNJ5, and CASQ2, were found to have causal effects on AF in the sensitivity and co-localization analyses (PPH4 > 0.50). At the circulating protein level, both ALAD (OR 0.898, 95% CI 0.845–0.954, PPH4 = 0.67) and APOH (OR 0.896, 95% CI 0.844–0.952, PPH4 = 0.93) were associated with a lower risk of AF, and APOH was validated in the replication group. After integrating the multi-omics data between mQTL and eQTL, we identified two oxidative stress-related genes, TTN and CASQ2. The methylation of cg09915519 and cg10087519 in TTN was associated with higher expression of TTN and a lower risk of AF, which aligns with the negative effect of TTN gene expression on AF risk. TTN may play a protective role in AF.
Conclusion: This study identified several OS-related genes, particularly TTN, as having causal roles in AF, which were verified across three-omics pathways. The findings underscore the importance of these genes in AF pathogenesis and highlight their potential as therapeutic targets. The integration of multi-omics data provides a comprehensive understanding of the molecular mechanisms underlying AF, paving the way for targeted therapeutic strategies.
Introduction
Atrial fibrillation is the most common sustained cardiac arrhythmia encountered in clinical practice, significantly contributing to morbidity and mortality globally (Sagris et al., 2021). The pathogenesis of AF is complex, involving various factors such as electrical and mechanical changes in the atria, inflammation, oxidative stress, and fibrosis (Gaudino et al., 2023). Inflammation has been well-documented as a critical factor in the development and progression of AF, leading to atrial fibrosis and structural remodeling. Similarly, OS, characterized by the excessive production of reactive oxygen species (ROS), has been implicated in atrial remodeling and the onset of AF (Ramos-Mondragón et al., 2023).
Previous studies have highlighted the interplay between inflammation and OS in the pathophysiology of AF (Ramos-Mondragón et al., 2023; Balan et al., 2024). Inflammatory markers such as high-sensitivity C-reactive protein and galectin-3 have been associated with AF incidence and recurrence, suggesting their role in atrial fibrosis and remodeling (Noubiap et al., 2021). Additionally, OS markers like myeloperoxidase and oxidized low-density lipoprotein have been linked to cardiac tissue damage and AF (Rafaqat et al., 2021). Despite these associations, the relative contributions of inflammation and OS to AF and their potential interactions remain not fully understood.
Recent advancements in multi-omics technologies provide a unique opportunity to investigate the molecular mechanisms underlying AF by integrating genomic, epigenomic, and proteomic data. Summary-based Mendelian randomization is a powerful analytical approach that leverages genetic variants as instrumental variables to infer causal relationships between risk factors and disease outcomes. By combining SMR with multi-omics data, it is possible to identify causal genes and pathways involved in AF, offering insights into potential therapeutic targets.
In this study, we aimed to identify therapeutic genes for AF by GWAS with mQTL, eQTL, and pQTL data using a comprehensive SMR approach. We hypothesized that OS-related genes play a causal role in AF and that their identification could provide novel targets for therapeutic intervention. Through rigorous statistical analysis and integration of multi-omics data, we sought to elucidate the molecular mechanisms linking OS to AF and highlight key genes for further investigation and potential therapeutic development.
Methods
Study design
Figure 1 illustrates the comprehensive design of the study. Initially, we identified 817 OS-related genes from the GeneCards database. Next, we integrated GWAS of AF with blood expression QTL (eQTL), methylation QTL (mQTL), and protein QTL (pQTL) summary data for analysis using the SMR method. Then, we identified potential causal OS-related genes through SMR analysis and Bayesian co-localization analysis, we also analyzed the molecular networks of OS-related genes within mQTL and eQTL through SMR analysis. Finally, we validated the robustness of the primary findings using a two-sample MR analysis (Supplementary Table S17).
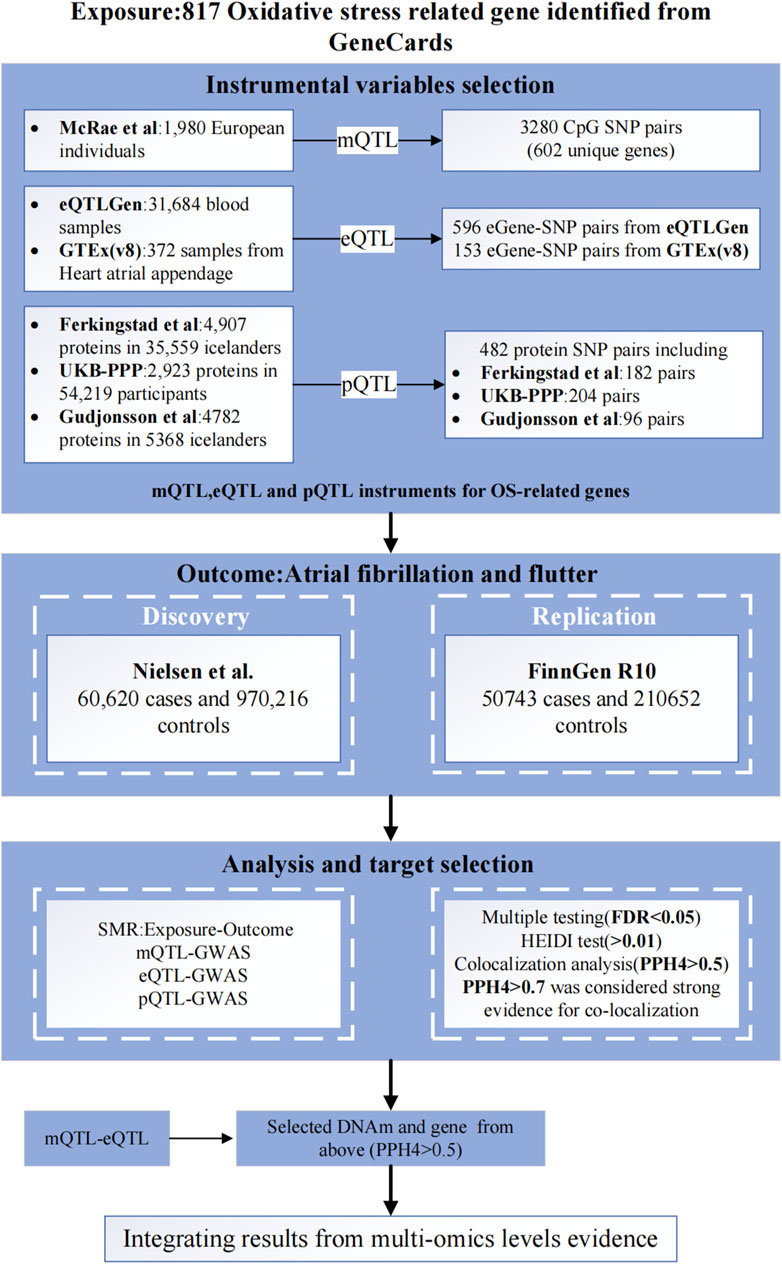
Figure 1. Study design. SMR, summary-based Mendelian randomization; QTL, quantitative trait loci; SNP, single nucleotide polymorphisms; OS, oxidative stress; GWAS, Genome-Wide Association Study; FDR, False Discovery Rate; PPH4, posterior probability of H4.
Data sources
The GWAS by Nielsen et al. encompassed 1,030,836 participants (60,620 AF cases and 970,216 controls) of European descent (Nielsen et al., 2018). The median age was not provided, and 53% of the participants were women. Summary-level data of genetic associations with AF were also obtained from the publicly available R10 data release of the FinnGen study (Kurki et al., 2023). The diagnosis of AF was based on ICD codes and confirmed by Social Insurance Institution codes, with a total of 50,743 AF cases and 210,652 controls. The discovery stage of the research utilized the Nielsen et al. study, while the replication stage used data from the FinnGen study.
By integrating multi-omic data, it is possible to illuminate the molecular networks underlying mitochondrial dysfunction. Quantitative trait loci (QTL) studies facilitate the understanding of associations between single nucleotide polymorphisms (SNPs) and levels of DNA methylation, gene expression, and protein abundance.
McRae et al. reported data on SNP-CpG associations in blood from a study of 1,980 individuals of European descent, focusing on mQTLs (McRae et al., 2018). These data were normalized using a generalized linear model that accounted for factors such as chip type, sex, age, age squared, and their interactions (McRae et al., 2018).
Gene expression data were sourced from the eQTLGen consortium, which offered a substantial sample size (n = 31,684) to identify SNPs associated with the expression of genes targeted by the corresponding plasma proteins (Võsa et al., 2021).
For protein abundance, summary statistics were obtained from three independent pQTL studies conducted by Ferkingstad et al., Gudjonsson et al., and the UKB-PPP. For Ferkingstad et al., 28,191 genetic associations (p < 1.8 × 10−9) for 4,907 aptamers were identified in 35,559 Icelanders based on the SomaScan platform (Ferkingstad et al., 2021). For Gudjonsson et al., 7,506,463 genetic associations (p < 1.046 × 10−11) for 4,782 serum proteins encoded by 4,135 unique human genes in the population-based AGES cohort of 5,368 elderly Icelanders were measured by the slow-off rate modified aptamer (SOMAmer) platform (Gudjonsson et al., 2022). For the UKB-PPP, a total of 23,588 primary (sentinel) genetic associations (p < 1.7 × 10−11, clumping ±1 Mb, r2 < 0.8) for 2,923 proteins in 54,219 participants from the UK Biobank Pharma Proteomics Project (UKB-PPP) were identified using the Olink platform (Sun et al., 2023). The protein levels were adjusted using rank-inverse normal transformation, taking into account age, sex, and sample storage age.
The tissue-specific expression of target genes that could potentially cause AF was further assessed using tissue-specific eQTL data from the Genotype Tissue Expression (GTEx) web portal (https://gtexportal.org/home/). The GTEx dataset includes information from 838 donors and 17,382 samples spanning 52 tissues and 2 cell lines (GTEx Consortium., 2020). This extensive resource enabled the exploration of gene expression across a variety of tissues, providing insights into the role of specific genes in AF pathogenesis.
OS-related genes were identified from the GeneCards database (version 5.10, https://www.genecards.org) using the keyword “oxidative stress” and a relevance score of 7 or higher, following previously established methods (Qiu et al., 2020; Fan et al., 2022; Sun et al., 2022). Ultimately, we identified 817 OS-related genes (Supplementary Table S1).
After the filtering process for OS-related genes, we identified 602 genes associated with methylation, 596 with expression from eQTLGen, 153 with expression from GTEx (v8), and 482 proteins with available instruments (mQTLs, eQTLs, and pQTLs with P < 5 × 10−8) from the respective datasets.
Summary-data-based MR analysis
SMR analysis was employed to assess the association between OS-related gene methylation, expression, and protein abundance with the risk of atrial fibrillation and flutter (Zhu et al., 2016). We constructed a hypothetical model of the mediation mechanism in which a single SNP influences a trait by altering the DNA methylation (DNAm) level, which in turn regulates the expression levels of a functional gene. Therefore, we also used the SMR to analyze the OS-related DNAm and gene within the multi-omics respectively (mQTL-eQTL). Utilizing the top associated cis-QTLs, the SMR method achieved significantly greater statistical power compared to conventional MR analysis, particularly when exposure and outcome data are derived from two independent samples with large sample sizes. The top associated cis-QTLs were selected by considering a window centered around each relevant gene (±1,000 kb) and meeting a p-value threshold of 5.0 × 10−8. SNPs with allele frequency differences exceeding the specified threshold (0.2 in this study) between any pairwise datasets, including the LD reference sample, the QTL summary data, and the outcome summary data, were excluded. The heterogeneity in the dependent instrument (HEIDI) test was employed to distinguish between pleiotropy and linkage, with P_HEIDI < 0.01 indicating likely pleiotropy, leading to exclusion from the analysis. P-values were adjusted to control the false discovery rate (FDR) at α = 0.05 using the Benjamini–Hochberg method. Associations with FDR-corrected P-values <0.05 and P_HEIDI >0.01 were subjected to co-localization analysis.
The SMR analysis and HEIDI test were performed using version 1.3.1 of the SMR software (https://yanglab.westlake.edu.cn/software/smr/#Download). Two-sample MR analysis were conducted using the “TwoSampleMR (version 0.5.6)” package of the R software (version 4.2.2).
Co-localization analysis
We conducted co-localization analyses to identify shared causal variants between AF and identified OS-related mQTLs, eQTLs, or pQTLs using the coloc R package (version 5.2.2) (Giambartolomei et al., 2014). In these analyses, five distinct posterior probabilities are reported, corresponding to the following five hypotheses: 1) no causal variants for either of the two traits (H0); 2) a causal variant for gene expression only (H1); 3) a causal variant for disease risk only (H2); 4) distinct causal variants for each trait (H3); and 5) a shared causal variant for both traits (H4). For the co-localization of pQTL-GWAS, eQTL-GWAS, and mQTL-GWAS, the colocalization region windows were all set at ±1,000 kb, ±1,000 kb, ±500 kb, respectively. The prior probabilities that the causal variants are associated with only trait 1, only trait 2 (AF), and both are respectively set at 1.0 × 10−4, 1.0 × 10−4, and 1.0 × 10−5. A posterior probability of H4 (PPH4) > 0.5 was considered evidence of co-localization, with this threshold corresponding to a false discovery rate of P-values <0.05, thereby reinforcing the evidence for a causal relationship (Huang et al., 2023).
Integrating results from multi-omics level of evidence
To obtain a full picture of the associations between the regulation of OS-related genes and AF at the genomic level, we conducted SMR analysis of the causal associations within OS-related gene methylation and expression, in order to explore the basic mechanism (FDR < 0.05, P_HEIDI > 0.05). We focused only on the genes that passed the screening criteria. The identification of the final putative causal relationships was defined as: 1) false discovery rate (FDR) < 0.05 in all three-step SMR; 2) P_HEIDI > 0.01 in mQTL-GWAS and eQTL-GWAS SMR, P_HEIDI > 0.05 in mQTL-eQTL SMR; 3) PPH4 > 0.5 in co-localization analysis of eQTL and AF GWAS; 4) the eQTL and mQTL should correspond to the same gene symbol.
Results
Oxidative stress-related gene methylation and AF GWAS data
Results for causal effects of oxidative stress-related gene methylation on AF are visualized in (Table 1). After the removal of associations with P HEIDI <0.01, a total of 346 CpG sites near 159 unique genes passed the marginal significance (PSMR < 0.05) (Supplementary Table S2). After correction for multiple testing (FDR < 0.05), we identified 40 CpG sites near 17 unique genes (Supplementary Table S3). 19 near 7 unique genes were found to have strong co-localization evidence support (PPH4 > 0.70) including MAPT (cg02228913, cg21705961, cg05301556, cg23202277, cg05772917, cg07163735, cg01934064, cg00846647), CRHR1 (cg16228356, cg23762722, cg05727186, cg24063856, cg16642545), TTN (cg09915519, cg10087519), ALPP (cg14659346), CREBBP (cg05194552), GPX4 (cg04903600) and MAP2K2 (cg21124940) (Table 1).
The direction of effect estimates were not always consistent for different CpG sites located in the same gene. For example, one SD increase in genetically predicted MAPT methylation at cg02228913 was associated with a decreased risk of AF (OR 0.967, 95% confidence interval [CI] 0.955–0.979), whereas one SD increase in genetically predicted MAPT methylation at cg07163735 was associated with a higher risk of AF (OR 1.189, 95% CI 1.101–1.284). Among these identified CpG sites, the associations for cg09915519 and cg10087519 near TTN were replicated in FinnGen with strong co-localization evidence support (PPH4 >0.70) (Supplementary Table S4).
Oxidative stress-related gene expression and AF GWAS data
Results for causal effects of oxidative stress related gene expression on AF are presented in (Table 2). In total, 83 associations were identified to be associated with AF at the nominally significant level (PSMR < 0.05, P HEIDI > 0.01) (Supplementary Tab le S5). After multiple testing correction and co-localization analysis (Supplementary Table S6), we selected those genes which PPH4 > 0.5. Genetically predicted higher levels expression of TNFSF10 (OR 1.160, 95% CI 1.074–1.254; PPH4 = 0.65), CDKN1A (OR 1.214, 95% CI 1.125–1.309; PPH4 = 0.64) and ALOX15(OR 1.063, 95% CI 1.028–1.099; PPH4 = 0.60) were positively associated with AF risk. Conversely, genetically predicted higher levels expression of TTN (OR 0.291, 95% CI 0.186–0.454; PPH4 = 0.65), PTK2 (OR 0.794, 95% CI 0.730–0.864; PPH4 = 0.67) and ALB(OR 0.291, 95% CI 0.186–0.454; PPH4 = 0.98) were inversely associated with AF risk (Table 2). Associations for TTN and PTK2 were all replicated in FinnGen, of which the genetically predicted high level expressions were negatively associated with AF risk (OR 0.181, 95% CI 0.098–0.336; OR 0.744, 95% CI 0.661–0.837, respectively). whereas CDKN1A replicated in FinnGen was positively associated with AF risk (OR 1.36, 95% CI 1.21–1.529, Supplementary Table S7).
Tissue-specific validation
Causal associations of the expression of identified genes with AF were further explored in atrial tissue (Table 3). In total, 22associations were identified to be associated with AF at the nominally significant level (PSMR < 0.05, P HEIDI > 0.01) (Supplementary Table S8). After multiple testing correction and co-localization analysis (Supplementary Table S9), genetically predicted expression levels of KCNJ5 was associated with increases in AF risk in atrial appendage (OR 1.293, 95% CI 1.169–1.431; PPH4 = 0.97). In contrast, genetically predicted expression levels of CASQ2 and ALOX15 were associated with reduction in AF risk in atrial appendage tissue (OR 0.652, 95% CI 0.538–0.789; PPH4 = 0.98 and OR 0.915, 95% CI 0.867–0.966; PPH4 = 0.76, respectively). KCNJ5 was replicated in the FinnGen study (Supplementary Table S10).
Oxidative stress-related gene relative proteins and AF GWAS data
There were 36 oxidative stress related proteins from 3 independent researches including Ferkingstad study, Gudjonsson study and UKB-PPP Olink which separately associated with AF risk at PSMR < 0.05 level (Supplementary Table S11). After adjustment for multiple testing, we obtained 2 proteins including ALAD, APOH (Supplementary Table S12). Both ALAD (Ferkingstad study) and APOH(Gudjonsson study) were associated with a lower risk of AF(OR 0.898, 95% CI 0.845–0.954, PPH4 = 0.67; OR 0.896, 95% CI 0.844–0.952, PPH4 = 0.93, respectively). APOH was replicated in the FinnGen study (Supplementary Table S13).
SMR analysis for mQTL and eQTL data
It is well known that gene methylation affects gene expression. Therefore, we proceeded to explore the possible link between DNAm and gene expression by using DNAm as the exposure and transcripts as the outcome. After screening the results by FDR < 0.05 and P HEID > 0.05, we obtained the regulatory relationships for the expression of 252 OS-related genes regulated by 595 DNA methylation CpG sites (Supplementary Table S14). We only analyse the genes which have higher co-localization evidence (PPH4 > 0.5) support with AF (Table 2). Finally we identified 1 OS-related genes regulated by 2 DNA methylation CpG sites (Supplementary Table S16; Figure 2). TTN, there were two significantly associated methylation sites (cg09915519 and cg10087519), two of which were positively correlated with TTN expression and passed the co-localization analysis in mQTL-GWAS (Figure 3).
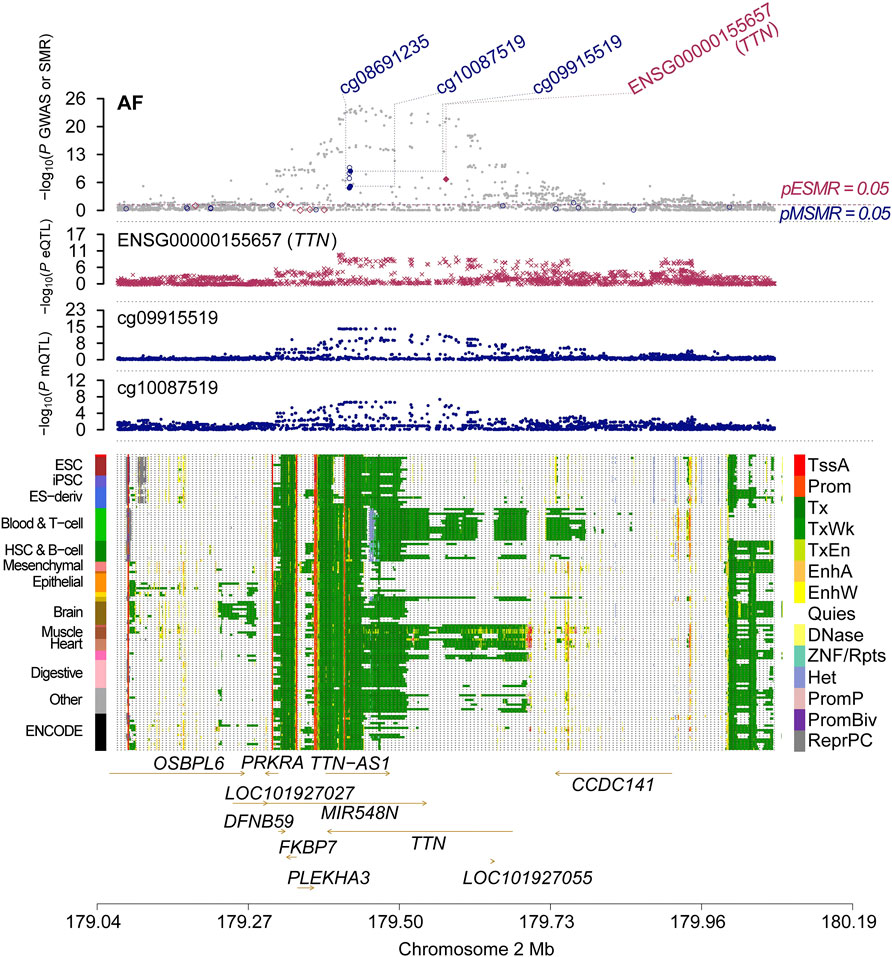
Figure 2. Multi-omics data integration for AF. Results of associations across mQTL, eQTL and GWAS at the TTN locus.
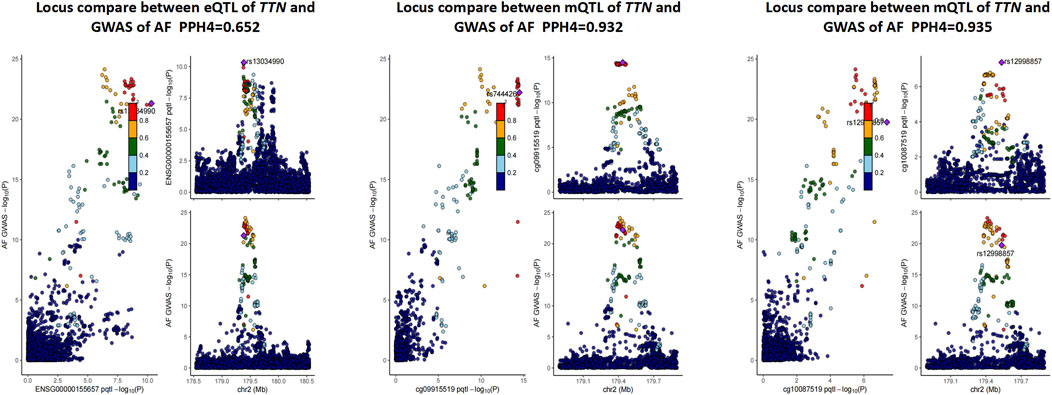
Figure 3. Result of co-localization analysis in mQTL, eQTL of TTN and GWAS of AF. QTL, quantitative trait loci; GWAS, Genome-Wide Association Study; PPH4, posterior probability of H4.
The top plot shows −log10 (p-values) of SNP from GWAS. The red diamonds and blue circles represent −log10 (p-values) from SMR tests for associations of gene expression and DNAm probes with trait, respectively. The solid diamonds and circles are the probes not rejected by the HEIDI test. The second plot shows −log10 (p-values) of the SNP associations for gene expression probe ENSG00000155657 (TTN). The third plot shows −log10 (p-values) of the SNP associations for DNAm probes. The bottom plot shows 14 chromatin state annotations (indicated by colours) of 127 samples from the REMC for different primary cells and tissue types (rows).
SMR analysis for mQTL and eQTL from GTEx(v8) tissue data
After screening the results by FDR < 0.05 and P HEID > 0.05, we obtained the regulatory relationships for the expression of 92 OS-related genes from tissue of heart atrial appendage regulated by 256 DNA methylation CpG sites (Supplementary Table S13). We analyse the genes which have co-localization evidence (PPH4>0.5) support with AF (Table 2). Finally we identified CASQ2 negatively regulated by cg20810993 CpG sites.
Multi-omics level integration
After integrating evidence from multi-omics data, we identified TTN from eQTLGen and CASQ2 from GTEx (v8) with higher evidence linking them to AF through the mQTL to eQTL pathway. We constructed a hypothetical model of the mediation mechanism: a single nucleotide polymorphism (SNP) affects the trait by altering DNA methylation (DNAm) levels, which then regulate the expression of a functional gene. One CpG sites (cg20810993) were excluded because it did not show significant results in the mQTL-GWAS SMR analysis.
In conclusion, the integration analysis results demonstrated that TTN methylation regulated by cg09915519 and cg10087519 increases TTN expression, which is associated with a lower risk of AF (Figure 4).
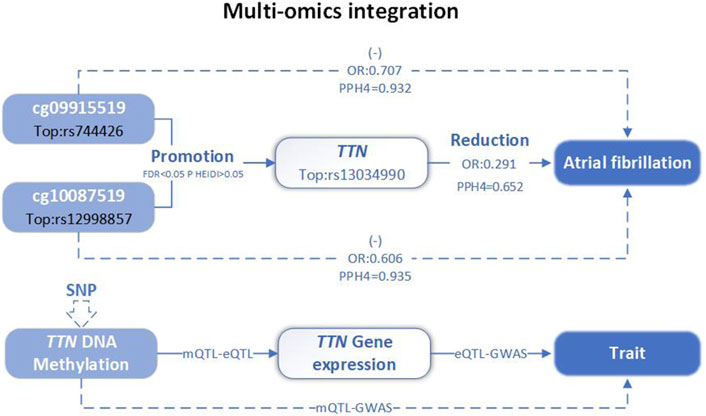
Figure 4. Multi-omics level integration of TTN. SNP, single nucleotide polymorphisms; QTL, quantitative trait loci; Top, top SNP; FDR, false discovery rate; PPH4, posterior probability of H4; OR, odds ratio.
Discussion
In this study, we employed an integrative multi-omics summary-based Mendelian randomization and Bayesian co-localization to identify key oxidative stress-related genes as therapeutic targets for AF. Integration of AF GWAS summary data and mQTL and eQTL for OS-related genes prioritized one gene expression (TTN) and two CpG sites (cg09915519 and cg10087519). Our findings significantly adance the understanding of the genetic underpinnings of AF, particularly in the context of oxidative stress pathways.
TTN (Titin) is an essential structural and functional protein in muscle tissue, particularly within the sarcomeres of cardiac muscle (Loescher et al., 2022). Mutations in the TTN gene, particularly truncating variants (TTNtv), are associated with various cardiomyopathies, most notably dilated cardiomyopathy (Santiago et al., 2021). Research by Akhtar et al. involved 537 individuals from 14 centers, with a median follow-up of 49 months, and concluded that TTNtv is associated with frequent arrhythmias and heart failure, with male sex and left ventricular systolic dysfunction being significant predictors of poor outcomes (Akhtar et al., 2020). TTNtv can lead to sarcomere insufficiency and altered mechanical properties of cardiac muscle (Fomin et al., 2021). These mutations can disrupt the ubiquitin-proteasome system and autophagy pathways, contributing to the accumulation of defective proteins and increased cellular stress (Müller et al., 2021). TTNtv are significantly associated with early-onset AF, especially in patients without traditional risk factors (Andreasen et al., 2020). Yoneda et al. recruited 1,293 participants who underwent whole genome sequencing, focusing on 145 genes associated with cardiomyopathy and arrhythmia syndromes. After screening, the most frequently affected genes included TTN, MYH7, MYH6, LMNA, and KCNQ1, with TTN variants being the most prevalent (Yoneda et al., 2021). The structural abnormalities caused by TTNtv, such as atrial dilation and fibrosis, contribute to the development of AF (Rudaka et al., 2023). TTNtv can act as a monogenic cause of AF, as the European Society of Cardiology reported that TTN gene variants were notably enriched in early-onset lone AF cases (Lazarte et al., 2021). The study concludes that lone AF is associated with an enrichment of rare loss-of-function variants in ventricular cardiomyopathy genes, particularly in the TTN gene, which suggests that atrial cardiomyopathy might be a genetic sub-phenotype of AF (Lazarte et al., 2021).
The expression of the TTN gene is primarily regulated by several CpG sites, with cg09915519 and cg10087519 being the most significant. Grzeczka et al. found that DNA methylation, particularly of certain CpG sites, was found to significantly affect the expression of genes involved in AF. Hypermethylation of key regulatory genes in atrial tissue, such as those involved in ion channel function and fibrosis, was associated with increased risk of AF (Grzeczka et al., 2023).
Ongoing research aims to translate these genetic insights into effective therapeutic strategies. Gene-editing technologies, such as CRISPR/Cas9, hold promise for correcting TTN mutations, thereby restoring normal protein function and enhancing cardiac contractility (Kang et al., 2022). Our findings support the hypothesis that certain benign mutations in TTN may reduce the risk of AF, thereby exerting a protective effect on the pathological processes underlying AF. Additionally, our SMR analysis provided evidence that the upregulation of methylation at cg09915519 and cg10087519 is associated with a decreased risk of AF through increased TTN expression levels.
In the transcriptomic and multi-omics studies of atrial tissue, we found that the expression of CASQ2 is associated with the occurrence of AF (OR 0.652, 95% CI 0.538–0.789, PPH4 = 0.98). CASQ2 (calsequestrin 2) is a calcium-binding protein primarily located in the sarcoplasmic reticulum of cardiac muscle cells. It plays a crucial role in calcium homeostasis by regulating calcium storage and release, which is essential for proper cardiac muscle contraction (Ng et al., 2020). Abnormalities in calcium handling are known to contribute to various cardiac arrhythmias, including ventricular tachycardia (Askarinejad et al., 2024). Studies have shown that altered CASQ2 expression may contribute to the development of AF by affecting the stability of intracellular calcium levels, leading to increased susceptibility to abnormal electrical activity in the atria (Wang et al., 2020). However, we were unable to identify the cg20810993 site from the mQTL-AF GWAS analysis in both the discovery and replication groups, which was expected to regulate the expression of CASQ2.
Our study involved three independent studies searching for the OS-related protein that regulated the risk of AF. APOH was associated with a lower risk of AF (PPH4 = 0.93), a finding that was also replicated in the FinnGen study. APOH, also known as apolipoprotein H or beta-2-glycoprotein I, is a multifunctional protein primarily involved in lipid metabolism and immune regulation (Kamboh and Mehdi, 1998). Hoekstra et al. identified a novel variant in the APOH gene through a GWAS involving 293,274 White British individuals from the United Kingdom Biobank, which was significantly associated with increased Lp(a) levels. The variant rs8178824 in APOH showed a strong association, even after adjusting for known variants in the LPA region (Hoekstra et al., 2021). Masson et al. conducted a systematic review to investigate the association between elevated Lp(a) levels and AF (Masson et al., 2023). Some studies found no significant association between Lp(a) levels and AF, while others reported both positive and inverse relationships. For instance, a Chinese study showed an inverse association between Lp(a) levels and AF, while other studies found no significant link or even a positive association (Volgman et al., 2024; Yang et al., 2023). Therefore, the causal relationship between APOH and AF remains controversial, and larger-scale studies are needed to further substantiate this.
While our multi-omics SMR approach is robust, several limitations should be noted. The population-specific nature of the datasets used, primarily of European descent, may limit the generalizability of our findings to other ethnic groups. Future research will aim to replicate our findings in more diverse populations to enhance the generalizability of the therapeutic targets identified. The current study was constrained by the limited availability of OS-related proteins within the pQTL dataset, preventing a comprehensive exploration of the causal relationship between oxidative stress proteins and AF risk. Although we conducted tissue-specific analyses of candidate genes using databases derived from the atrial appendage, the genetic data obtained from circulating blood may not fully represent the status of OS-related genes in myocardial tissue. Additionally, our analysis was restricted to cis-eQTL and cis-mQTL data for OS-related genes, leaving the potential influence of trans-eQTL and trans-mQTL data on the regulatory network largely unexplored. The cross-sectional nature of the data used limits our ability to observe the progression of AF over time, which is vital for understanding the disease’s development and the long-term effects of potential genetic risk factors. Moreover, functional validation of our findings through experimental studies remains necessary. Considering the multifactorial influences on OS gene expression, we anticipate that integrating data across multiple molecular levels with GWAS data could yield novel insights in future research.
Conclusion
Our study identifies TTN as key OS-related genes with causal roles in AF, providing novel insights into AF pathogenesis and potential therapeutic targets. The integration of genetic, epigenetic, and proteomic data offers a powerful approach to uncovering the complex molecular underpinnings of AF, paving the way for precision medicine strategies in AF management.
Data availability statement
The original contributions presented in the study are publicly available. This data can be found here: Atrial fibrillation from Nielsen JB: https://gwas.mrcieu.ac.uk/datasets/ebi-a-GCST006414/; Atrial fibrillation from FinnGen: https://storage.googleapis.com/finngen-public-data-r10/summary_stats/finngen_R10_I9_AF.gz; Decode 2021: Large-scale integration of the plasma proteome with genetics and disease | Nature Genetics: https://www.nature.com/articles/s41588-021-00978-w; UKB-PPP: Plasma proteomic associations with genetics and health in the UK Biobank | Nature https://www.nature.com/articles/s41586-023-06592-6; Gudjonsson: A genome-wide association study of serum proteins reveals shared loci with common diseases | Nature Communications: https://www.nature.com/articles/s41467-021-27850-z; GTEx: GTEx Consortium (2020): https://gtexportal.org/home/; Data for SMR was downloaded from: https://yanglab.westlake.edu.cn/software/smr/#eQTLsummarydata; mQTL summary data: Identification of 55,000 Replicated DNA Methylation QTL | Scientific Reports (nature.com): https://www.nature.com/articles/s41598-018-35871-w; Data for SMR was downloaded from: https://yanglab.westlake.edu.cn/software/smr/#mQTLsummarydata; eQTL data Large-scale cis- and trans-eQTL analyses identify thousands of genetic loci and polygenic scores that regulate blood gene expression | Nature Genetics: https://www.nature.com/articles/s41588-021-00913-z; Data for SMR was downloaded from: https://yanglab.westlake.edu.cn/software/smr/#eQTLsummarydata.
Author contributions
SC: Data curation, Methodology, Writing–original draft. JS: Data curation, Writing–original draft. WW: Data curation, Visualization, Writing–original draft. ZC: Supervision, Validation, Writing – review and editing. ZY: Conceptualization, Data curation, Methodology, Writing–review and editing.
Funding
The author(s) declare that financial support was received for the research, authorship, and/or publication of this article. Zhejiang Provincial Health Department Clinical Research Application (2022KY359).
Conflict of interest
The authors declare that the research was conducted in the absence of any commercial or financial relationships that could be construed as a potential conflict of interest.
Publisher’s note
All claims expressed in this article are solely those of the authors and do not necessarily represent those of their affiliated organizations, or those of the publisher, the editors and the reviewers. Any product that may be evaluated in this article, or claim that may be made by its manufacturer, is not guaranteed or endorsed by the publisher.
Supplementary material
The Supplementary Material for this article can be found online at: https://www.frontiersin.org/articles/10.3389/fgene.2024.1447872/full#supplementary-material
References
Akhtar, M. M., Lorenzini, M., Cicerchia, M., Ochoa, J. P., Hey, T. M., Sabater Molina, M., et al. (2020). Clinical phenotypes and prognosis of dilated cardiomyopathy caused by truncating variants in the TTN gene. Circ. Heart Fail 13 (10), e006832. doi:10.1161/CIRCHEARTFAILURE.119.006832
Andreasen, L., Bertelsen, L., Ghouse, J., Lundegaard, P. R., Ahlberg, G., Refsgaard, L., et al. (2020). Early-onset atrial fibrillation patients show reduced left ventricular ejection fraction and increased atrial fibrosis. Sci. Rep. 10 (1), 10039. doi:10.1038/s41598-020-66671-w
Askarinejad, A., Esmaeili, S., Dalili, M., Biglari, A., Kohansal, E., Maleki, M., et al. (2024). Catecholaminergic polymorphic ventricular tachycardia (and seizure) caused by a novel homozygous likely pathogenic variant in CASQ2 gene. Gene 895, 148012. doi:10.1016/j.gene.2023.148012
Balan, A. I., Halațiu, V. B., and Scridon, A. (2024). Oxidative stress, inflammation, and mitochondrial dysfunction: a link between obesity and atrial fibrillation. Antioxidants (Basel) 13 (1), 117. doi:10.3390/antiox13010117
Fan, J., Cao, S., Chen, M., Yao, Q., Zhang, X., Du, S., et al. (2022). Investigating the AC079305/DUSP1 Axis as oxidative stress-related signatures and immune infiltration characteristics in ischemic stroke. Oxid. Med. Cell Longev. 2022, 8432352. doi:10.1155/2022/8432352
Ferkingstad, E., Sulem, P., Atlason, B. A., Sveinbjornsson, G., Magnusson, M. I., Styrmisdottir, E. L., et al. (2021). Large-scale integration of the plasma proteome with genetics and disease. Nat. Genet. 53 (12), 1712–1721. doi:10.1038/s41588-021-00978-w
Fomin, A., Gärtner, A., Cyganek, L., Tiburcy, M., Tuleta, I., Wellers, L., et al. (2021). Truncated titin proteins and titin haploinsufficiency are targets for functional recovery in human cardiomyopathy due to TTN mutations. Sci. Transl. Med. 13 (618), eabd3079. doi:10.1126/scitranslmed.abd3079
Gaudino, M., Di Franco, A., Rong, L. Q., Piccini, J., and Mack, M. (2023). Postoperative atrial fibrillation: from mechanisms to treatment. Eur. Heart J. 44 (12), 1020–1039. doi:10.1093/eurheartj/ehad019
Giambartolomei, C., Vukcevic, D., Schadt, E. E., Franke, L., Hingorani, A. D., Wallace, C., et al. (2014). Bayesian test for colocalisation between pairs of genetic association studies using summary statistics. PLoS Genet. 10 (5), e1004383. doi:10.1371/journal.pgen.1004383
Grzeczka, A., Graczyk, S., and Kordowitzki, P. (2023). DNA methylation and telomeres-their impact on the occurrence of atrial fibrillation during cardiac aging. Int. J. Mol. Sci. 24 (21), 15699. doi:10.3390/ijms242115699
GTEx Consortium (2020). The GTEx Consortium atlas of genetic regulatory effects across human tissues. Science. 369 (6509), 1318–1330. doi:10.1126/science.aaz1776
Gudjonsson, A., Gudmundsdottir, V., Axelsson, G. T., Gudmundsson, E. F., Jonsson, B. G., Launer, L. J., et al. (2022). A genome-wide association study of serum proteins reveals shared loci with common diseases. Nat. Commun. 13 (1), 480. doi:10.1038/s41467-021-27850-z
Hoekstra, M., Chen, H. Y., Rong, J., Dufresne, L., Yao, J., Guo, X., et al. (2021). Genome-wide association study highlights APOH as a novel locus for lipoprotein(a) levels-brief report. Arterioscler. Thromb. Vasc. Biol. 41 (1), 458–464. doi:10.1161/ATVBAHA.120.314965
Huang, Y., Shan, Y., Zhang, W., Lee, A. M., Li, F., Stranger, B. E., et al. (2023). Deciphering genetic causes for sex differences in human health through drug metabolism and transporter genes. Nat. Commun. 14 (1), 175. doi:10.1038/s41467-023-35808-6
Kamboh, M. I., and Mehdi, H. (1998). Genetics of apolipoprotein H (beta2-glycoprotein I) and anionic phospholipid binding. Lupus. 7 (Suppl. 2), S10–S13. doi:10.1177/096120339800700203
Kang, J. Y., Mun, D., Chun, Y., Park, D. S., Kim, H., Yun, N., et al. (2022). Generation of three TTN knock-out human induced pluripotent stem cell lines using CRISPR/Cas9 system. Stem Cell Res. 64, 102901. doi:10.1016/j.scr.2022.102901
Kurki, M. I., Karjalainen, J., Palta, P., Sipilä, T. P., Kristiansson, K., Donner, K. M., et al. (2023). Author Correction: FinnGen provides genetic insights from a well-phenotyped isolated population. Nature 615 (7952), E19. doi:10.1038/s41586-023-05837-8
Lazarte, J., Laksman, Z. W., Wang, J., Robinson, J. F., Dron, J. S., Leach, E., et al. (2021). Enrichment of loss-of-function and copy number variants in ventricular cardiomyopathy genes in 'lone' atrial fibrillation. Europace 23 (6), 844–850. doi:10.1093/europace/euaa421
Loescher, C. M., Hobbach, A. J., and Linke, W. A. (2022). Titin (TTN): from molecule to modifications, mechanics, and medical significance. Cardiovasc Res. 118 (14), 2903–2918. doi:10.1093/cvr/cvab328
Masson, W., Barbagelata, L., Nogueira, J. P., Corral, P., Lavalle-Cobo, A., and Romeo, F. J. (2023). Elevated lipoprotein(a) levels and atrial fibrillation: a systematic review. J. Lipid Atheroscler. 12 (3), 267–276. doi:10.12997/jla.2023.12.3.267
McRae, A. F., Marioni, R. E., Shah, S., Yang, J., Powell, J. E., Harris, S. E., et al. (2018). Identification of 55,000 replicated DNA methylation QTL. Sci. Rep. 8 (1), 17605. doi:10.1038/s41598-018-35871-w
Müller, E., Salcan, S., Bongardt, S., Barbosa, D. M., Krüger, M., and Kötter, S. (2021). E3-ligase knock down revealed differential titin degradation by autopagy and the ubiquitin proteasome system. Sci. Rep. 11 (1), 21134. doi:10.1038/s41598-021-00618-7
Ng, K., Titus, E. W., Lieve, K. V., Roston, T. M., Mazzanti, A., Deiter, F. H., et al. (2020). An international multicenter evaluation of inheritance patterns, arrhythmic risks, and underlying mechanisms of CASQ2-catecholaminergic polymorphic ventricular tachycardia. Circulation 142 (10), 932–947. doi:10.1161/CIRCULATIONAHA.120.045723
Nielsen, J. B., Thorolfsdottir, R. B., Fritsche, L. G., Zhou, W., Skov, M. W., Graham, S. E., et al. (2018). Biobank-driven genomic discovery yields new insight into atrial fibrillation biology. Nat. Genet. 50 (9), 1234–1239. doi:10.1038/s41588-018-0171-3
Noubiap, J. J., Sanders, P., Nattel, S., and Lau, D. H. (2021). Biomarkers in atrial fibrillation: pathogenesis and clinical implications. Card. Electrophysiol. Clin. 13 (1), 221–233. doi:10.1016/j.ccep.2020.10.006
Qiu, X., Hou, Q. H., Shi, Q. Y., Jiang, H. X., and Qin, S. Y. (2020). Identification of hub prognosis-associated oxidative stress genes in pancreatic cancer using integrated bioinformatics analysis. Front. Genet. 11, 595361. doi:10.3389/fgene.2020.595361
Rafaqat, S., Sharif, S., Majeed, M., Naz, S., Manzoor, F., and Rafaqat, S. (2021). Biomarkers of metabolic syndrome: role in pathogenesis and pathophysiology of atrial fibrillation. J. Atr. Fibrillation 14 (2), 20200495. doi:10.4022/jafib.20200495
Ramos-Mondragón, R., Lozhkin, A., Vendrov, A. E., Runge, M. S., Isom, L. L., and Madamanchi, N. R. (2023). NADPH oxidases and oxidative stress in the pathogenesis of atrial fibrillation. Antioxidants (Basel) 12 (10), 1833. doi:10.3390/antiox12101833
Rudaka, I., Vilne, B., Isakova, J., Kalejs, O., Gailite, L., and Rots, D. (2023). Genetic basis of early onset atrial fibrillation in patients without risk factors. J. Cardiovasc Dev. Dis. 10 (3), 104. doi:10.3390/jcdd10030104
Sagris, M., Vardas, E. P., Theofilis, P., Antonopoulos, A. S., Oikonomou, E., and Tousoulis, D. (2021). Atrial fibrillation: pathogenesis, predisposing factors, and genetics. Int. J. Mol. Sci. 23 (1), 6. doi:10.3390/ijms23010006
Santiago, C. F., Huttner, I. G., and Fatkin, D. (2021). Mechanisms of TTNtv-related dilated cardiomyopathy: insights from zebrafish models. J. Cardiovasc Dev. Dis. 8 (2), 10. doi:10.3390/jcdd8020010
Sun, B. B., Chiou, J., Traylor, M., Benner, C., Hsu, Y. H., Richardson, T. G., et al. (2023). Plasma proteomic associations with genetics and health in the United Kingdom Biobank. Nature 622 (7982), 329–338. doi:10.1038/s41586-023-06592-6
Sun, X., Huang, X., Sun, X., Chen, S., Zhang, Z., Yu, Y., et al. (2022). Oxidative stress-related lncRNAs are potential biomarkers for predicting prognosis and immune responses in patients with LUAD. Front. Genet. 13, 909797. doi:10.3389/fgene.2022.909797
Volgman, A. S., Koschinsky, M. L., Mehta, A., and Rosenson, R. S. (2024). Genetics and pathophysiological mechanisms of lipoprotein(a)-associated cardiovascular risk. J. Am. Heart Assoc. 13 (12), e033654. doi:10.1161/JAHA.123.033654
Võsa, U., Claringbould, A., Westra, H. J., Bonder, M. J., Deelen, P., Zeng, B., et al. (2021). Large-scale cis- and trans-eQTL analyses identify thousands of genetic loci and polygenic scores that regulate blood gene expression. Nat. Genet. 53 (9), 1300–1310. doi:10.1038/s41588-021-00913-z
Wang, Q., Paskevicius, T., Filbert, A., Qin, W., Kim, H. J., Chen, X. Z., et al. (2020). Phylogenetic and biochemical analysis of calsequestrin structure and association of its variants with cardiac disorders. Sci. Rep. 10 (1), 18115. doi:10.1038/s41598-020-75097-3
Yang, M., Nasr, B., Liu, J., Du, Y., and Yang, J. (2023). The association between lipoprotein(a) and atrial fibrillation: a systemic review and meta-analysis. Clin. Cardiol. 46 (8), 899–905. doi:10.1002/clc.24086
Yoneda, Z. T., Anderson, K. C., Quintana, J. A., O'Neill, M. J., Sims, R. A., Glazer, A. M., et al. (2021). Early-onset atrial fibrillation and the prevalence of rare variants in cardiomyopathy and arrhythmia genes. JAMA Cardiol. 6 (12), 1371–1379. doi:10.1001/jamacardio.2021.3370
Keywords: atrial fibrillation, oxidative stress, summary-based mendelian randomization, QTL, TTN
Citation: Chen S, Sun J, Wen W, Chen Z and Yu Z (2024) Integrative multi-omics summary-based mendelian randomization identifies key oxidative stress-related genes as therapeutic targets for atrial fibrillation and flutter. Front. Genet. 15:1447872. doi: 10.3389/fgene.2024.1447872
Received: 12 June 2024; Accepted: 29 August 2024;
Published: 18 September 2024.
Edited by:
Xiao Chang, Children’s Hospital of Philadelphia, United StatesReviewed by:
Lili Zhi, Shandong Provincial Qianfoshan Hospital, ChinaYanping Qiu, California Institute of Technology, United States
Copyright © 2024 Chen, Sun, Wen, Chen and Yu. This is an open-access article distributed under the terms of the Creative Commons Attribution License (CC BY). The use, distribution or reproduction in other forums is permitted, provided the original author(s) and the copyright owner(s) are credited and that the original publication in this journal is cited, in accordance with accepted academic practice. No use, distribution or reproduction is permitted which does not comply with these terms.
*Correspondence: Ziheng Yu, 60090@zjhu.edu.cn