- 1Faculty of Engineering and Natural Sciences, Tampere University, Tampere, Finland
- 2Natural Resources Institute Finland, Helsinki, Finland
- 3Department of Bioproducts and Biosystems, School of Chemical Engineering, Aalto University, Espoo, Finland
- 4Department of Geography, Institute of Ecology and Earth Sciences, University of Tartu, Tartu, Estonia
1 Introduction
Methanotrophic bacteria are a special group of bacteria that consume methane as their energy and carbon source. They are roughly divided into aerobic gammaproteobacterial, alphaproteobacterial and verrucomicrobial methanotrophs (that use O2 as their primary electron acceptor), and anaerobic bacteria of genus Candidatus Methylomirabilis (that use nitrite to oxidize methane) (Guerrero-Cruz et al., 2021). Methanotrophic bacteria play a crucial role in reducing the methane emissions from natural methanogenic ecosystems, like rivers, lakes and wetlands (Hanson and Hanson, 1996). They are also actively harnessed for their biotechnological potential to mitigate methane emissions from anthropogenic ecosystems (e.g., biofilters at landfills) and to convert methane in biogas and natural gas into various value-added products (e.g., single-cell protein and bioplastics) (Strong et al., 2015).
Comparative genomics of bacteria form the basis of the current bacterial taxonomy, like that of methanotrophic bacteria (Orata et al., 2018). In addition to comparative analysis, genome sequences of methanotroph isolates provide an important backbone database for taxonomic and functional analysis of the vast and constantly increasing shotgun metagenomic data, especially metagenome-assembled genomes (MAG) of putative methanotrophs, collected from environment (Buck et al., 2021; Khanongnuch et al., 2023). Furthermore, genomic data provide crucial insights into potentially novel and testable metabolisms in methanotrophic bacteria, relevant both to the understanding of environmental methane cycling and for biotechnological applications, such as fermentation, i.e., conversion of methane to organic acids and H2 (Kalyuzhnaya et al., 2013), denitrification (Kits et al., 2015), extracellular electron transfer (Zheng et al., 2020), and oxidation of alternative electron donors (Gwak et al., 2022). Genetic engineering of methanotrophs to enhance their methane consumption and bioconversion efficiencies, and to increase the range of methane-derived products, also benefit greatly from the genomic data (Henard and Guarnieri, 2018; Jeong et al., 2023).
Here, we report the genome sequence of a strain Methylomonas sp. AM2-LC, which was isolated from the water column of a boreal, humic, O2-stratified lake, located in Southern Finland. It represents a putatively novel species of Methylomonas sp., a methanotroph genus widely present in various methanogenic ecosystems (Bussmann et al., 2021; Danilova et al., 2013; Hoefman et al., 2014; Ogiso et al., 2012; Zhu et al., 2020), and an attractive methane bioconversion candidate (Patel et al., 2018; Patel et al., 2022; Tikhonova et al., 2023).
2 Value of the data
The genome of Methylomonas sp. AM2-LC can be used as a valuable resource to conduct comparative functional and taxonomic analyses among methanotroph isolates and environmental MAGs representing methanotrophic bacteria, especially Methylomonas sp. It will aid in refining the taxonomy of Methylomonas sp. and in enhancing the understanding of the metabolic capabilities of methanotrophs and their distribution in environment. More specifically, the genome can be explored for putative novel functions having biogeochemical and/or biotechnological interest, and to design genetic engineering experiments.
3 Materials and methods
3.1 Strain isolation and DNA extraction
The strain AM2-LC was isolated from water samples collected at 4.5 m depth of Lake Alinen Mustajärvi, Southern Finland, on 27 September 2022. The temperature, pH and concentrations of O2, NH4+-N, NO3− + NO2−-N and PO43--P at the time of sampling were 5.3°C, 5.54, 0.41 mg/L, 335 μg/L, <5 μg/L and 4 μg/L. When the lake water arrived at the laboratory, pre-enrichment was conducted by transferring 100 mL of the lake water to 500 mL sterile glass bottles sealed with septum and screw cap, and the headspace was replaced with 20% CH4. After ∼30 days, the pre-enriched lake water was used as an inoculum to further enrich methanotrophs. This was done by diluting the pre-enriched lake water 1:10 in the modified ammonium mineral salts (AMS) medium. The latter consists of (g/L): NH4Cl (0.03), MgSO4·7H2O (1), CaCl2·2H2O (0.2), phosphate buffer containing K2HPO4 (0.28), KH2PO4 (0.33), pH 6.6, iron (III)-EDTA (0.004), Na2MoO4·2H2O (0.00023), 0.1% (v/v) of trace element containing (g/L): CuSO4·5H2O (1), FeSO4·7H2O (0.5), ZnSO4·7H2O (0.4), H3BO3 (0.015), CoCl2·6H2O (0.05), EDTA-Na2 (0.25), MnCl·4H2O (0.02), NiCl2·6H2O (0.01), and 0.5% (v/v) vitamin solution containing (mg/L), pyridoxine hydrochloride (10), thiamine-HCl (5), riboflavin (5), nicotinic acid (5), thioctic acid (5), folic acid (2). Additionally, 1 µM La2O3 and CeCl3·7H2O were supplemented to the modified AMS medium. To inhibit fungal growth, 2.5 μg/mL of amphotericin B solution (Sigma-Aldrich Ltd.) was added to the modified AMS medium. The enrichment was conducted in serum bottles filled with ∼8% (v/v) of the modified AMS medium, and CH4, sterilized with 0.22 µm sterile syringe filter, was added to the headspace to obtain a 20:80 ratio CH4 and air. When the turbidity was observed, the enriched culture was transferred into a new bottle with fresh medium and headspace gas replenishment. The serial dilutions of 1:106 was performed until a pure culture was obtained. The culture purity was verified by streaking onto nutrient-rich agar (5 g/L tryptone, 2.5 g/L yeast extract, 1 g/L glucose, and 20 g/L agar) showing no growth and observing by a light microscope. So far, the strain AM2-LC could grow at pH 6.0, 6.6, and 6.8 in a cold room (5°C ± 1.5 °C) and room temperature (20°C ± 2 °C). The growth was not observed in a liquid medium when ammonium was replaced with nitrate as a nitrogen source. The isolate morphology was observed by microscopy as rod shape (0.9–2.5 µm in length and 0.8–1.3 µm in width) (Supplementary Figure S1 in Supplementary Material S1). Furthermore, the strain was able to grow with 2% CH4 in the headspace (Supplementary Figure S2C in Supplementary Material S1). The culture is available at the laboratory at Tampere University, Finland. To preserve it, the cell pellets were resuspended in 1XPBS (pH 7.4) containing 7% DMSO and stored at −80 °C.
Genomic DNA was extracted using GeneJET Genomic DNA Purification Kit and quantified using a Qubit 3.0 Fluorometer and a dsDNA HS Assay Kit (Thermo Fisher Scientific, Waltham, MA, United States).
3.2 16S rRNA gene sequencing and phylogenetic analysis
Using the identification service offered by Macrogen (Amsterdam, Netherlands), the 16S rRNA genes of the strain AM2-LC were amplified from the genomic DNA (gDNA) using primers 27F (AGAGTTTGATCMTGGCTCAG) and 1492R (TACGGYTACCTTGTTACGACTT) and sequenced using primer pairs 785F (GGATTAGATACCCTGGTA) and 907R (CCGTCAATTCMTTTRAGTTT). The 16S rRNA gene sequence alignment with reference sequences (SINA aligner v.1.2.12) and the phylogenetic tree analysis (FastTree v. 2.1.11, ML model: Generalized Time-Reversible) was performed using the Silva Alignment, Classification and Tree Service (Pruesse et al., 2012).
3.3 Genome sequencing and analysis
The gDNA sequencing, including library preparation and sequencing of both long (PacBio SMRT, CCS sequencing mode, PacBio Revio) and short reads [Illumina NovaSeq X (PE150)] was performed as a service provided by Biomarker Technologies (BMK) GmbH. The sequencing facility also provided bioinformatic services such as filtering of long (reads with length <2 kb removed) and short reads [fastp v0.23.2, (Chen et al., 2018), to remove adapter and low quality reads], genome assembly [Hifiasm v. 0.14, (Cheng et al., 2021; Cheng et al., 2022)], genome assembly improvement [Pilon v 1.22, (Walker et al., 2014)], and genome cyclizing [circlator v. 1.5.5, (Hunt et al., 2015)]. The genome completeness and contamination was assessed using checkM (v1.2.2, Methylococcales. ms marker set) (Parks et al., 2015).
The protein sequences, repetitive sequences, tRNAs, rRNAs, CRISPR regions, genomic islands, prophages, and biosynthetic gene clusters (BGC) were predicted using Prodigal (v. 2.6) (Hyatt et al., 2010), RepeatMasker (v4.0.5) (Tarailo-Graovac and Chen, 2009), tRNAscan-SE (v2.0) (Chan and Lowe, 2019), Infernal (v1.1.3) (Nawrocki and Eddy, 2013), CRT (v1.2) (Bland et al., 2007), IslandPath-DIMOB (v0.2) (Bertelli and Brinkman, 2018), PhiSpy (v2.3) (Akhter et al., 2012), and antiSMASH (v5.0.0) (Blin et al., 2019), respectively. The genome-wide phylogenetic tree was built from protein alignments generated in PhyloPhlAn (v. 3.0.67; PhyloPhlAn database including 400 universal marker genes and “-diversity low” - argument) (Segata et al., 2013; Asnicar et al., 2020) using the maximum-likelihood algorithm (PROTCATLG − model) with 100 bootstrap replicates in RAxML (v. 8.2.12) (Stamatakis, 2014). Average nucleotide identities (ANI) with reference genomes were calculated using ANI calculator (http://enve-omics.ce.gatech.edu/ani/, accessed on April 2024) (Goris et al., 2007). Digital DNA-DNA hybridization (dDDH) comparisons with reference genomes were done using the Type Strain Genome Server (TYGS) online service (https://tygs.dsmz.de/, accessed on April 2024) (Meier-Kolthoff and Göker, 2019). The functional annotation was performed via BLAST search against NCBI´s Nr, eggNOG (Powell et al., 2014), GO (Ashburner et al., 2000), Pfam (Finn et al., 2014), SwissProt and TrEMBL databases (Bairoch and Apweiler, 1996). KofamKOALA (https://www.genome.jp/tools/kofamkoala/, accessed on April 2024) was used to search the predicted genes against the KEGG database (Kanehisa et al., 2004; Aramaki et al., 2020).
3.4 Preliminary data analysis
The statistics of de novo assembly and genome characteristics are reported in Table 1. The genome, with full length of 5394081 bp and G + C content of 42.7%, consisted of three contigs of which one was the chromosome (4971665 bp) and two were plasmids (288757 bp and 133659 bp) (Supplementary Figure S3 in Supplementary Material S1). The genome was of very high quality as judged by the high completeness and low contamination estimates (Table 1) (Bowers et al., 2017). Furthermore, the genome contained 4,933 coding sequences, 9 rRNA and 48 tRNA genes, 14 CRISPR regions, 24 genomic island regions, 10 prophages, 6 biosynthetic gene clusters, and had 6,780 bp of repetitive sequences (Table 1). See further results on the analysis of these genome characteristics (including predicted protein sequences) in Supplementary Material S2.
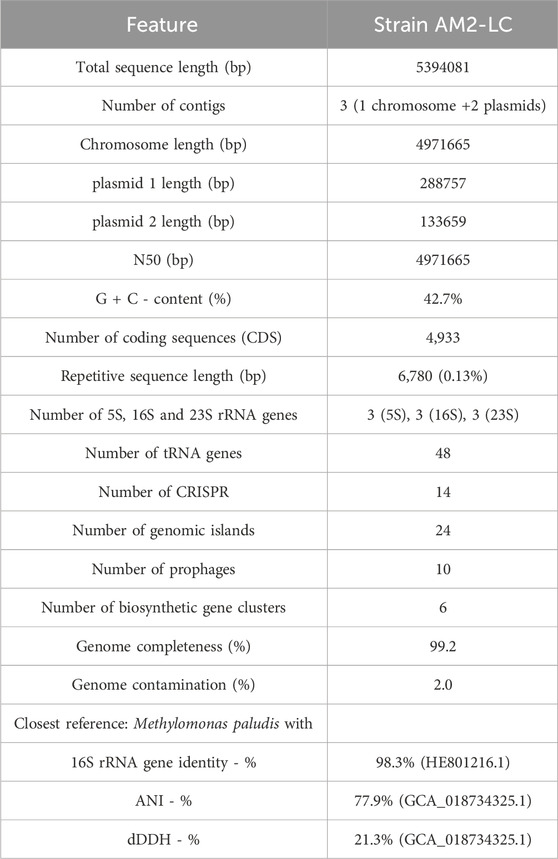
Table 1. Statistics of de novo genome assembly, genome characteristics and taxonomically closest reference strain of Methylomonas sp. AM2-LC.
The strain AM2-LC was most closely related with M. paludis (Danilova et al., 2013; Rissanen et al., 2021) with 98.3% similarity in the 16S rRNA gene comparisons and with 77.9% ANI and 21.3% dDDH in the genome-level comparisons (Table 1). It also positioned closest to M. paludis in phylogenetic and phylogenomic tree analyses (Supplementary Figure S4 in Supplementary Material S1). The respective similarities with representatives of Methylomonas sp. generally varied (min-max) 94.3%–98.3% (16S rRNA genes), 76.3%–77.9% (ANI) and 18.6%–21.3% (dDDH). Given these being below the widely used thresholds to delineate unique species, 98.65%, 95%, and 70%, for 16S rRNA genes, ANI, and dDDH, respectively (Goris et al., 2007; Auch et al., 2010; Meier-Kolthoff et al., 2013; Kim et al., 2014; Chun et al., 2018; Orata et al., 2018), the strain AM2-LC very likely represents a novel species of genus Methylomonas.
According to the preliminary annotation analysis (Figure 1), the strain AM2-LC’s genome contained particulate methane monooxygenases (pmoCAB) for methane conversion into methanol, while soluble methane monooxygenases (mmoXYBZDC) were absent. In addition, the strain encoded the pxm operon (pxmABC), i.e., a copper membrane monooxygenase of unknown function (Tavormina et al., 2011). The genome contained both calcium- (mxaLKCAIGJFD) and lanthanide-dependent (xoxF) methanol dehydrogenases for converting methanol to formaldehyde. Genes involved in tetrahydromethanopterin (H4MPT)-mediated pathway, catalyzing the conversion of formaldehyde into formate, were also present in the genome. The genome also contained genes encoding the RuMP pathway [for carbon (formaldehyde) assimilation], the oxidative TCA cycle, and Entner-Doudoroff and Embden-Meyerhof-Parnas pathways for energy conservation (Figure 1). Similar to the closely related strain M. paludis S2AM, the strain AM2-LC can potentially convert methane into industrially important organic acids, i.e., formic acid, lactic acid, acetic acid, and succinic acid (Figure 1) (Khanongnuch et al., 2023; Strong et al., 2015). Furthermore, the genome included genes encoding N2 fixation (nitrogenase, nifKDH), assimilation of nitrate (nitrate reductase, nasA and nitrite reductase, nirDB), oxidation of hydroxylamine into nitrite (hydroxylamine dehydrogenase, hao). Interestingly, the strain contains the genetic potential to oxidize other toxic compounds commonly found in biogas, including the conversion of hydrogen sulfide to sulfur (sulfide dehydrogenase, fccBA; sulfide-quinone oxidoreductase, sqr) and conversion of methylmercaptan into formaldehyde and hydrogen sulfide (methylmercaptan (MM)-oxidase) (Figure 1). The strain is likely incapable of carotenoid biosynthesis as lacking the relevant functional genes. See the detailed results on the functional annotation analyses in Supplementary Material S3. The results of COG annotation analysis are also visualized in Supplementary Figure S3 in Supplementary Material S1.
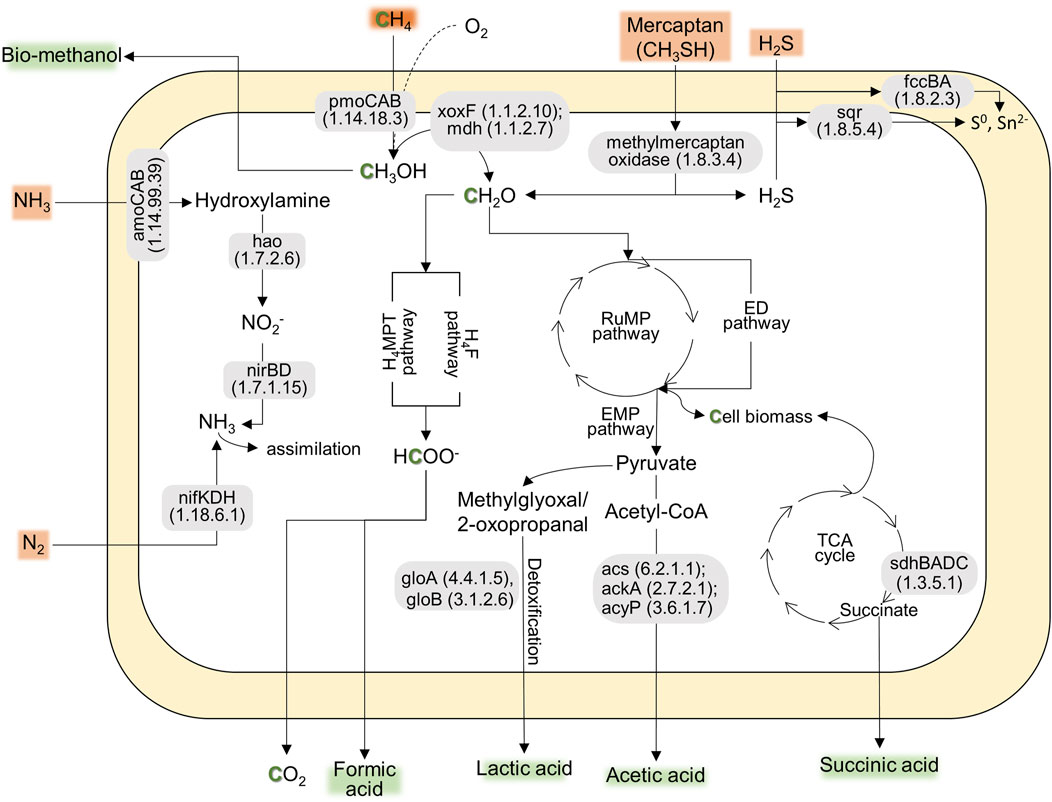
Figure 1. The predicted pathways indicating the genetic potential of AM2-LC for CH4 conversion into CH4-derived compounds (e.g., methanol and organic acids) and nitrogen fixation, contributing to the ecosystem function and bioconversion of CH4. Additionally, the strain shows genetic potential for tolerating toxic compounds commonly found in biogas, including genes for converting hydrogen sulfide, mercaptan, and NH3. The genes present in the pathway are based on KofamKOALA (KEGG Orthology and Function Annotation by KEGG Orthology And Links Annotation) (Supplementary Material S3).
Data availability statement
All data presented in this study is publicly available. The raw sequence data is deposited in NCBI’s SRA under the Bioproject PRJNA1112122 and Biosample SAMN41414985. The assembled genome has been deposited at NCBI’s Genbank under accession number CP157005 for chromosome, CP157006 for plasmid 1, and CP157007 for plasmid 2. The independently sequenced 16S rRNA gene sequence is deposited in Genbank under accession number PP800278. Data on genome characteristics (incl. predicted protein sequences) and functional annotation analyses are found in Supplementary Material S2, S3.
Author contributions
AR: Conceptualization, Formal Analysis, Funding acquisition, Investigation, Project administration, Visualization, Writing–original draft, Writing–review and editing. RM: Conceptualization, Funding acquisition, Investigation, Writing–review and editing. RK: Conceptualization, Formal Analysis, Investigation, Visualization, Writing–review and editing.
Funding
The author(s) declare that financial support was received for the research, authorship, and/or publication of this article. The study was funded by Academy of Finland (Project No. 346751 and 353750 for AR and RK, and 346983 for RM).
Acknowledgments
The authors thank Prof. Mette Marianne Svenning and Anne Grethe Hestnes, The Arctic University of Norway, Tromsø, Norway, for their guidance and support in methanotroph isolation and cultivation, as well as the staff at Lammi Biological Station (Finland) for their support in sampling.
Conflict of interest
The authors declare that the research was conducted in the absence of any commercial or financial relationships that could be construed as a potential conflict of interest.
Publisher’s note
All claims expressed in this article are solely those of the authors and do not necessarily represent those of their affiliated organizations, or those of the publisher, the editors and the reviewers. Any product that may be evaluated in this article, or claim that may be made by its manufacturer, is not guaranteed or endorsed by the publisher.
Supplementary material
The Supplementary Material for this article can be found online at: https://www.frontiersin.org/articles/10.3389/fgene.2024.1440435/full#supplementary-material
References
Akhter, S., Aziz, R. K., and Edwards, R. A. (2012). PhiSpy: a novel algorithm for finding prophages in bacterial genomes that combines similarity- and composition-based strategies. Nucleic Acids Res. 40, e126. doi:10.1093/nar/gks406
Aramaki, T., Blanc-Mathieu, R., Endo, H., Ohkubo, K., Kanehisa, M., Goto, S., et al. (2020). KofamKOALA: KEGG Ortholog assignment based on profile HMM and adaptive score threshold. Bioinformatics 36, 2251–2252. doi:10.1093/bioinformatics/btz859
Ashburner, M., Ball, C. A., Blake, J. A., Botstein, D., Butler, H., Cherry, J. M., et al. (2000). Gene ontology: tool for the unification of biology. The Gene Ontology Consortium. Nat. Genet. 25, 25–29. doi:10.1038/75556
Asnicar, F., Thomas, A. M., Beghini, F., Mengoni, C., Manara, S., Manghi, P., et al. (2020). Precise phylogenetic analysis of microbial isolates and genomes from metagenomes using PhyloPhlAn 3.0. Nat. Commun. 11, 2500. doi:10.1038/s41467-020-16366-7
Auch, A. F., von Jan, M., Klenk, H.-P., and Göker, M. (2010). Digital DNA-DNA hybridization for microbial species delineation by means of genome-to-genome sequence comparison. Stand. Genomic Sci. 2, 117–134. doi:10.4056/sigs.531120
Bairoch, A., and Apweiler, R. (1996). The SWISS-PROT protein sequence data bank and its new supplement TREMBL. Nucleic Acids Res. 24, 21–25. doi:10.1093/nar/24.1.21
Bertelli, C., and Brinkman, F. S. L. (2018). Improved genomic island predictions with IslandPath-DIMOB. Bioinformatics 34, 2161–2167. doi:10.1093/bioinformatics/bty095
Bland, C., Ramsey, T. L., Sabree, F., Lowe, M., Brown, K., Kyrpides, N. C., et al. (2007). CRISPR Recognition Tool (CRT): a tool for automatic detection of clustered regularly interspaced palindromic repeats. BMC Bioinforma. 8, 209. doi:10.1186/1471-2105-8-209
Blin, K., Shaw, S., Steinke, K., Villebro, R., Ziemert, N., Lee, S. Y., et al. (2019). antiSMASH 5.0: updates to the secondary metabolite genome mining pipeline. Nucleic Acids Res. 47, W81-W87–W87. doi:10.1093/nar/gkz310
Bowers, R. M., Kyrpides, N. C., Stepanauskas, R., Harmon-Smith, M., Doud, D., Reddy, T. B. K., et al. (2017). Minimum information about a single amplified genome (MISAG) and a metagenome-assembled genome (MIMAG) of bacteria and archaea. Nat. Biotechnol. 35, 725–731. doi:10.1038/nbt.3893
Buck, M., Garcia, S. L., Fernandez, L., Martin, G., Martinez-Rodriguez, G. A., Saarenheimo, J., et al. (2021). Comprehensive dataset of shotgun metagenomes from oxygen stratified freshwater lakes and ponds. Sci. Data 8, 131. doi:10.1038/s41597-021-00910-1
Bussmann, I., Horn, F., Hoppert, M., Klings, K.-W., Saborowski, A., Warnstedt, J., et al. (2021). Methylomonas albis sp. nov. and Methylomonas fluvii sp. nov.: two cold-adapted methanotrophs from the River Elbe and emended description of the species Methylovulum psychrotolerans. Syst. Appl. Microbiol. 44, 126248. doi:10.1016/j.syapm.2021.126248
Chan, P. P., and Lowe, T. M. (2019). “tRNAscan-SE: searching for tRNA genes in genomic sequences,” in Gene prediction: methods and protocols. Editor M. Kollmar (New York, NY: Springer New York), 1–14. doi:10.1007/978-1-4939-9173-0_1
Chen, S., Zhou, Y., Chen, Y., and Gu, J. (2018). fastp: an ultra-fast all-in-one FASTQ preprocessor. Bioinformatics 34, i884–i890. doi:10.1093/bioinformatics/bty560
Cheng, H., Concepcion, G. T., Feng, X., Zhang, H., and Li, H. (2021). Haplotype-resolved de novo assembly using phased assembly graphs with hifiasm. Nat. Methods 18, 170–175. doi:10.1038/s41592-020-01056-5
Cheng, H., Jarvis, E. D., Fedrigo, O., Koepfli, K.-P., Urban, L., Gemmell, N. J., et al. (2022). Haplotype-resolved assembly of diploid genomes without parental data. Nat. Biotechnol. 40, 1332–1335. doi:10.1038/s41587-022-01261-x
Chun, J., Oren, A., Ventosa, A., Christensen, H., Arahal, D. R., da Costa, M. S., et al. (2018). Proposed minimal standards for the use of genome data for the taxonomy of prokaryotes. Int. J. Syst. Evol. Microbiol. 68, 461–466. doi:10.1099/ijsem.0.002516
Danilova, O. V., Kulichevskaya, I. S., Rozova, O. N., Detkova, E. N., Bodelier, P. L. E., Trotsenko, Y. A., et al. (2013). Methylomonas paludis sp. nov., the first acid-tolerant member of the genus Methylomonas, from an acidic wetland. Int. J. Syst. Evol. Microbiol. 63, 2282–2289. doi:10.1099/ijs.0.045658-0
Finn, R. D., Bateman, A., Clements, J., Coggill, P., Eberhardt, R. Y., Eddy, S. R., et al. (2014). Pfam: the protein families database. Nucleic Acids Res. 42, D222–D230. doi:10.1093/nar/gkt1223
Goris, J., Konstantinidis, K. T., Klappenbach, J. A., Coenye, T., Vandamme, P., and Tiedje, J. M. (2007). DNA–DNA hybridization values and their relationship to whole-genome sequence similarities. Int. J. Syst. Evol. Microbiol. 57, 81–91. doi:10.1099/ijs.0.64483-0
Guerrero-Cruz, S., Vaksmaa, A., Horn, M. A., Niemann, H., Pijuan, M., and Ho, A. (2021). Methanotrophs: discoveries, environmental relevance, and a perspective on current and future applications. Front. Microbiol. 12, 678057. doi:10.3389/fmicb.2021.678057
Gwak, J.-H., Awala, S. I., Nguyen, N.-L., Yu, W.-J., Yang, H.-Y., von Bergen, M., et al. (2022). Sulfur and methane oxidation by a single microorganism. Proc. Natl. Acad. Sci. U. S. A. 119, e2114799119. doi:10.1073/pnas.2114799119
Hanson, R. S., and Hanson, T. E. (1996). Methanotrophic bacteria. Microbiol. Rev. 60, 439–471. doi:10.1128/mr.60.2.439-471.1996
Henard, C. A., and Guarnieri, M. T. (2018). “Metabolic engineering of methanotrophic bacteria for industrial biomanufacturing,” in Methane biocatalysis: paving the way to sustainability. Editors M. G. Kalyuzhnaya, and X.-H. Xing (Cham: Springer International Publishing), 117–132. doi:10.1007/978-3-319-74866-5_8
Hoefman, S., Heylen, K., and De Vos, P. (2014). Methylomonas lenta sp. nov., a methanotroph isolated from manure and a denitrification tank. Int. J. Syst. Evol. Microbiol. 64, 1210–1217. doi:10.1099/ijs.0.057794-0
Hunt, M., Silva, N. D., Otto, T. D., Parkhill, J., Keane, J. A., and Harris, S. R. (2015). Circlator: automated circularization of genome assemblies using long sequencing reads. Genome Biol. 16, 294. doi:10.1186/s13059-015-0849-0
Hyatt, D., Chen, G.-L., Locascio, P. F., Land, M. L., Larimer, F. W., and Hauser, L. J. (2010). Prodigal: prokaryotic gene recognition and translation initiation site identification. BMC Bioinforma. 11, 119. doi:10.1186/1471-2105-11-119
Jeong, J., Kim, T. H., Jang, N., Ko, M., Kim, S. K., Baek, J. I., et al. (2023). A highly efficient and versatile genetic engineering toolkit for a methanotroph-based biorefinery. Chem. Eng. J. 453, 139911. doi:10.1016/j.cej.2022.139911
Kalyuzhnaya, M. G., Yang, S., Rozova, O. N., Smalley, N. E., Clubb, J., Lamb, A., et al. (2013). Highly efficient methane biocatalysis revealed in a methanotrophic bacterium. Nat. Commun. 4, 2785. doi:10.1038/ncomms3785
Kanehisa, M., Goto, S., Kawashima, S., Okuno, Y., and Hattori, M. (2004). The KEGG resource for deciphering the genome. Nucleic Acids Res. 32, D277–D280. doi:10.1093/nar/gkh063
Khanongnuch, R., Mangayil, R., and Rissanen, A. J. (2023). Conversion of methane to organic acids is a widely found trait among gammaproteobacterial methanotrophs of freshwater lake and pond ecosystems. Microbiol. Spectr. 11, e0174223. doi:10.1128/spectrum.01742-23
Kim, M., Oh, H.-S., Park, S.-C., and Chun, J. (2014). Towards a taxonomic coherence between average nucleotide identity and 16S rRNA gene sequence similarity for species demarcation of prokaryotes. Int. J. Syst. Evol. Microbiol. 64, 346–351. doi:10.1099/ijs.0.059774-0
Kits, K. D., Klotz, M. G., and Stein, L. Y. (2015). Methane oxidation coupled to nitrate reduction under hypoxia by the Gammaproteobacterium Methylomonas denitrificans, sp. nov. type strain FJG1. Environ. Microbiol. 17, 3219–3232. doi:10.1111/1462-2920.12772
Meier-Kolthoff, J. P., Auch, A. F., Klenk, H.-P., and Göker, M. (2013). Genome sequence-based species delimitation with confidence intervals and improved distance functions. BMC Bioinforma. 14, 60. doi:10.1186/1471-2105-14-60
Meier-Kolthoff, J. P., and Göker, M. (2019). TYGS is an automated high-throughput platform for state-of-the-art genome-based taxonomy. Nat. Commun. 10, 2182. doi:10.1038/s41467-019-10210-3
Nawrocki, E. P., and Eddy, S. R. (2013). Infernal 1.1: 100-fold faster RNA homology searches. Bioinformatics 29, 2933–2935. doi:10.1093/bioinformatics/btt509
Ogiso, T., Ueno, C., Dianou, D., Huy, T. V., Katayama, A., Kimura, M., et al. (2012). Methylomonas koyamae sp. nov., a type I methane-oxidizing bacterium from floodwater of a rice paddy field. Int. J. Syst. Evol. Microbiol. 62, 1832–1837. doi:10.1099/ijs.0.035261-0
Orata, F. D., Meier-Kolthoff, J. P., Sauvageau, D., and Stein, L. Y. (2018). Phylogenomic analysis of the gammaproteobacterial methanotrophs (order Methylococcales) calls for the reclassification of members at the genus and species levels. Front. Microbiol. 9, 3162. doi:10.3389/fmicb.2018.03162
Parks, D. H., Imelfort, M., Skennerton, C. T., Hugenholtz, P., and Tyson, G. W. (2015). CheckM: assessing the quality of microbial genomes recovered from isolates, single cells, and metagenomes. Genome Res. 25, 1043–1055. doi:10.1101/gr.186072.114
Patel, S. K. S., Gupta, R. K., Kalia, V. C., and Lee, J.-K. (2022). Synthetic design of methanotroph co-cultures and their immobilization within polymers containing magnetic nanoparticles to enhance methanol production from wheat straw-based biogas. Bioresour. Technol. 364, 128032. doi:10.1016/j.biortech.2022.128032
Patel, S. K. S., Kumar, V., Mardina, P., Li, J., Lestari, R., Kalia, V. C., et al. (2018). Methanol production from simulated biogas mixtures by co-immobilized Methylomonas methanica and Methylocella tundrae. Bioresour. Technol. 263, 25–32. doi:10.1016/j.biortech.2018.04.096
Powell, S., Forslund, K., Szklarczyk, D., Trachana, K., Roth, A., Huerta-Cepas, J., et al. (2014). eggNOG v4.0: nested orthology inference across 3686 organisms. Nucleic Acids Res. 42, D231–D239. doi:10.1093/nar/gkt1253
Pruesse, E., Peplies, J., and Glöckner, F. O. (2012). SINA: accurate high-throughput multiple sequence alignment of ribosomal RNA genes. Bioinformatics 28, 1823–1829. doi:10.1093/bioinformatics/bts252
Rissanen, A. J., Mangayil, R., Svenning, M. M., and Khanongnuch, R. (2021). Draft genome sequence data of methanotrophic Methylovulum psychrotolerans strain S1L and Methylomonas paludis strain S2AM isolated from hypoxic water column layers of boreal lakes. Data Brief 38, 107364. doi:10.1016/j.dib.2021.107364
Segata, N., Börnigen, D., Morgan, X. C., and Huttenhower, C. (2013). PhyloPhlAn is a new method for improved phylogenetic and taxonomic placement of microbes. Nat. Commun. 4, 2304. doi:10.1038/ncomms3304
Stamatakis, A. (2014). RAxML version 8: a tool for phylogenetic analysis and post-analysis of large phylogenies. Bioinforma. Oxf. Engl. 30, 1312–1313. doi:10.1093/bioinformatics/btu033
Strong, P. J., Xie, S., and Clarke, W. P. (2015). Methane as a resource: can the methanotrophs add value? Environ. Sci. and Technol. 49, 4001–4018. doi:10.1021/es504242n
Tarailo-Graovac, M., and Chen, N. (2009). Using RepeatMasker to identify repetitive elements in genomic sequences. Curr. Protoc. Bioinforma. 25, Unit 4.10–4. doi:10.1002/0471250953.bi0410s25
Tavormina, P. L., Orphan, V. J., Kalyuzhnaya, M. G., Jetten, M. S. M., and Klotz, M. G. (2011). A novel family of functional operons encoding methane/ammonia monooxygenase-related proteins in gammaproteobacterial methanotrophs. Environ. Microbiol. Rep. 3, 91–100. doi:10.1111/j.1758-2229.2010.00192.x
Tikhonova, E. N., Suleimanov, R. Z., Miroshnikov, K. K., Oshkin, I. Y., Belova, S. E., Danilova, O. V., et al. (2023). Methylomonas rapida sp. nov., a novel species of fast-growing, carotenoid-producing obligate methanotrophs with high biotechnological potential. Syst. Appl. Microbiol. 46, 126398. doi:10.1016/j.syapm.2023.126398
Walker, B. J., Abeel, T., Shea, T., Priest, M., Abouelliel, A., Sakthikumar, S., et al. (2014). Pilon: an integrated tool for comprehensive microbial variant detection and genome assembly improvement. PLOS ONE 9, e112963. doi:10.1371/journal.pone.0112963
Zheng, Y., Wang, H., Liu, Y., Zhu, B., Li, J., Yang, Y., et al. (2020). Methane-dependent mineral reduction by aerobic methanotrophs under hypoxia. Environ. Sci. and Technol. Lett. 7, 606–612. doi:10.1021/acs.estlett.0c00436
Keywords: methanotroph, greenhouse gas, methane bioconversion, Methylomonas, biogas, natural gas, climate change, lake
Citation: Rissanen AJ, Mangayil R and Khanongnuch R (2024) Genome of Methylomonas sp. AM2-LC, representing a methanotrophic bacterial species isolated from water column of a boreal, oxygen-stratified lake. Front. Genet. 15:1440435. doi: 10.3389/fgene.2024.1440435
Received: 29 May 2024; Accepted: 19 August 2024;
Published: 30 August 2024.
Edited by:
Richard John Edwards, University of Western Australia, AustraliaReviewed by:
Monali C. Rahalkar, Agharkar Research Institute, IndiaSanjay Kumar Singh Patel, Hemwati Nandan Bahuguna Garhwal University, India
Copyright © 2024 Rissanen, Mangayil and Khanongnuch. This is an open-access article distributed under the terms of the Creative Commons Attribution License (CC BY). The use, distribution or reproduction in other forums is permitted, provided the original author(s) and the copyright owner(s) are credited and that the original publication in this journal is cited, in accordance with accepted academic practice. No use, distribution or reproduction is permitted which does not comply with these terms.
*Correspondence: Antti J. Rissanen, YW50dGkucmlzc2FuZW5AdHVuaS5maQ==